Spaces:
Runtime error
Runtime error
import gradio as gr | |
from base64 import b64encode | |
import numpy | |
import torch | |
from diffusers import AutoencoderKL, LMSDiscreteScheduler, UNet2DConditionModel | |
from PIL import Image | |
from torch import autocast | |
from torchvision import transforms as tfms | |
from tqdm.auto import tqdm | |
from transformers import CLIPTextModel, CLIPTokenizer, logging | |
import torchvision.transforms as T | |
torch.manual_seed(1) | |
# Supress some unnecessary warnings when loading the CLIPTextModel | |
logging.set_verbosity_error() | |
torch_device = "cpu" | |
# Load the autoencoder model which will be used to decode the latents into image space. | |
vae = AutoencoderKL.from_pretrained("CompVis/stable-diffusion-v1-4", subfolder="vae") | |
# Load the tokenizer and text encoder to tokenize and encode the text. | |
tokenizer = CLIPTokenizer.from_pretrained("openai/clip-vit-large-patch14") | |
text_encoder = CLIPTextModel.from_pretrained("openai/clip-vit-large-patch14") | |
# The UNet model for generating the latents. | |
unet = UNet2DConditionModel.from_pretrained("CompVis/stable-diffusion-v1-4", subfolder="unet") | |
# The noise scheduler | |
scheduler = LMSDiscreteScheduler(beta_start=0.00085, beta_end=0.012, beta_schedule="scaled_linear", num_train_timesteps=1000) | |
# To the GPU we go! | |
vae = vae.to(torch_device) | |
text_encoder = text_encoder.to(torch_device) | |
unet = unet.to(torch_device) | |
token_emb_layer = text_encoder.text_model.embeddings.token_embedding | |
pos_emb_layer = text_encoder.text_model.embeddings.position_embedding | |
position_ids = text_encoder.text_model.embeddings.position_ids[:, :77] | |
# Prep Scheduler | |
def set_timesteps(scheduler, num_inference_steps): | |
scheduler.set_timesteps(num_inference_steps) | |
scheduler.timesteps = scheduler.timesteps.to(torch.float32) # minor fix to ensure MPS compatibility, fixed in diffusers PR 3925 | |
batch_size = 1 | |
height = 512 # default height of Stable Diffusion | |
width = 512 # default width of Stable Diffusion | |
num_inference_steps = 10 # Number of denoising steps | |
guidance_scale = 7.5 # Scale for classifier-free guidance | |
generator = torch.manual_seed(32) # Seed generator to create the inital latent noise | |
# Prep latents | |
latents = torch.randn( | |
(batch_size, unet.config.in_channels, height // 8, width // 8), | |
generator=generator, | |
) | |
latents = latents.to(torch_device) | |
latents = latents * scheduler.init_noise_sigma # Scaling (previous versions did latents = latents * self.scheduler.sigmas[0] | |
# Loop | |
def pil_to_latent(input_im): | |
# Single image -> single latent in a batch (so size 1, 4, 64, 64) | |
with torch.no_grad(): | |
latent = vae.encode(tfms.ToTensor()(input_im).unsqueeze(0).to(torch_device)*2-1) # Note scaling | |
return 0.18215 * latent.latent_dist.sample() | |
def latents_to_pil(latents): | |
# bath of latents -> list of images | |
latents = (1 / 0.18215) * latents | |
with torch.no_grad(): | |
image = vae.decode(latents).sample | |
image = (image / 2 + 0.5).clamp(0, 1) | |
image = image.detach().cpu().permute(0, 2, 3, 1).numpy() | |
images = (image * 255).round().astype("uint8") | |
pil_images = [Image.fromarray(image) for image in images] | |
return pil_images | |
# | |
# Our text prompt | |
prompt = 'A picture of a puppy' | |
"""We begin with tokenization:""" | |
# Turn the text into a sequnce of tokens: | |
text_input = tokenizer(prompt, padding="max_length", max_length=tokenizer.model_max_length, truncation=True, return_tensors="pt") | |
text_input['input_ids'][0] # View the tokens | |
# See the individual tokens | |
for t in text_input['input_ids'][0][:8]: # We'll just look at the first 7 to save you from a wall of '<|endoftext|>' | |
print(t, tokenizer.decoder.get(int(t))) | |
# TODO call out that 6829 is puppy | |
"""We can jump straight to the final (output) embeddings like so:""" | |
# Grab the output embeddings | |
output_embeddings = text_encoder(text_input.input_ids.to(torch_device))[0] | |
print('Shape:', output_embeddings.shape) | |
output_embeddings | |
"""We pass our tokens through the text_encoder and we magically get some numbers we can feed to the model. | |
How are these generated? The tokens are transformed into a set of input embeddings, which are then fed through the transformer model to get the final output embeddings. | |
To get these input embeddings, there are actually two steps - as revealed by inspecting `text_encoder.text_model.embeddings`: | |
""" | |
text_encoder.text_model.embeddings | |
"""### Token embeddings | |
The token is fed to the `token_embedding` to transform it into a vector. The function name `get_input_embeddings` here is misleading since these token embeddings need to be combined with the position embeddings before they are actually used as inputs to the model! Anyway, let's look at just the token embedding part first | |
We can look at the embedding layer: | |
""" | |
# Access the embedding layer | |
token_emb_layer = text_encoder.text_model.embeddings.token_embedding | |
token_emb_layer # Vocab size 49408, emb_dim 768 | |
"""And embed a token like so:""" | |
# Embed a token - in this case the one for 'puppy' | |
embedding = token_emb_layer(torch.tensor(6829, device=torch_device)) | |
embedding.shape # 768-dim representation | |
"""This single token has been mapped to a 768-dimensional vector - the token embedding. | |
We can do the same with all of the tokens in the prompt to get all the token embeddings: | |
""" | |
token_embeddings = token_emb_layer(text_input.input_ids.to(torch_device)) | |
print(token_embeddings.shape) # batch size 1, 77 tokens, 768 values for each | |
token_embeddings | |
"""### Positional Embeddings | |
Positional embeddings tell the model where in a sequence a token is. Much like the token embedding, this is a set of (optionally learnable) parameters. But now instead of dealing with ~50k tokens we just need one for each position (77 total): | |
""" | |
pos_emb_layer = text_encoder.text_model.embeddings.position_embedding | |
pos_emb_layer | |
"""We can get the positional embedding for each position:""" | |
position_ids = text_encoder.text_model.embeddings.position_ids[:, :77] | |
position_embeddings = pos_emb_layer(position_ids) | |
print(position_embeddings.shape) | |
position_embeddings | |
"""### Combining token and position embeddings | |
Time to combine the two. How do we do this? Just add them! Other approaches are possible but for this model this is how it is done. | |
Combining them in this way gives us the final input embeddings ready to feed through the transformer model: | |
""" | |
# And combining them we get the final input embeddings | |
input_embeddings = token_embeddings + position_embeddings | |
print(input_embeddings.shape) | |
input_embeddings | |
"""We can check that these are the same as the result we'd get from `text_encoder.text_model.embeddings`:""" | |
# The following combines all the above steps (but doesn't let us fiddle with them!) | |
text_encoder.text_model.embeddings(text_input.input_ids.to(torch_device)) | |
"""### Feeding these through the transformer model | |
 | |
We want to mess with these input embeddings (specifically the token embeddings) before we send them through the rest of the model, but first we should check that we know how to do that. I read the code of the text_encoders `forward` method, and based on that the code for the `forward` method of the text_model that the text_encoder wraps. To inspect it yourself, type `??text_encoder.text_model.forward` and you'll get the function info and source code - a useful debugging trick! | |
Anyway, based on that we can copy in the bits we need to get the so-called 'last hidden state' and thus generate our final embeddings: | |
""" | |
def get_output_embeds(input_embeddings): | |
# CLIP's text model uses causal mask, so we prepare it here: | |
bsz, seq_len = input_embeddings.shape[:2] | |
causal_attention_mask = text_encoder.text_model._build_causal_attention_mask(bsz, seq_len, dtype=input_embeddings.dtype) | |
# Getting the output embeddings involves calling the model with passing output_hidden_states=True | |
# so that it doesn't just return the pooled final predictions: | |
encoder_outputs = text_encoder.text_model.encoder( | |
inputs_embeds=input_embeddings, | |
attention_mask=None, # We aren't using an attention mask so that can be None | |
causal_attention_mask=causal_attention_mask.to(torch_device), | |
output_attentions=None, | |
output_hidden_states=True, # We want the output embs not the final output | |
return_dict=None, | |
) | |
# We're interested in the output hidden state only | |
output = encoder_outputs[0] | |
# There is a final layer norm we need to pass these through | |
output = text_encoder.text_model.final_layer_norm(output) | |
# And now they're ready! | |
return output | |
out_embs_test = get_output_embeds(input_embeddings) # Feed through the model with our new function | |
print(out_embs_test.shape) # Check the output shape | |
out_embs_test # Inspect the output | |
"""Note that these match the `output_embeddings` we saw near the start - we've figured out how to split up that one step ("get the text embeddings") into multiple sub-steps ready for us to modify. | |
Now that we have this process in place, we can replace the input embedding of a token with a new one of our choice - which in our final use-case will be something we learn. To demonstrate the concept though, let's replace the input embedding for 'puppy' in the prompt we've been playing with with the embedding for token 2368, get a new set of output embeddings based on this, and use these to generate an image to see what we get: | |
""" | |
prompt = 'A picture of a puppy' | |
# Tokenize | |
text_input = tokenizer(prompt, padding="max_length", max_length=tokenizer.model_max_length, truncation=True, return_tensors="pt") | |
input_ids = text_input.input_ids.to(torch_device) | |
# Get token embeddings | |
token_embeddings = token_emb_layer(input_ids) | |
# The new embedding. In this case just the input embedding of token 2368... | |
replacement_token_embedding = text_encoder.get_input_embeddings()(torch.tensor(2368, device=torch_device)) | |
# Insert this into the token embeddings ( | |
token_embeddings[0, torch.where(input_ids[0]==6829)] = replacement_token_embedding.to(torch_device) | |
# Combine with pos embs | |
input_embeddings = token_embeddings + position_embeddings | |
# Feed through to get final output embs | |
modified_output_embeddings = get_output_embeds(input_embeddings) | |
print(modified_output_embeddings.shape) | |
modified_output_embeddings | |
"""The first few are the same, the last aren't. Everything at and after the position of the token we're replacing will be affected. | |
If all went well, we should see something other than a puppy when we use these to generate an image. And sure enough, we do! | |
""" | |
#Generating an image with these modified embeddings | |
def generate_with_embs(text_embeddings): | |
height = 512 # default height of Stable Diffusion | |
width = 512 # default width of Stable Diffusion | |
num_inference_steps = 30 # Number of denoising steps | |
guidance_scale = 7.5 # Scale for classifier-free guidance | |
generator = torch.manual_seed(32) # Seed generator to create the inital latent noise | |
batch_size = 1 | |
max_length = text_input.input_ids.shape[-1] | |
uncond_input = tokenizer( | |
[""] * batch_size, padding="max_length", max_length=max_length, return_tensors="pt" | |
) | |
with torch.no_grad(): | |
uncond_embeddings = text_encoder(uncond_input.input_ids.to(torch_device))[0] | |
text_embeddings = torch.cat([uncond_embeddings, text_embeddings]) | |
# Prep Scheduler | |
set_timesteps(scheduler, num_inference_steps) | |
# Prep latents | |
latents = torch.randn( | |
(batch_size, unet.in_channels, height // 8, width // 8), | |
generator=generator, | |
) | |
latents = latents.to(torch_device) | |
latents = latents * scheduler.init_noise_sigma | |
# Loop | |
for i, t in tqdm(enumerate(scheduler.timesteps), total=len(scheduler.timesteps)): | |
# expand the latents if we are doing classifier-free guidance to avoid doing two forward passes. | |
latent_model_input = torch.cat([latents] * 2) | |
sigma = scheduler.sigmas[i] | |
latent_model_input = scheduler.scale_model_input(latent_model_input, t) | |
# predict the noise residual | |
with torch.no_grad(): | |
noise_pred = unet(latent_model_input, t, encoder_hidden_states=text_embeddings)["sample"] | |
# perform guidance | |
noise_pred_uncond, noise_pred_text = noise_pred.chunk(2) | |
noise_pred = noise_pred_uncond + guidance_scale * (noise_pred_text - noise_pred_uncond) | |
# compute the previous noisy sample x_t -> x_t-1 | |
latents = scheduler.step(noise_pred, t, latents).prev_sample | |
return latents_to_pil(latents)[0] | |
#Generating an image with these modified embeddings | |
def generate_with_embs_seed(text_embeddings, seed, max_length): | |
""" | |
Args: | |
text_embeddings: | |
seed: | |
max_length: | |
Returns: | |
""" | |
height = 512 # default height of Stable Diffusion | |
width = 512 # default width of Stable Diffusion | |
num_inference_steps = 30 # Number of denoising steps | |
guidance_scale = 7.5 # Scale for classifier-free guidance | |
generator = torch.manual_seed(32) # Seed generator to create the inital latent noise | |
batch_size = 1 | |
# max_length = text_input.input_ids.shape[-1] | |
uncond_input = tokenizer( | |
[""] * batch_size, padding="max_length", max_length=max_length, return_tensors="pt" | |
) | |
with torch.no_grad(): | |
uncond_embeddings = text_encoder(uncond_input.input_ids.to(torch_device))[0] | |
text_embeddings = torch.cat([uncond_embeddings, text_embeddings]) | |
# Prep Scheduler | |
set_timesteps(scheduler, num_inference_steps) | |
# Prep latents | |
latents = torch.randn( | |
(batch_size, unet.in_channels, height // 8, width // 8), | |
generator=generator, | |
) | |
latents = latents.to(torch_device) | |
latents = latents * scheduler.init_noise_sigma | |
# Loop | |
for i, t in tqdm(enumerate(scheduler.timesteps), total=len(scheduler.timesteps)): | |
# expand the latents if we are doing classifier-free guidance to avoid doing two forward passes. | |
latent_model_input = torch.cat([latents] * 2) | |
sigma = scheduler.sigmas[i] | |
latent_model_input = scheduler.scale_model_input(latent_model_input, t) | |
# predict the noise residual | |
with torch.no_grad(): | |
noise_pred = unet(latent_model_input, t, encoder_hidden_states=text_embeddings)["sample"] | |
# perform guidance | |
noise_pred_uncond, noise_pred_text = noise_pred.chunk(2) | |
noise_pred = noise_pred_uncond + guidance_scale * (noise_pred_text - noise_pred_uncond) | |
# compute the previous noisy sample x_t -> x_t-1 | |
latents = scheduler.step(noise_pred, t, latents).prev_sample | |
return latents_to_pil(latents)[0] | |
generate_with_embs(modified_output_embeddings) | |
"""Suprise! Now you know what token 2368 means ;) | |
**What can we do with this?** Why did we go to all of this trouble? Well, we'll see a more compelling use-case shortly but the tl;dr is that once we can access and modify the token embeddings we can do tricks like replacing them with something else. In the example we just did, that was just another token embedding from the model's vocabulary, equivalent to just editing the prompt. But we can also mix tokens - for example, here's a half-puppy-half-skunk: | |
""" | |
# In case you're wondering how to get the token for a word, or the embedding for a token: | |
prompt = 'skunk' | |
print('tokenizer(prompt):', tokenizer(prompt)) | |
print('token_emb_layer([token_id]) shape:', token_emb_layer(torch.tensor([8797], device=torch_device)).shape) | |
prompt = 'A picture of a puppy' | |
# Tokenize | |
text_input = tokenizer(prompt, padding="max_length", max_length=tokenizer.model_max_length, truncation=True, return_tensors="pt") | |
input_ids = text_input.input_ids.to(torch_device) | |
# Get token embeddings | |
token_embeddings = token_emb_layer(input_ids) | |
# The new embedding. Which is now a mixture of the token embeddings for 'puppy' and 'skunk' | |
puppy_token_embedding = token_emb_layer(torch.tensor(6829, device=torch_device)) | |
skunk_token_embedding = token_emb_layer(torch.tensor(42194, device=torch_device)) | |
replacement_token_embedding = 0.5*puppy_token_embedding + 0.5*skunk_token_embedding | |
# Insert this into the token embeddings ( | |
token_embeddings[0, torch.where(input_ids[0]==6829)] = replacement_token_embedding.to(torch_device) | |
# Combine with pos embs | |
input_embeddings = token_embeddings + position_embeddings | |
# Feed through to get final output embs | |
modified_output_embeddings = get_output_embeds(input_embeddings) | |
# Generate an image with these | |
generate_with_embs(modified_output_embeddings) | |
"""### Textual Inversion | |
OK, so we can slip in a modified token embedding, and use this to generate an image. We used the token embedding for 'cat' in the above example, but what if instead could 'learn' a new token embedding for a specific concept? This is the idea behind 'Textual Inversion', in which a few example images are used to create a new token embedding: | |
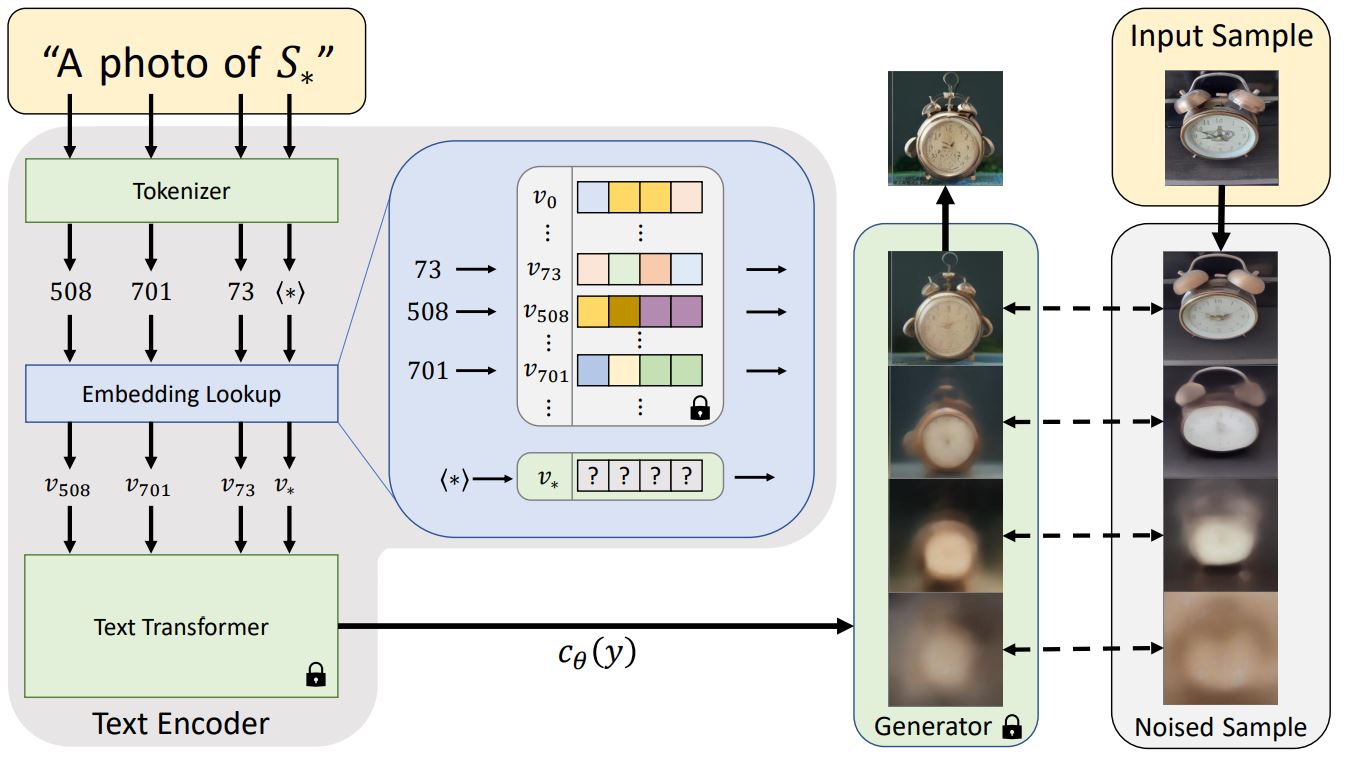 | |
_Diagram from the [textual inversion blog post](https://textual-inversion.github.io/static/images/training/training.JPG) - note it doesn't show the positional embeddings step for simplicity_ | |
We won't cover how this training works, but we can try loading one of these new 'concepts' from the [community-created SD concepts library](https://huggingface.co/sd-concepts-library) and see how it fits in with our example above. I'll use https://huggingface.co/sd-concepts-library/birb-style since it was the first one I made :) Download the learned_embeds.bin file from there and upload the file to wherever this notebook is before running this next cell: | |
""" | |
birb_embed = torch.load('learned_embeds.bin') | |
birb_embed.keys(), birb_embed['<birb-style>'].shape | |
"""We get a dictionary with a key (the special placeholder I used, <birb-style>) and the corresponding token embedding. As in the previous example, let's replace the 'puppy' token embedding with this and see what happens:""" | |
prompt = 'A mouse in the style of puppy' | |
# Tokenize | |
text_input = tokenizer(prompt, padding="max_length", max_length=tokenizer.model_max_length, truncation=True, return_tensors="pt") | |
input_ids = text_input.input_ids.to(torch_device) | |
# Get token embeddings | |
token_embeddings = token_emb_layer(input_ids) | |
# The new embedding - our special birb word | |
replacement_token_embedding = birb_embed['<birb-style>'].to(torch_device) | |
# Insert this into the token embeddings | |
token_embeddings[0, torch.where(input_ids[0]==6829)] = replacement_token_embedding.to(torch_device) | |
# Combine with pos embs | |
input_embeddings = token_embeddings + position_embeddings | |
# Feed through to get final output embs | |
modified_output_embeddings = get_output_embeds(input_embeddings) | |
# And generate an image with this: | |
generate_with_embs(modified_output_embeddings) | |
"""The token for 'puppy' was replaced with one that captures a particular style of painting, but it could just as easily represent a specific object or class of objects. | |
Again, there is [a nice inference notebook ](https://colab.research.google.com/github/huggingface/notebooks/blob/main/diffusers/stable_conceptualizer_inference.ipynb) from hf to make it easy to use the different concepts, that properly handles using the names in prompts ("A \<cat-toy> in the style of \<birb-style>") without worrying about all this manual stuff. The goal of this notebook is to pull back the curtain a bit so you know what is going on behind the scenes :) | |
## Messing with Embeddings | |
Besides just replacing the token embedding of a single word, there are various other tricks we can try. For example, what if we create a 'chimera' by averaging the embeddings of two different prompts? | |
""" | |
# Embed two prompts | |
text_input1 = tokenizer(["A mouse"], padding="max_length", max_length=tokenizer.model_max_length, truncation=True, return_tensors="pt") | |
text_input2 = tokenizer(["A leopard"], padding="max_length", max_length=tokenizer.model_max_length, truncation=True, return_tensors="pt") | |
with torch.no_grad(): | |
text_embeddings1 = text_encoder(text_input1.input_ids.to(torch_device))[0] | |
text_embeddings2 = text_encoder(text_input2.input_ids.to(torch_device))[0] | |
# Mix them together | |
mix_factor = 0.35 | |
mixed_embeddings = (text_embeddings1*mix_factor + \ | |
text_embeddings2*(1-mix_factor)) | |
# Generate! | |
generate_with_embs(mixed_embeddings) | |
"""## The UNET and CFG | |
Now it's time we looked at the actual diffusion model. This is typically a Unet that takes in the noisy latents (x) and predicts the noise. We use a conditional model that also takes in the timestep (t) and our text embedding (aka encoder_hidden_states) as conditioning. Feeding all of these into the model looks like this: | |
`noise_pred = unet(latents, t, encoder_hidden_states=text_embeddings)["sample"]` | |
We can try it out and see what the output looks like: | |
""" | |
# Prep Scheduler | |
set_timesteps(scheduler, num_inference_steps) | |
# What is our timestep | |
t = scheduler.timesteps[0] | |
sigma = scheduler.sigmas[0] | |
# A noisy latent | |
latents = torch.randn( | |
(batch_size, unet.in_channels, height // 8, width // 8), | |
generator=generator, | |
) | |
latents = latents.to(torch_device) | |
latents = latents * scheduler.init_noise_sigma | |
# Text embedding | |
text_input = tokenizer(['A macaw'], padding="max_length", max_length=tokenizer.model_max_length, truncation=True, return_tensors="pt") | |
with torch.no_grad(): | |
text_embeddings = text_encoder(text_input.input_ids.to(torch_device))[0] | |
# Run this through the unet to predict the noise residual | |
with torch.no_grad(): | |
noise_pred = unet(latents, t, encoder_hidden_states=text_embeddings)["sample"] | |
latents.shape, noise_pred.shape # We get preds in the same shape as the input | |
"""Given a set of noisy latents, the model predicts the noise component. We can remove this noise from the noisy latents to see what the output image looks like (`latents_x0 = latents - sigma * noise_pred`). And we can add most of the noise back to this predicted output to get the (slightly less noisy hopefully) input for the next diffusion step. To visualize this let's generate another image, saving both the predicted output (x0) and the next step (xt-1) after every step:""" | |
prompt = 'Oil painting of an otter in a top hat' | |
height = 512 | |
width = 512 | |
num_inference_steps = 50 | |
guidance_scale = 8 | |
generator = torch.manual_seed(32) | |
batch_size = 1 | |
# Make a folder to store results | |
#!rm -rf steps/ | |
#!mkdir -p steps/ | |
# Prep text | |
text_input = tokenizer([prompt], padding="max_length", max_length=tokenizer.model_max_length, truncation=True, return_tensors="pt") | |
with torch.no_grad(): | |
text_embeddings = text_encoder(text_input.input_ids.to(torch_device))[0] | |
max_length = text_input.input_ids.shape[-1] | |
uncond_input = tokenizer( | |
[""] * batch_size, padding="max_length", max_length=max_length, return_tensors="pt" | |
) | |
with torch.no_grad(): | |
uncond_embeddings = text_encoder(uncond_input.input_ids.to(torch_device))[0] | |
text_embeddings = torch.cat([uncond_embeddings, text_embeddings]) | |
# Prep Scheduler | |
set_timesteps(scheduler, num_inference_steps) | |
# Prep latents | |
latents = torch.randn( | |
(batch_size, unet.in_channels, height // 8, width // 8), | |
generator=generator, | |
) | |
latents = latents.to(torch_device) | |
latents = latents * scheduler.init_noise_sigma | |
# Loop | |
for i, t in tqdm(enumerate(scheduler.timesteps), total=len(scheduler.timesteps)): | |
# expand the latents if we are doing classifier-free guidance to avoid doing two forward passes. | |
latent_model_input = torch.cat([latents] * 2) | |
sigma = scheduler.sigmas[i] | |
latent_model_input = scheduler.scale_model_input(latent_model_input, t) | |
# predict the noise residual | |
with torch.no_grad(): | |
noise_pred = unet(latent_model_input, t, encoder_hidden_states=text_embeddings)["sample"] | |
# perform guidance | |
noise_pred_uncond, noise_pred_text = noise_pred.chunk(2) | |
noise_pred = noise_pred_uncond + guidance_scale * (noise_pred_text - noise_pred_uncond) | |
# Get the predicted x0: | |
# latents_x0 = latents - sigma * noise_pred # Calculating ourselves | |
scheduler_step = scheduler.step(noise_pred, t, latents) | |
latents_x0 = scheduler_step.pred_original_sample # Using the scheduler (Diffusers 0.4 and above) | |
# compute the previous noisy sample x_t -> x_t-1 | |
latents = scheduler_step.prev_sample | |
# To PIL Images | |
im_t0 = latents_to_pil(latents_x0)[0] | |
im_next = latents_to_pil(latents)[0] | |
# Combine the two images and save for later viewing | |
im = Image.new('RGB', (1024, 512)) | |
im.paste(im_next, (0, 0)) | |
im.paste(im_t0, (512, 0)) | |
im.save(f'steps/{i:04}.jpeg') | |
# Make and show the progress video (change width to 1024 for full res) | |
#!ffmpeg -v 1 -y -f image2 -framerate 12 -i steps/%04d.jpeg -c:v libx264 -preset slow -qp 18 -pix_fmt yuv420p out.mp4 | |
#mp4 = open('out.mp4','rb').read() | |
#data_url = "data:video/mp4;base64," + b64encode(mp4).decode() | |
#HTML(""" | |
#<video width=600 controls> | |
# <source src="%s" type="video/mp4"> | |
#</video> | |
#""" % data_url) | |
#"""The version on the right shows the predicted 'final output' (x0) at each step, and this is what is usually used for progress videos etc. The version on the left is the 'next step'. I found it interesteing to compare the two - watching the progress videos only you'd think drastic changes are happening expecially at early stages, but since the changes made per-step are relatively small the actual process is much more gradual. | |
# Guidance | |
def blue_loss(images): | |
# How far are the blue channel values to 0.9: | |
error = torch.abs(images[:,2] - 0.9).mean() # [:,2] -> all images in batch, only the blue channel | |
return error | |
def orange_loss(images): | |
""" | |
Calculate the mean absolute error between the RGB values of the images and the target orange color. | |
Parameters: | |
- images (torch.Tensor): A batch of images with shape (batch_size, channels, height, width). | |
The images are assumed to be in RGB format. | |
Returns: | |
- torch.Tensor: The mean absolute error for the orange color. | |
""" | |
# Define the target RGB values for the color orange | |
target_orange = torch.tensor([255/255, 200/255, 0/255]).view(1, 3, 1, 1).to(images.device) # (R, G, B) | |
# Normalize images to [0, 1] range if not already normalized | |
images = images / 255.0 if images.max() > 1.0 else images | |
# Calculate the mean absolute error between the RGB values and the target orange values | |
error = torch.abs(images - target_orange).mean() | |
return error | |
"""During each update step, we find the gradient of the loss with respect to the current noisy latents, and tweak them in the direction that reduces this loss as well as performing the normal update step:""" | |
prompt = 'A campfire (oil on canvas)' #@param | |
height = 512 # default height of Stable Diffusion | |
width = 512 # default width of Stable Diffusion | |
num_inference_steps = 50 #@param # Number of denoising steps | |
guidance_scale = 8 #@param # Scale for classifier-free guidance | |
generator = torch.manual_seed(32) # Seed generator to create the inital latent noise | |
batch_size = 1 | |
orange_loss_scale = 200 #@param | |
# Prep text | |
text_input = tokenizer([prompt], padding="max_length", max_length=tokenizer.model_max_length, truncation=True, return_tensors="pt") | |
with torch.no_grad(): | |
text_embeddings = text_encoder(text_input.input_ids.to(torch_device))[0] | |
# And the uncond. input as before: | |
max_length = text_input.input_ids.shape[-1] | |
uncond_input = tokenizer( | |
[""] * batch_size, padding="max_length", max_length=max_length, return_tensors="pt" | |
) | |
with torch.no_grad(): | |
uncond_embeddings = text_encoder(uncond_input.input_ids.to(torch_device))[0] | |
text_embeddings = torch.cat([uncond_embeddings, text_embeddings]) | |
# Prep Scheduler | |
set_timesteps(scheduler, num_inference_steps) | |
# Prep latents | |
latents = torch.randn( | |
(batch_size, unet.in_channels, height // 8, width // 8), | |
generator=generator, | |
) | |
latents = latents.to(torch_device) | |
latents = latents * scheduler.init_noise_sigma | |
# Loop | |
for i, t in tqdm(enumerate(scheduler.timesteps), total=len(scheduler.timesteps)): | |
# expand the latents if we are doing classifier-free guidance to avoid doing two forward passes. | |
latent_model_input = torch.cat([latents] * 2) | |
sigma = scheduler.sigmas[i] | |
latent_model_input = scheduler.scale_model_input(latent_model_input, t) | |
# predict the noise residual | |
with torch.no_grad(): | |
noise_pred = unet(latent_model_input, t, encoder_hidden_states=text_embeddings)["sample"] | |
# perform CFG | |
noise_pred_uncond, noise_pred_text = noise_pred.chunk(2) | |
noise_pred = noise_pred_uncond + guidance_scale * (noise_pred_text - noise_pred_uncond) | |
#### ADDITIONAL GUIDANCE ### | |
if i%5 == 0: | |
# Requires grad on the latents | |
latents = latents.detach().requires_grad_() | |
# Get the predicted x0: | |
latents_x0 = latents - sigma * noise_pred | |
# latents_x0 = scheduler.step(noise_pred, t, latents).pred_original_sample | |
# Decode to image space | |
denoised_images = vae.decode((1 / 0.18215) * latents_x0).sample / 2 + 0.5 # range (0, 1) | |
# Calculate loss | |
loss = blue_loss(denoised_images) * orange_loss_scale | |
# Occasionally print it out | |
if i%10==0: | |
print(i, 'loss:', loss.item()) | |
# Get gradient | |
cond_grad = torch.autograd.grad(loss, latents)[0] | |
# Modify the latents based on this gradient | |
latents = latents.detach() - cond_grad * sigma**2 | |
# Now step with scheduler | |
latents = scheduler.step(noise_pred, t, latents).prev_sample | |
latents_to_pil(latents)[0] | |
prompt = 'A mouse in the style of puppy' | |
# Tokenize | |
text_input = tokenizer(prompt, padding="max_length", max_length=tokenizer.model_max_length, truncation=True, return_tensors="pt") | |
text_input | |
input_ids = text_input.input_ids.to(torch_device) | |
# Get token embeddings | |
token_embeddings = token_emb_layer(input_ids) | |
# The new embedding - our special birb word | |
replacement_token_embedding = birb_embed['<birb-style>'].to(torch_device) | |
# Insert this into the token embeddings | |
token_embeddings[0, torch.where(input_ids[0]==6829)] = replacement_token_embedding.to(torch_device) | |
# Combine with pos embs | |
input_embeddings = token_embeddings + position_embeddings | |
# Feed through to get final output embs | |
modified_output_embeddings = get_output_embeds(input_embeddings) | |
# And generate an image with this: | |
generate_with_embs(modified_output_embeddings) | |
text_input, input_ids,token_embeddings | |
def generate_loss(modified_output_embeddings, seed, max_length): | |
# prompt = 'A campfire (oil on canvas)' #@param | |
height = 512 # default height of Stable Diffusion | |
width = 512 # default width of Stable Diffusion | |
num_inference_steps = 50 #@param # Number of denoising steps | |
guidance_scale = 8 #@param # Scale for classifier-free guidance | |
generator = torch.manual_seed(32) # Seed generator to create the initial latent noise | |
batch_size = 1 | |
blue_loss_scale = 200 #@param | |
# Prep text | |
# text_input = tokenizer([""] * batch_size, padding="max_length", max_length=tokenizer.model_max_length, truncation=True, return_tensors="pt") | |
#input_ids = text_input.input_ids.to(torch_device) | |
# Get token embeddings | |
#token_embeddings = token_emb_layer(input_ids) | |
# The new embedding - our special birb word | |
#replacement_token_embedding = birb_embed['<birb-style>'].to(torch_device) | |
# Insert this into the token embeddings | |
#indices = torch.where(input_ids[0] == 6829)[0] | |
#token_embeddings[0, indices] = replacement_token_embedding.expand_as(token_embeddings[0, indices]) | |
# Combine with pos embs | |
#input_embeddings = token_embeddings + position_embeddings | |
# Pass the modified embeddings to the text encoder | |
#with torch.no_grad(): | |
# text_embeddings = text_encoder(inputs_embeds=input_embeddings)[0] | |
# And the uncond. input as before: | |
# max_length = input_ids.shape[-1] | |
uncond_input = tokenizer( | |
[""] * batch_size, padding="max_length", max_length=max_length, return_tensors="pt" | |
) | |
with torch.no_grad(): | |
uncond_embeddings = text_encoder(uncond_input.input_ids.to(torch_device))[0] | |
# Ensure both embeddings have the same shape | |
if uncond_embeddings.shape != modified_output_embeddings.shape: | |
raise ValueError(f"Shape mismatch: uncond_embeddings {uncond_embeddings.shape} vs modified_output_embeddings {modified_output_embeddings.shape}") | |
text_embeddings = torch.cat([uncond_embeddings, modified_output_embeddings]) | |
# Prep Scheduler | |
set_timesteps(scheduler, num_inference_steps) | |
# Prep latents | |
latents = torch.randn( | |
(batch_size, unet.in_channels, height // 8, width // 8), | |
generator=generator, | |
) | |
latents = latents.to(torch_device) | |
latents = latents * scheduler.init_noise_sigma | |
# Loop | |
for i, t in tqdm(enumerate(scheduler.timesteps), total=len(scheduler.timesteps)): | |
# expand the latents if we are doing classifier-free guidance to avoid doing two forward passes. | |
latent_model_input = torch.cat([latents] * 2) | |
sigma = scheduler.sigmas[i] | |
latent_model_input = scheduler.scale_model_input(latent_model_input, t) | |
# predict the noise residual | |
with torch.no_grad(): | |
noise_pred = unet(latent_model_input, t, encoder_hidden_states=text_embeddings)["sample"] | |
# perform CFG | |
noise_pred_uncond, noise_pred_text = noise_pred.chunk(2) | |
noise_pred = noise_pred_uncond + guidance_scale * (noise_pred_text - noise_pred_uncond) | |
#### ADDITIONAL GUIDANCE ### | |
if i % 5 == 0: | |
# Requires grad on the latents | |
latents = latents.detach().requires_grad_() | |
# Get the predicted x0: | |
latents_x0 = latents - sigma * noise_pred | |
# latents_x0 = scheduler.step(noise_pred, t, latents).pred_original_sample | |
# Decode to image space | |
denoised_images = vae.decode((1 / 0.18215) * latents_x0).sample / 2 + 0.5 # range (0, 1) | |
# Calculate loss | |
loss = orange_loss(denoised_images) * blue_loss_scale | |
# Occasionally print it out | |
if i % 10 == 0: | |
print(i, 'loss:', loss.item()) | |
# Get gradient | |
cond_grad = torch.autograd.grad(loss, latents)[0] | |
# Modify the latents based on this gradient | |
latents = latents.detach() - cond_grad * sigma ** 2 | |
# Now step with scheduler | |
latents = scheduler.step(noise_pred, t, latents).prev_sample | |
# Convert the final latents to an image and display it | |
image = latents_to_pil(latents)[0] | |
image.show() | |
return image | |
def generate_loss_style(prompt, style_embed, style_seed): | |
# Tokenize | |
text_input = tokenizer(prompt, padding="max_length", max_length=tokenizer.model_max_length, truncation=True, return_tensors="pt") | |
input_ids = text_input.input_ids.to(torch_device) | |
# Get token embeddings | |
token_embeddings = token_emb_layer(input_ids) | |
if isinstance(style_embed, dict): | |
style_embed = style_embed['<gartic-phone>'] | |
# The new embedding - our special birb word | |
replacement_token_embedding = style_embed.to(torch_device) | |
# Assuming token_embeddings has shape [batch_size, seq_length, embedding_dim] | |
replacement_token_embedding = replacement_token_embedding[:768] # Adjust the size | |
replacement_token_embedding = replacement_token_embedding.unsqueeze(0) # Make it [1, 768] if necessary | |
indices = torch.where(input_ids[0] == 6829)[0] # Extract indices where the condition is True | |
print(f"indices: {indices}") # Debug print | |
for index in indices: | |
print(f"index: {index}") # Debug print | |
token_embeddings[0, index] = replacement_token_embedding.to(torch_device) # Update each index | |
# Insert this into the token embeddings | |
# token_embeddings[0, torch.where(input_ids[0]==6829)] = replacement_token_embedding.to(torch_device) | |
# Combine with pos embs | |
input_embeddings = token_embeddings + position_embeddings | |
# Feed through to get final output embs | |
modified_output_embeddings = get_output_embeds(input_embeddings) | |
# And generate an image with this: | |
max_length = text_input.input_ids.shape[-1] | |
return generate_loss(modified_output_embeddings, style_seed,max_length) | |
def generate_embed_style(prompt, learned_style, seed): | |
# prompt = 'A campfire (oil on canvas)' #@param | |
height = 512 # default height of Stable Diffusion | |
width = 512 # default width of Stable Diffusion | |
num_inference_steps = 50 #@param # Number of denoising steps | |
guidance_scale = 8 #@param # Scale for classifier-free guidance | |
generator = torch.manual_seed(32) # Seed generator to create the initial latent noise | |
batch_size = 1 | |
blue_loss_scale = 200 #@param | |
if isinstance(learned_style, dict): | |
learned_style = learned_style['<gartic-phone>'] | |
# Prep text | |
text_input = tokenizer([prompt], padding="max_length", max_length=tokenizer.model_max_length, truncation=True, return_tensors="pt") | |
input_ids = text_input.input_ids.to(torch_device) | |
# Get token embeddings | |
token_embeddings = text_encoder.get_input_embeddings()(input_ids) | |
# The new embedding - our special birb word | |
replacement_token_embedding = learned_style.to(torch_device) | |
replacement_token_embedding = replacement_token_embedding[:768] # Adjust the size | |
replacement_token_embedding = replacement_token_embedding.unsqueeze(0) # Make it [1, 768] if necessary | |
# Insert this into the token embeddings | |
indices = torch.where(input_ids[0] == 6829)[0] | |
for index in indices: | |
token_embeddings[0, index] = replacement_token_embedding.to(torch_device) | |
# Combine with pos embs | |
position_ids = torch.arange(token_embeddings.shape[1], dtype=torch.long, device=torch_device) | |
position_ids = position_ids.unsqueeze(0).expand_as(input_ids) | |
position_ids = text_encoder.text_model.embeddings.position_ids[:, :77] | |
position_embeddings = pos_emb_layer(position_ids) | |
#position_embeddings = text_encoder.get_position_embeddings()(position_ids) | |
input_embeddings = token_embeddings + position_embeddings | |
# Feed through to get final output embs | |
modified_output_embeddings = get_output_embeds(input_embeddings) | |
# And generate an image with this: | |
max_length = text_input.input_ids.shape[-1] | |
emb_seed = generate_with_embs_seed(modified_output_embeddings, seed, max_length) | |
#generate_loss_details = generate_loss(modified_output_embeddings, seed, max_length) | |
return emb_seed | |
# And generate an , generateimage with this: | |
def generate_image_from_prompt(text_in, style_in): | |
STYLE_LIST = ['learned_embeds_gartic-phone_style.bin', 'learned_embeds_hawaiian-shirt_style.bin', 'learned_embeds_phone01_style.bin', 'learned_embeds_style-spdmn_style.bin', 'learned_embedssd_yvmqznrm_style.bin'] | |
#learned_embeds = [learned_embeds_gartic-phone.bin,learned_embeds_libraryhawaiian-shirt.bin, learned_embeds_phone0.bin1,learned_embeds_style-spdmn.bin,learned_embedssd_yvmqznrm.bin] | |
STYLE_SEEDS = [128, 64, 128, 64, 128] | |
print(text_in) | |
print(style_in) | |
style_file = style_in + '_style.bin' | |
idx = STYLE_LIST.index(style_file) | |
print(style_file) | |
print(idx) | |
prompt = text_in | |
style_seed = STYLE_SEEDS[idx] | |
style_dict = torch.load(style_file) | |
style_embed = [v for v in style_dict.values()] | |
generated_image = generate_embed_style(prompt,style_embed[0], style_seed) | |
generate_loss_details = (generate_loss_style(prompt, birb_embed, style_seed)) | |
#generate_loss_style(prompt, style_embed, style_seed): | |
#loss_generated_img = (loss_style(prompt, style_embed[0], style_seed)) | |
return [generated_image,generate_loss_details] | |
# Define Interface | |
title = 'Stable Diffusion Art Generator' | |
# Add clear and concise labels and instructions | |
prompt_label = "Enter a prompt (e.g., 'A campfire (oil on canvas)'" | |
styles_label = "Select a Pretrained Style:" | |
instructions = "Explore creative art generation using Stable Diffusion. Enter a prompt and choose a style to get started." | |
demo = gr.Interface(generate_image_from_prompt, | |
inputs=[ | |
gr.Textbox('A campfire (oil on canvas)', label=prompt_label), | |
gr.Dropdown( | |
['learned_embeds_gartic-phone', 'learned_embeds_hawaiian-shirt', 'learned_embeds_phone01', 'learned_embeds_style-spdmn', 'learned_embedssd_yvmqznrm'], | |
value="learned_embeds_gartic-phone", | |
label=styles_label | |
), | |
], | |
outputs=[ | |
gr.Gallery(label="Generated Images", show_label=False, elem_id="gallery", columns=2, rows=2, | |
object_fit="contain"), | |
], | |
title=title, | |
description=instructions | |
) | |
demo.launch(debug=True) | |