complete the model package
Browse files- LICENSE +201 -0
- README.md +154 -0
- configs/evaluate.json +38 -0
- configs/inference.json +151 -0
- configs/logging.conf +21 -0
- configs/metadata.json +115 -0
- configs/multi_gpu_train.json +36 -0
- configs/train.json +525 -0
- docs/README.md +147 -0
- docs/data_license.txt +6 -0
- models/model.pt +3 -0
- models/stage0/model.pt +3 -0
- scripts/prepare_patches.py +232 -0
LICENSE
ADDED
@@ -0,0 +1,201 @@
|
|
|
|
|
|
|
|
|
|
|
|
|
|
|
|
|
|
|
|
|
|
|
|
|
|
|
|
|
|
|
|
|
|
|
|
|
|
|
|
|
|
|
|
|
|
|
|
|
|
|
|
|
|
|
|
|
|
|
|
|
|
|
|
|
|
|
|
|
|
|
|
|
|
|
|
|
|
|
|
|
|
|
|
|
|
|
|
|
|
|
|
|
|
|
|
|
|
|
|
|
|
|
|
|
|
|
|
|
|
|
|
|
|
|
|
|
|
|
|
|
|
|
|
|
|
|
|
|
|
|
|
|
|
|
|
|
|
|
|
|
|
|
|
|
|
|
|
|
|
|
|
|
|
|
|
|
|
|
|
|
|
|
|
|
|
|
|
|
|
|
|
|
|
|
|
|
|
|
|
|
|
|
|
|
|
|
|
|
|
|
|
|
|
|
|
|
|
|
|
|
|
|
|
|
|
|
|
|
|
|
|
|
|
|
|
|
|
|
|
|
|
|
|
|
|
|
|
|
|
|
|
|
|
|
|
|
|
|
|
|
|
|
|
|
|
|
|
|
|
|
|
|
|
|
|
|
|
|
|
|
|
|
|
|
|
|
|
|
|
|
|
|
|
|
|
|
|
|
|
|
|
|
|
|
|
|
|
|
|
|
|
|
|
|
|
|
|
|
|
|
|
|
|
|
|
|
|
|
|
|
|
|
|
|
|
|
|
|
|
|
|
|
|
|
|
|
|
|
|
|
|
|
|
|
|
|
|
|
|
|
|
|
|
|
|
|
|
|
|
|
|
|
|
|
|
|
|
|
|
|
|
|
|
|
|
|
|
|
|
|
|
|
|
|
|
|
|
|
|
|
|
|
|
|
|
|
|
|
|
|
|
|
|
|
|
|
|
|
|
|
|
|
|
|
1 |
+
Apache License
|
2 |
+
Version 2.0, January 2004
|
3 |
+
http://www.apache.org/licenses/
|
4 |
+
|
5 |
+
TERMS AND CONDITIONS FOR USE, REPRODUCTION, AND DISTRIBUTION
|
6 |
+
|
7 |
+
1. Definitions.
|
8 |
+
|
9 |
+
"License" shall mean the terms and conditions for use, reproduction,
|
10 |
+
and distribution as defined by Sections 1 through 9 of this document.
|
11 |
+
|
12 |
+
"Licensor" shall mean the copyright owner or entity authorized by
|
13 |
+
the copyright owner that is granting the License.
|
14 |
+
|
15 |
+
"Legal Entity" shall mean the union of the acting entity and all
|
16 |
+
other entities that control, are controlled by, or are under common
|
17 |
+
control with that entity. For the purposes of this definition,
|
18 |
+
"control" means (i) the power, direct or indirect, to cause the
|
19 |
+
direction or management of such entity, whether by contract or
|
20 |
+
otherwise, or (ii) ownership of fifty percent (50%) or more of the
|
21 |
+
outstanding shares, or (iii) beneficial ownership of such entity.
|
22 |
+
|
23 |
+
"You" (or "Your") shall mean an individual or Legal Entity
|
24 |
+
exercising permissions granted by this License.
|
25 |
+
|
26 |
+
"Source" form shall mean the preferred form for making modifications,
|
27 |
+
including but not limited to software source code, documentation
|
28 |
+
source, and configuration files.
|
29 |
+
|
30 |
+
"Object" form shall mean any form resulting from mechanical
|
31 |
+
transformation or translation of a Source form, including but
|
32 |
+
not limited to compiled object code, generated documentation,
|
33 |
+
and conversions to other media types.
|
34 |
+
|
35 |
+
"Work" shall mean the work of authorship, whether in Source or
|
36 |
+
Object form, made available under the License, as indicated by a
|
37 |
+
copyright notice that is included in or attached to the work
|
38 |
+
(an example is provided in the Appendix below).
|
39 |
+
|
40 |
+
"Derivative Works" shall mean any work, whether in Source or Object
|
41 |
+
form, that is based on (or derived from) the Work and for which the
|
42 |
+
editorial revisions, annotations, elaborations, or other modifications
|
43 |
+
represent, as a whole, an original work of authorship. For the purposes
|
44 |
+
of this License, Derivative Works shall not include works that remain
|
45 |
+
separable from, or merely link (or bind by name) to the interfaces of,
|
46 |
+
the Work and Derivative Works thereof.
|
47 |
+
|
48 |
+
"Contribution" shall mean any work of authorship, including
|
49 |
+
the original version of the Work and any modifications or additions
|
50 |
+
to that Work or Derivative Works thereof, that is intentionally
|
51 |
+
submitted to Licensor for inclusion in the Work by the copyright owner
|
52 |
+
or by an individual or Legal Entity authorized to submit on behalf of
|
53 |
+
the copyright owner. For the purposes of this definition, "submitted"
|
54 |
+
means any form of electronic, verbal, or written communication sent
|
55 |
+
to the Licensor or its representatives, including but not limited to
|
56 |
+
communication on electronic mailing lists, source code control systems,
|
57 |
+
and issue tracking systems that are managed by, or on behalf of, the
|
58 |
+
Licensor for the purpose of discussing and improving the Work, but
|
59 |
+
excluding communication that is conspicuously marked or otherwise
|
60 |
+
designated in writing by the copyright owner as "Not a Contribution."
|
61 |
+
|
62 |
+
"Contributor" shall mean Licensor and any individual or Legal Entity
|
63 |
+
on behalf of whom a Contribution has been received by Licensor and
|
64 |
+
subsequently incorporated within the Work.
|
65 |
+
|
66 |
+
2. Grant of Copyright License. Subject to the terms and conditions of
|
67 |
+
this License, each Contributor hereby grants to You a perpetual,
|
68 |
+
worldwide, non-exclusive, no-charge, royalty-free, irrevocable
|
69 |
+
copyright license to reproduce, prepare Derivative Works of,
|
70 |
+
publicly display, publicly perform, sublicense, and distribute the
|
71 |
+
Work and such Derivative Works in Source or Object form.
|
72 |
+
|
73 |
+
3. Grant of Patent License. Subject to the terms and conditions of
|
74 |
+
this License, each Contributor hereby grants to You a perpetual,
|
75 |
+
worldwide, non-exclusive, no-charge, royalty-free, irrevocable
|
76 |
+
(except as stated in this section) patent license to make, have made,
|
77 |
+
use, offer to sell, sell, import, and otherwise transfer the Work,
|
78 |
+
where such license applies only to those patent claims licensable
|
79 |
+
by such Contributor that are necessarily infringed by their
|
80 |
+
Contribution(s) alone or by combination of their Contribution(s)
|
81 |
+
with the Work to which such Contribution(s) was submitted. If You
|
82 |
+
institute patent litigation against any entity (including a
|
83 |
+
cross-claim or counterclaim in a lawsuit) alleging that the Work
|
84 |
+
or a Contribution incorporated within the Work constitutes direct
|
85 |
+
or contributory patent infringement, then any patent licenses
|
86 |
+
granted to You under this License for that Work shall terminate
|
87 |
+
as of the date such litigation is filed.
|
88 |
+
|
89 |
+
4. Redistribution. You may reproduce and distribute copies of the
|
90 |
+
Work or Derivative Works thereof in any medium, with or without
|
91 |
+
modifications, and in Source or Object form, provided that You
|
92 |
+
meet the following conditions:
|
93 |
+
|
94 |
+
(a) You must give any other recipients of the Work or
|
95 |
+
Derivative Works a copy of this License; and
|
96 |
+
|
97 |
+
(b) You must cause any modified files to carry prominent notices
|
98 |
+
stating that You changed the files; and
|
99 |
+
|
100 |
+
(c) You must retain, in the Source form of any Derivative Works
|
101 |
+
that You distribute, all copyright, patent, trademark, and
|
102 |
+
attribution notices from the Source form of the Work,
|
103 |
+
excluding those notices that do not pertain to any part of
|
104 |
+
the Derivative Works; and
|
105 |
+
|
106 |
+
(d) If the Work includes a "NOTICE" text file as part of its
|
107 |
+
distribution, then any Derivative Works that You distribute must
|
108 |
+
include a readable copy of the attribution notices contained
|
109 |
+
within such NOTICE file, excluding those notices that do not
|
110 |
+
pertain to any part of the Derivative Works, in at least one
|
111 |
+
of the following places: within a NOTICE text file distributed
|
112 |
+
as part of the Derivative Works; within the Source form or
|
113 |
+
documentation, if provided along with the Derivative Works; or,
|
114 |
+
within a display generated by the Derivative Works, if and
|
115 |
+
wherever such third-party notices normally appear. The contents
|
116 |
+
of the NOTICE file are for informational purposes only and
|
117 |
+
do not modify the License. You may add Your own attribution
|
118 |
+
notices within Derivative Works that You distribute, alongside
|
119 |
+
or as an addendum to the NOTICE text from the Work, provided
|
120 |
+
that such additional attribution notices cannot be construed
|
121 |
+
as modifying the License.
|
122 |
+
|
123 |
+
You may add Your own copyright statement to Your modifications and
|
124 |
+
may provide additional or different license terms and conditions
|
125 |
+
for use, reproduction, or distribution of Your modifications, or
|
126 |
+
for any such Derivative Works as a whole, provided Your use,
|
127 |
+
reproduction, and distribution of the Work otherwise complies with
|
128 |
+
the conditions stated in this License.
|
129 |
+
|
130 |
+
5. Submission of Contributions. Unless You explicitly state otherwise,
|
131 |
+
any Contribution intentionally submitted for inclusion in the Work
|
132 |
+
by You to the Licensor shall be under the terms and conditions of
|
133 |
+
this License, without any additional terms or conditions.
|
134 |
+
Notwithstanding the above, nothing herein shall supersede or modify
|
135 |
+
the terms of any separate license agreement you may have executed
|
136 |
+
with Licensor regarding such Contributions.
|
137 |
+
|
138 |
+
6. Trademarks. This License does not grant permission to use the trade
|
139 |
+
names, trademarks, service marks, or product names of the Licensor,
|
140 |
+
except as required for reasonable and customary use in describing the
|
141 |
+
origin of the Work and reproducing the content of the NOTICE file.
|
142 |
+
|
143 |
+
7. Disclaimer of Warranty. Unless required by applicable law or
|
144 |
+
agreed to in writing, Licensor provides the Work (and each
|
145 |
+
Contributor provides its Contributions) on an "AS IS" BASIS,
|
146 |
+
WITHOUT WARRANTIES OR CONDITIONS OF ANY KIND, either express or
|
147 |
+
implied, including, without limitation, any warranties or conditions
|
148 |
+
of TITLE, NON-INFRINGEMENT, MERCHANTABILITY, or FITNESS FOR A
|
149 |
+
PARTICULAR PURPOSE. You are solely responsible for determining the
|
150 |
+
appropriateness of using or redistributing the Work and assume any
|
151 |
+
risks associated with Your exercise of permissions under this License.
|
152 |
+
|
153 |
+
8. Limitation of Liability. In no event and under no legal theory,
|
154 |
+
whether in tort (including negligence), contract, or otherwise,
|
155 |
+
unless required by applicable law (such as deliberate and grossly
|
156 |
+
negligent acts) or agreed to in writing, shall any Contributor be
|
157 |
+
liable to You for damages, including any direct, indirect, special,
|
158 |
+
incidental, or consequential damages of any character arising as a
|
159 |
+
result of this License or out of the use or inability to use the
|
160 |
+
Work (including but not limited to damages for loss of goodwill,
|
161 |
+
work stoppage, computer failure or malfunction, or any and all
|
162 |
+
other commercial damages or losses), even if such Contributor
|
163 |
+
has been advised of the possibility of such damages.
|
164 |
+
|
165 |
+
9. Accepting Warranty or Additional Liability. While redistributing
|
166 |
+
the Work or Derivative Works thereof, You may choose to offer,
|
167 |
+
and charge a fee for, acceptance of support, warranty, indemnity,
|
168 |
+
or other liability obligations and/or rights consistent with this
|
169 |
+
License. However, in accepting such obligations, You may act only
|
170 |
+
on Your own behalf and on Your sole responsibility, not on behalf
|
171 |
+
of any other Contributor, and only if You agree to indemnify,
|
172 |
+
defend, and hold each Contributor harmless for any liability
|
173 |
+
incurred by, or claims asserted against, such Contributor by reason
|
174 |
+
of your accepting any such warranty or additional liability.
|
175 |
+
|
176 |
+
END OF TERMS AND CONDITIONS
|
177 |
+
|
178 |
+
APPENDIX: How to apply the Apache License to your work.
|
179 |
+
|
180 |
+
To apply the Apache License to your work, attach the following
|
181 |
+
boilerplate notice, with the fields enclosed by brackets "[]"
|
182 |
+
replaced with your own identifying information. (Don't include
|
183 |
+
the brackets!) The text should be enclosed in the appropriate
|
184 |
+
comment syntax for the file format. We also recommend that a
|
185 |
+
file or class name and description of purpose be included on the
|
186 |
+
same "printed page" as the copyright notice for easier
|
187 |
+
identification within third-party archives.
|
188 |
+
|
189 |
+
Copyright [yyyy] [name of copyright owner]
|
190 |
+
|
191 |
+
Licensed under the Apache License, Version 2.0 (the "License");
|
192 |
+
you may not use this file except in compliance with the License.
|
193 |
+
You may obtain a copy of the License at
|
194 |
+
|
195 |
+
http://www.apache.org/licenses/LICENSE-2.0
|
196 |
+
|
197 |
+
Unless required by applicable law or agreed to in writing, software
|
198 |
+
distributed under the License is distributed on an "AS IS" BASIS,
|
199 |
+
WITHOUT WARRANTIES OR CONDITIONS OF ANY KIND, either express or implied.
|
200 |
+
See the License for the specific language governing permissions and
|
201 |
+
limitations under the License.
|
README.md
ADDED
@@ -0,0 +1,154 @@
|
|
|
|
|
|
|
|
|
|
|
|
|
|
|
|
|
|
|
|
|
|
|
|
|
|
|
|
|
|
|
|
|
|
|
|
|
|
|
|
|
|
|
|
|
|
|
|
|
|
|
|
|
|
|
|
|
|
|
|
|
|
|
|
|
|
|
|
|
|
|
|
|
|
|
|
|
|
|
|
|
|
|
|
|
|
|
|
|
|
|
|
|
|
|
|
|
|
|
|
|
|
|
|
|
|
|
|
|
|
|
|
|
|
|
|
|
|
|
|
|
|
|
|
|
|
|
|
|
|
|
|
|
|
|
|
|
|
|
|
|
|
|
|
|
|
|
|
|
|
|
|
|
|
|
|
|
|
|
|
|
|
|
|
|
|
|
|
|
|
|
|
|
|
|
|
|
|
|
|
|
|
|
|
|
|
|
|
|
|
|
|
|
|
|
|
|
|
|
|
|
|
|
|
|
|
|
|
|
|
|
|
|
|
|
|
|
|
|
|
|
|
|
|
|
|
|
|
|
|
|
|
|
|
|
|
|
|
|
|
|
|
|
|
|
|
|
|
|
|
|
|
|
|
|
|
|
|
|
|
|
|
|
|
|
|
|
|
|
|
|
|
|
|
|
|
|
|
|
|
|
|
|
|
|
|
|
|
|
|
|
|
|
|
|
|
|
|
|
|
|
|
|
|
|
|
|
|
|
|
|
1 |
+
---
|
2 |
+
tags:
|
3 |
+
- monai
|
4 |
+
- medical
|
5 |
+
library_name: monai
|
6 |
+
license: apache-2.0
|
7 |
+
---
|
8 |
+
# Model Overview
|
9 |
+
|
10 |
+
A pre-trained model for simultaneous segmentation and classification of nuclei within multi-tissue histology images based on CoNSeP data. The details of the model can be found in [1].
|
11 |
+
|
12 |
+
## Workflow
|
13 |
+
|
14 |
+
The model is trained to simultaneous segment and classify nuclei. Training is done via a two-stage approach. First initialized the model with pre-trained weights on the [ImageNet dataset](https://ieeexplore.ieee.org/document/5206848), trained only the decoders for the first 50 epochs, and then fine-tuned all layers for another 50 epochs. There are two training modes in total. If "original" mode is specified, it uses [270, 270] and [80, 80] for `patch_size` and `out_size` respectively. If "fast" mode is specified, it uses [256, 256] and [164, 164] for `patch_size` and `out_size` respectively. The results we show below are based on the "fast" model.
|
15 |
+
|
16 |
+
- We train the first stage with pre-trained weights from some internal data.
|
17 |
+
|
18 |
+
- The original author's repo also has pre-trained weights which is for non-commercial use. Each user is responsible for checking the content of models/datasets and the applicable licenses and determining if suitable for the intended use. The license for the pre-trained model is different than MONAI license. Please check the source where these weights are obtained from: <https://github.com/vqdang/hover_net#data-format>
|
19 |
+
|
20 |
+
`PRETRAIN_MODEL_URL` is "https://drive.google.com/u/1/uc?id=1KntZge40tAHgyXmHYVqZZ5d2p_4Qr2l5&export=download" which can be used in bash code below.
|
21 |
+
|
22 |
+

|
23 |
+
|
24 |
+
## Data
|
25 |
+
|
26 |
+
The training data is from <https://warwick.ac.uk/fac/cross_fac/tia/data/hovernet/>.
|
27 |
+
|
28 |
+
- Target: segment instance-level nuclei and classify the nuclei type
|
29 |
+
- Task: Segmentation and classification
|
30 |
+
- Modality: RGB images
|
31 |
+
- Size: 41 image tiles (2009 patches)
|
32 |
+
|
33 |
+
The provided labelled data was partitioned, based on the original split, into training (27 tiles) and testing (14 tiles) datasets.
|
34 |
+
|
35 |
+
After download the datasets, please run `scripts/prepare_patches.py` to prepare patches from tiles. Prepared patches are saved in `your-concep-dataset-path`/Prepared. The implementation is referring to <https://github.com/vqdang/hover_net/blob/master/extract_patches.py>. The command is like:
|
36 |
+
|
37 |
+
```
|
38 |
+
python scripts/prepare_patches.py -root your-concep-dataset-path
|
39 |
+
```
|
40 |
+
|
41 |
+
## Training configuration
|
42 |
+
|
43 |
+
This model utilized a two-stage approach. The training was performed with the following:
|
44 |
+
|
45 |
+
- GPU: At least 24GB of GPU memory.
|
46 |
+
- Actual Model Input: 256 x 256
|
47 |
+
- AMP: True
|
48 |
+
- Optimizer: Adam
|
49 |
+
- Learning Rate: 1e-4
|
50 |
+
- Loss: HoVerNetLoss
|
51 |
+
|
52 |
+
## Input
|
53 |
+
|
54 |
+
Input: RGB images
|
55 |
+
|
56 |
+
## Output
|
57 |
+
|
58 |
+
Output: a dictionary with the following keys:
|
59 |
+
|
60 |
+
1. nucleus_prediction: predict whether or not a pixel belongs to the nuclei or background
|
61 |
+
2. horizontal_vertical: predict the horizontal and vertical distances of nuclear pixels to their centres of mass
|
62 |
+
3. type_prediction: predict the type of nucleus for each pixel
|
63 |
+
|
64 |
+
## Model Performance
|
65 |
+
|
66 |
+
The achieved metrics on the validation data are:
|
67 |
+
|
68 |
+
Fast mode:
|
69 |
+
- Binary Dice: 0.8293
|
70 |
+
- PQ: 0.4936
|
71 |
+
- F1d: 0.7480
|
72 |
+
|
73 |
+
#### Training Loss and Dice
|
74 |
+
|
75 |
+
stage1:
|
76 |
+
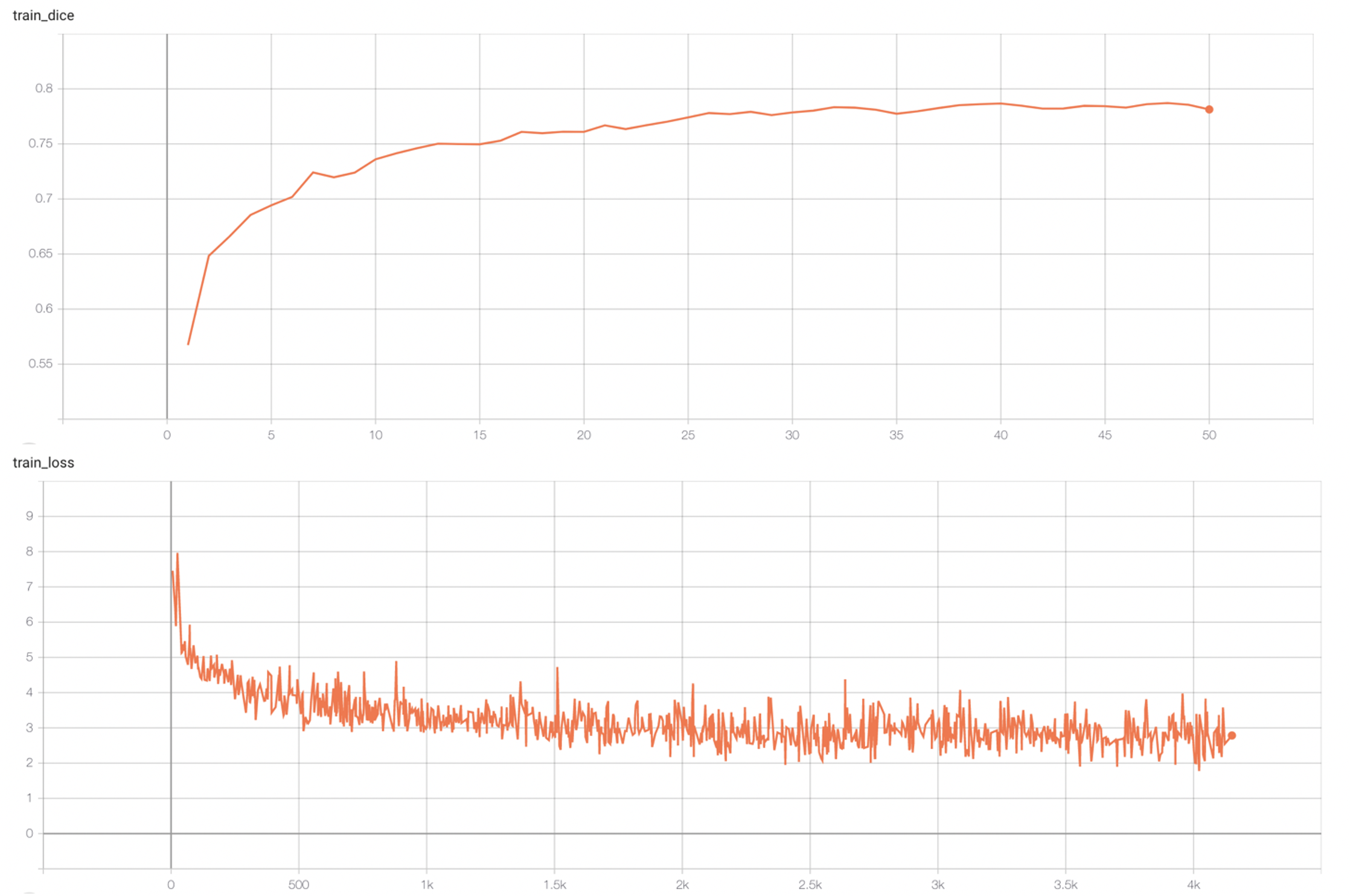
|
77 |
+
|
78 |
+
stage2:
|
79 |
+
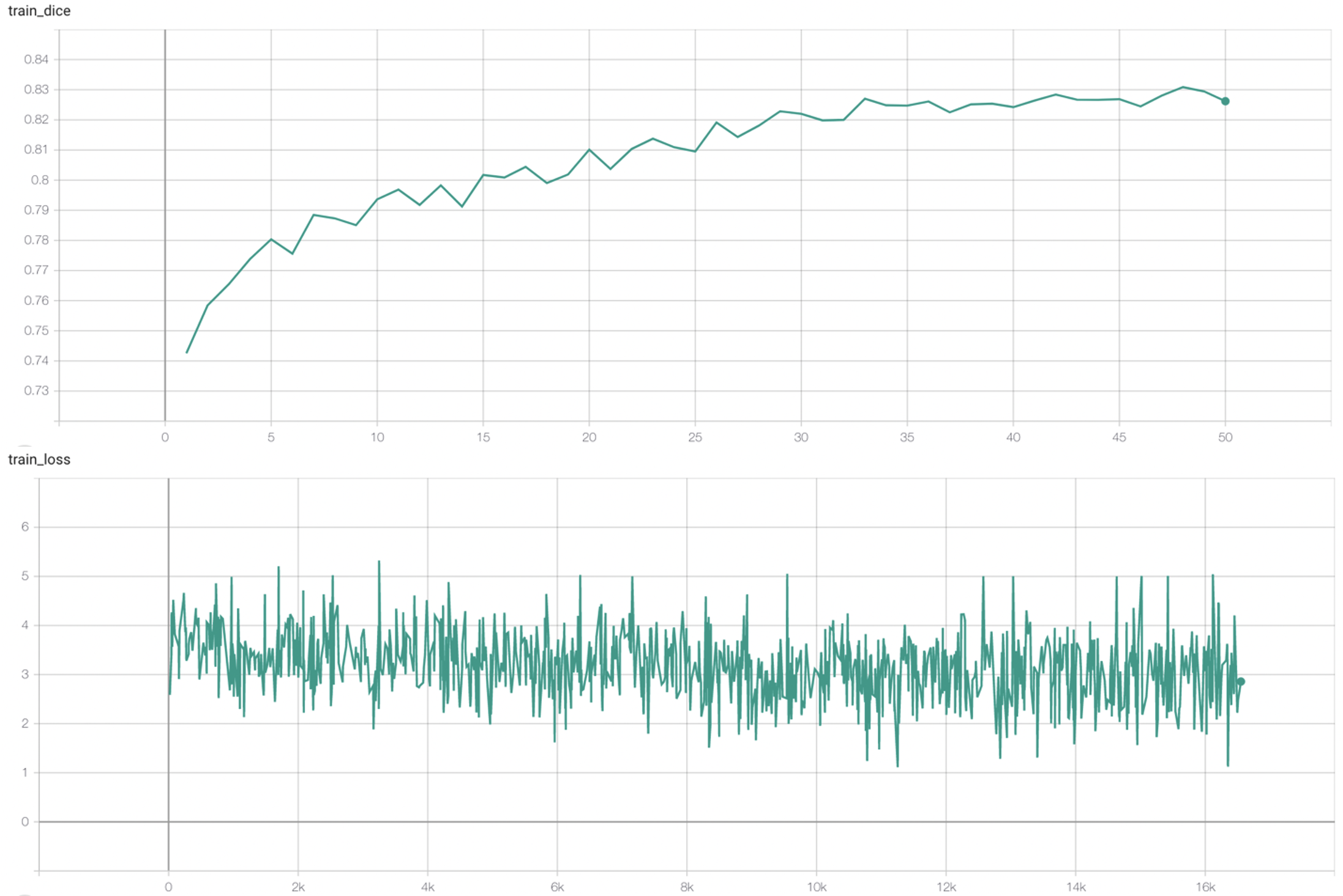
|
80 |
+
|
81 |
+
#### Validation Dice
|
82 |
+
|
83 |
+
stage1:
|
84 |
+
|
85 |
+
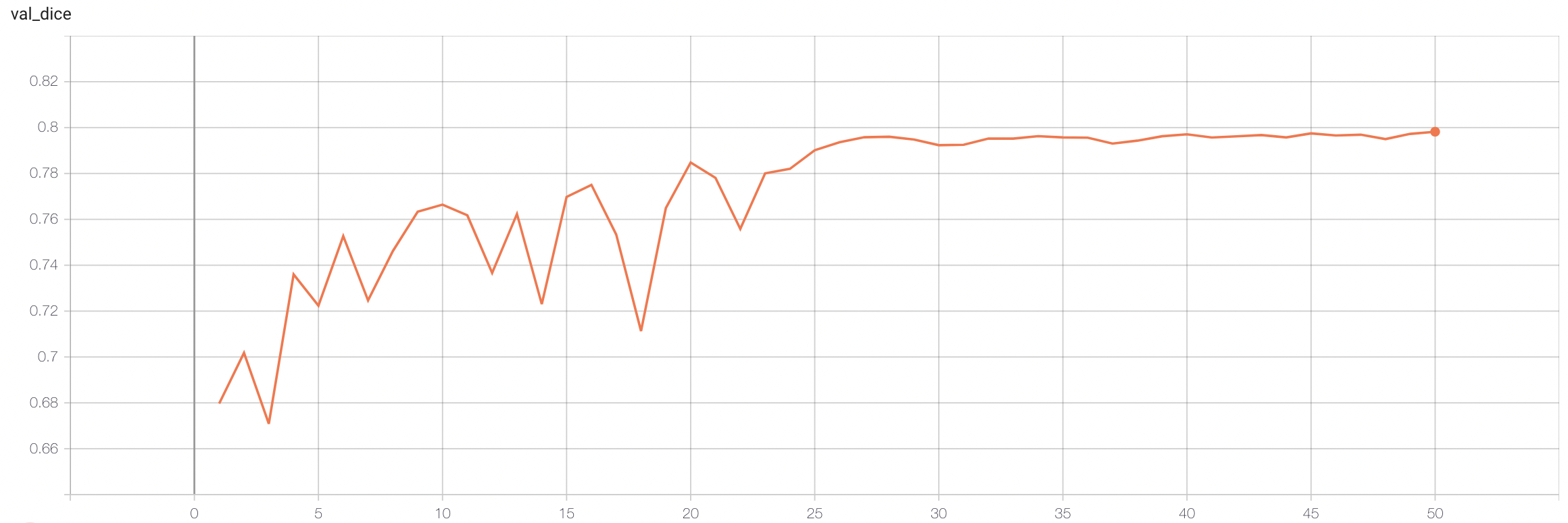
|
86 |
+
|
87 |
+
stage2:
|
88 |
+
|
89 |
+
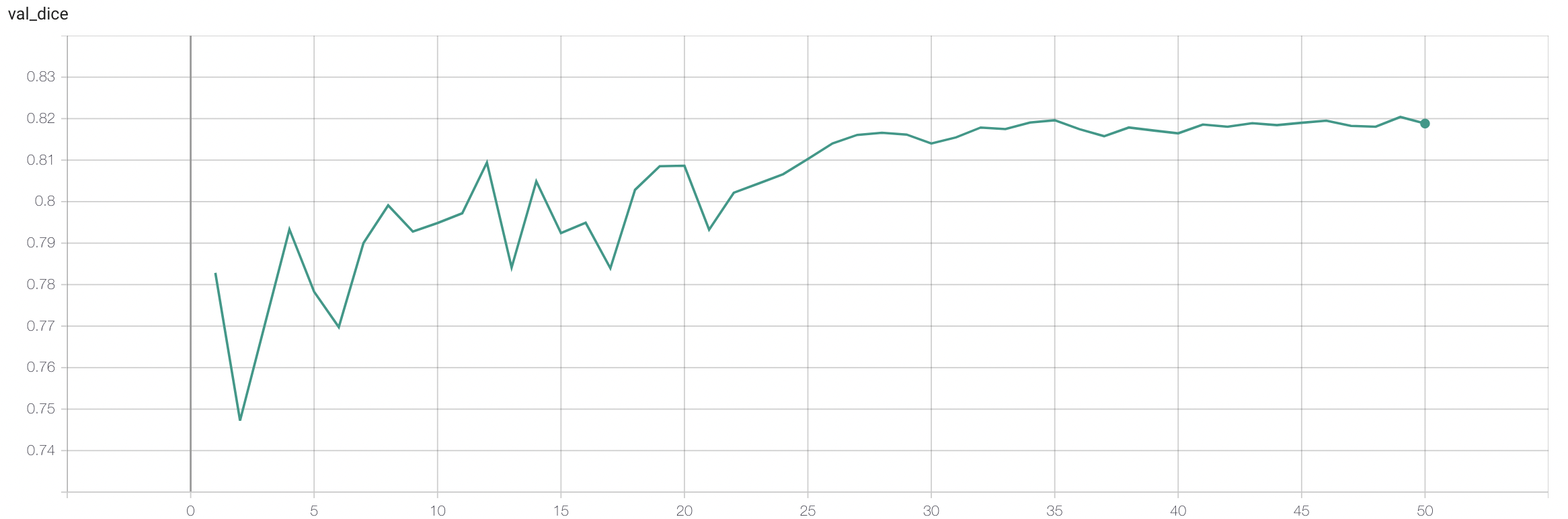
|
90 |
+
|
91 |
+
## commands example
|
92 |
+
|
93 |
+
Execute training:
|
94 |
+
|
95 |
+
- Run first stage
|
96 |
+
|
97 |
+
```
|
98 |
+
python -m monai.bundle run training --meta_file configs/metadata.json --config_file configs/train.json --logging_file configs/logging.conf --network_def#pretrained_url `PRETRAIN_MODEL_URL` --stage 0
|
99 |
+
```
|
100 |
+
|
101 |
+
- Run second stage
|
102 |
+
|
103 |
+
```
|
104 |
+
python -m monai.bundle run training --meta_file configs/metadata.json --config_file configs/train.json --logging_file configs/logging.conf --network_def#freeze_encoder false --network_def#pretrained_url None --stage 1
|
105 |
+
```
|
106 |
+
|
107 |
+
Override the `train` config to execute multi-GPU training:
|
108 |
+
|
109 |
+
- Run first stage
|
110 |
+
|
111 |
+
```
|
112 |
+
torchrun --standalone --nnodes=1 --nproc_per_node=2 -m monai.bundle run training --meta_file configs/metadata.json --config_file "['configs/train.json','configs/multi_gpu_train.json']" --logging_file configs/logging.conf --train#dataloader#batch_size 8 --network_def#freeze_encoder true --network_def#pretrained_url `PRETRAIN_MODEL_URL` --stage 0
|
113 |
+
```
|
114 |
+
|
115 |
+
- Run second stage
|
116 |
+
|
117 |
+
```
|
118 |
+
torchrun --standalone --nnodes=1 --nproc_per_node=2 -m monai.bundle run training --meta_file configs/metadata.json --config_file "['configs/train.json','configs/multi_gpu_train.json']" --logging_file configs/logging.conf --train#dataloader#batch_size 4 --network_def#freeze_encoder false --network_def#pretrained_url None --stage 1
|
119 |
+
```
|
120 |
+
|
121 |
+
Override the `train` config to execute evaluation with the trained model:
|
122 |
+
|
123 |
+
```
|
124 |
+
python -m monai.bundle run evaluating --meta_file configs/metadata.json --config_file "['configs/train.json','configs/evaluate.json']" --logging_file configs/logging.conf
|
125 |
+
```
|
126 |
+
|
127 |
+
### Execute inference
|
128 |
+
|
129 |
+
```
|
130 |
+
python -m monai.bundle run evaluating --meta_file configs/metadata.json --config_file configs/inference.json --logging_file configs/logging.conf
|
131 |
+
```
|
132 |
+
|
133 |
+
# Disclaimer
|
134 |
+
|
135 |
+
This is an example, not to be used for diagnostic purposes.
|
136 |
+
|
137 |
+
# References
|
138 |
+
|
139 |
+
[1] Simon Graham, Quoc Dang Vu, Shan E Ahmed Raza, Ayesha Azam, Yee Wah Tsang, Jin Tae Kwak, Nasir Rajpoot, Hover-Net: Simultaneous segmentation and classification of nuclei in multi-tissue histology images, Medical Image Analysis, 2019 https://doi.org/10.1016/j.media.2019.101563
|
140 |
+
|
141 |
+
# License
|
142 |
+
Copyright (c) MONAI Consortium
|
143 |
+
|
144 |
+
Licensed under the Apache License, Version 2.0 (the "License");
|
145 |
+
you may not use this file except in compliance with the License.
|
146 |
+
You may obtain a copy of the License at
|
147 |
+
|
148 |
+
http://www.apache.org/licenses/LICENSE-2.0
|
149 |
+
|
150 |
+
Unless required by applicable law or agreed to in writing, software
|
151 |
+
distributed under the License is distributed on an "AS IS" BASIS,
|
152 |
+
WITHOUT WARRANTIES OR CONDITIONS OF ANY KIND, either express or implied.
|
153 |
+
See the License for the specific language governing permissions and
|
154 |
+
limitations under the License.
|
configs/evaluate.json
ADDED
@@ -0,0 +1,38 @@
|
|
|
|
|
|
|
|
|
|
|
|
|
|
|
|
|
|
|
|
|
|
|
|
|
|
|
|
|
|
|
|
|
|
|
|
|
|
|
|
|
|
|
|
|
|
|
|
|
|
|
|
|
|
|
|
|
|
|
|
|
|
|
|
|
|
|
|
|
|
|
|
|
|
|
|
|
|
|
1 |
+
{
|
2 |
+
"network_def": {
|
3 |
+
"_target_": "HoVerNet",
|
4 |
+
"mode": "@hovernet_mode",
|
5 |
+
"adapt_standard_resnet": true,
|
6 |
+
"in_channels": 3,
|
7 |
+
"out_classes": 5
|
8 |
+
},
|
9 |
+
"validate#handlers": [
|
10 |
+
{
|
11 |
+
"_target_": "CheckpointLoader",
|
12 |
+
"load_path": "$os.path.join(@bundle_root, 'models', 'model.pt')",
|
13 |
+
"load_dict": {
|
14 |
+
"model": "@network"
|
15 |
+
}
|
16 |
+
},
|
17 |
+
{
|
18 |
+
"_target_": "StatsHandler",
|
19 |
+
"iteration_log": false
|
20 |
+
},
|
21 |
+
{
|
22 |
+
"_target_": "MetricsSaver",
|
23 |
+
"save_dir": "@output_dir",
|
24 |
+
"metrics": [
|
25 |
+
"val_mean_dice"
|
26 |
+
],
|
27 |
+
"metric_details": [
|
28 |
+
"val_mean_dice"
|
29 |
+
],
|
30 |
+
"batch_transform": "$monai.handlers.from_engine(['image_meta_dict'])",
|
31 |
+
"summary_ops": "*"
|
32 |
+
}
|
33 |
+
],
|
34 |
+
"evaluating": [
|
35 |
+
"$setattr(torch.backends.cudnn, 'benchmark', True)",
|
36 |
+
"$@validate#evaluator.run()"
|
37 |
+
]
|
38 |
+
}
|
configs/inference.json
ADDED
@@ -0,0 +1,151 @@
|
|
|
|
|
|
|
|
|
|
|
|
|
|
|
|
|
|
|
|
|
|
|
|
|
|
|
|
|
|
|
|
|
|
|
|
|
|
|
|
|
|
|
|
|
|
|
|
|
|
|
|
|
|
|
|
|
|
|
|
|
|
|
|
|
|
|
|
|
|
|
|
|
|
|
|
|
|
|
|
|
|
|
|
|
|
|
|
|
|
|
|
|
|
|
|
|
|
|
|
|
|
|
|
|
|
|
|
|
|
|
|
|
|
|
|
|
|
|
|
|
|
|
|
|
|
|
|
|
|
|
|
|
|
|
|
|
|
|
|
|
|
|
|
|
|
|
|
|
|
|
|
|
|
|
|
|
|
|
|
|
|
|
|
|
|
|
|
|
|
|
|
|
|
|
|
|
|
|
|
|
|
|
|
|
|
|
|
|
|
|
|
|
|
|
|
|
|
|
|
|
|
|
|
|
|
|
|
|
|
|
|
|
|
|
|
|
|
|
|
|
|
|
|
|
|
|
|
|
|
|
|
|
|
|
|
|
|
|
|
|
|
|
|
|
|
|
|
|
|
|
|
|
|
|
|
|
|
|
|
|
|
|
|
|
|
|
|
|
|
|
|
|
|
|
|
|
|
|
|
|
|
|
|
|
|
|
|
|
|
|
|
|
|
|
|
|
|
|
|
|
|
|
|
|
1 |
+
{
|
2 |
+
"imports": [
|
3 |
+
"$import glob",
|
4 |
+
"$import os"
|
5 |
+
],
|
6 |
+
"bundle_root": "$os.getcwd()",
|
7 |
+
"output_dir": "$os.path.join(@bundle_root, 'eval')",
|
8 |
+
"dataset_dir": "/workspace/Data/Pathology/CoNSeP/Test/Images",
|
9 |
+
"num_cpus": 2,
|
10 |
+
"batch_size": 1,
|
11 |
+
"sw_batch_size": 16,
|
12 |
+
"hovernet_mode": "fast",
|
13 |
+
"patch_size": 256,
|
14 |
+
"out_size": 164,
|
15 |
+
"device": "$torch.device('cuda:0' if torch.cuda.is_available() else 'cpu')",
|
16 |
+
"network_def": {
|
17 |
+
"_target_": "HoVerNet",
|
18 |
+
"mode": "@hovernet_mode",
|
19 |
+
"adapt_standard_resnet": true,
|
20 |
+
"in_channels": 3,
|
21 |
+
"out_classes": 5
|
22 |
+
},
|
23 |
+
"network": "$@network_def.to(@device)",
|
24 |
+
"preprocessing": {
|
25 |
+
"_target_": "Compose",
|
26 |
+
"transforms": [
|
27 |
+
{
|
28 |
+
"_target_": "LoadImaged",
|
29 |
+
"keys": "image",
|
30 |
+
"reader": "$monai.data.PILReader",
|
31 |
+
"converter": "$lambda x: x.convert('RGB')"
|
32 |
+
},
|
33 |
+
{
|
34 |
+
"_target_": "EnsureChannelFirstd",
|
35 |
+
"keys": "image"
|
36 |
+
},
|
37 |
+
{
|
38 |
+
"_target_": "CastToTyped",
|
39 |
+
"keys": "image",
|
40 |
+
"dtype": "float32"
|
41 |
+
},
|
42 |
+
{
|
43 |
+
"_target_": "ScaleIntensityRanged",
|
44 |
+
"keys": "image",
|
45 |
+
"a_min": 0.0,
|
46 |
+
"a_max": 255.0,
|
47 |
+
"b_min": 0.0,
|
48 |
+
"b_max": 1.0,
|
49 |
+
"clip": true
|
50 |
+
}
|
51 |
+
]
|
52 |
+
},
|
53 |
+
"data_list": "$[{'image': image} for image in glob.glob(os.path.join(@dataset_dir, '*.png'))]",
|
54 |
+
"dataset": {
|
55 |
+
"_target_": "Dataset",
|
56 |
+
"data": "@data_list",
|
57 |
+
"transform": "@preprocessing"
|
58 |
+
},
|
59 |
+
"dataloader": {
|
60 |
+
"_target_": "DataLoader",
|
61 |
+
"dataset": "@dataset",
|
62 |
+
"batch_size": "@batch_size",
|
63 |
+
"shuffle": false,
|
64 |
+
"num_workers": "@num_cpus",
|
65 |
+
"pin_memory": true
|
66 |
+
},
|
67 |
+
"inferer": {
|
68 |
+
"_target_": "SlidingWindowHoVerNetInferer",
|
69 |
+
"roi_size": "@patch_size",
|
70 |
+
"sw_batch_size": "@sw_batch_size",
|
71 |
+
"overlap": "$1.0 - float(@out_size) / float(@patch_size)",
|
72 |
+
"padding_mode": "constant",
|
73 |
+
"cval": 0,
|
74 |
+
"progress": true,
|
75 |
+
"extra_input_padding": "$((@patch_size - @out_size) // 2,) * 4"
|
76 |
+
},
|
77 |
+
"postprocessing": {
|
78 |
+
"_target_": "Compose",
|
79 |
+
"transforms": [
|
80 |
+
{
|
81 |
+
"_target_": "FlattenSubKeysd",
|
82 |
+
"keys": "pred",
|
83 |
+
"sub_keys": [
|
84 |
+
"horizontal_vertical",
|
85 |
+
"nucleus_prediction",
|
86 |
+
"type_prediction"
|
87 |
+
],
|
88 |
+
"delete_keys": true
|
89 |
+
},
|
90 |
+
{
|
91 |
+
"_target_": "HoVerNetInstanceMapPostProcessingd",
|
92 |
+
"sobel_kernel_size": 21,
|
93 |
+
"marker_threshold": 0.4,
|
94 |
+
"marker_radius": 2
|
95 |
+
},
|
96 |
+
{
|
97 |
+
"_target_": "HoVerNetNuclearTypePostProcessingd"
|
98 |
+
},
|
99 |
+
{
|
100 |
+
"_target_": "FromMetaTensord",
|
101 |
+
"keys": [
|
102 |
+
"image"
|
103 |
+
]
|
104 |
+
},
|
105 |
+
{
|
106 |
+
"_target_": "SaveImaged",
|
107 |
+
"keys": "instance_map",
|
108 |
+
"meta_keys": "image_meta_dict",
|
109 |
+
"output_ext": ".nii.gz",
|
110 |
+
"output_dir": "@output_dir",
|
111 |
+
"output_postfix": "instance_map",
|
112 |
+
"output_dtype": "uint32",
|
113 |
+
"separate_folder": false
|
114 |
+
},
|
115 |
+
{
|
116 |
+
"_target_": "SaveImaged",
|
117 |
+
"keys": "type_map",
|
118 |
+
"meta_keys": "image_meta_dict",
|
119 |
+
"output_ext": ".nii.gz",
|
120 |
+
"output_dir": "@output_dir",
|
121 |
+
"output_postfix": "type_map",
|
122 |
+
"output_dtype": "uint8",
|
123 |
+
"separate_folder": false
|
124 |
+
}
|
125 |
+
]
|
126 |
+
},
|
127 |
+
"handlers": [
|
128 |
+
{
|
129 |
+
"_target_": "CheckpointLoader",
|
130 |
+
"load_path": "$os.path.join(@bundle_root, 'models', 'model.pt')",
|
131 |
+
"map_location": "@device",
|
132 |
+
"load_dict": {
|
133 |
+
"model": "@network"
|
134 |
+
}
|
135 |
+
}
|
136 |
+
],
|
137 |
+
"evaluator": {
|
138 |
+
"_target_": "SupervisedEvaluator",
|
139 |
+
"device": "@device",
|
140 |
+
"val_data_loader": "@dataloader",
|
141 |
+
"val_handlers": "@handlers",
|
142 |
+
"network": "@network",
|
143 |
+
"postprocessing": "@postprocessing",
|
144 |
+
"inferer": "@inferer",
|
145 |
+
"amp": true
|
146 |
+
},
|
147 |
+
"evaluating": [
|
148 |
+
"$setattr(torch.backends.cudnn, 'benchmark', True)",
|
149 |
+
"[email protected]()"
|
150 |
+
]
|
151 |
+
}
|
configs/logging.conf
ADDED
@@ -0,0 +1,21 @@
|
|
|
|
|
|
|
|
|
|
|
|
|
|
|
|
|
|
|
|
|
|
|
|
|
|
|
|
|
|
|
|
|
|
|
|
|
|
|
|
|
|
|
|
|
1 |
+
[loggers]
|
2 |
+
keys=root
|
3 |
+
|
4 |
+
[handlers]
|
5 |
+
keys=consoleHandler
|
6 |
+
|
7 |
+
[formatters]
|
8 |
+
keys=fullFormatter
|
9 |
+
|
10 |
+
[logger_root]
|
11 |
+
level=INFO
|
12 |
+
handlers=consoleHandler
|
13 |
+
|
14 |
+
[handler_consoleHandler]
|
15 |
+
class=StreamHandler
|
16 |
+
level=INFO
|
17 |
+
formatter=fullFormatter
|
18 |
+
args=(sys.stdout,)
|
19 |
+
|
20 |
+
[formatter_fullFormatter]
|
21 |
+
format=%(asctime)s - %(name)s - %(levelname)s - %(message)s
|
configs/metadata.json
ADDED
@@ -0,0 +1,115 @@
|
|
|
|
|
|
|
|
|
|
|
|
|
|
|
|
|
|
|
|
|
|
|
|
|
|
|
|
|
|
|
|
|
|
|
|
|
|
|
|
|
|
|
|
|
|
|
|
|
|
|
|
|
|
|
|
|
|
|
|
|
|
|
|
|
|
|
|
|
|
|
|
|
|
|
|
|
|
|
|
|
|
|
|
|
|
|
|
|
|
|
|
|
|
|
|
|
|
|
|
|
|
|
|
|
|
|
|
|
|
|
|
|
|
|
|
|
|
|
|
|
|
|
|
|
|
|
|
|
|
|
|
|
|
|
|
|
|
|
|
|
|
|
|
|
|
|
|
|
|
|
|
|
|
|
|
|
|
|
|
|
|
|
|
|
|
|
|
|
|
|
|
|
|
|
|
|
|
|
|
|
|
|
|
|
|
|
|
|
|
|
|
|
|
|
|
|
|
|
|
|
|
|
|
|
|
|
|
|
|
|
|
|
|
|
|
|
|
|
|
|
|
|
|
|
|
|
|
|
|
|
|
|
1 |
+
{
|
2 |
+
"schema": "https://github.com/Project-MONAI/MONAI-extra-test-data/releases/download/0.8.1/meta_schema_hovernet_20221124.json",
|
3 |
+
"version": "0.1.0",
|
4 |
+
"changelog": {
|
5 |
+
"0.1.0": "complete the model package"
|
6 |
+
},
|
7 |
+
"monai_version": "1.1.0rc2",
|
8 |
+
"pytorch_version": "1.13.0",
|
9 |
+
"numpy_version": "1.22.2",
|
10 |
+
"optional_packages_version": {
|
11 |
+
"scikit-image": "0.19.3",
|
12 |
+
"scipy": "1.8.1",
|
13 |
+
"tqdm": "4.64.1",
|
14 |
+
"pillow": "9.0.1"
|
15 |
+
},
|
16 |
+
"task": "Nuclear segmentation and classification",
|
17 |
+
"description": "A simultaneous segmentation and classification of nuclei within multitissue histology images based on CoNSeP data",
|
18 |
+
"authors": "MONAI team",
|
19 |
+
"copyright": "Copyright (c) MONAI Consortium",
|
20 |
+
"data_source": "https://warwick.ac.uk/fac/cross_fac/tia/data/hovernet/",
|
21 |
+
"data_type": "numpy",
|
22 |
+
"image_classes": "RGB image with intensity between 0 and 255",
|
23 |
+
"label_classes": "a dictionary contains binary nuclear segmentation, hover map and pixel-level classification",
|
24 |
+
"pred_classes": "a dictionary contains scalar probability for binary nuclear segmentation, hover map and pixel-level classification",
|
25 |
+
"eval_metrics": {
|
26 |
+
"Binary Dice": 0.8293,
|
27 |
+
"PQ": 0.4936,
|
28 |
+
"F1d": 0.748
|
29 |
+
},
|
30 |
+
"intended_use": "This is an example, not to be used for diagnostic purposes",
|
31 |
+
"references": [
|
32 |
+
"Simon Graham. 'HoVer-Net: Simultaneous Segmentation and Classification of Nuclei in Multi-Tissue Histology Images.' Medical Image Analysis, 2019. https://arxiv.org/abs/1812.06499"
|
33 |
+
],
|
34 |
+
"network_data_format": {
|
35 |
+
"inputs": {
|
36 |
+
"image": {
|
37 |
+
"type": "image",
|
38 |
+
"format": "magnitude",
|
39 |
+
"num_channels": 3,
|
40 |
+
"spatial_shape": [
|
41 |
+
"256",
|
42 |
+
"256"
|
43 |
+
],
|
44 |
+
"dtype": "float32",
|
45 |
+
"value_range": [
|
46 |
+
0,
|
47 |
+
255
|
48 |
+
],
|
49 |
+
"is_patch_data": true,
|
50 |
+
"channel_def": {
|
51 |
+
"0": "image"
|
52 |
+
}
|
53 |
+
}
|
54 |
+
},
|
55 |
+
"outputs": {
|
56 |
+
"nucleus_prediction": {
|
57 |
+
"type": "probability",
|
58 |
+
"format": "segmentation",
|
59 |
+
"num_channels": 3,
|
60 |
+
"spatial_shape": [
|
61 |
+
"164",
|
62 |
+
"164"
|
63 |
+
],
|
64 |
+
"dtype": "float32",
|
65 |
+
"value_range": [
|
66 |
+
0,
|
67 |
+
1
|
68 |
+
],
|
69 |
+
"is_patch_data": true,
|
70 |
+
"channel_def": {
|
71 |
+
"0": "background",
|
72 |
+
"1": "nuclei"
|
73 |
+
}
|
74 |
+
},
|
75 |
+
"horizontal_vertical": {
|
76 |
+
"type": "probability",
|
77 |
+
"format": "regression",
|
78 |
+
"num_channels": 2,
|
79 |
+
"spatial_shape": [
|
80 |
+
"164",
|
81 |
+
"164"
|
82 |
+
],
|
83 |
+
"dtype": "float32",
|
84 |
+
"value_range": [
|
85 |
+
0,
|
86 |
+
1
|
87 |
+
],
|
88 |
+
"is_patch_data": true,
|
89 |
+
"channel_def": {
|
90 |
+
"0": "horizontal distances map",
|
91 |
+
"1": "vertical distances map"
|
92 |
+
}
|
93 |
+
},
|
94 |
+
"type_prediction": {
|
95 |
+
"type": "probability",
|
96 |
+
"format": "classification",
|
97 |
+
"num_channels": 2,
|
98 |
+
"spatial_shape": [
|
99 |
+
"164",
|
100 |
+
"164"
|
101 |
+
],
|
102 |
+
"dtype": "float32",
|
103 |
+
"value_range": [
|
104 |
+
0,
|
105 |
+
1
|
106 |
+
],
|
107 |
+
"is_patch_data": true,
|
108 |
+
"channel_def": {
|
109 |
+
"0": "background",
|
110 |
+
"1": "type of nucleus for each pixel"
|
111 |
+
}
|
112 |
+
}
|
113 |
+
}
|
114 |
+
}
|
115 |
+
}
|
configs/multi_gpu_train.json
ADDED
@@ -0,0 +1,36 @@
|
|
|
|
|
|
|
|
|
|
|
|
|
|
|
|
|
|
|
|
|
|
|
|
|
|
|
|
|
|
|
|
|
|
|
|
|
|
|
|
|
|
|
|
|
|
|
|
|
|
|
|
|
|
|
|
|
|
|
|
|
|
|
|
|
|
|
|
|
|
|
|
|
|
|
1 |
+
{
|
2 |
+
"device": "$torch.device(f'cuda:{dist.get_rank()}')",
|
3 |
+
"network": {
|
4 |
+
"_target_": "torch.nn.parallel.DistributedDataParallel",
|
5 |
+
"module": "$@network_def.to(@device)",
|
6 |
+
"device_ids": [
|
7 |
+
"@device"
|
8 |
+
]
|
9 |
+
},
|
10 |
+
"train#sampler": {
|
11 |
+
"_target_": "DistributedSampler",
|
12 |
+
"dataset": "@train#dataset",
|
13 |
+
"even_divisible": true,
|
14 |
+
"shuffle": true
|
15 |
+
},
|
16 |
+
"train#dataloader#sampler": "@train#sampler",
|
17 |
+
"train#dataloader#shuffle": false,
|
18 |
+
"train#trainer#train_handlers": "$@train#train_handlers[: -2 if dist.get_rank() > 0 else None]",
|
19 |
+
"validate#sampler": {
|
20 |
+
"_target_": "DistributedSampler",
|
21 |
+
"dataset": "@validate#dataset",
|
22 |
+
"even_divisible": false,
|
23 |
+
"shuffle": false
|
24 |
+
},
|
25 |
+
"validate#dataloader#sampler": "@validate#sampler",
|
26 |
+
"validate#evaluator#val_handlers": "$None if dist.get_rank() > 0 else @validate#handlers",
|
27 |
+
"training": [
|
28 |
+
"$import torch.distributed as dist",
|
29 |
+
"$dist.init_process_group(backend='nccl')",
|
30 |
+
"$torch.cuda.set_device(@device)",
|
31 |
+
"$monai.utils.set_determinism(seed=321)",
|
32 |
+
"$setattr(torch.backends.cudnn, 'benchmark', True)",
|
33 |
+
"$@train#trainer.run()",
|
34 |
+
"$dist.destroy_process_group()"
|
35 |
+
]
|
36 |
+
}
|
configs/train.json
ADDED
@@ -0,0 +1,525 @@
|
|
|
|
|
|
|
|
|
|
|
|
|
|
|
|
|
|
|
|
|
|
|
|
|
|
|
|
|
|
|
|
|
|
|
|
|
|
|
|
|
|
|
|
|
|
|
|
|
|
|
|
|
|
|
|
|
|
|
|
|
|
|
|
|
|
|
|
|
|
|
|
|
|
|
|
|
|
|
|
|
|
|
|
|
|
|
|
|
|
|
|
|
|
|
|
|
|
|
|
|
|
|
|
|
|
|
|
|
|
|
|
|
|
|
|
|
|
|
|
|
|
|
|
|
|
|
|
|
|
|
|
|
|
|
|
|
|
|
|
|
|
|
|
|
|
|
|
|
|
|
|
|
|
|
|
|
|
|
|
|
|
|
|
|
|
|
|
|
|
|
|
|
|
|
|
|
|
|
|
|
|
|
|
|
|
|
|
|
|
|
|
|
|
|
|
|
|
|
|
|
|
|
|
|
|
|
|
|
|
|
|
|
|
|
|
|
|
|
|
|
|
|
|
|
|
|
|
|
|
|
|
|
|
|
|
|
|
|
|
|
|
|
|
|
|
|
|
|
|
|
|
|
|
|
|
|
|
|
|
|
|
|
|
|
|
|
|
|
|
|
|
|
|
|
|
|
|
|
|
|
|
|
|
|
|
|
|
|
|
|
|
|
|
|
|
|
|
|
|
|
|
|
|
|
|
|
|
|
|
|
|
|
|
|
|
|
|
|
|
|
|
|
|
|
|
|
|
|
|
|
|
|
|
|
|
|
|
|
|
|
|
|
|
|
|
|
|
|
|
|
|
|
|
|
|
|
|
|
|
|
|
|
|
|
|
|
|
|
|
|
|
|
|
|
|
|
|
|
|
|
|
|
|
|
|
|
|
|
|
|
|
|
|
|
|
|
|
|
|
|
|
|
|
|
|
|
|
|
|
|
|
|
|
|
|
|
|
|
|
|
|
|
|
|
|
|
|
|
|
|
|
|
|
|
|
|
|
|
|
|
|
|
|
|
|
|
|
|
|
|
|
|
|
|
|
|
|
|
|
|
|
|
|
|
|
|
|
|
|
|
|
|
|
|
|
|
|
|
|
|
|
|
|
|
|
|
|
|
|
|
|
|
|
|
|
|
|
|
|
|
|
|
|
|
|
|
|
|
|
|
|
|
|
|
|
|
|
|
|
|
|
|
|
|
|
|
|
|
|
|
|
|
|
|
|
|
|
|
|
|
|
|
|
|
|
|
|
|
|
|
|
|
|
|
|
|
|
|
|
|
|
|
|
|
|
|
|
|
|
|
|
|
|
|
|
|
|
|
|
|
|
|
|
|
|
|
|
|
|
|
|
|
|
|
|
|
|
|
|
|
|
|
|
|
|
|
|
|
|
|
|
|
|
|
|
|
|
|
|
|
|
|
|
|
|
|
|
|
|
|
|
|
|
|
|
|
|
|
|
|
|
|
|
|
|
|
|
|
|
|
|
|
|
|
|
|
|
|
|
|
|
|
|
|
|
|
|
|
|
|
|
|
|
|
|
|
|
|
|
|
|
|
|
|
|
|
|
|
|
|
|
|
|
|
|
|
|
|
|
|
|
|
|
|
|
|
|
|
|
|
|
|
|
|
|
|
|
|
|
|
|
|
|
|
|
|
|
|
|
|
|
|
|
|
|
|
|
|
|
|
|
|
|
|
|
|
|
|
|
|
|
|
|
|
|
|
|
|
|
|
|
|
|
|
|
|
|
|
|
|
|
|
|
|
|
|
|
|
|
|
|
|
|
|
|
|
|
|
|
|
|
|
|
|
|
|
|
|
|
|
|
|
|
|
|
|
|
|
|
|
|
|
|
|
|
|
|
|
|
|
|
|
|
|
|
|
|
|
|
|
|
|
|
|
|
|
|
|
|
|
|
|
|
|
|
|
|
|
|
|
|
|
|
|
|
|
|
|
|
|
|
|
|
|
|
|
|
|
|
|
|
|
|
|
|
|
|
|
|
|
|
|
|
|
|
|
|
|
|
|
|
|
|
|
|
|
|
|
|
|
|
|
|
|
|
|
|
|
|
|
|
|
|
|
|
|
|
|
|
|
|
|
|
|
|
|
|
|
|
|
|
|
|
|
|
|
|
|
|
|
|
|
|
|
|
|
|
|
|
|
|
|
|
|
|
|
|
|
|
|
|
|
|
|
|
|
|
|
|
|
|
|
|
|
|
|
|
|
|
|
|
|
|
|
|
|
|
|
|
|
|
|
|
|
|
|
|
|
|
|
|
|
|
|
|
|
|
|
|
|
|
|
|
|
|
|
|
|
|
|
|
|
|
|
|
|
|
|
|
|
|
|
|
|
|
|
|
|
|
|
|
|
|
|
|
|
|
|
|
|
|
|
1 |
+
{
|
2 |
+
"imports": [
|
3 |
+
"$import glob",
|
4 |
+
"$import os",
|
5 |
+
"$import skimage"
|
6 |
+
],
|
7 |
+
"bundle_root": "$os.getcwd()",
|
8 |
+
"ckpt_dir_stage0": "$os.path.join(@bundle_root, 'models', 'stage0')",
|
9 |
+
"ckpt_dir_stage1": "$os.path.join(@bundle_root, 'models')",
|
10 |
+
"ckpt_path_stage0": "$os.path.join(@ckpt_dir_stage0, 'model.pt')",
|
11 |
+
"output_dir": "$os.path.join(@bundle_root, 'eval')",
|
12 |
+
"dataset_dir": "/workspace/Data/Pathology/CoNSeP/Prepared/",
|
13 |
+
"train_images": "$list(sorted(glob.glob(@dataset_dir + '/Train/*image.npy')))",
|
14 |
+
"val_images": "$list(sorted(glob.glob(@dataset_dir + '/Test/*image.npy')))",
|
15 |
+
"train_inst_map": "$list(sorted(glob.glob(@dataset_dir + '/Train/*inst_map.npy')))",
|
16 |
+
"val_inst_map": "$list(sorted(glob.glob(@dataset_dir + '/Test/*inst_map.npy')))",
|
17 |
+
"train_type_map": "$list(sorted(glob.glob(@dataset_dir + '/Train/*type_map.npy')))",
|
18 |
+
"val_type_map": "$list(sorted(glob.glob(@dataset_dir + '/Test/*type_map.npy')))",
|
19 |
+
"device": "$torch.device('cuda:0' if torch.cuda.is_available() else 'cpu')",
|
20 |
+
"stage": 0,
|
21 |
+
"epochs": 50,
|
22 |
+
"val_interval": 1,
|
23 |
+
"learning_rate": 0.0001,
|
24 |
+
"amp": true,
|
25 |
+
"hovernet_mode": "fast",
|
26 |
+
"patch_size": 256,
|
27 |
+
"out_size": 164,
|
28 |
+
"ckpt_dir": "$@ckpt_dir_stage0 if @stage == 0 else @ckpt_dir_stage1",
|
29 |
+
"network_def": {
|
30 |
+
"_target_": "HoVerNet",
|
31 |
+
"mode": "@hovernet_mode",
|
32 |
+
"in_channels": 3,
|
33 |
+
"out_classes": 5,
|
34 |
+
"adapt_standard_resnet": true,
|
35 |
+
"pretrained_url": "$None",
|
36 |
+
"freeze_encoder": true
|
37 |
+
},
|
38 |
+
"network": "$@network_def.to(@device)",
|
39 |
+
"loss": {
|
40 |
+
"_target_": "HoVerNetLoss",
|
41 |
+
"lambda_hv_mse": 1.0
|
42 |
+
},
|
43 |
+
"optimizer": {
|
44 |
+
"_target_": "torch.optim.Adam",
|
45 |
+
"params": "$filter(lambda p: p.requires_grad, @network.parameters())",
|
46 |
+
"lr": "@learning_rate",
|
47 |
+
"weight_decay": 1e-05
|
48 |
+
},
|
49 |
+
"lr_scheduler": {
|
50 |
+
"_target_": "torch.optim.lr_scheduler.StepLR",
|
51 |
+
"optimizer": "@optimizer",
|
52 |
+
"step_size": 25
|
53 |
+
},
|
54 |
+
"train": {
|
55 |
+
"preprocessing_transforms": [
|
56 |
+
{
|
57 |
+
"_target_": "LoadImaged",
|
58 |
+
"keys": [
|
59 |
+
"image",
|
60 |
+
"label_inst",
|
61 |
+
"label_type"
|
62 |
+
]
|
63 |
+
},
|
64 |
+
{
|
65 |
+
"_target_": "EnsureChannelFirstd",
|
66 |
+
"keys": [
|
67 |
+
"image",
|
68 |
+
"label_inst",
|
69 |
+
"label_type"
|
70 |
+
],
|
71 |
+
"channel_dim": -1
|
72 |
+
},
|
73 |
+
{
|
74 |
+
"_target_": "Lambdad",
|
75 |
+
"keys": "label_inst",
|
76 |
+
"func": "$lambda x: skimage.measure.label(x)"
|
77 |
+
},
|
78 |
+
{
|
79 |
+
"_target_": "RandAffined",
|
80 |
+
"keys": [
|
81 |
+
"image",
|
82 |
+
"label_inst",
|
83 |
+
"label_type"
|
84 |
+
],
|
85 |
+
"prob": 1.0,
|
86 |
+
"rotate_range": [
|
87 |
+
"$np.pi"
|
88 |
+
],
|
89 |
+
"scale_range": [
|
90 |
+
[
|
91 |
+
-0.2,
|
92 |
+
0.2
|
93 |
+
],
|
94 |
+
[
|
95 |
+
-0.2,
|
96 |
+
0.2
|
97 |
+
]
|
98 |
+
],
|
99 |
+
"shear_range": [
|
100 |
+
[
|
101 |
+
-0.05,
|
102 |
+
0.05
|
103 |
+
],
|
104 |
+
[
|
105 |
+
-0.05,
|
106 |
+
0.05
|
107 |
+
]
|
108 |
+
],
|
109 |
+
"translate_range": [
|
110 |
+
[
|
111 |
+
-6,
|
112 |
+
6
|
113 |
+
],
|
114 |
+
[
|
115 |
+
-6,
|
116 |
+
6
|
117 |
+
]
|
118 |
+
],
|
119 |
+
"padding_mode": "zeros",
|
120 |
+
"mode": "nearest"
|
121 |
+
},
|
122 |
+
{
|
123 |
+
"_target_": "CenterSpatialCropd",
|
124 |
+
"keys": [
|
125 |
+
"image"
|
126 |
+
],
|
127 |
+
"roi_size": [
|
128 |
+
"@patch_size",
|
129 |
+
"@patch_size"
|
130 |
+
]
|
131 |
+
},
|
132 |
+
{
|
133 |
+
"_target_": "RandFlipd",
|
134 |
+
"keys": [
|
135 |
+
"image",
|
136 |
+
"label_inst",
|
137 |
+
"label_type"
|
138 |
+
],
|
139 |
+
"prob": 0.5,
|
140 |
+
"spatial_axis": 0
|
141 |
+
},
|
142 |
+
{
|
143 |
+
"_target_": "RandFlipd",
|
144 |
+
"keys": [
|
145 |
+
"image",
|
146 |
+
"label_inst",
|
147 |
+
"label_type"
|
148 |
+
],
|
149 |
+
"prob": 0.5,
|
150 |
+
"spatial_axis": 1
|
151 |
+
},
|
152 |
+
{
|
153 |
+
"_target_": "OneOf",
|
154 |
+
"transforms": [
|
155 |
+
{
|
156 |
+
"_target_": "RandGaussianSmoothd",
|
157 |
+
"keys": [
|
158 |
+
"image"
|
159 |
+
],
|
160 |
+
"sigma_x": [
|
161 |
+
0.1,
|
162 |
+
1.1
|
163 |
+
],
|
164 |
+
"sigma_y": [
|
165 |
+
0.1,
|
166 |
+
1.1
|
167 |
+
],
|
168 |
+
"prob": 1.0
|
169 |
+
},
|
170 |
+
{
|
171 |
+
"_target_": "MedianSmoothd",
|
172 |
+
"keys": [
|
173 |
+
"image"
|
174 |
+
],
|
175 |
+
"radius": 1
|
176 |
+
},
|
177 |
+
{
|
178 |
+
"_target_": "RandGaussianNoised",
|
179 |
+
"keys": [
|
180 |
+
"image"
|
181 |
+
],
|
182 |
+
"std": 0.05,
|
183 |
+
"prob": 1.0
|
184 |
+
}
|
185 |
+
]
|
186 |
+
},
|
187 |
+
{
|
188 |
+
"_target_": "CastToTyped",
|
189 |
+
"keys": "image",
|
190 |
+
"dtype": "$np.uint8"
|
191 |
+
},
|
192 |
+
{
|
193 |
+
"_target_": "TorchVisiond",
|
194 |
+
"keys": "image",
|
195 |
+
"name": "ColorJitter",
|
196 |
+
"brightness": [
|
197 |
+
0.9,
|
198 |
+
1.0
|
199 |
+
],
|
200 |
+
"contrast": [
|
201 |
+
0.95,
|
202 |
+
1.1
|
203 |
+
],
|
204 |
+
"saturation": [
|
205 |
+
0.8,
|
206 |
+
1.2
|
207 |
+
],
|
208 |
+
"hue": [
|
209 |
+
-0.04,
|
210 |
+
0.04
|
211 |
+
]
|
212 |
+
},
|
213 |
+
{
|
214 |
+
"_target_": "AsDiscreted",
|
215 |
+
"keys": "label_type",
|
216 |
+
"to_onehot": 5
|
217 |
+
},
|
218 |
+
{
|
219 |
+
"_target_": "ScaleIntensityRanged",
|
220 |
+
"keys": "image",
|
221 |
+
"a_min": 0.0,
|
222 |
+
"a_max": 255.0,
|
223 |
+
"b_min": 0.0,
|
224 |
+
"b_max": 1.0,
|
225 |
+
"clip": true
|
226 |
+
},
|
227 |
+
{
|
228 |
+
"_target_": "CastToTyped",
|
229 |
+
"keys": "label_inst",
|
230 |
+
"dtype": "$torch.int"
|
231 |
+
},
|
232 |
+
{
|
233 |
+
"_target_": "ComputeHoVerMapsd",
|
234 |
+
"keys": "label_inst"
|
235 |
+
},
|
236 |
+
{
|
237 |
+
"_target_": "Lambdad",
|
238 |
+
"keys": "label_inst",
|
239 |
+
"func": "$lambda x: x > 0",
|
240 |
+
"overwrite": "label"
|
241 |
+
},
|
242 |
+
{
|
243 |
+
"_target_": "CenterSpatialCropd",
|
244 |
+
"keys": [
|
245 |
+
"label",
|
246 |
+
"hover_label_inst",
|
247 |
+
"label_inst",
|
248 |
+
"label_type"
|
249 |
+
],
|
250 |
+
"roi_size": [
|
251 |
+
"@out_size",
|
252 |
+
"@out_size"
|
253 |
+
]
|
254 |
+
},
|
255 |
+
{
|
256 |
+
"_target_": "AsDiscreted",
|
257 |
+
"keys": "label",
|
258 |
+
"to_onehot": 2
|
259 |
+
},
|
260 |
+
{
|
261 |
+
"_target_": "CastToTyped",
|
262 |
+
"keys": [
|
263 |
+
"image",
|
264 |
+
"label_inst",
|
265 |
+
"label_type"
|
266 |
+
],
|
267 |
+
"dtype": "$torch.float32"
|
268 |
+
}
|
269 |
+
],
|
270 |
+
"preprocessing": {
|
271 |
+
"_target_": "Compose",
|
272 |
+
"transforms": "$@train#preprocessing_transforms"
|
273 |
+
},
|
274 |
+
"dataset": {
|
275 |
+
"_target_": "Dataset",
|
276 |
+
"data": "$[{'image': i, 'label_inst': j, 'label_type': k} for i, j, k in zip(@train_images, @train_inst_map, @train_type_map)]",
|
277 |
+
"transform": "@train#preprocessing"
|
278 |
+
},
|
279 |
+
"dataloader": {
|
280 |
+
"_target_": "DataLoader",
|
281 |
+
"dataset": "@train#dataset",
|
282 |
+
"batch_size": 16,
|
283 |
+
"shuffle": true,
|
284 |
+
"num_workers": 4
|
285 |
+
},
|
286 |
+
"inferer": {
|
287 |
+
"_target_": "SimpleInferer"
|
288 |
+
},
|
289 |
+
"postprocessing_np": {
|
290 |
+
"_target_": "Compose",
|
291 |
+
"transforms": [
|
292 |
+
{
|
293 |
+
"_target_": "Activationsd",
|
294 |
+
"keys": "nucleus_prediction",
|
295 |
+
"softmax": true
|
296 |
+
},
|
297 |
+
{
|
298 |
+
"_target_": "AsDiscreted",
|
299 |
+
"keys": "nucleus_prediction",
|
300 |
+
"argmax": true
|
301 |
+
}
|
302 |
+
]
|
303 |
+
},
|
304 |
+
"postprocessing": {
|
305 |
+
"_target_": "Lambdad",
|
306 |
+
"keys": "pred",
|
307 |
+
"func": "$@train#postprocessing_np"
|
308 |
+
},
|
309 |
+
"handlers": [
|
310 |
+
{
|
311 |
+
"_target_": "LrScheduleHandler",
|
312 |
+
"lr_scheduler": "@lr_scheduler",
|
313 |
+
"print_lr": true
|
314 |
+
},
|
315 |
+
{
|
316 |
+
"_target_": "ValidationHandler",
|
317 |
+
"validator": "@validate#evaluator",
|
318 |
+
"epoch_level": true,
|
319 |
+
"interval": "@val_interval"
|
320 |
+
},
|
321 |
+
{
|
322 |
+
"_target_": "CheckpointSaver",
|
323 |
+
"save_dir": "@ckpt_dir",
|
324 |
+
"save_dict": {
|
325 |
+
"model": "@network"
|
326 |
+
},
|
327 |
+
"save_interval": 10,
|
328 |
+
"epoch_level": true,
|
329 |
+
"save_final": true,
|
330 |
+
"final_filename": "model.pt"
|
331 |
+
},
|
332 |
+
{
|
333 |
+
"_target_": "StatsHandler",
|
334 |
+
"tag_name": "train_loss",
|
335 |
+
"output_transform": "$monai.handlers.from_engine(['loss'], first=True)"
|
336 |
+
},
|
337 |
+
{
|
338 |
+
"_target_": "TensorBoardStatsHandler",
|
339 |
+
"log_dir": "@output_dir",
|
340 |
+
"tag_name": "train_loss",
|
341 |
+
"output_transform": "$monai.handlers.from_engine(['loss'], first=True)"
|
342 |
+
}
|
343 |
+
],
|
344 |
+
"extra_handlers": [
|
345 |
+
{
|
346 |
+
"_target_": "CheckpointLoader",
|
347 |
+
"load_path": "$os.path.join(@ckpt_dir_stage0, 'model.pt')",
|
348 |
+
"load_dict": {
|
349 |
+
"model": "@network"
|
350 |
+
}
|
351 |
+
}
|
352 |
+
],
|
353 |
+
"train_handlers": "$@train#extra_handlers + @train#handlers if @stage==1 else @train#handlers",
|
354 |
+
"key_metric": {
|
355 |
+
"train_mean_dice": {
|
356 |
+
"_target_": "MeanDice",
|
357 |
+
"include_background": false,
|
358 |
+
"output_transform": "$monai.apps.pathology.handlers.utils.from_engine_hovernet(keys=['pred', 'label'], nested_key='nucleus_prediction')"
|
359 |
+
}
|
360 |
+
},
|
361 |
+
"trainer": {
|
362 |
+
"_target_": "SupervisedTrainer",
|
363 |
+
"max_epochs": "@epochs",
|
364 |
+
"device": "@device",
|
365 |
+
"train_data_loader": "@train#dataloader",
|
366 |
+
"prepare_batch": "$monai.apps.pathology.engines.utils.PrepareBatchHoVerNet(extra_keys=['label_type', 'hover_label_inst'])",
|
367 |
+
"network": "@network",
|
368 |
+
"loss_function": "@loss",
|
369 |
+
"optimizer": "@optimizer",
|
370 |
+
"inferer": "@train#inferer",
|
371 |
+
"postprocessing": "@train#postprocessing",
|
372 |
+
"key_train_metric": "@train#key_metric",
|
373 |
+
"train_handlers": "@train#train_handlers",
|
374 |
+
"amp": "@amp"
|
375 |
+
}
|
376 |
+
},
|
377 |
+
"validate": {
|
378 |
+
"preprocessing_transforms": [
|
379 |
+
{
|
380 |
+
"_target_": "LoadImaged",
|
381 |
+
"keys": [
|
382 |
+
"image",
|
383 |
+
"label_inst",
|
384 |
+
"label_type"
|
385 |
+
]
|
386 |
+
},
|
387 |
+
{
|
388 |
+
"_target_": "EnsureChannelFirstd",
|
389 |
+
"keys": [
|
390 |
+
"image",
|
391 |
+
"label_inst",
|
392 |
+
"label_type"
|
393 |
+
],
|
394 |
+
"channel_dim": -1
|
395 |
+
},
|
396 |
+
{
|
397 |
+
"_target_": "Lambdad",
|
398 |
+
"keys": "label_inst",
|
399 |
+
"func": "$lambda x: skimage.measure.label(x)"
|
400 |
+
},
|
401 |
+
{
|
402 |
+
"_target_": "CastToTyped",
|
403 |
+
"keys": [
|
404 |
+
"image",
|
405 |
+
"label_inst"
|
406 |
+
],
|
407 |
+
"dtype": "$torch.int"
|
408 |
+
},
|
409 |
+
{
|
410 |
+
"_target_": "CenterSpatialCropd",
|
411 |
+
"keys": [
|
412 |
+
"image"
|
413 |
+
],
|
414 |
+
"roi_size": [
|
415 |
+
"@patch_size",
|
416 |
+
"@patch_size"
|
417 |
+
]
|
418 |
+
},
|
419 |
+
{
|
420 |
+
"_target_": "ScaleIntensityRanged",
|
421 |
+
"keys": "image",
|
422 |
+
"a_min": 0.0,
|
423 |
+
"a_max": 255.0,
|
424 |
+
"b_min": 0.0,
|
425 |
+
"b_max": 1.0,
|
426 |
+
"clip": true
|
427 |
+
},
|
428 |
+
{
|
429 |
+
"_target_": "ComputeHoVerMapsd",
|
430 |
+
"keys": "label_inst"
|
431 |
+
},
|
432 |
+
{
|
433 |
+
"_target_": "Lambdad",
|
434 |
+
"keys": "label_inst",
|
435 |
+
"func": "$lambda x: x > 0",
|
436 |
+
"overwrite": "label"
|
437 |
+
},
|
438 |
+
{
|
439 |
+
"_target_": "CenterSpatialCropd",
|
440 |
+
"keys": [
|
441 |
+
"label",
|
442 |
+
"hover_label_inst",
|
443 |
+
"label_inst",
|
444 |
+
"label_type"
|
445 |
+
],
|
446 |
+
"roi_size": [
|
447 |
+
"@out_size",
|
448 |
+
"@out_size"
|
449 |
+
]
|
450 |
+
},
|
451 |
+
{
|
452 |
+
"_target_": "CastToTyped",
|
453 |
+
"keys": [
|
454 |
+
"image",
|
455 |
+
"label_inst",
|
456 |
+
"label_type"
|
457 |
+
],
|
458 |
+
"dtype": "$torch.float32"
|
459 |
+
}
|
460 |
+
],
|
461 |
+
"preprocessing": {
|
462 |
+
"_target_": "Compose",
|
463 |
+
"transforms": "$@validate#preprocessing_transforms"
|
464 |
+
},
|
465 |
+
"dataset": {
|
466 |
+
"_target_": "Dataset",
|
467 |
+
"data": "$[{'image': i, 'label_inst': j, 'label_type': k} for i, j, k in zip(@val_images, @val_inst_map, @val_type_map)]",
|
468 |
+
"transform": "@validate#preprocessing"
|
469 |
+
},
|
470 |
+
"dataloader": {
|
471 |
+
"_target_": "DataLoader",
|
472 |
+
"dataset": "@validate#dataset",
|
473 |
+
"batch_size": 16,
|
474 |
+
"shuffle": false,
|
475 |
+
"num_workers": 4
|
476 |
+
},
|
477 |
+
"inferer": {
|
478 |
+
"_target_": "SimpleInferer"
|
479 |
+
},
|
480 |
+
"postprocessing": "$@train#postprocessing",
|
481 |
+
"handlers": [
|
482 |
+
{
|
483 |
+
"_target_": "StatsHandler",
|
484 |
+
"iteration_log": false
|
485 |
+
},
|
486 |
+
{
|
487 |
+
"_target_": "TensorBoardStatsHandler",
|
488 |
+
"log_dir": "@output_dir",
|
489 |
+
"iteration_log": false
|
490 |
+
},
|
491 |
+
{
|
492 |
+
"_target_": "CheckpointSaver",
|
493 |
+
"save_dir": "@ckpt_dir",
|
494 |
+
"save_dict": {
|
495 |
+
"model": "@network"
|
496 |
+
},
|
497 |
+
"save_key_metric": true
|
498 |
+
}
|
499 |
+
],
|
500 |
+
"key_metric": {
|
501 |
+
"val_mean_dice": {
|
502 |
+
"_target_": "MeanDice",
|
503 |
+
"include_background": false,
|
504 |
+
"output_transform": "$monai.apps.pathology.handlers.utils.from_engine_hovernet(keys=['pred', 'label'], nested_key='nucleus_prediction')"
|
505 |
+
}
|
506 |
+
},
|
507 |
+
"evaluator": {
|
508 |
+
"_target_": "SupervisedEvaluator",
|
509 |
+
"device": "@device",
|
510 |
+
"val_data_loader": "@validate#dataloader",
|
511 |
+
"prepare_batch": "$monai.apps.pathology.engines.utils.PrepareBatchHoVerNet(extra_keys=['label_type', 'hover_label_inst'])",
|
512 |
+
"network": "@network",
|
513 |
+
"inferer": "@validate#inferer",
|
514 |
+
"postprocessing": "@validate#postprocessing",
|
515 |
+
"key_val_metric": "@validate#key_metric",
|
516 |
+
"val_handlers": "@validate#handlers",
|
517 |
+
"amp": "@amp"
|
518 |
+
}
|
519 |
+
},
|
520 |
+
"training": [
|
521 |
+
"$monai.utils.set_determinism(seed=321)",
|
522 |
+
"$setattr(torch.backends.cudnn, 'benchmark', True)",
|
523 |
+
"$@train#trainer.run()"
|
524 |
+
]
|
525 |
+
}
|
docs/README.md
ADDED
@@ -0,0 +1,147 @@
|
|
|
|
|
|
|
|
|
|
|
|
|
|
|
|
|
|
|
|
|
|
|
|
|
|
|
|
|
|
|
|
|
|
|
|
|
|
|
|
|
|
|
|
|
|
|
|
|
|
|
|
|
|
|
|
|
|
|
|
|
|
|
|
|
|
|
|
|
|
|
|
|
|
|
|
|
|
|
|
|
|
|
|
|
|
|
|
|
|
|
|
|
|
|
|
|
|
|
|
|
|
|
|
|
|
|
|
|
|
|
|
|
|
|
|
|
|
|
|
|
|
|
|
|
|
|
|
|
|
|
|
|
|
|
|
|
|
|
|
|
|
|
|
|
|
|
|
|
|
|
|
|
|
|
|
|
|
|
|
|
|
|
|
|
|
|
|
|
|
|
|
|
|
|
|
|
|
|
|
|
|
|
|
|
|
|
|
|
|
|
|
|
|
|
|
|
|
|
|
|
|
|
|
|
|
|
|
|
|
|
|
|
|
|
|
|
|
|
|
|
|
|
|
|
|
|
|
|
|
|
|
|
|
|
|
|
|
|
|
|
|
|
|
|
|
|
|
|
|
|
|
|
|
|
|
|
|
|
|
|
|
|
|
|
|
|
|
|
|
|
|
|
|
|
|
|
|
|
|
|
|
|
|
|
|
|
|
|
|
|
|
|
|
|
|
|
1 |
+
# Model Overview
|
2 |
+
|
3 |
+
A pre-trained model for simultaneous segmentation and classification of nuclei within multi-tissue histology images based on CoNSeP data. The details of the model can be found in [1].
|
4 |
+
|
5 |
+
## Workflow
|
6 |
+
|
7 |
+
The model is trained to simultaneous segment and classify nuclei. Training is done via a two-stage approach. First initialized the model with pre-trained weights on the [ImageNet dataset](https://ieeexplore.ieee.org/document/5206848), trained only the decoders for the first 50 epochs, and then fine-tuned all layers for another 50 epochs. There are two training modes in total. If "original" mode is specified, it uses [270, 270] and [80, 80] for `patch_size` and `out_size` respectively. If "fast" mode is specified, it uses [256, 256] and [164, 164] for `patch_size` and `out_size` respectively. The results we show below are based on the "fast" model.
|
8 |
+
|
9 |
+
- We train the first stage with pre-trained weights from some internal data.
|
10 |
+
|
11 |
+
- The original author's repo also has pre-trained weights which is for non-commercial use. Each user is responsible for checking the content of models/datasets and the applicable licenses and determining if suitable for the intended use. The license for the pre-trained model is different than MONAI license. Please check the source where these weights are obtained from: <https://github.com/vqdang/hover_net#data-format>
|
12 |
+
|
13 |
+
`PRETRAIN_MODEL_URL` is "https://drive.google.com/u/1/uc?id=1KntZge40tAHgyXmHYVqZZ5d2p_4Qr2l5&export=download" which can be used in bash code below.
|
14 |
+
|
15 |
+

|
16 |
+
|
17 |
+
## Data
|
18 |
+
|
19 |
+
The training data is from <https://warwick.ac.uk/fac/cross_fac/tia/data/hovernet/>.
|
20 |
+
|
21 |
+
- Target: segment instance-level nuclei and classify the nuclei type
|
22 |
+
- Task: Segmentation and classification
|
23 |
+
- Modality: RGB images
|
24 |
+
- Size: 41 image tiles (2009 patches)
|
25 |
+
|
26 |
+
The provided labelled data was partitioned, based on the original split, into training (27 tiles) and testing (14 tiles) datasets.
|
27 |
+
|
28 |
+
After download the datasets, please run `scripts/prepare_patches.py` to prepare patches from tiles. Prepared patches are saved in `your-concep-dataset-path`/Prepared. The implementation is referring to <https://github.com/vqdang/hover_net/blob/master/extract_patches.py>. The command is like:
|
29 |
+
|
30 |
+
```
|
31 |
+
python scripts/prepare_patches.py -root your-concep-dataset-path
|
32 |
+
```
|
33 |
+
|
34 |
+
## Training configuration
|
35 |
+
|
36 |
+
This model utilized a two-stage approach. The training was performed with the following:
|
37 |
+
|
38 |
+
- GPU: At least 24GB of GPU memory.
|
39 |
+
- Actual Model Input: 256 x 256
|
40 |
+
- AMP: True
|
41 |
+
- Optimizer: Adam
|
42 |
+
- Learning Rate: 1e-4
|
43 |
+
- Loss: HoVerNetLoss
|
44 |
+
|
45 |
+
## Input
|
46 |
+
|
47 |
+
Input: RGB images
|
48 |
+
|
49 |
+
## Output
|
50 |
+
|
51 |
+
Output: a dictionary with the following keys:
|
52 |
+
|
53 |
+
1. nucleus_prediction: predict whether or not a pixel belongs to the nuclei or background
|
54 |
+
2. horizontal_vertical: predict the horizontal and vertical distances of nuclear pixels to their centres of mass
|
55 |
+
3. type_prediction: predict the type of nucleus for each pixel
|
56 |
+
|
57 |
+
## Model Performance
|
58 |
+
|
59 |
+
The achieved metrics on the validation data are:
|
60 |
+
|
61 |
+
Fast mode:
|
62 |
+
- Binary Dice: 0.8293
|
63 |
+
- PQ: 0.4936
|
64 |
+
- F1d: 0.7480
|
65 |
+
|
66 |
+
#### Training Loss and Dice
|
67 |
+
|
68 |
+
stage1:
|
69 |
+
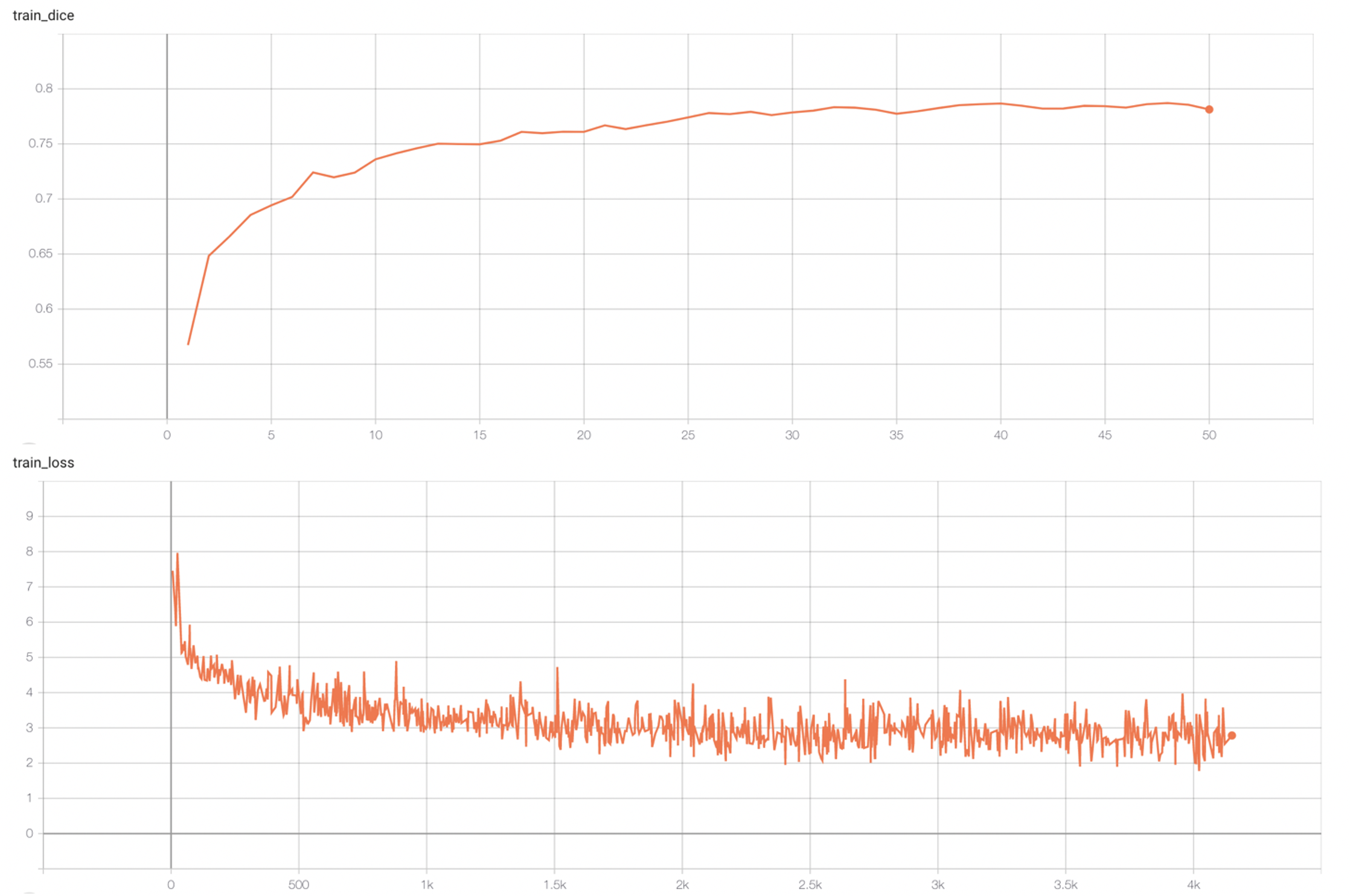
|
70 |
+
|
71 |
+
stage2:
|
72 |
+
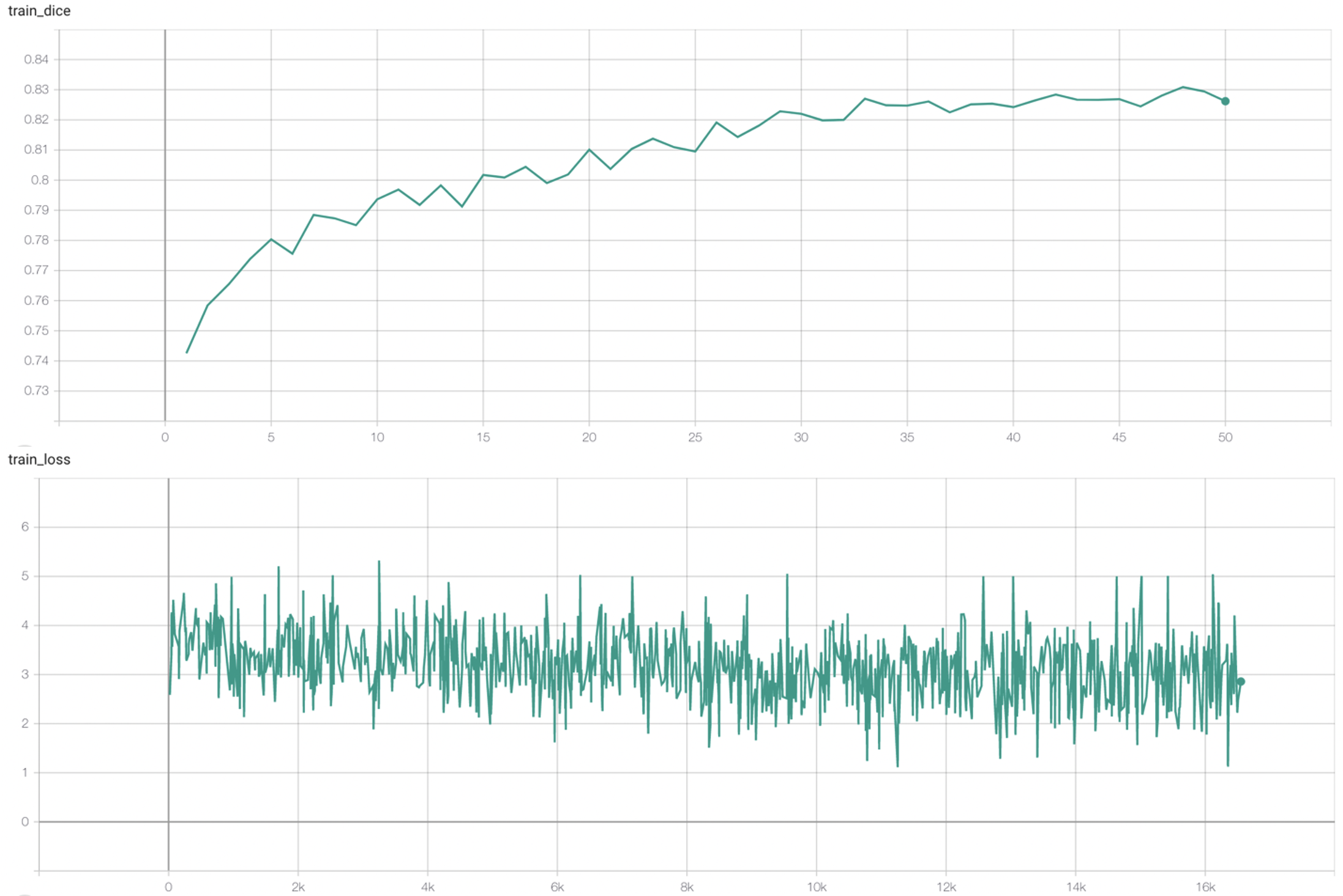
|
73 |
+
|
74 |
+
#### Validation Dice
|
75 |
+
|
76 |
+
stage1:
|
77 |
+
|
78 |
+
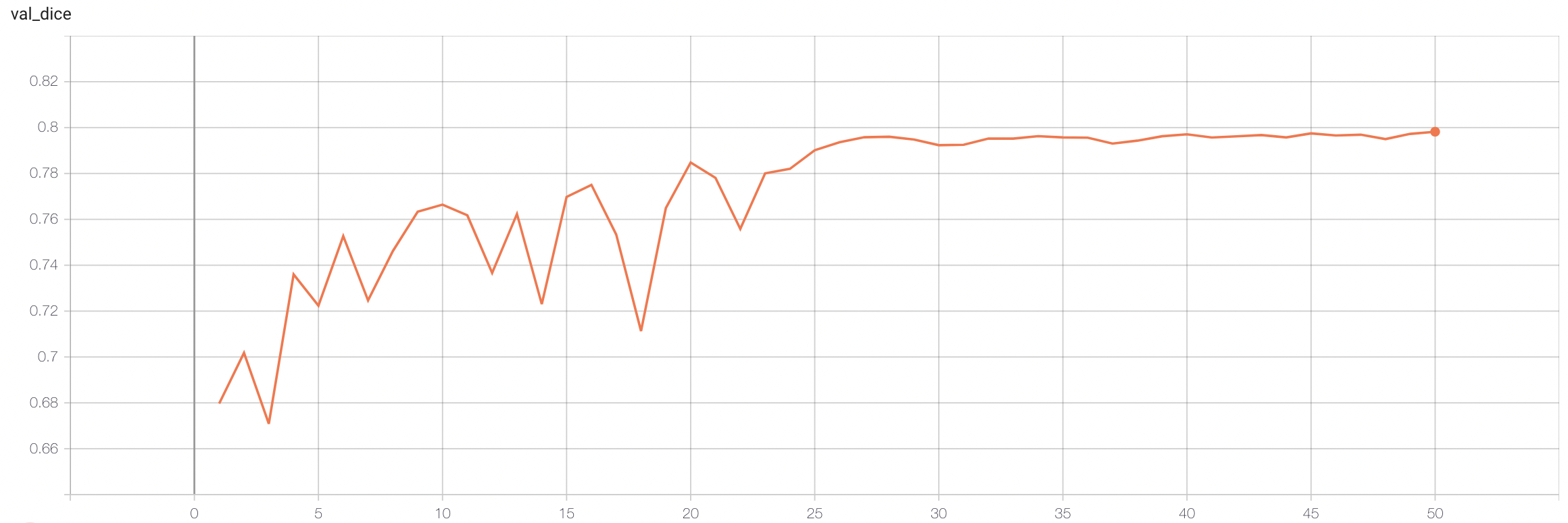
|
79 |
+
|
80 |
+
stage2:
|
81 |
+
|
82 |
+
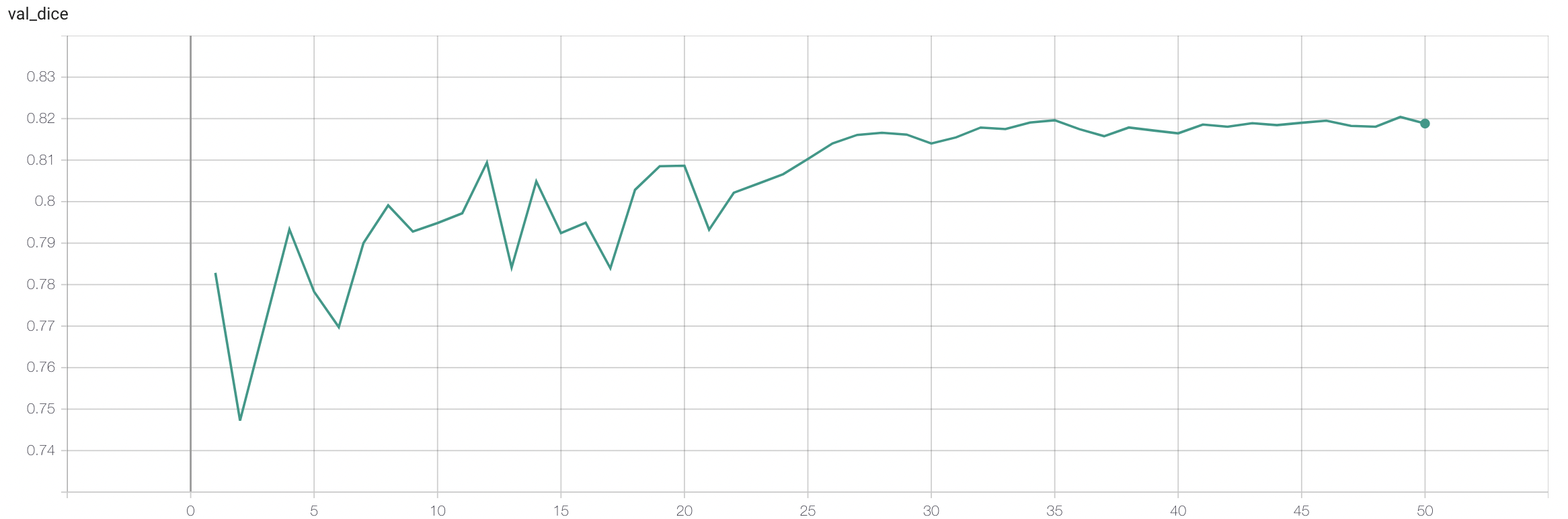
|
83 |
+
|
84 |
+
## commands example
|
85 |
+
|
86 |
+
Execute training:
|
87 |
+
|
88 |
+
- Run first stage
|
89 |
+
|
90 |
+
```
|
91 |
+
python -m monai.bundle run training --meta_file configs/metadata.json --config_file configs/train.json --logging_file configs/logging.conf --network_def#pretrained_url `PRETRAIN_MODEL_URL` --stage 0
|
92 |
+
```
|
93 |
+
|
94 |
+
- Run second stage
|
95 |
+
|
96 |
+
```
|
97 |
+
python -m monai.bundle run training --meta_file configs/metadata.json --config_file configs/train.json --logging_file configs/logging.conf --network_def#freeze_encoder false --network_def#pretrained_url None --stage 1
|
98 |
+
```
|
99 |
+
|
100 |
+
Override the `train` config to execute multi-GPU training:
|
101 |
+
|
102 |
+
- Run first stage
|
103 |
+
|
104 |
+
```
|
105 |
+
torchrun --standalone --nnodes=1 --nproc_per_node=2 -m monai.bundle run training --meta_file configs/metadata.json --config_file "['configs/train.json','configs/multi_gpu_train.json']" --logging_file configs/logging.conf --train#dataloader#batch_size 8 --network_def#freeze_encoder true --network_def#pretrained_url `PRETRAIN_MODEL_URL` --stage 0
|
106 |
+
```
|
107 |
+
|
108 |
+
- Run second stage
|
109 |
+
|
110 |
+
```
|
111 |
+
torchrun --standalone --nnodes=1 --nproc_per_node=2 -m monai.bundle run training --meta_file configs/metadata.json --config_file "['configs/train.json','configs/multi_gpu_train.json']" --logging_file configs/logging.conf --train#dataloader#batch_size 4 --network_def#freeze_encoder false --network_def#pretrained_url None --stage 1
|
112 |
+
```
|
113 |
+
|
114 |
+
Override the `train` config to execute evaluation with the trained model:
|
115 |
+
|
116 |
+
```
|
117 |
+
python -m monai.bundle run evaluating --meta_file configs/metadata.json --config_file "['configs/train.json','configs/evaluate.json']" --logging_file configs/logging.conf
|
118 |
+
```
|
119 |
+
|
120 |
+
### Execute inference
|
121 |
+
|
122 |
+
```
|
123 |
+
python -m monai.bundle run evaluating --meta_file configs/metadata.json --config_file configs/inference.json --logging_file configs/logging.conf
|
124 |
+
```
|
125 |
+
|
126 |
+
# Disclaimer
|
127 |
+
|
128 |
+
This is an example, not to be used for diagnostic purposes.
|
129 |
+
|
130 |
+
# References
|
131 |
+
|
132 |
+
[1] Simon Graham, Quoc Dang Vu, Shan E Ahmed Raza, Ayesha Azam, Yee Wah Tsang, Jin Tae Kwak, Nasir Rajpoot, Hover-Net: Simultaneous segmentation and classification of nuclei in multi-tissue histology images, Medical Image Analysis, 2019 https://doi.org/10.1016/j.media.2019.101563
|
133 |
+
|
134 |
+
# License
|
135 |
+
Copyright (c) MONAI Consortium
|
136 |
+
|
137 |
+
Licensed under the Apache License, Version 2.0 (the "License");
|
138 |
+
you may not use this file except in compliance with the License.
|
139 |
+
You may obtain a copy of the License at
|
140 |
+
|
141 |
+
http://www.apache.org/licenses/LICENSE-2.0
|
142 |
+
|
143 |
+
Unless required by applicable law or agreed to in writing, software
|
144 |
+
distributed under the License is distributed on an "AS IS" BASIS,
|
145 |
+
WITHOUT WARRANTIES OR CONDITIONS OF ANY KIND, either express or implied.
|
146 |
+
See the License for the specific language governing permissions and
|
147 |
+
limitations under the License.
|
docs/data_license.txt
ADDED
@@ -0,0 +1,6 @@
|
|
|
|
|
|
|
|
|
|
|
|
|
|
|
1 |
+
Third Party Licenses
|
2 |
+
-----------------------------------------------------------------------
|
3 |
+
|
4 |
+
/*********************************************************************/
|
5 |
+
i. CoNSeP dataset
|
6 |
+
https://warwick.ac.uk/fac/cross_fac/tia/data/hovernet/
|
models/model.pt
ADDED
@@ -0,0 +1,3 @@
|
|
|
|
|
|
|
|
|
1 |
+
version https://git-lfs.github.com/spec/v1
|
2 |
+
oid sha256:3aa9651e11eca4c17d89fe59b46b8a51c7899decb03df538f5092ee9e55967ef
|
3 |
+
size 151214500
|
models/stage0/model.pt
ADDED
@@ -0,0 +1,3 @@
|
|
|
|
|
|
|
|
|
1 |
+
version https://git-lfs.github.com/spec/v1
|
2 |
+
oid sha256:6a394593bc2ed0ef53188ffc96174ae492b59448a37b4b4a161214a30b173785
|
3 |
+
size 151220093
|
scripts/prepare_patches.py
ADDED
@@ -0,0 +1,232 @@
|
|
|
|
|
|
|
|
|
|
|
|
|
|
|
|
|
|
|
|
|
|
|
|
|
|
|
|
|
|
|
|
|
|
|
|
|
|
|
|
|
|
|
|
|
|
|
|
|
|
|
|
|
|
|
|
|
|
|
|
|
|
|
|
|
|
|
|
|
|
|
|
|
|
|
|
|
|
|
|
|
|
|
|
|
|
|
|
|
|
|
|
|
|
|
|
|
|
|
|
|
|
|
|
|
|
|
|
|
|
|
|
|
|
|
|
|
|
|
|
|
|
|
|
|
|
|
|
|
|
|
|
|
|
|
|
|
|
|
|
|
|
|
|
|
|
|
|
|
|
|
|
|
|
|
|
|
|
|
|
|
|
|
|
|
|
|
|
|
|
|
|
|
|
|
|
|
|
|
|
|
|
|
|
|
|
|
|
|
|
|
|
|
|
|
|
|
|
|
|
|
|
|
|
|
|
|
|
|
|
|
|
|
|
|
|
|
|
|
|
|
|
|
|
|
|
|
|
|
|
|
|
|
|
|
|
|
|
|
|
|
|
|
|
|
|
|
|
|
|
|
|
|
|
|
|
|
|
|
|
|
|
|
|
|
|
|
|
|
|
|
|
|
|
|
|
|
|
|
|
|
|
|
|
|
|
|
|
|
|
|
|
|
|
|
|
|
|
|
|
|
|
|
|
|
|
|
|
|
|
|
|
|
|
|
|
|
|
|
|
|
|
|
|
|
|
|
|
|
|
|
|
|
|
|
|
|
|
|
|
|
|
|
|
|
|
|
|
|
|
|
|
|
|
|
|
|
|
|
|
|
|
|
|
|
|
|
|
|
|
|
|
|
|
|
|
|
|
|
|
|
|
|
|
|
|
|
|
|
|
|
|
|
|
|
|
|
|
|
|
|
|
|
|
|
|
|
|
|
|
|
|
|
|
|
|
|
|
|
|
|
|
|
|
|
|
|
|
|
|
|
|
|
|
|
|
|
|
|
|
|
|
|
|
|
|
|
|
|
|
|
|
|
|
|
|
|
|
|
|
|
|
|
|
|
|
|
1 |
+
import glob
|
2 |
+
import math
|
3 |
+
import os
|
4 |
+
import pathlib
|
5 |
+
import shutil
|
6 |
+
from argparse import ArgumentParser
|
7 |
+
|
8 |
+
import numpy as np
|
9 |
+
import scipy.io as sio
|
10 |
+
import tqdm
|
11 |
+
from PIL import Image
|
12 |
+
|
13 |
+
|
14 |
+
def load_img(path):
|
15 |
+
return np.array(Image.open(path).convert("RGB"))
|
16 |
+
|
17 |
+
|
18 |
+
def load_ann(path):
|
19 |
+
"""
|
20 |
+
This function is specific to CoNSeP dataset.
|
21 |
+
If using other datasets, the code below may need to be modified.
|
22 |
+
"""
|
23 |
+
# assumes that ann is HxW
|
24 |
+
ann_inst = sio.loadmat(path)["inst_map"]
|
25 |
+
ann_type = sio.loadmat(path)["type_map"]
|
26 |
+
|
27 |
+
# merge classes for CoNSeP (utilise 3 nuclei classes and background keep the same with paper)
|
28 |
+
ann_type[(ann_type == 3) | (ann_type == 4)] = 3
|
29 |
+
ann_type[(ann_type == 5) | (ann_type == 6) | (ann_type == 7)] = 4
|
30 |
+
|
31 |
+
ann = np.dstack([ann_inst, ann_type])
|
32 |
+
ann = ann.astype("int32")
|
33 |
+
|
34 |
+
return ann
|
35 |
+
|
36 |
+
|
37 |
+
class PatchExtractor:
|
38 |
+
"""Extractor to generate patches with or without padding.
|
39 |
+
Turn on debug mode to see how it is done.
|
40 |
+
|
41 |
+
Args:
|
42 |
+
x : input image, should be of shape HWC
|
43 |
+
patch_size : a tuple of (h, w)
|
44 |
+
step_size : a tuple of (h, w)
|
45 |
+
Return:
|
46 |
+
a list of sub patches, each patch has dtype same as x
|
47 |
+
|
48 |
+
Examples:
|
49 |
+
>>> xtractor = PatchExtractor((450, 450), (120, 120))
|
50 |
+
>>> img = np.full([1200, 1200, 3], 255, np.uint8)
|
51 |
+
>>> patches = xtractor.extract(img, 'mirror')
|
52 |
+
|
53 |
+
"""
|
54 |
+
|
55 |
+
def __init__(self, patch_size, step_size):
|
56 |
+
self.patch_type = "mirror"
|
57 |
+
self.patch_size = patch_size
|
58 |
+
self.step_size = step_size
|
59 |
+
|
60 |
+
def __get_patch(self, x, ptx):
|
61 |
+
pty = (ptx[0] + self.patch_size[0], ptx[1] + self.patch_size[1])
|
62 |
+
win = x[ptx[0] : pty[0], ptx[1] : pty[1]]
|
63 |
+
assert (
|
64 |
+
win.shape[0] == self.patch_size[0] and win.shape[1] == self.patch_size[1]
|
65 |
+
), "[BUG] Incorrect Patch Size {0}".format(win.shape)
|
66 |
+
return win
|
67 |
+
|
68 |
+
def __extract_valid(self, x):
|
69 |
+
"""Extracted patches without padding, only work in case patch_size > step_size.
|
70 |
+
|
71 |
+
Note: to deal with the remaining portions which are at the boundary a.k.a
|
72 |
+
those which do not fit when slide left->right, top->bottom), we flip
|
73 |
+
the sliding direction then extract 1 patch starting from right / bottom edge.
|
74 |
+
There will be 1 additional patch extracted at the bottom-right corner.
|
75 |
+
|
76 |
+
Args:
|
77 |
+
x : input image, should be of shape HWC
|
78 |
+
patch_size : a tuple of (h, w)
|
79 |
+
step_size : a tuple of (h, w)
|
80 |
+
Return:
|
81 |
+
a list of sub patches, each patch is same dtype as x
|
82 |
+
|
83 |
+
"""
|
84 |
+
im_h = x.shape[0]
|
85 |
+
im_w = x.shape[1]
|
86 |
+
|
87 |
+
def extract_infos(length, patch_size, step_size):
|
88 |
+
flag = (length - patch_size) % step_size != 0
|
89 |
+
last_step = math.floor((length - patch_size) / step_size)
|
90 |
+
last_step = (last_step + 1) * step_size
|
91 |
+
return flag, last_step
|
92 |
+
|
93 |
+
h_flag, h_last = extract_infos(im_h, self.patch_size[0], self.step_size[0])
|
94 |
+
w_flag, w_last = extract_infos(im_w, self.patch_size[1], self.step_size[1])
|
95 |
+
|
96 |
+
sub_patches = []
|
97 |
+
# Deal with valid block
|
98 |
+
for row in range(0, h_last, self.step_size[0]):
|
99 |
+
for col in range(0, w_last, self.step_size[1]):
|
100 |
+
win = self.__get_patch(x, (row, col))
|
101 |
+
sub_patches.append(win)
|
102 |
+
# Deal with edge case
|
103 |
+
if h_flag:
|
104 |
+
row = im_h - self.patch_size[0]
|
105 |
+
for col in range(0, w_last, self.step_size[1]):
|
106 |
+
win = self.__get_patch(x, (row, col))
|
107 |
+
sub_patches.append(win)
|
108 |
+
if w_flag:
|
109 |
+
col = im_w - self.patch_size[1]
|
110 |
+
for row in range(0, h_last, self.step_size[0]):
|
111 |
+
win = self.__get_patch(x, (row, col))
|
112 |
+
sub_patches.append(win)
|
113 |
+
if h_flag and w_flag:
|
114 |
+
ptx = (im_h - self.patch_size[0], im_w - self.patch_size[1])
|
115 |
+
win = self.__get_patch(x, ptx)
|
116 |
+
sub_patches.append(win)
|
117 |
+
return sub_patches
|
118 |
+
|
119 |
+
def __extract_mirror(self, x):
|
120 |
+
"""Extracted patches with mirror padding the boundary such that the
|
121 |
+
central region of each patch is always within the orginal (non-padded)
|
122 |
+
image while all patches' central region cover the whole orginal image.
|
123 |
+
|
124 |
+
Args:
|
125 |
+
x : input image, should be of shape HWC
|
126 |
+
patch_size : a tuple of (h, w)
|
127 |
+
step_size : a tuple of (h, w)
|
128 |
+
Return:
|
129 |
+
a list of sub patches, each patch is same dtype as x
|
130 |
+
|
131 |
+
"""
|
132 |
+
diff_h = self.patch_size[0] - self.step_size[0]
|
133 |
+
padt = diff_h // 2
|
134 |
+
padb = diff_h - padt
|
135 |
+
|
136 |
+
diff_w = self.patch_size[1] - self.step_size[1]
|
137 |
+
padl = diff_w // 2
|
138 |
+
padr = diff_w - padl
|
139 |
+
|
140 |
+
pad_type = "reflect"
|
141 |
+
x = np.lib.pad(x, ((padt, padb), (padl, padr), (0, 0)), pad_type)
|
142 |
+
sub_patches = self.__extract_valid(x)
|
143 |
+
return sub_patches
|
144 |
+
|
145 |
+
def extract(self, x, patch_type):
|
146 |
+
patch_type = patch_type.lower()
|
147 |
+
self.patch_type = patch_type
|
148 |
+
if patch_type == "valid":
|
149 |
+
return self.__extract_valid(x)
|
150 |
+
elif patch_type == "mirror":
|
151 |
+
return self.__extract_mirror(x)
|
152 |
+
else:
|
153 |
+
raise ValueError(f"Unknown Patch Type {patch_type}")
|
154 |
+
|
155 |
+
|
156 |
+
def main(cfg):
|
157 |
+
xtractor = PatchExtractor(cfg["patch_size"], cfg["step_size"])
|
158 |
+
for phase in cfg["phase"]:
|
159 |
+
img_dir = os.path.join(cfg["root"], f"{phase}/Images")
|
160 |
+
ann_dir = os.path.join(cfg["root"], f"{phase}/Labels")
|
161 |
+
|
162 |
+
file_list = glob.glob(os.path.join(ann_dir, f"*{cfg['label_suffix']}"))
|
163 |
+
file_list.sort() # ensure same ordering across platform
|
164 |
+
|
165 |
+
out_dir = f"{cfg['root']}/Prepared/{phase}"
|
166 |
+
if os.path.isdir(out_dir):
|
167 |
+
shutil.rmtree(out_dir)
|
168 |
+
os.makedirs(out_dir)
|
169 |
+
|
170 |
+
pbar_format = "Process File: |{bar}| {n_fmt}/{total_fmt}[{elapsed}<{remaining},{rate_fmt}]"
|
171 |
+
pbarx = tqdm.tqdm(total=len(file_list), bar_format=pbar_format, ascii=True, position=0)
|
172 |
+
|
173 |
+
for file_path in file_list:
|
174 |
+
base_name = pathlib.Path(file_path).stem
|
175 |
+
|
176 |
+
img = load_img(f"{img_dir}/{base_name}.{cfg['image_suffix']}")
|
177 |
+
ann = load_ann(f"{ann_dir}/{base_name}.{cfg['label_suffix']}")
|
178 |
+
|
179 |
+
# *
|
180 |
+
img = np.concatenate([img, ann], axis=-1)
|
181 |
+
sub_patches = xtractor.extract(img, cfg["extract_type"])
|
182 |
+
|
183 |
+
pbar_format = "Extracting : |{bar}| {n_fmt}/{total_fmt}[{elapsed}<{remaining},{rate_fmt}]"
|
184 |
+
pbar = tqdm.tqdm(total=len(sub_patches), leave=False, bar_format=pbar_format, ascii=True, position=1)
|
185 |
+
|
186 |
+
for idx, patch in enumerate(sub_patches):
|
187 |
+
image_patch = patch[..., :3]
|
188 |
+
inst_map_patch = patch[..., 3:4]
|
189 |
+
type_map_patch = patch[..., 4:5]
|
190 |
+
np.save("{0}/{1}_{2:03d}_image.npy".format(out_dir, base_name, idx), image_patch)
|
191 |
+
np.save("{0}/{1}_{2:03d}_inst_map.npy".format(out_dir, base_name, idx), inst_map_patch)
|
192 |
+
np.save("{0}/{1}_{2:03d}_type_map.npy".format(out_dir, base_name, idx), type_map_patch)
|
193 |
+
pbar.update()
|
194 |
+
pbar.close()
|
195 |
+
# *
|
196 |
+
|
197 |
+
pbarx.update()
|
198 |
+
pbarx.close()
|
199 |
+
|
200 |
+
|
201 |
+
def parse_arguments():
|
202 |
+
parser = ArgumentParser(description="Extract patches from the original images")
|
203 |
+
|
204 |
+
parser.add_argument(
|
205 |
+
"--root",
|
206 |
+
type=str,
|
207 |
+
default="/workspace/Data/Pathology/CoNSeP",
|
208 |
+
help="root path to image folder containing training/test",
|
209 |
+
)
|
210 |
+
parser.add_argument(
|
211 |
+
"--phase",
|
212 |
+
nargs="+",
|
213 |
+
type=str,
|
214 |
+
default=["Train", "Test"],
|
215 |
+
dest="phase",
|
216 |
+
help="Phases of data need to be extracted",
|
217 |
+
)
|
218 |
+
parser.add_argument("--type", type=str, default="mirror", dest="extract_type", help="Choose 'mirror' or 'valid'")
|
219 |
+
parser.add_argument("--is", type=str, default="png", dest="image_suffix", help="image file name suffix")
|
220 |
+
parser.add_argument("--ls", type=str, default="mat", dest="label_suffix", help="label file name suffix")
|
221 |
+
parser.add_argument("--ps", nargs="+", type=int, default=[540, 540], dest="patch_size", help="patch size")
|
222 |
+
parser.add_argument("--ss", nargs="+", type=int, default=[164, 164], dest="step_size", help="patch size")
|
223 |
+
args = parser.parse_args()
|
224 |
+
config_dict = vars(args)
|
225 |
+
|
226 |
+
return config_dict
|
227 |
+
|
228 |
+
|
229 |
+
if __name__ == "__main__":
|
230 |
+
cfg = parse_arguments()
|
231 |
+
|
232 |
+
main(cfg)
|