File size: 6,243 Bytes
1c0f54c 017791b 1c0f54c 017791b 1c0f54c 017791b 1c0f54c 017791b 1c0f54c 017791b 1c0f54c 017791b 5634da1 9fe5c93 1c0f54c 017791b 1c0f54c 017791b 1c0f54c 017791b 1c0f54c 017791b 1c0f54c 017791b 1c0f54c 017791b 1c0f54c d65ae07 017791b 1c0f54c 017791b 1c0f54c 017791b 1c0f54c 017791b 1c0f54c 017791b 1c0f54c 017791b 1c0f54c 017791b 1c0f54c 017791b 1c0f54c 017791b 1c0f54c 017791b 1c0f54c 017791b 1c0f54c 017791b 1c0f54c 017791b 1c0f54c 017791b 1c0f54c 017791b 1c0f54c 017791b 1c0f54c 017791b 1c0f54c 017791b 1c0f54c 017791b |
1 2 3 4 5 6 7 8 9 10 11 12 13 14 15 16 17 18 19 20 21 22 23 24 25 26 27 28 29 30 31 32 33 34 35 36 37 38 39 40 41 42 43 44 45 46 47 48 49 50 51 52 53 54 55 56 57 58 59 60 61 62 63 64 65 66 67 68 69 70 71 72 73 74 75 76 77 78 79 80 81 82 83 84 85 86 87 88 89 90 91 92 93 94 95 96 97 98 99 100 101 102 103 104 105 106 107 108 109 110 111 112 |
---
license: gemma
library_name: transformers
pipeline_tag: text-generation
tags:
- conversational
base_model:
- google/gemma-2-2b-jpn-it
---
# [AXCXEPT/EZO-gemma-2-2b-jpn-it]

## [Model Information]
This model is based on google/gemma-2-2b-jpn-it, enhanced with multiple tuning techniques to improve its general performance. While it excels in Japanese language tasks, it's designed to meet diverse needs globally.
google/gemma-2-2b-jpn-itをベースとして、複数のチューニング手法を採用のうえ、汎用的に性能を向上させたモデルです。日本語タスクに優れつつ、世界中の多様なニーズに応える設計となっています。
### [Benchmark Results]

**Terms of Use**: [Terms](https://www.kaggle.com/models/google/gemma/license/consent/verify/huggingface?returnModelRepoId=google/gemma-2-2b-it)
This model is based on google/gemma-2-2b-jpn-it and is subject to the Gemma Terms of Use. For detailed information, please refer to the official Gemma license page.
このモデルはgoogle/gemma-2-2b-jpn-itをベースにしており、Gemmaの利用規約に従います。詳細については、Gemmaの公式ライセンスページをご参照ください。
### [Usage]
Here are some code snippets to quickly get started with the model. First, run:
`pip install -U transformers accelerate`
Then, copy the snippet from the relevant section for your use case.
以下に、モデルの実行を素早く開始するためのコードスニペットをいくつか紹介します。
まず、
`pip install -U transformers`
を実行し、使用例に関連するセクションのスニペットをコピーしてください。
### [Chat Template]
```py
from transformers import AutoTokenizer, AutoModelForCausalLM
model_id = "AXCXEPT/EZO-gemma-2-2b-jpn-it"
tokenizer = AutoTokenizer.from_pretrained(model_id)
model = AutoModelForCausalLM.from_pretrained(
model_id,
device_map="auto",
)
messages = [
{"role": "user", "content": f"""
以下の記事から日付をすべて抽出せよ。
あすから今冬一番の寒波 “10年に一度の低温に” 大雪に備えを
この冬一番の非常に強い寒気が流れ込むため、24日から北日本から西日本の日本海側で大雪や猛吹雪となるほか、ふだん雪の少ない太平洋側の平地でも大雪となるおそれがあります。
全国的に10年に一度程度の低い気温になる見込みで、路面や水道管の凍結による被害が出るおそれもあります。予定の変更を検討するなど、大雪や低温への備えを進めてください。
気象庁によりますと、日本付近は24日から26日 木曜日ごろにかけて冬型の気圧配置が強まり、北日本から西日本の上空には、この冬一番の非常に強い寒気が流れ込む見込みです。
このため、北日本から西日本の日本海側を中心に大雪や猛吹雪となり、短時間で積雪が急激に増えるおそれがあります。
"""},
]
input_ids = tokenizer.apply_chat_template(messages, return_tensors="pt", return_dict=True).to("cuda")
outputs = model.generate(**input_ids, max_new_tokens=512)
print(tokenizer.decode(outputs[0]))
```
### [Template]
```
<bos><start_of_turn>user
Write a hello world program<end_of_turn>
<start_of_turn>model
XXXXXX<end_of_turn><eos>
```
### [Model Data]
#### Training Dataset]
We extracted high-quality data from Japanese Wikipedia and FineWeb to create instruction data. Our innovative training approach allows for performance improvements across various languages and domains, making the model suitable for global use despite its focus on Japanese data.
日本語のWikiデータおよび、FineWebから良質なデータのみを抽出し、Instructionデータを作成しました。このモデルでは日本語に特化させていますが、世界中のどんなユースケースでも利用可能なアプローチです。
https://huggingface.co/datasets/legacy-datasets/wikipedia
https://huggingface.co/datasets/HuggingFaceFW/fineweb
#### Data Preprocessing
We used a plain instruction tuning method to train the model on exemplary responses. This approach enhances the model's ability to understand and generate high-quality responses across various languages and contexts.
プレインストラクトチューニング手法を用いて、模範的回答を学習させました。この手法により、モデルは様々な言語やコンテキストにおいて高品質な応答を理解し生成する能力が向上しています。
#### Implementation Information
[Pre-Instruction Training]
https://huggingface.co/instruction-pretrain/instruction-synthesizer
### [Disclaimer]
このモデルは研究開発のみを目的として提供されるものであり、実験的なプロトタイプとみなされるべきモデルです。
商業的な使用やミッションクリティカルな環境への配備を意図したものではありません。
本モデルの使用は、使用者の責任において行われるものとし、その性能および結果は保証されません。
Axcxept株式会社は、直接的、間接的、特別、偶発的、結果的な損害、または本モデルの使用から生じるいかなる損失に対しても、得られた結果にかかわらず、一切の責任を負いません。
利用者は、本モデルの使用に伴うリスクを十分に理解し、自己の判断で使用するものとします。
### [Hardware]
A100 × 8(Running in 2h)
### [謝辞]
本ベースモデルを開発してくださったGoogle社ならびに当該チームの開発者の方々、また自動評価の手法を提供してくださった多数の方々に感謝と尊敬の意を表します。
### [We are.]
[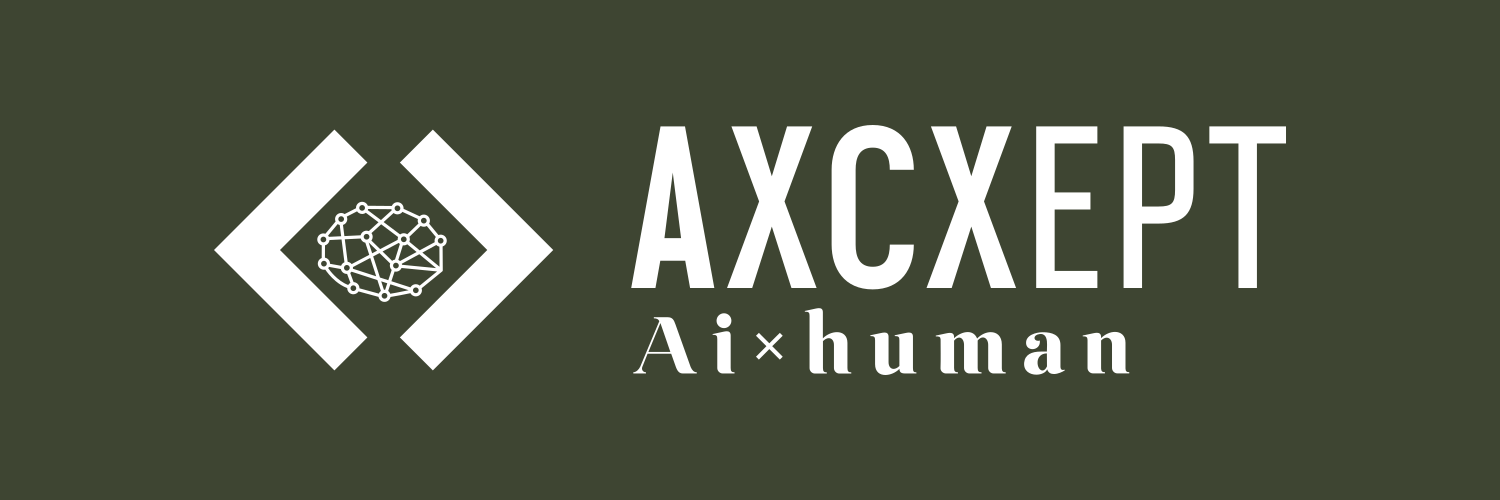](https://axcxept.com) |