File size: 8,231 Bytes
bd174da |
1 2 3 4 5 6 7 8 9 10 11 12 13 14 15 16 17 18 19 20 21 22 23 24 25 26 27 28 29 30 31 32 33 34 35 36 37 38 39 40 41 42 43 44 45 46 47 48 49 50 51 52 53 54 55 56 57 58 59 60 61 62 63 64 65 66 67 68 69 70 71 72 73 74 75 76 77 78 79 80 81 82 83 84 85 86 87 88 89 90 91 92 93 94 95 96 97 98 99 100 101 102 103 104 105 106 107 108 109 110 111 112 113 114 115 116 117 118 119 120 121 122 123 124 125 126 127 128 129 130 131 132 133 134 135 136 137 138 139 140 141 142 143 144 145 146 147 148 149 150 151 152 153 154 155 156 157 158 159 160 161 162 163 164 165 166 167 168 169 170 171 172 173 174 175 176 177 178 179 180 181 182 183 184 185 186 187 188 189 190 191 192 193 194 195 196 197 198 199 200 201 202 203 204 205 206 207 208 209 210 211 212 213 214 215 216 217 218 219 220 221 222 223 224 225 226 227 228 229 230 231 232 233 234 235 236 237 238 239 240 241 242 243 244 245 246 247 248 |
---
license: other
license_name: llama-3
license_link: https://llama.meta.com/llama3/license/
tags:
- text-generation-inference
- transformers
- unsloth
- llama
datasets:
- Replete-AI/code_bagel_hermes-2.5
- Replete-AI/code_bagel
- Replete-AI/OpenHermes-2.5-Uncensored
- teknium/OpenHermes-2.5
- layoric/tiny-codes-alpaca
- glaiveai/glaive-code-assistant-v3
- ajibawa-2023/Code-290k-ShareGPT
- TIGER-Lab/MathInstruct
- chargoddard/commitpack-ft-instruct-rated
- iamturun/code_instructions_120k_alpaca
- ise-uiuc/Magicoder-Evol-Instruct-110K
- cognitivecomputations/dolphin-coder
- nickrosh/Evol-Instruct-Code-80k-v1
- coseal/CodeUltraFeedback_binarized
- glaiveai/glaive-function-calling-v2
- CyberNative/Code_Vulnerability_Security_DPO
- jondurbin/airoboros-2.2
- camel-ai
- lmsys/lmsys-chat-1m
- CollectiveCognition/chats-data-2023-09-22
- CoT-Alpaca-GPT4
- WizardLM/WizardLM_evol_instruct_70k
- WizardLM/WizardLM_evol_instruct_V2_196k
- teknium/GPT4-LLM-Cleaned
- GPTeacher
- OpenGPT
- meta-math/MetaMathQA
- Open-Orca/SlimOrca
- garage-bAInd/Open-Platypus
- anon8231489123/ShareGPT_Vicuna_unfiltered
- Unnatural-Instructions-GPT4
model-index:
- name: Replete-Coder-llama3-8b
results:
- task:
name: HumanEval
type: text-generation
dataset:
type: openai_humaneval
name: HumanEval
metrics:
- name: pass@1
type: pass@1
value: 0.6468383584267833
verified: true
- task:
name: AI2 Reasoning Challenge
type: text-generation
dataset:
name: AI2 Reasoning Challenge (25-Shot)
type: ai2_arc
config: ARC-Challenge
split: test
args:
num_few_shot: 25
metrics:
- type: accuracy
value: null
name: normalized accuracy
source:
url: https://www.placeholderurl.com
name: Open LLM Leaderboard
- task:
name: Text Generation
type: text-generation
dataset:
name: HellaSwag (10-Shot)
type: hellaswag
split: validation
args:
num_few_shot: 10
metrics:
- type: accuracy
value: null
name: normalized accuracy
source:
url: https://www.placeholderurl.com
name: Open LLM Leaderboard
- task:
name: Text Generation
type: text-generation
dataset:
name: MMLU (5-Shot)
type: cais/mmlu
config: all
split: test
args:
num_few_shot: 5
metrics:
- type: accuracy
value: null
name: accuracy
source:
url: https://www.placeholderurl.com
name: Open LLM Leaderboard
- task:
name: Text Generation
type: text-generation
dataset:
name: TruthfulQA (0-shot)
type: truthful_qa
config: multiple_choice
split: validation
args:
num_few_shot: 0
metrics:
- type: multiple_choice_accuracy
value: null
source:
url: https://www.placeholderurl.com
name: Open LLM Leaderboard
- task:
name: Text Generation
type: text-generation
dataset:
name: Winogrande (5-shot)
type: winogrande
config: winogrande_xl
split: validation
args:
num_few_shot: 5
metrics:
- type: accuracy
value: null
name: accuracy
source:
url: https://www.placeholderurl.com
name: Open LLM Leaderboard
- task:
name: Text Generation
type: text-generation
dataset:
name: GSM8k (5-shot)
type: gsm8k
config: main
split: test
args:
num_few_shot: 5
metrics:
- type: accuracy
value: null
name: accuracy
source:
url: https://www.placeholderurl.com
name: Open LLM Leaderboard
base_model: Replete-AI/Llama3-8B-Instruct-Replete-Adapted
pipeline_tag: text-generation
---
# QuantFactory/Llama3-8B-Instruct-Replete-Adapted-GGUF
This is quantized version of [Replete-AI/Llama3-8B-Instruct-Replete-Adapted](https://huggingface.co/Replete-AI/Llama3-8B-Instruct-Replete-Adapted) created using llama.cpp
# Model Description
This is the meta-llama/Meta-Llama-3-8B-Instruct model with the Replete-AI/Replete-Coder-Llama3-8B adapter applied on top of it.
This is mostly an experinment to see how the model would perform.
Links to the oringal model and adapter are bellow:
Orginal model:
- https://huggingface.co/Replete-AI/Replete-Coder-Llama3-8B
Adapter:
- Coming soon
_________________________________________________________________________________________________________
# Replete-Coder-llama3-8b
Finetuned by: Rombodawg
### More than just a coding model!
Although Replete-Coder has amazing coding capabilities, its trained on vaste amount of non-coding data, fully cleaned and uncensored. Dont just use it for coding, use it for all your needs! We are truly trying to make the GPT killer!
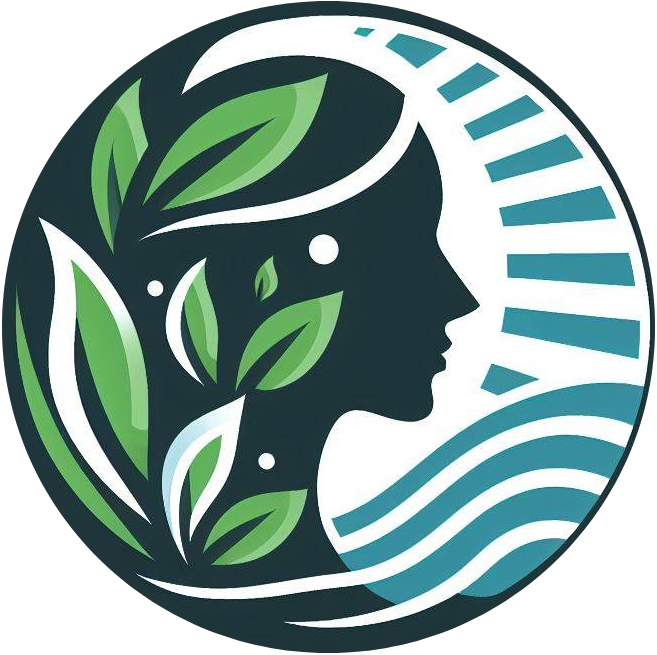
Thank you to TensorDock for sponsoring Replete-Coder-llama3-8b and Replete-Coder-Qwen2-1.5b
you can check out their website for cloud compute rental below.
- https://tensordock.com
__________________________________________________________________________________________________
Replete-Coder-llama3-8b is a general purpose model that is specially trained in coding in over 100 coding languages. The data used to train the model contains 25% non-code instruction data and 75% coding instruction data totaling up to 3.9 million lines, roughly 1 billion tokens, or 7.27gb of instruct data. The data used to train this model was 100% uncensored, then fully deduplicated, before training happened.
The Replete-Coder models (including Replete-Coder-llama3-8b and Replete-Coder-Qwen2-1.5b) feature the following:
- Advanced coding capabilities in over 100 coding languages
- Advanced code translation (between languages)
- Security and vulnerability prevention related coding capabilities
- General purpose use
- Uncensored use
- Function calling
- Advanced math use
- Use on low end (8b) and mobile (1.5b) platforms
Notice: Replete-Coder series of models are fine-tuned on a context window of 8192 tokens. Performance past this context window is not guaranteed.

__________________________________________________________________________________________________
You can find the 25% non-coding instruction below:
- https://huggingface.co/datasets/Replete-AI/OpenHermes-2.5-Uncensored
And the 75% coding specific instruction data below:
- https://huggingface.co/datasets/Replete-AI/code_bagel
These two datasets were combined to create the final dataset for training, which is linked below:
- https://huggingface.co/datasets/Replete-AI/code_bagel_hermes-2.5
__________________________________________________________________________________________________
## Prompt Template: Custom Alpaca
```
### System:
{}
### Instruction:
{}
### Response:
{}
```
Note: The system prompt varies in training data, but the most commonly used one is:
```
Below is an instruction that describes a task, Write a response that appropriately completes the request.
```
End token:
```
<|endoftext|>
```
__________________________________________________________________________________________________
Thank you to the community for your contributions to the Replete-AI/code_bagel_hermes-2.5 dataset. Without the participation of so many members making their datasets free and open source for any to use, this amazing AI model wouldn't be possible.
Extra special thanks to Teknium for the Open-Hermes-2.5 dataset and jondurbin for the bagel dataset and the naming idea for the code_bagel series of datasets. You can find both of their huggingface accounts linked below:
- https://huggingface.co/teknium
- https://huggingface.co/jondurbin
Another special thanks to unsloth for being the main method of training for Replete-Coder. Bellow you can find their github, as well as the special Replete-Ai secret sause (Unsloth + Qlora + Galore) colab code document that was used to train this model.
- https://github.com/unslothai/unsloth
- https://colab.research.google.com/drive/1VAaxMQJN9-78WLsPU0GWg5tEkasXoTP9?usp=sharing |