Upload folder using huggingface_hub
Browse filesThis view is limited to 50 files because it contains too many changes.
See raw diff
- .env_gpt4all +17 -0
- .gitattributes +26 -0
- .github/workflows/snyk-scan.yml +76 -0
- .gitignore +37 -0
- Dockerfile +20 -0
- Dockerfile-runner.in +15 -0
- LICENSE +201 -0
- Makefile +63 -0
- README.md +209 -7
- __pycache__/enums.cpython-310.pyc +0 -0
- __pycache__/generate.cpython-310.pyc +0 -0
- __pycache__/gpt4all_llm.cpython-310.pyc +0 -0
- __pycache__/gpt_langchain.cpython-310.pyc +0 -0
- __pycache__/gradio_runner.cpython-310.pyc +0 -0
- __pycache__/gradio_themes.cpython-310.pyc +0 -0
- __pycache__/h2oai_pipeline.cpython-310.pyc +0 -0
- __pycache__/loaders.cpython-310.pyc +0 -0
- __pycache__/prompter.cpython-310.pyc +0 -0
- __pycache__/stopping.cpython-310.pyc +0 -0
- __pycache__/utils.cpython-310.pyc +0 -0
- __pycache__/utils_langchain.cpython-310.pyc +0 -0
- blog/README.md +81 -0
- ci/jenkinsfile +158 -0
- cli.py +108 -0
- client/.gitignore +164 -0
- client/Makefile +25 -0
- client/README.md +41 -0
- client/h2ogpt_client/__init__.py +4 -0
- client/h2ogpt_client/core.py +314 -0
- client/poetry.lock +876 -0
- client/poetry.toml +1 -0
- client/pyproject.toml +39 -0
- client/tests/__init__.py +0 -0
- client/tests/test_client.py +55 -0
- client_test.py +337 -0
- create_data.py +1809 -0
- data/NGSL_1.2_stats.csv.zip +3 -0
- data/README-template.md +23 -0
- data/censor_words.txt +10 -0
- data/config.json +0 -0
- data/count_1w.txt.zip +3 -0
- data/create_data_cards.py +144 -0
- data/dai_docs.train.json +0 -0
- data/dai_docs.train_cleaned.json +0 -0
- data/dai_docs.valid.json +101 -0
- data/dai_faq.json +477 -0
- data/h2ogpt-personality.json +642 -0
- data/merged.json +0 -0
- data/pexels-evg-kowalievska-1170986_small.jpg +0 -0
- docker-compose.yml +28 -0
.env_gpt4all
ADDED
@@ -0,0 +1,17 @@
|
|
|
|
|
|
|
|
|
|
|
|
|
|
|
|
|
|
|
|
|
|
|
|
|
|
|
|
|
|
|
|
|
|
|
|
|
1 |
+
# GPT4ALL or llama-cpp-python model_kwargs
|
2 |
+
|
3 |
+
# GPT4ALl GPT-J type, from model explorer choice, so downloads
|
4 |
+
model_name_gptj=ggml-gpt4all-j-v1.3-groovy.bin
|
5 |
+
|
6 |
+
# llama-cpp-python type, supporting version 3 quantization, here from locally built llama.cpp q4 v3 quantization
|
7 |
+
# below uses prompt_type=wizard2
|
8 |
+
model_path_llama=WizardLM-7B-uncensored.ggmlv3.q8_0.bin
|
9 |
+
# below assumes max_new_tokens=256
|
10 |
+
n_ctx=1792
|
11 |
+
# uncomment below if using llama-cpp-pyton with cublas built in
|
12 |
+
# n_gpu_layers=20
|
13 |
+
|
14 |
+
# GPT4ALl LLaMa type, supporting version 2 quantization, here from model explorer choice so downloads
|
15 |
+
model_name_gpt4all_llama=ggml-wizardLM-7B.q4_2.bin
|
16 |
+
|
17 |
+
# PDF_CLASS_NAME=UnstructuredPDFLoader
|
.gitattributes
CHANGED
@@ -33,3 +33,29 @@ saved_model/**/* filter=lfs diff=lfs merge=lfs -text
|
|
33 |
*.zip filter=lfs diff=lfs merge=lfs -text
|
34 |
*.zst filter=lfs diff=lfs merge=lfs -text
|
35 |
*tfevents* filter=lfs diff=lfs merge=lfs -text
|
|
|
|
|
|
|
|
|
|
|
|
|
|
|
|
|
|
|
|
|
|
|
|
|
|
|
|
|
|
|
|
|
|
|
|
|
|
|
|
|
|
|
|
|
|
|
|
|
|
|
|
|
|
33 |
*.zip filter=lfs diff=lfs merge=lfs -text
|
34 |
*.zst filter=lfs diff=lfs merge=lfs -text
|
35 |
*tfevents* filter=lfs diff=lfs merge=lfs -text
|
36 |
+
offline_folder/embed_out.weight.dat filter=lfs diff=lfs merge=lfs -text
|
37 |
+
offline_folder/gpt_neox.layers.27.attention.bias.dat filter=lfs diff=lfs merge=lfs -text
|
38 |
+
offline_folder/gpt_neox.layers.27.attention.dense.weight.dat filter=lfs diff=lfs merge=lfs -text
|
39 |
+
offline_folder/gpt_neox.layers.27.attention.query_key_value.weight.dat filter=lfs diff=lfs merge=lfs -text
|
40 |
+
offline_folder/gpt_neox.layers.27.mlp.dense_4h_to_h.weight.dat filter=lfs diff=lfs merge=lfs -text
|
41 |
+
offline_folder/gpt_neox.layers.27.mlp.dense_h_to_4h.weight.dat filter=lfs diff=lfs merge=lfs -text
|
42 |
+
offline_folder/gpt_neox.layers.28.attention.bias.dat filter=lfs diff=lfs merge=lfs -text
|
43 |
+
offline_folder/gpt_neox.layers.28.attention.dense.weight.dat filter=lfs diff=lfs merge=lfs -text
|
44 |
+
offline_folder/gpt_neox.layers.28.attention.query_key_value.weight.dat filter=lfs diff=lfs merge=lfs -text
|
45 |
+
offline_folder/gpt_neox.layers.28.mlp.dense_4h_to_h.weight.dat filter=lfs diff=lfs merge=lfs -text
|
46 |
+
offline_folder/gpt_neox.layers.28.mlp.dense_h_to_4h.weight.dat filter=lfs diff=lfs merge=lfs -text
|
47 |
+
offline_folder/gpt_neox.layers.29.attention.bias.dat filter=lfs diff=lfs merge=lfs -text
|
48 |
+
offline_folder/gpt_neox.layers.29.attention.dense.weight.dat filter=lfs diff=lfs merge=lfs -text
|
49 |
+
offline_folder/gpt_neox.layers.29.attention.query_key_value.weight.dat filter=lfs diff=lfs merge=lfs -text
|
50 |
+
offline_folder/gpt_neox.layers.29.mlp.dense_4h_to_h.weight.dat filter=lfs diff=lfs merge=lfs -text
|
51 |
+
offline_folder/gpt_neox.layers.29.mlp.dense_h_to_4h.weight.dat filter=lfs diff=lfs merge=lfs -text
|
52 |
+
offline_folder/gpt_neox.layers.30.attention.bias.dat filter=lfs diff=lfs merge=lfs -text
|
53 |
+
offline_folder/gpt_neox.layers.30.attention.dense.weight.dat filter=lfs diff=lfs merge=lfs -text
|
54 |
+
offline_folder/gpt_neox.layers.30.attention.query_key_value.weight.dat filter=lfs diff=lfs merge=lfs -text
|
55 |
+
offline_folder/gpt_neox.layers.30.mlp.dense_4h_to_h.weight.dat filter=lfs diff=lfs merge=lfs -text
|
56 |
+
offline_folder/gpt_neox.layers.30.mlp.dense_h_to_4h.weight.dat filter=lfs diff=lfs merge=lfs -text
|
57 |
+
offline_folder/gpt_neox.layers.31.attention.bias.dat filter=lfs diff=lfs merge=lfs -text
|
58 |
+
offline_folder/gpt_neox.layers.31.attention.dense.weight.dat filter=lfs diff=lfs merge=lfs -text
|
59 |
+
offline_folder/gpt_neox.layers.31.attention.query_key_value.weight.dat filter=lfs diff=lfs merge=lfs -text
|
60 |
+
offline_folder/gpt_neox.layers.31.mlp.dense_4h_to_h.weight.dat filter=lfs diff=lfs merge=lfs -text
|
61 |
+
offline_folder/gpt_neox.layers.31.mlp.dense_h_to_4h.weight.dat filter=lfs diff=lfs merge=lfs -text
|
.github/workflows/snyk-scan.yml
ADDED
@@ -0,0 +1,76 @@
|
|
|
|
|
|
|
|
|
|
|
|
|
|
|
|
|
|
|
|
|
|
|
|
|
|
|
|
|
|
|
|
|
|
|
|
|
|
|
|
|
|
|
|
|
|
|
|
|
|
|
|
|
|
|
|
|
|
|
|
|
|
|
|
|
|
|
|
|
|
|
|
|
|
|
|
|
|
|
|
|
|
|
|
|
|
|
|
|
|
|
|
|
|
|
|
|
|
|
|
|
|
|
|
|
|
|
|
|
|
|
|
|
|
|
|
|
|
|
|
|
|
|
|
|
|
|
|
|
|
|
|
|
|
|
|
|
|
|
|
|
|
|
|
|
|
|
|
|
|
|
|
|
|
|
1 |
+
name: Snyk Security Vulnerability Scan
|
2 |
+
|
3 |
+
on:
|
4 |
+
workflow_dispatch:
|
5 |
+
pull_request:
|
6 |
+
push:
|
7 |
+
tags:
|
8 |
+
- 'v[0-9]+.[0-9]+.[0-9]+'
|
9 |
+
branches:
|
10 |
+
- main
|
11 |
+
|
12 |
+
jobs:
|
13 |
+
snyk_scan_test:
|
14 |
+
if: ${{ github.event_name == 'pull_request' || github.event_name == 'workflow_dispatch' }}
|
15 |
+
runs-on: ubuntu-latest
|
16 |
+
steps:
|
17 |
+
- uses: actions/checkout@master
|
18 |
+
- uses: snyk/actions/setup@master
|
19 |
+
|
20 |
+
- uses: actions/setup-python@v4
|
21 |
+
with:
|
22 |
+
python-version: '3.10'
|
23 |
+
|
24 |
+
- name: Check changed Deps files
|
25 |
+
uses: tj-actions/changed-files@v35
|
26 |
+
id: changed-files
|
27 |
+
with:
|
28 |
+
files: | # This will match all the files with below patterns
|
29 |
+
requirements.txt
|
30 |
+
|
31 |
+
- name: Scan python dependencies
|
32 |
+
if: contains(steps.changed-files.outputs.all_changed_and_modified_files, 'requirements.txt')
|
33 |
+
env:
|
34 |
+
SNYK_TOKEN: '${{ secrets.SNYK_TOKEN }}'
|
35 |
+
run: |
|
36 |
+
head -n 41 requirements.txt > temp-requirements.txt #remove test deps
|
37 |
+
python3.10 -m pip install -r temp-requirements.txt
|
38 |
+
snyk test \
|
39 |
+
-d \
|
40 |
+
--file=temp-requirements.txt \
|
41 |
+
--package-manager=pip \
|
42 |
+
--command=python3.10 \
|
43 |
+
--skip-unresolved \
|
44 |
+
--severity-threshold=high
|
45 |
+
|
46 |
+
snyk_scan_monitor:
|
47 |
+
if: ${{ github.event_name == 'push' || github.event_name == 'workflow_dispatch'}}
|
48 |
+
runs-on: ubuntu-latest
|
49 |
+
steps:
|
50 |
+
- uses: actions/checkout@master
|
51 |
+
- uses: snyk/actions/setup@master
|
52 |
+
|
53 |
+
- uses: actions/setup-python@v4
|
54 |
+
with:
|
55 |
+
python-version: '3.10'
|
56 |
+
|
57 |
+
- name: Extract github branch/tag name
|
58 |
+
shell: bash
|
59 |
+
run: echo "ref=$(echo ${GITHUB_REF##*/})" >> $GITHUB_OUTPUT
|
60 |
+
id: extract_ref
|
61 |
+
|
62 |
+
- name: Monitor python dependencies
|
63 |
+
env:
|
64 |
+
SNYK_TOKEN: '${{ secrets.SNYK_TOKEN }}'
|
65 |
+
run: |
|
66 |
+
head -n 41 requirements.txt > temp-requirements.txt #remove test deps
|
67 |
+
python3.10 -m pip install -r temp-requirements.txt
|
68 |
+
snyk monitor \
|
69 |
+
-d \
|
70 |
+
--file=temp-requirements.txt \
|
71 |
+
--command=python3.10 \
|
72 |
+
--package-manager=pip \
|
73 |
+
--skip-unresolved \
|
74 |
+
--remote-repo-url=h2ogpt/${{ steps.extract_ref.outputs.ref }} \
|
75 |
+
--org=h2o-gpt \
|
76 |
+
--project-name=H2O-GPT/h2ogpt/${{ steps.extract_ref.outputs.ref }}/requirements.txt
|
.gitignore
ADDED
@@ -0,0 +1,37 @@
|
|
|
|
|
|
|
|
|
|
|
|
|
|
|
|
|
|
|
|
|
|
|
|
|
|
|
|
|
|
|
|
|
|
|
|
|
|
|
|
|
|
|
|
|
|
|
|
|
|
|
|
|
|
|
|
|
|
|
|
|
|
|
|
|
|
|
|
|
|
|
|
|
|
|
|
|
1 |
+
out/
|
2 |
+
7B/
|
3 |
+
13B/
|
4 |
+
__pycache__/
|
5 |
+
checkpoint**
|
6 |
+
minimal-llama**
|
7 |
+
upload.py
|
8 |
+
lora-**
|
9 |
+
*ckpt
|
10 |
+
wandb
|
11 |
+
evaluate.py
|
12 |
+
test_data.json
|
13 |
+
todo.txt
|
14 |
+
.neptune/
|
15 |
+
*.bin
|
16 |
+
db_dir_UserData
|
17 |
+
temp_path_do_doc1
|
18 |
+
offline_folder
|
19 |
+
flagged_data_points
|
20 |
+
.pytest_cache
|
21 |
+
user_path
|
22 |
+
user_path_test
|
23 |
+
build
|
24 |
+
h2ogpt.egg-info
|
25 |
+
dist
|
26 |
+
.idea
|
27 |
+
.cache
|
28 |
+
.local
|
29 |
+
.bash_history
|
30 |
+
.benchmarks
|
31 |
+
Dockerfile-runner.dockerfile
|
32 |
+
|
33 |
+
# IDEs
|
34 |
+
.idea/
|
35 |
+
|
36 |
+
# virtual envs
|
37 |
+
venv
|
Dockerfile
ADDED
@@ -0,0 +1,20 @@
|
|
|
|
|
|
|
|
|
|
|
|
|
|
|
|
|
|
|
|
|
|
|
|
|
|
|
|
|
|
|
|
|
|
|
|
|
|
|
|
|
|
|
1 |
+
# devel needed for bitsandbytes requirement of libcudart.so, otherwise runtime sufficient
|
2 |
+
FROM nvidia/cuda:12.1.1-cudnn8-devel-ubuntu20.04
|
3 |
+
|
4 |
+
ARG DEBIAN_FRONTEND=noninteractive
|
5 |
+
|
6 |
+
RUN apt-get update && apt-get install -y \
|
7 |
+
git \
|
8 |
+
curl \
|
9 |
+
wget \
|
10 |
+
software-properties-common \
|
11 |
+
pandoc \
|
12 |
+
&& add-apt-repository ppa:deadsnakes/ppa \
|
13 |
+
&& apt install -y python3.10 python3-dev libpython3.10-dev \
|
14 |
+
&& rm -rf /var/lib/apt/lists/*
|
15 |
+
WORKDIR /workspace
|
16 |
+
COPY requirements.txt requirements.txt
|
17 |
+
RUN curl -sS https://bootstrap.pypa.io/get-pip.py | python3.10
|
18 |
+
RUN python3.10 -m pip install -r requirements.txt
|
19 |
+
COPY . .
|
20 |
+
ENTRYPOINT [ "python3.10"]
|
Dockerfile-runner.in
ADDED
@@ -0,0 +1,15 @@
|
|
|
|
|
|
|
|
|
|
|
|
|
|
|
|
|
|
|
|
|
|
|
|
|
|
|
|
|
|
|
|
|
1 |
+
FROM BASE_DOCKER_IMAGE_SUBST
|
2 |
+
|
3 |
+
LABEL imagetype="runtime-h2ogpt"
|
4 |
+
LABEL maintainer="H2O.ai <[email protected]>"
|
5 |
+
|
6 |
+
ENV DEBIAN_FRONTEND=noninteractive
|
7 |
+
ENV TRANSFORMERS_CACHE=/h2ogpt_env/.cache
|
8 |
+
ENV HF_MODEL=h2oai/h2ogpt-gm-oasst1-en-2048-falcon-7b-v3
|
9 |
+
|
10 |
+
COPY run-gpt.sh /run-gpt.sh
|
11 |
+
|
12 |
+
EXPOSE 8888
|
13 |
+
EXPOSE 7860
|
14 |
+
|
15 |
+
ENTRYPOINT ["/run-gpt.sh"]
|
LICENSE
ADDED
@@ -0,0 +1,201 @@
|
|
|
|
|
|
|
|
|
|
|
|
|
|
|
|
|
|
|
|
|
|
|
|
|
|
|
|
|
|
|
|
|
|
|
|
|
|
|
|
|
|
|
|
|
|
|
|
|
|
|
|
|
|
|
|
|
|
|
|
|
|
|
|
|
|
|
|
|
|
|
|
|
|
|
|
|
|
|
|
|
|
|
|
|
|
|
|
|
|
|
|
|
|
|
|
|
|
|
|
|
|
|
|
|
|
|
|
|
|
|
|
|
|
|
|
|
|
|
|
|
|
|
|
|
|
|
|
|
|
|
|
|
|
|
|
|
|
|
|
|
|
|
|
|
|
|
|
|
|
|
|
|
|
|
|
|
|
|
|
|
|
|
|
|
|
|
|
|
|
|
|
|
|
|
|
|
|
|
|
|
|
|
|
|
|
|
|
|
|
|
|
|
|
|
|
|
|
|
|
|
|
|
|
|
|
|
|
|
|
|
|
|
|
|
|
|
|
|
|
|
|
|
|
|
|
|
|
|
|
|
|
|
|
|
|
|
|
|
|
|
|
|
|
|
|
|
|
|
|
|
|
|
|
|
|
|
|
|
|
|
|
|
|
|
|
|
|
|
|
|
|
|
|
|
|
|
|
|
|
|
|
|
|
|
|
|
|
|
|
|
|
|
|
|
|
|
|
|
|
|
|
|
|
|
|
|
|
|
|
|
|
|
|
|
|
|
|
|
|
|
|
|
|
|
|
|
|
|
|
|
|
|
|
|
|
|
|
|
|
|
|
|
|
|
|
|
|
|
|
|
|
|
|
|
|
|
|
|
|
|
|
|
|
|
|
|
|
|
|
|
|
|
|
|
|
|
|
|
|
|
|
|
|
|
|
|
|
|
|
|
|
|
|
|
|
|
|
|
|
|
|
|
|
|
1 |
+
Apache License
|
2 |
+
Version 2.0, January 2004
|
3 |
+
http://www.apache.org/licenses/
|
4 |
+
|
5 |
+
TERMS AND CONDITIONS FOR USE, REPRODUCTION, AND DISTRIBUTION
|
6 |
+
|
7 |
+
1. Definitions.
|
8 |
+
|
9 |
+
"License" shall mean the terms and conditions for use, reproduction,
|
10 |
+
and distribution as defined by Sections 1 through 9 of this document.
|
11 |
+
|
12 |
+
"Licensor" shall mean the copyright owner or entity authorized by
|
13 |
+
the copyright owner that is granting the License.
|
14 |
+
|
15 |
+
"Legal Entity" shall mean the union of the acting entity and all
|
16 |
+
other entities that control, are controlled by, or are under common
|
17 |
+
control with that entity. For the purposes of this definition,
|
18 |
+
"control" means (i) the power, direct or indirect, to cause the
|
19 |
+
direction or management of such entity, whether by contract or
|
20 |
+
otherwise, or (ii) ownership of fifty percent (50%) or more of the
|
21 |
+
outstanding shares, or (iii) beneficial ownership of such entity.
|
22 |
+
|
23 |
+
"You" (or "Your") shall mean an individual or Legal Entity
|
24 |
+
exercising permissions granted by this License.
|
25 |
+
|
26 |
+
"Source" form shall mean the preferred form for making modifications,
|
27 |
+
including but not limited to software source code, documentation
|
28 |
+
source, and configuration files.
|
29 |
+
|
30 |
+
"Object" form shall mean any form resulting from mechanical
|
31 |
+
transformation or translation of a Source form, including but
|
32 |
+
not limited to compiled object code, generated documentation,
|
33 |
+
and conversions to other media types.
|
34 |
+
|
35 |
+
"Work" shall mean the work of authorship, whether in Source or
|
36 |
+
Object form, made available under the License, as indicated by a
|
37 |
+
copyright notice that is included in or attached to the work
|
38 |
+
(an example is provided in the Appendix below).
|
39 |
+
|
40 |
+
"Derivative Works" shall mean any work, whether in Source or Object
|
41 |
+
form, that is based on (or derived from) the Work and for which the
|
42 |
+
editorial revisions, annotations, elaborations, or other modifications
|
43 |
+
represent, as a whole, an original work of authorship. For the purposes
|
44 |
+
of this License, Derivative Works shall not include works that remain
|
45 |
+
separable from, or merely link (or bind by name) to the interfaces of,
|
46 |
+
the Work and Derivative Works thereof.
|
47 |
+
|
48 |
+
"Contribution" shall mean any work of authorship, including
|
49 |
+
the original version of the Work and any modifications or additions
|
50 |
+
to that Work or Derivative Works thereof, that is intentionally
|
51 |
+
submitted to Licensor for inclusion in the Work by the copyright owner
|
52 |
+
or by an individual or Legal Entity authorized to submit on behalf of
|
53 |
+
the copyright owner. For the purposes of this definition, "submitted"
|
54 |
+
means any form of electronic, verbal, or written communication sent
|
55 |
+
to the Licensor or its representatives, including but not limited to
|
56 |
+
communication on electronic mailing lists, source code control systems,
|
57 |
+
and issue tracking systems that are managed by, or on behalf of, the
|
58 |
+
Licensor for the purpose of discussing and improving the Work, but
|
59 |
+
excluding communication that is conspicuously marked or otherwise
|
60 |
+
designated in writing by the copyright owner as "Not a Contribution."
|
61 |
+
|
62 |
+
"Contributor" shall mean Licensor and any individual or Legal Entity
|
63 |
+
on behalf of whom a Contribution has been received by Licensor and
|
64 |
+
subsequently incorporated within the Work.
|
65 |
+
|
66 |
+
2. Grant of Copyright License. Subject to the terms and conditions of
|
67 |
+
this License, each Contributor hereby grants to You a perpetual,
|
68 |
+
worldwide, non-exclusive, no-charge, royalty-free, irrevocable
|
69 |
+
copyright license to reproduce, prepare Derivative Works of,
|
70 |
+
publicly display, publicly perform, sublicense, and distribute the
|
71 |
+
Work and such Derivative Works in Source or Object form.
|
72 |
+
|
73 |
+
3. Grant of Patent License. Subject to the terms and conditions of
|
74 |
+
this License, each Contributor hereby grants to You a perpetual,
|
75 |
+
worldwide, non-exclusive, no-charge, royalty-free, irrevocable
|
76 |
+
(except as stated in this section) patent license to make, have made,
|
77 |
+
use, offer to sell, sell, import, and otherwise transfer the Work,
|
78 |
+
where such license applies only to those patent claims licensable
|
79 |
+
by such Contributor that are necessarily infringed by their
|
80 |
+
Contribution(s) alone or by combination of their Contribution(s)
|
81 |
+
with the Work to which such Contribution(s) was submitted. If You
|
82 |
+
institute patent litigation against any entity (including a
|
83 |
+
cross-claim or counterclaim in a lawsuit) alleging that the Work
|
84 |
+
or a Contribution incorporated within the Work constitutes direct
|
85 |
+
or contributory patent infringement, then any patent licenses
|
86 |
+
granted to You under this License for that Work shall terminate
|
87 |
+
as of the date such litigation is filed.
|
88 |
+
|
89 |
+
4. Redistribution. You may reproduce and distribute copies of the
|
90 |
+
Work or Derivative Works thereof in any medium, with or without
|
91 |
+
modifications, and in Source or Object form, provided that You
|
92 |
+
meet the following conditions:
|
93 |
+
|
94 |
+
(a) You must give any other recipients of the Work or
|
95 |
+
Derivative Works a copy of this License; and
|
96 |
+
|
97 |
+
(b) You must cause any modified files to carry prominent notices
|
98 |
+
stating that You changed the files; and
|
99 |
+
|
100 |
+
(c) You must retain, in the Source form of any Derivative Works
|
101 |
+
that You distribute, all copyright, patent, trademark, and
|
102 |
+
attribution notices from the Source form of the Work,
|
103 |
+
excluding those notices that do not pertain to any part of
|
104 |
+
the Derivative Works; and
|
105 |
+
|
106 |
+
(d) If the Work includes a "NOTICE" text file as part of its
|
107 |
+
distribution, then any Derivative Works that You distribute must
|
108 |
+
include a readable copy of the attribution notices contained
|
109 |
+
within such NOTICE file, excluding those notices that do not
|
110 |
+
pertain to any part of the Derivative Works, in at least one
|
111 |
+
of the following places: within a NOTICE text file distributed
|
112 |
+
as part of the Derivative Works; within the Source form or
|
113 |
+
documentation, if provided along with the Derivative Works; or,
|
114 |
+
within a display generated by the Derivative Works, if and
|
115 |
+
wherever such third-party notices normally appear. The contents
|
116 |
+
of the NOTICE file are for informational purposes only and
|
117 |
+
do not modify the License. You may add Your own attribution
|
118 |
+
notices within Derivative Works that You distribute, alongside
|
119 |
+
or as an addendum to the NOTICE text from the Work, provided
|
120 |
+
that such additional attribution notices cannot be construed
|
121 |
+
as modifying the License.
|
122 |
+
|
123 |
+
You may add Your own copyright statement to Your modifications and
|
124 |
+
may provide additional or different license terms and conditions
|
125 |
+
for use, reproduction, or distribution of Your modifications, or
|
126 |
+
for any such Derivative Works as a whole, provided Your use,
|
127 |
+
reproduction, and distribution of the Work otherwise complies with
|
128 |
+
the conditions stated in this License.
|
129 |
+
|
130 |
+
5. Submission of Contributions. Unless You explicitly state otherwise,
|
131 |
+
any Contribution intentionally submitted for inclusion in the Work
|
132 |
+
by You to the Licensor shall be under the terms and conditions of
|
133 |
+
this License, without any additional terms or conditions.
|
134 |
+
Notwithstanding the above, nothing herein shall supersede or modify
|
135 |
+
the terms of any separate license agreement you may have executed
|
136 |
+
with Licensor regarding such Contributions.
|
137 |
+
|
138 |
+
6. Trademarks. This License does not grant permission to use the trade
|
139 |
+
names, trademarks, service marks, or product names of the Licensor,
|
140 |
+
except as required for reasonable and customary use in describing the
|
141 |
+
origin of the Work and reproducing the content of the NOTICE file.
|
142 |
+
|
143 |
+
7. Disclaimer of Warranty. Unless required by applicable law or
|
144 |
+
agreed to in writing, Licensor provides the Work (and each
|
145 |
+
Contributor provides its Contributions) on an "AS IS" BASIS,
|
146 |
+
WITHOUT WARRANTIES OR CONDITIONS OF ANY KIND, either express or
|
147 |
+
implied, including, without limitation, any warranties or conditions
|
148 |
+
of TITLE, NON-INFRINGEMENT, MERCHANTABILITY, or FITNESS FOR A
|
149 |
+
PARTICULAR PURPOSE. You are solely responsible for determining the
|
150 |
+
appropriateness of using or redistributing the Work and assume any
|
151 |
+
risks associated with Your exercise of permissions under this License.
|
152 |
+
|
153 |
+
8. Limitation of Liability. In no event and under no legal theory,
|
154 |
+
whether in tort (including negligence), contract, or otherwise,
|
155 |
+
unless required by applicable law (such as deliberate and grossly
|
156 |
+
negligent acts) or agreed to in writing, shall any Contributor be
|
157 |
+
liable to You for damages, including any direct, indirect, special,
|
158 |
+
incidental, or consequential damages of any character arising as a
|
159 |
+
result of this License or out of the use or inability to use the
|
160 |
+
Work (including but not limited to damages for loss of goodwill,
|
161 |
+
work stoppage, computer failure or malfunction, or any and all
|
162 |
+
other commercial damages or losses), even if such Contributor
|
163 |
+
has been advised of the possibility of such damages.
|
164 |
+
|
165 |
+
9. Accepting Warranty or Additional Liability. While redistributing
|
166 |
+
the Work or Derivative Works thereof, You may choose to offer,
|
167 |
+
and charge a fee for, acceptance of support, warranty, indemnity,
|
168 |
+
or other liability obligations and/or rights consistent with this
|
169 |
+
License. However, in accepting such obligations, You may act only
|
170 |
+
on Your own behalf and on Your sole responsibility, not on behalf
|
171 |
+
of any other Contributor, and only if You agree to indemnify,
|
172 |
+
defend, and hold each Contributor harmless for any liability
|
173 |
+
incurred by, or claims asserted against, such Contributor by reason
|
174 |
+
of your accepting any such warranty or additional liability.
|
175 |
+
|
176 |
+
END OF TERMS AND CONDITIONS
|
177 |
+
|
178 |
+
APPENDIX: How to apply the Apache License to your work.
|
179 |
+
|
180 |
+
To apply the Apache License to your work, attach the following
|
181 |
+
boilerplate notice, with the fields enclosed by brackets "[]"
|
182 |
+
replaced with your own identifying information. (Don't include
|
183 |
+
the brackets!) The text should be enclosed in the appropriate
|
184 |
+
comment syntax for the file format. We also recommend that a
|
185 |
+
file or class name and description of purpose be included on the
|
186 |
+
same "printed page" as the copyright notice for easier
|
187 |
+
identification within third-party archives.
|
188 |
+
|
189 |
+
Copyright 2023 Rohan Taori, Ishaan Gulrajani, Tianyi Zhang, Yann Dubois, Xuechen Li
|
190 |
+
|
191 |
+
Licensed under the Apache License, Version 2.0 (the "License");
|
192 |
+
you may not use this file except in compliance with the License.
|
193 |
+
You may obtain a copy of the License at
|
194 |
+
|
195 |
+
http://www.apache.org/licenses/LICENSE-2.0
|
196 |
+
|
197 |
+
Unless required by applicable law or agreed to in writing, software
|
198 |
+
distributed under the License is distributed on an "AS IS" BASIS,
|
199 |
+
WITHOUT WARRANTIES OR CONDITIONS OF ANY KIND, either express or implied.
|
200 |
+
See the License for the specific language governing permissions and
|
201 |
+
limitations under the License.
|
Makefile
ADDED
@@ -0,0 +1,63 @@
|
|
|
|
|
|
|
|
|
|
|
|
|
|
|
|
|
|
|
|
|
|
|
|
|
|
|
|
|
|
|
|
|
|
|
|
|
|
|
|
|
|
|
|
|
|
|
|
|
|
|
|
|
|
|
|
|
|
|
|
|
|
|
|
|
|
|
|
|
|
|
|
|
|
|
|
|
|
|
|
|
|
|
|
|
|
|
|
|
|
|
|
|
|
|
|
|
|
|
|
|
|
|
|
|
|
|
|
|
|
|
|
|
|
|
|
|
|
|
|
|
|
|
|
|
|
|
|
|
1 |
+
all: clean dist
|
2 |
+
|
3 |
+
PACKAGE_VERSION := `cat version.txt | tr -d '\n'`
|
4 |
+
BUILD_TAG_FILES := requirements.txt Dockerfile `ls reqs_optional/*.txt | sort`
|
5 |
+
BUILD_TAG := $(shell md5sum $(BUILD_TAG_FILES) 2> /dev/null | sort | md5sum | cut -d' ' -f1)
|
6 |
+
DOCKER_TEST_IMAGE := harbor.h2o.ai/h2ogpt/test-image:$(BUILD_TAG)
|
7 |
+
DOCKER_RUN_IMAGE := $(DOCKER_TEST_IMAGE)-runtime
|
8 |
+
PYTHON_BINARY ?= `which python`
|
9 |
+
DEFAULT_MARKERS ?= "not need_tokens and not need_gpu"
|
10 |
+
|
11 |
+
.PHONY: reqs_optional/req_constraints.txt venv dist test publish docker_build
|
12 |
+
|
13 |
+
reqs_optional/req_constraints.txt:
|
14 |
+
grep -v '#\|peft\|transformers\|accelerate' requirements.txt > $@
|
15 |
+
|
16 |
+
clean:
|
17 |
+
rm -rf dist build h2ogpt.egg-info
|
18 |
+
|
19 |
+
venv:
|
20 |
+
$(PYTHON_BINARY) -m virtualenv -p $(PYTHON_BINARY) venv
|
21 |
+
|
22 |
+
install:
|
23 |
+
$(PYTHON_BINARY) -m pip install dist/h2ogpt-$(PACKAGE_VERSION)-py3-none-any.whl
|
24 |
+
|
25 |
+
install-%:
|
26 |
+
$(PYTHON_BINARY) -m pip install dist/h2ogpt-$(PACKAGE_VERSION)-py3-none-any.whl[$*]
|
27 |
+
|
28 |
+
dist:
|
29 |
+
$(PYTHON_BINARY) setup.py bdist_wheel
|
30 |
+
|
31 |
+
test:
|
32 |
+
$(PYTHON_BINARY) -m pip install requirements-parser -c reqs_optional/req_constraints.txt
|
33 |
+
$(PYTHON_BINARY) -m pytest tests --disable-warnings --junit-xml=test_report.xml -m "$(DEFAULT_MARKERS)"
|
34 |
+
|
35 |
+
test_imports:
|
36 |
+
$(PYTHON_BINARY) -m pytest tests/test_imports.py --disable-warnings --junit-xml=test_report.xml -m "$(DEFAULT_MARKERS)"
|
37 |
+
|
38 |
+
publish:
|
39 |
+
echo "Publishing not implemented yet."
|
40 |
+
|
41 |
+
docker_build:
|
42 |
+
ifeq ($(shell curl --write-out %{http_code} -sS --output /dev/null -X GET http://harbor.h2o.ai/api/v2.0/projects/h2ogpt/repositories/test-image/artifacts/$(BUILD_TAG)/tags),200)
|
43 |
+
@echo "Image already pushed to Harbor: $(DOCKER_TEST_IMAGE)"
|
44 |
+
else
|
45 |
+
DOCKER_BUILDKIT=1 docker build -t $(DOCKER_TEST_IMAGE) -f Dockerfile .
|
46 |
+
docker push $(DOCKER_TEST_IMAGE)
|
47 |
+
endif
|
48 |
+
|
49 |
+
.PHONY: Dockerfile-runner.dockerfile
|
50 |
+
|
51 |
+
Dockerfile-runner.dockerfile: Dockerfile-runner.in
|
52 |
+
cat $< \
|
53 |
+
| sed 's|BASE_DOCKER_IMAGE_SUBST|$(DOCKER_TEST_IMAGE)|g' \
|
54 |
+
> $@
|
55 |
+
|
56 |
+
docker_build_runner: docker_build Dockerfile-runner.dockerfile
|
57 |
+
docker pull $(DOCKER_TEST_IMAGE)
|
58 |
+
DOCKER_BUILDKIT=1 docker build -t $(DOCKER_RUN_IMAGE) -f Dockerfile-runner.dockerfile .
|
59 |
+
docker push $(DOCKER_RUN_IMAGE)
|
60 |
+
docker tag $(DOCKER_RUN_IMAGE) gcr.io/vorvan/h2oai/h2ogpt-runtime:$(BUILD_TAG)
|
61 |
+
|
62 |
+
print-%:
|
63 |
+
@echo $($*)
|
README.md
CHANGED
@@ -1,12 +1,214 @@
|
|
1 |
---
|
2 |
title: H2OGPT
|
3 |
-
|
4 |
-
colorFrom: pink
|
5 |
-
colorTo: red
|
6 |
sdk: gradio
|
7 |
-
sdk_version: 3.
|
8 |
-
app_file: app.py
|
9 |
-
pinned: false
|
10 |
---
|
|
|
|
|
|
|
|
|
|
|
|
|
|
|
|
|
|
|
|
|
|
|
|
|
|
|
|
|
|
|
|
|
|
|
|
|
|
|
|
|
|
|
|
|
|
|
|
|
|
|
|
|
|
|
|
|
|
|
|
|
|
|
|
|
|
|
|
|
|
|
|
|
|
|
|
|
|
|
|
|
|
|
|
|
|
|
|
|
|
|
|
|
|
|
|
|
|
|
|
|
|
|
|
|
|
|
|
|
|
|
|
|
|
|
|
|
|
|
|
|
|
|
|
|
|
|
|
|
|
|
|
|
|
|
|
|
|
|
|
|
|
|
|
|
|
|
|
|
|
|
|
|
|
|
|
|
|
|
|
|
|
|
|
|
|
|
|
|
|
|
|
|
|
|
|
|
|
|
|
|
|
|
|
|
|
|
|
|
|
|
|
|
|
|
|
|
|
|
|
|
|
|
|
|
|
|
|
|
|
|
|
|
|
|
|
|
|
|
|
|
|
|
|
|
|
|
|
|
|
|
|
|
|
|
|
|
|
|
|
|
|
|
|
|
|
|
|
|
|
|
|
|
|
|
|
|
|
|
|
|
|
|
|
|
|
|
|
|
|
|
|
|
|
|
|
|
|
|
|
|
|
|
|
|
|
|
|
|
|
|
|
|
|
|
|
|
|
|
|
|
|
|
|
|
|
|
|
|
|
|
|
|
|
|
|
|
|
|
|
|
|
|
|
|
|
|
|
|
|
|
|
|
|
|
|
|
|
|
|
|
|
|
|
|
|
|
|
|
|
|
|
|
|
|
|
|
|
|
|
|
|
|
|
|
|
|
|
|
|
|
|
|
|
|
|
|
|
|
|
|
|
|
|
|
|
|
|
|
|
|
|
|
|
|
|
|
|
|
|
|
|
|
|
|
|
|
|
|
|
|
|
|
|
|
11 |
|
12 |
-
Check out the configuration reference at https://huggingface.co/docs/hub/spaces-config-reference
|
|
|
1 |
---
|
2 |
title: H2OGPT
|
3 |
+
app_file: gradio_runner.py
|
|
|
|
|
4 |
sdk: gradio
|
5 |
+
sdk_version: 3.35.2
|
|
|
|
|
6 |
---
|
7 |
+
## h2oGPT
|
8 |
+
|
9 |
+
[ Live h2oGPT Demo](https://gpt.h2o.ai/)
|
10 |
+
|
11 |
+
For questions, discussing, or just hanging out, come and join our <a href="https://discord.gg/WKhYMWcVbq"><b>Discord</b></a>!
|
12 |
+
|
13 |
+
Technical Paper: [https://arxiv.org/pdf/2306.08161.pdf](https://arxiv.org/pdf/2306.08161.pdf)
|
14 |
+
|
15 |
+
h2oGPT is a large language model (LLM) fine-tuning framework and chatbot UI with document(s) question-answer capabilities. Documents help to **ground** LLMs against hallucinations by providing them context relevant to the instruction. h2oGPT is fully permissive Apache V2 open-source project for 100% private and secure use of LLMs and document embeddings for document question-answer.
|
16 |
+
|
17 |
+
Welcome! Join us and make an issue or a PR, and contribute to making the best fine-tuned LLMs, chatbot UI, and document question-answer framework!
|
18 |
+
|
19 |
+
Turn ★ into ⭐ (top-right corner) if you like the project!
|
20 |
+
|
21 |
+
<!-- cat README.md | ./gh-md-toc - But Help is heavily processed -->
|
22 |
+
* [Supported OS and Hardware](#supported-os-and-hardware)
|
23 |
+
* [Apache V2 ChatBot with LangChain Integration](#apache-v2-chatbot-with-langchain-integration)
|
24 |
+
* [Apache V2 Data Preparation code, Training code, and Models](#apache-v2-data-preparation-code-training-code-and-models)
|
25 |
+
* [Roadmap](#roadmap)
|
26 |
+
* [Getting Started](#getting-started)
|
27 |
+
* [TLDR Install & Run](#tldr)
|
28 |
+
* [GPU (CUDA)](docs/README_GPU.md)
|
29 |
+
* [CPU](docs/README_CPU.md)
|
30 |
+
* [MACOS](docs/README_MACOS.md#macos)
|
31 |
+
* [Windows 10/11](docs/README_WINDOWS.md)
|
32 |
+
* [CLI chat](docs/README_CLI.md)
|
33 |
+
* [Gradio UI](docs/README_GRADIOUI.md)
|
34 |
+
* [Client API](docs/README_CLIENT.md)
|
35 |
+
* [Connect to Inference Servers](docs/README_InferenceServers.md)
|
36 |
+
* [Python Wheel](docs/README_WHEEL.md)
|
37 |
+
* [Development](#development)
|
38 |
+
* [Help](#help)
|
39 |
+
* [LangChain file types supported](docs/README_LangChain.md#supported-datatypes)
|
40 |
+
* [CLI Database control](docs/README_LangChain.md#database-creation)
|
41 |
+
* [Why h2oGPT for Doc Q&A](docs/README_LangChain.md#what-is-h2ogpts-langchain-integration-like)
|
42 |
+
* [FAQ](docs/FAQ.md)
|
43 |
+
* [Useful Links](docs/LINKS.md)
|
44 |
+
* [Fine-Tuning](docs/FINETUNE.md)
|
45 |
+
* [Docker](docs/INSTALL-DOCKER.md)
|
46 |
+
* [Triton](docs/TRITON.md)
|
47 |
+
* [Acknowledgements](#acknowledgements)
|
48 |
+
* [Why H2O.ai?](#why-h2oai)
|
49 |
+
* [Disclaimer](#disclaimer)
|
50 |
+
|
51 |
+
### Supported OS and Hardware
|
52 |
+
|
53 |
+
[](https://raw.githubusercontent.com/h2oai/h2ogpt/main/LICENSE)
|
54 |
+

|
55 |
+

|
56 |
+

|
57 |
+

|
58 |
+
|
59 |
+
**GPU** mode requires CUDA support via torch and transformers. A 6.9B (or 12GB) model in 8-bit uses 8GB (or 13GB) of GPU memory. 8-bit or 4-bit precision can further reduce memory requirements down no more than about 6.5GB when asking a question about your documents (see [low-memory mode](docs/FAQ.md#low-memory-mode)).
|
60 |
+
|
61 |
+
**CPU** mode uses GPT4ALL and LLaMa.cpp, e.g. gpt4all-j, requiring about 14GB of system RAM in typical use.
|
62 |
+
|
63 |
+
GPU and CPU mode tested on variety of NVIDIA GPUs in Ubuntu 18-22, but any modern Linux variant should work. MACOS support tested on Macbook Pro running Monterey v12.3.1 using CPU mode.
|
64 |
+
|
65 |
+
### Apache V2 ChatBot with LangChain Integration
|
66 |
+
|
67 |
+
- [**LangChain**](docs/README_LangChain.md) equipped Chatbot integration and streaming responses
|
68 |
+
- **Persistent** database using Chroma or in-memory with FAISS
|
69 |
+
- **Original** content url links and scores to rank content against query
|
70 |
+
- **Private** offline database of any documents ([PDFs, Images, and many more](docs/README_LangChain.md#supported-datatypes))
|
71 |
+
- **Upload** documents via chatbot into shared space or only allow scratch space
|
72 |
+
- **Control** data sources and the context provided to LLM
|
73 |
+
- **Efficient** use of context using instruct-tuned LLMs (no need for many examples)
|
74 |
+
- **API** for client-server control
|
75 |
+
- **CPU and GPU** support from variety of HF models, and CPU support using GPT4ALL and LLaMa cpp
|
76 |
+
- **Linux, MAC, and Windows** support
|
77 |
+
|
78 |
+
Light mode with soft colors talking to cat image:
|
79 |
+
|
80 |
+

|
81 |
+
|
82 |
+
Dark mode with H2O.ai colors:
|
83 |
+
<img src="docs/langchain.png" alt="VectorDB" title="VectorDB via LangChain">
|
84 |
+
|
85 |
+
### Apache V2 Data Preparation code, Training code, and Models
|
86 |
+
|
87 |
+
- **Variety** of models (h2oGPT, WizardLM, Vicuna, OpenAssistant, etc.) supported
|
88 |
+
- **Fully Commercially** Apache V2 code, data and models
|
89 |
+
- **High-Quality** data cleaning of large open-source instruction datasets
|
90 |
+
- **LoRA** and **QLoRA** (low-rank approximation) efficient 4-bit, 8-bit and 16-bit fine-tuning and generation
|
91 |
+
- **Large** (up to 65B parameters) models built on commodity or enterprise GPUs (single or multi node)
|
92 |
+
- **Evaluate** performance using RLHF-based reward models
|
93 |
+
|
94 |
+
https://user-images.githubusercontent.com/6147661/232924684-6c0e2dfb-2f24-4098-848a-c3e4396f29f6.mov
|
95 |
+
|
96 |
+
All open-source datasets and models are posted on [🤗 H2O.ai's Hugging Face page](https://huggingface.co/h2oai/).
|
97 |
+
|
98 |
+
Also check out [H2O LLM Studio](https://github.com/h2oai/h2o-llmstudio) for our no-code LLM fine-tuning framework!
|
99 |
+
|
100 |
+
### Roadmap
|
101 |
+
|
102 |
+
- Integration of code and resulting LLMs with downstream applications and low/no-code platforms
|
103 |
+
- Complement h2oGPT chatbot with search and other APIs
|
104 |
+
- High-performance distributed training of larger models on trillion tokens
|
105 |
+
- Enhance the model's code completion, reasoning, and mathematical capabilities, ensure factual correctness, minimize hallucinations, and avoid repetitive output
|
106 |
+
- Add other tools like search
|
107 |
+
- Add agents for SQL and CSV question/answer
|
108 |
+
|
109 |
+
### Getting Started
|
110 |
+
|
111 |
+
First one needs a Python 3.10 environment. For help installing a Python 3.10 environment, see [Install Python 3.10 Environment](docs/INSTALL.md#install-python-environment). On newer Ubuntu systems and environment may be installed by just doing:
|
112 |
+
```bash
|
113 |
+
sudo apt-get install -y build-essential gcc python3.10-dev
|
114 |
+
virtualenv -p python3 h2ogpt
|
115 |
+
source h2ogpt/bin/activate
|
116 |
+
```
|
117 |
+
Check your installation by doing:
|
118 |
+
```bash
|
119 |
+
python --version # should say 3.10.xx
|
120 |
+
pip --version # should say pip 23.x.y ... (python 3.10)
|
121 |
+
```
|
122 |
+
On some systems, `pip` still refers back to the system one, then one can use `python -m pip` or `pip3` instead of `pip` or try `python3` instead of `python`.
|
123 |
+
|
124 |
+
#### TLDR
|
125 |
+
|
126 |
+
After Python 3.10 environment installed:
|
127 |
+
```bash
|
128 |
+
git clone https://github.com/h2oai/h2ogpt.git
|
129 |
+
cd h2ogpt
|
130 |
+
# fix any bad env
|
131 |
+
pip uninstall -y pandoc pypandoc pypandoc-binary
|
132 |
+
# broad support, but no training-time or data creation dependencies
|
133 |
+
for fil in requirements.txt reqs_optional/requirements_optional_langchain.txt reqs_optional/requirements_optional_gpt4all.txt reqs_optional/requirements_optional_langchain.gpllike.txt reqs_optional/requirements_optional_langchain.urls.txt ; do pip install -r $fil ; done
|
134 |
+
# Optional: support docx, pptx, ArXiv, etc.
|
135 |
+
sudo apt-get install -y libmagic-dev poppler-utils tesseract-ocr libreoffice
|
136 |
+
# Optional: for supporting unstructured package
|
137 |
+
python -m nltk.downloader all
|
138 |
+
````
|
139 |
+
|
140 |
+
Place all documents in `user_path` or upload in UI.
|
141 |
+
|
142 |
+
UI using GPU with at least 24GB with streaming:
|
143 |
+
```bash
|
144 |
+
python generate.py --base_model=h2oai/h2ogpt-oasst1-512-12b --load_8bit=True --score_model=None --langchain_mode='UserData' --user_path=user_path
|
145 |
+
```
|
146 |
+
UI using CPU
|
147 |
+
```bash
|
148 |
+
python generate.py --base_model='llama' --prompt_type=wizard2 --score_model=None --langchain_mode='UserData' --user_path=user_path
|
149 |
+
```
|
150 |
+
|
151 |
+
### Development
|
152 |
+
|
153 |
+
- To create a development environment for training and generation, follow the [installation instructions](docs/INSTALL.md).
|
154 |
+
- To fine-tune any LLM models on your data, follow the [fine-tuning instructions](docs/FINETUNE.md).
|
155 |
+
- To create a container for deployment, follow the [Docker instructions](docs/INSTALL-DOCKER.md).
|
156 |
+
|
157 |
+
### Help
|
158 |
+
|
159 |
+
- Flash attention support, see [Flash Attention](docs/INSTALL.md#flash-attention)
|
160 |
+
|
161 |
+
- [Docker](docs/INSTALL-DOCKER.md#containerized-installation-for-inference-on-linux-gpu-servers) for inference.
|
162 |
+
|
163 |
+
- [FAQs](docs/FAQ.md)
|
164 |
+
|
165 |
+
- [README for LangChain](docs/README_LangChain.md)
|
166 |
+
|
167 |
+
- More [Links](docs/LINKS.md), context, competitors, models, datasets
|
168 |
+
|
169 |
+
### Acknowledgements
|
170 |
+
|
171 |
+
* Some training code was based upon March 24 version of [Alpaca-LoRA](https://github.com/tloen/alpaca-lora/).
|
172 |
+
* Used high-quality created data by [OpenAssistant](https://open-assistant.io/).
|
173 |
+
* Used base models by [EleutherAI](https://www.eleuther.ai/).
|
174 |
+
* Used OIG data created by [LAION](https://laion.ai/blog/oig-dataset/).
|
175 |
+
|
176 |
+
### Why H2O.ai?
|
177 |
+
|
178 |
+
Our [Makers](https://h2o.ai/company/team/) at [H2O.ai](https://h2o.ai) have built several world-class Machine Learning, Deep Learning and AI platforms:
|
179 |
+
- #1 open-source machine learning platform for the enterprise [H2O-3](https://github.com/h2oai/h2o-3)
|
180 |
+
- The world's best AutoML (Automatic Machine Learning) with [H2O Driverless AI](https://h2o.ai/platform/ai-cloud/make/h2o-driverless-ai/)
|
181 |
+
- No-Code Deep Learning with [H2O Hydrogen Torch](https://h2o.ai/platform/ai-cloud/make/hydrogen-torch/)
|
182 |
+
- Document Processing with Deep Learning in [Document AI](https://h2o.ai/platform/ai-cloud/make/document-ai/)
|
183 |
+
|
184 |
+
We also built platforms for deployment and monitoring, and for data wrangling and governance:
|
185 |
+
- [H2O MLOps](https://h2o.ai/platform/ai-cloud/operate/h2o-mlops/) to deploy and monitor models at scale
|
186 |
+
- [H2O Feature Store](https://h2o.ai/platform/ai-cloud/make/feature-store/) in collaboration with AT&T
|
187 |
+
- Open-source Low-Code AI App Development Frameworks [Wave](https://wave.h2o.ai/) and [Nitro](https://nitro.h2o.ai/)
|
188 |
+
- Open-source Python [datatable](https://github.com/h2oai/datatable/) (the engine for H2O Driverless AI feature engineering)
|
189 |
+
|
190 |
+
Many of our customers are creating models and deploying them enterprise-wide and at scale in the [H2O AI Cloud](https://h2o.ai/platform/ai-cloud/):
|
191 |
+
- Multi-Cloud or on Premises
|
192 |
+
- [Managed Cloud (SaaS)](https://h2o.ai/platform/ai-cloud/managed)
|
193 |
+
- [Hybrid Cloud](https://h2o.ai/platform/ai-cloud/hybrid)
|
194 |
+
- [AI Appstore](https://docs.h2o.ai/h2o-ai-cloud/)
|
195 |
+
|
196 |
+
We are proud to have over 25 (of the world's 280) [Kaggle Grandmasters](https://h2o.ai/company/team/kaggle-grandmasters/) call H2O home, including three Kaggle Grandmasters who have made it to world #1.
|
197 |
+
|
198 |
+
### Disclaimer
|
199 |
+
|
200 |
+
Please read this disclaimer carefully before using the large language model provided in this repository. Your use of the model signifies your agreement to the following terms and conditions.
|
201 |
+
|
202 |
+
- Biases and Offensiveness: The large language model is trained on a diverse range of internet text data, which may contain biased, racist, offensive, or otherwise inappropriate content. By using this model, you acknowledge and accept that the generated content may sometimes exhibit biases or produce content that is offensive or inappropriate. The developers of this repository do not endorse, support, or promote any such content or viewpoints.
|
203 |
+
- Limitations: The large language model is an AI-based tool and not a human. It may produce incorrect, nonsensical, or irrelevant responses. It is the user's responsibility to critically evaluate the generated content and use it at their discretion.
|
204 |
+
- Use at Your Own Risk: Users of this large language model must assume full responsibility for any consequences that may arise from their use of the tool. The developers and contributors of this repository shall not be held liable for any damages, losses, or harm resulting from the use or misuse of the provided model.
|
205 |
+
- Ethical Considerations: Users are encouraged to use the large language model responsibly and ethically. By using this model, you agree not to use it for purposes that promote hate speech, discrimination, harassment, or any form of illegal or harmful activities.
|
206 |
+
- Reporting Issues: If you encounter any biased, offensive, or otherwise inappropriate content generated by the large language model, please report it to the repository maintainers through the provided channels. Your feedback will help improve the model and mitigate potential issues.
|
207 |
+
- Changes to this Disclaimer: The developers of this repository reserve the right to modify or update this disclaimer at any time without prior notice. It is the user's responsibility to periodically review the disclaimer to stay informed about any changes.
|
208 |
+
|
209 |
+
By using the large language model provided in this repository, you agree to accept and comply with the terms and conditions outlined in this disclaimer. If you do not agree with any part of this disclaimer, you should refrain from using the model and any content generated by it.
|
210 |
+
|
211 |
+
## Star History
|
212 |
+
|
213 |
+
[](https://star-history.com/#h2oai/h2ogpt&Timeline)
|
214 |
|
|
__pycache__/enums.cpython-310.pyc
ADDED
Binary file (2.34 kB). View file
|
|
__pycache__/generate.cpython-310.pyc
ADDED
Binary file (63.9 kB). View file
|
|
__pycache__/gpt4all_llm.cpython-310.pyc
ADDED
Binary file (8.27 kB). View file
|
|
__pycache__/gpt_langchain.cpython-310.pyc
ADDED
Binary file (60.1 kB). View file
|
|
__pycache__/gradio_runner.cpython-310.pyc
ADDED
Binary file (67.4 kB). View file
|
|
__pycache__/gradio_themes.cpython-310.pyc
ADDED
Binary file (6.64 kB). View file
|
|
__pycache__/h2oai_pipeline.cpython-310.pyc
ADDED
Binary file (5.3 kB). View file
|
|
__pycache__/loaders.cpython-310.pyc
ADDED
Binary file (1.66 kB). View file
|
|
__pycache__/prompter.cpython-310.pyc
ADDED
Binary file (15.3 kB). View file
|
|
__pycache__/stopping.cpython-310.pyc
ADDED
Binary file (2.98 kB). View file
|
|
__pycache__/utils.cpython-310.pyc
ADDED
Binary file (28.5 kB). View file
|
|
__pycache__/utils_langchain.cpython-310.pyc
ADDED
Binary file (2.76 kB). View file
|
|
blog/README.md
ADDED
@@ -0,0 +1,81 @@
|
|
|
|
|
|
|
|
|
|
|
|
|
|
|
|
|
|
|
|
|
|
|
|
|
|
|
|
|
|
|
|
|
|
|
|
|
|
|
|
|
|
|
|
|
|
|
|
|
|
|
|
|
|
|
|
|
|
|
|
|
|
|
|
|
|
|
|
|
|
|
|
|
|
|
|
|
|
|
|
|
|
|
|
|
|
|
|
|
|
|
|
|
|
|
|
|
|
|
|
|
|
|
|
|
|
|
|
|
|
|
|
|
|
|
|
|
|
|
|
|
|
|
|
|
|
|
|
|
|
|
|
|
|
|
|
|
|
|
|
|
|
|
|
|
|
|
|
|
|
|
|
|
|
|
|
|
|
|
|
|
|
|
|
|
1 |
+
# Building the World's Best Open-Source Large Language Model: H2O.ai's Journey
|
2 |
+
|
3 |
+
by Arno Candel, PhD, CTO H2O.ai, April 19 2023
|
4 |
+
|
5 |
+
At H2O.ai, we pride ourselves on developing world-class Machine Learning, Deep Learning, and AI platforms. We released H2O, the most widely used open-source distributed and scalable machine learning platform, before XGBoost, TensorFlow and PyTorch existed. H2O.ai is home to over 25 Kaggle grandmasters, including the current #1. In 2017, we used GPUs to create the world's best AutoML in H2O Driverless AI. We have witnessed first-hand how Large Language Models (LLMs) have taken over the world by storm.
|
6 |
+
|
7 |
+
We are proud to announce that we are building h2oGPT, an LLM that not only excels in performance but is also fully open-source and commercially usable, providing a valuable resource for developers, researchers, and organizations worldwide.
|
8 |
+
|
9 |
+
In this blog, we'll explore our journey in building h2oGPT in our effort to further democratize AI.
|
10 |
+
|
11 |
+
## Why Open-Source LLMs?
|
12 |
+
|
13 |
+
While LLMs like OpenAI's ChatGPT/GPT-4, Anthropic's Claude, Microsoft's Bing AI Chat, Google's Bard, and Cohere are powerful and effective, they have certain limitations compared to open-source LLMs:
|
14 |
+
|
15 |
+
1. **Data Privacy and Security**: Using hosted LLMs requires sending data to external servers. This can raise concerns about data privacy, security, and compliance, especially for sensitive information or industries with strict regulations.
|
16 |
+
2. **Dependency and Customization**: Hosted LLMs often limit the extent of customization and control, as users rely on the service provider's infrastructure and predefined models. Open-source LLMs allow users to tailor the models to their specific needs, deploy on their own infrastructure, and even modify the underlying code.
|
17 |
+
3. **Cost and Scalability**: Hosted LLMs usually come with usage fees, which can increase significantly with large-scale applications. Open-source LLMs can be more cost-effective, as users can scale the models on their own infrastructure without incurring additional costs from the service provider.
|
18 |
+
4. **Access and Availability**: Hosted LLMs may be subject to downtime or limited availability, affecting users' access to the models. Open-source LLMs can be deployed on-premises or on private clouds, ensuring uninterrupted access and reducing reliance on external providers.
|
19 |
+
|
20 |
+
Overall, open-source LLMs offer greater flexibility, control, and cost-effectiveness, while addressing data privacy and security concerns. They foster a competitive landscape in the AI industry and empower users to innovate and customize models to suit their specific needs.
|
21 |
+
|
22 |
+
## The H2O.ai LLM Ecosystem
|
23 |
+
|
24 |
+
Our open-source LLM ecosystem currently includes the following components:
|
25 |
+
|
26 |
+
1. **Code, data, and models**: Fully permissive, commercially usable [code](https://github.com/h2oai/h2ogpt), curated fine-tuning [data](https://huggingface.co/h2oai), and fine-tuned [models](https://huggingface.co/h2oai) ranging from 7 to 20 billion parameters.
|
27 |
+
2. **State-of-the-art fine-tuning**: We provide code for highly efficient fine-tuning, including targeted data preparation, prompt engineering, and computational optimizations to fine-tune LLMs with up to 20 billion parameters (even larger models expected soon) in hours on commodity hardware or enterprise servers. Techniques like low-rank approximations (LoRA) and data compression allow computational savings of several orders of magnitude.
|
28 |
+
3. **Chatbot**: We provide code to run a multi-tenant chatbot on GPU servers, with an easily shareable end-point and a Python client API, allowing you to evaluate and compare the performance of fine-tuned LLMs.
|
29 |
+
4. **H2O LLM Studio**: Our no-code LLM fine-tuning framework created by the world's top Kaggle grandmasters makes it even easier to fine-tune and evaluate LLMs.
|
30 |
+
|
31 |
+
Everything we release is based on fully permissive data and models, with all code open-sourced, enabling broader access for businesses and commercial products without legal concerns, thus expanding access to cutting-edge AI while adhering to licensing requirements.
|
32 |
+
|
33 |
+
## Roadmap and Future Plans
|
34 |
+
|
35 |
+
We have an ambitious roadmap for our LLM ecosystem, including:
|
36 |
+
|
37 |
+
1. Integration with downstream applications and low/no-code platforms (H2O Document AI, H2O LLM Studio, etc.)
|
38 |
+
2. Improved validation and benchmarking frameworks of LLMs
|
39 |
+
3. Complementing our chatbot with search and other APIs (LangChain, etc.)
|
40 |
+
4. Contribute to large-scale data cleaning efforts (Open Assistant, Stability AI, RedPajama, etc.)
|
41 |
+
5. High-performance distributed training of larger models on trillion tokens
|
42 |
+
6. High-performance scalable on-premises hosting for high-throughput endpoints
|
43 |
+
7. Improvements in code completion, reasoning, mathematics, factual correctness, hallucinations, and reducing repetitions
|
44 |
+
|
45 |
+
## Getting Started with H2O.ai's LLMs
|
46 |
+
|
47 |
+
You can [Chat with h2oGPT](https://gpt.h2o.ai/) right now!
|
48 |
+
|
49 |
+
https://user-images.githubusercontent.com/6147661/232924684-6c0e2dfb-2f24-4098-848a-c3e4396f29f6.mov
|
50 |
+
|
51 |
+
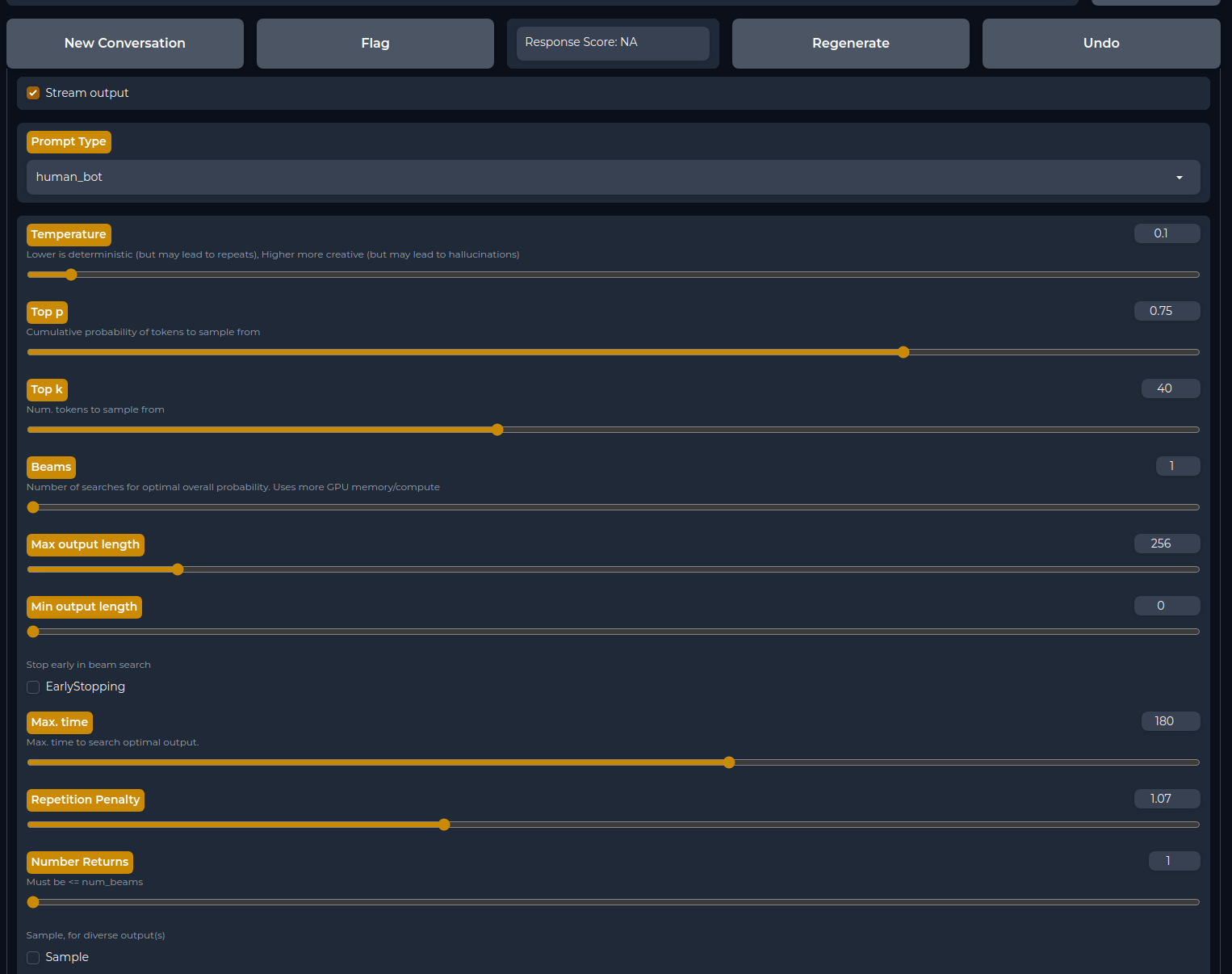
|
52 |
+
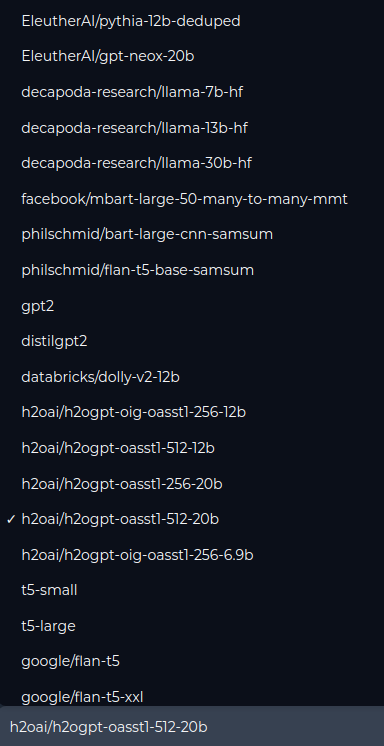
|
53 |
+
|
54 |
+
To start using our LLM as a developer, follow the steps below:
|
55 |
+
|
56 |
+
1. Clone the repository: `git clone https://github.com/h2oai/h2ogpt.git`
|
57 |
+
2. Change to the repository directory: `cd h2ogpt`
|
58 |
+
3. Install the requirements: `pip install -r requirements.txt`
|
59 |
+
4. Run the chatbot: `python generate.py --base_model=h2oai/h2ogpt-oig-oasst1-256-6_9b`
|
60 |
+
5. Open your browser at `http://0.0.0.0:7860` or the public live URL printed by the server.
|
61 |
+
|
62 |
+
For more information, visit [h2oGPT GitHub page](https://github.com/h2oai/h2ogpt), [H2O.ai's Hugging Face page](https://huggingface.co/h2oai) and [H2O LLM Studio GitHub page](https://github.com/h2oai/h2o-llmstudio).
|
63 |
+
|
64 |
+
Join us on this exciting journey as we continue to improve and expand the capabilities of our open-source LLM ecosystem!
|
65 |
+
|
66 |
+
## Acknowledgements
|
67 |
+
|
68 |
+
We appreciate the work by many open-source contributors, especially:
|
69 |
+
|
70 |
+
* [H2O.ai makers](https://h2o.ai/company/team/)
|
71 |
+
* [Alpaca-LoRA](https://github.com/tloen/alpaca-lora/)
|
72 |
+
* [LoRA](https://github.com/microsoft/LoRA/)
|
73 |
+
* [Stanford Alpaca](https://github.com/tatsu-lab/stanford_alpaca/)
|
74 |
+
* [Hugging Face](https://huggingface.co/)
|
75 |
+
* [OpenAssistant](https://open-assistant.io/)
|
76 |
+
* [EleutherAI](https://www.eleuther.ai/)
|
77 |
+
* [LAION](https://laion.ai/blog/oig-dataset/)
|
78 |
+
* [BigScience](https://github.com/bigscience-workshop/bigscience/)
|
79 |
+
* [LLaMa](https://github.com/facebookresearch/llama/)
|
80 |
+
* [StableLM](https://github.com/Stability-AI/StableLM/)
|
81 |
+
* [Vicuna](https://github.com/lm-sys/FastChat/)
|
ci/jenkinsfile
ADDED
@@ -0,0 +1,158 @@
|
|
|
|
|
|
|
|
|
|
|
|
|
|
|
|
|
|
|
|
|
|
|
|
|
|
|
|
|
|
|
|
|
|
|
|
|
|
|
|
|
|
|
|
|
|
|
|
|
|
|
|
|
|
|
|
|
|
|
|
|
|
|
|
|
|
|
|
|
|
|
|
|
|
|
|
|
|
|
|
|
|
|
|
|
|
|
|
|
|
|
|
|
|
|
|
|
|
|
|
|
|
|
|
|
|
|
|
|
|
|
|
|
|
|
|
|
|
|
|
|
|
|
|
|
|
|
|
|
|
|
|
|
|
|
|
|
|
|
|
|
|
|
|
|
|
|
|
|
|
|
|
|
|
|
|
|
|
|
|
|
|
|
|
|
|
|
|
|
|
|
|
|
|
|
|
|
|
|
|
|
|
|
|
|
|
|
|
|
|
|
|
|
|
|
|
|
|
|
|
|
|
|
|
|
|
|
|
|
|
|
|
|
|
|
|
|
|
|
|
|
|
|
|
|
|
|
|
|
|
|
|
|
|
|
|
|
|
|
|
|
|
|
|
|
|
|
|
|
|
|
|
|
|
|
|
|
|
|
|
|
|
|
|
|
|
|
|
|
|
|
|
|
|
|
|
|
|
|
|
|
|
|
|
|
|
|
|
|
|
|
|
|
|
|
|
|
|
|
|
|
|
|
|
|
|
|
|
|
|
|
|
|
|
|
|
|
|
|
1 |
+
#!/usr/bin/groovy
|
2 |
+
|
3 |
+
@Library('test-shared-library@dai_pipeline') _
|
4 |
+
|
5 |
+
import ai.h2o.ci.buildsummary.StagesSummary
|
6 |
+
import groovy.json.JsonOutput
|
7 |
+
|
8 |
+
buildSummary('https://github.com/h2oai/h2ogpt', true)
|
9 |
+
buildSummary.get().addStagesSummary(this, new StagesSummary())
|
10 |
+
|
11 |
+
def ALL_TESTS = [
|
12 |
+
"test_osx": [
|
13 |
+
install_deps: "TRAINING",
|
14 |
+
test_target: "test_imports",
|
15 |
+
node: "osx",
|
16 |
+
test_markers: "not need_tokens and not need_gpu",
|
17 |
+
timeout: 90,
|
18 |
+
use_docker: false,
|
19 |
+
env: ['PYTHON_BINARY=/Users/jenkins/anaconda/envs/h2ogpt-py3.10/bin/python']
|
20 |
+
],
|
21 |
+
"test_all": [
|
22 |
+
install_deps: "TRAINING,WIKI_EXTRA",
|
23 |
+
test_target: "test",
|
24 |
+
test_markers: "not need_tokens and not need_gpu",
|
25 |
+
node: "DAIDEV-GPU || DAIDEV-2GPU",
|
26 |
+
timeout: 90,
|
27 |
+
use_docker: true,
|
28 |
+
env: []
|
29 |
+
],
|
30 |
+
]
|
31 |
+
|
32 |
+
pipeline {
|
33 |
+
agent none
|
34 |
+
parameters {
|
35 |
+
booleanParam(name: 'skipTesting', defaultValue: false, description: 'Skip testing')
|
36 |
+
text(name: "testTargets", defaultValue: "${ALL_TESTS.keySet().join('\n')}", description: "A select set of tests to run")
|
37 |
+
booleanParam(name: 'publish', defaultValue: false, description: 'Upload to HF')
|
38 |
+
}
|
39 |
+
options {
|
40 |
+
ansiColor('xterm')
|
41 |
+
timestamps()
|
42 |
+
}
|
43 |
+
stages {
|
44 |
+
stage('Build') {
|
45 |
+
agent {
|
46 |
+
label "linux && docker"
|
47 |
+
}
|
48 |
+
steps {
|
49 |
+
script {
|
50 |
+
def shortHash = sh(returnStdout: true, script: 'git rev-parse --short HEAD').trim()
|
51 |
+
def commitMsg = sh(returnStdout: true, script: 'git log -1 --pretty=format:"[%an] %s"').trim()
|
52 |
+
currentBuild.displayName = "${env.BUILD_ID} - [${shortHash}]"
|
53 |
+
currentBuild.description = "${commitMsg}"
|
54 |
+
|
55 |
+
sh "make docker_build"
|
56 |
+
docker.image("harbor.h2o.ai/library/python:3.10").inside("--entrypoint='' --security-opt seccomp=unconfined -e USE_WHEEL=1 -e HOME=${WORKSPACE}") {
|
57 |
+
sh "make clean dist"
|
58 |
+
}
|
59 |
+
|
60 |
+
archiveArtifacts allowEmptyArchive: true, artifacts: "dist/h2ogpt-*.whl"
|
61 |
+
stash includes: "dist/h2ogpt-*.whl", name: "wheel_file"
|
62 |
+
}
|
63 |
+
}
|
64 |
+
}
|
65 |
+
|
66 |
+
stage('Tests') {
|
67 |
+
when {
|
68 |
+
anyOf {
|
69 |
+
expression { return !params.skipTesting }
|
70 |
+
}
|
71 |
+
beforeAgent true
|
72 |
+
}
|
73 |
+
agent {
|
74 |
+
label "linux && docker"
|
75 |
+
}
|
76 |
+
steps {
|
77 |
+
script {
|
78 |
+
def testTargets = [:]
|
79 |
+
params.testTargets.split('\n').findAll{ it.contains("test_") }.each { testName ->
|
80 |
+
testTargets[testName] = {
|
81 |
+
node("${ALL_TESTS[testName].node}") {
|
82 |
+
buildSummary.stageWithSummary("${testName}", "${testName}") {
|
83 |
+
buildSummary.setStageUrl("${testName}")
|
84 |
+
timeout(time: ALL_TESTS[testName].timeout, unit: 'MINUTES') {
|
85 |
+
script {
|
86 |
+
try {
|
87 |
+
dir("${testName}") {
|
88 |
+
withEnv(ALL_TESTS[testName].env + ["PYTEST_TEST_NAME=_${testName}", "IS_PR_BUILD=${isPrBranch()}", "USE_WHEEL=1"]) {
|
89 |
+
|
90 |
+
// cleanup and force the use of the installed wheel
|
91 |
+
deleteDir()
|
92 |
+
checkout scm
|
93 |
+
unstash "wheel_file"
|
94 |
+
sh "rm -rf *.py spaces models"
|
95 |
+
|
96 |
+
// pull runtime details
|
97 |
+
def dockerImage = sh(returnStdout: true, script: "make print-DOCKER_TEST_IMAGE").trim()
|
98 |
+
def nvidiaSmiExitCode = sh(returnStdout: false, returnStatus: true, script: "nvidia-smi")
|
99 |
+
// def dockerRuntime = "${nvidiaSmiExitCode}" == "0" ? "--runtime nvidia" : ""
|
100 |
+
def dockerRuntime = "" // TODO: keep until lab machines are upgraded
|
101 |
+
|
102 |
+
if (ALL_TESTS[testName].use_docker) {
|
103 |
+
docker.image("${dockerImage}").inside("--entrypoint='' --security-opt seccomp=unconfined --ulimit core=-1 --init --pid=host -e USE_WHEEL=1 -e HOME=${WORKSPACE}/${testName} ${dockerRuntime}") {
|
104 |
+
sh "nvidia-smi || true"
|
105 |
+
sh "SKIP_MANUAL_TESTS=1 PYTHON_BINARY=/usr/bin/python3.10 make install"
|
106 |
+
sh "SKIP_MANUAL_TESTS=1 PYTHON_BINARY=/usr/bin/python3.10 make install-${ALL_TESTS[testName].install_deps}"
|
107 |
+
sh """DEFAULT_MARKERS="${ALL_TESTS[testName].test_markers}" SKIP_MANUAL_TESTS=1 PYTHON_BINARY=/usr/bin/python3.10 make ${ALL_TESTS[testName].test_target}"""
|
108 |
+
}
|
109 |
+
} else {
|
110 |
+
sh "make venv"
|
111 |
+
sh "SKIP_MANUAL_TESTS=1 PYTHON_BINARY=${WORKSPACE}/${testName}/venv/bin/python make install"
|
112 |
+
sh "SKIP_MANUAL_TESTS=1 PYTHON_BINARY=${WORKSPACE}/${testName}/venv/bin/python make install-${ALL_TESTS[testName].install_deps}"
|
113 |
+
sh """DEFAULT_MARKERS="${ALL_TESTS[testName].test_markers}" SKIP_MANUAL_TESTS=1 PYTHON_BINARY=${WORKSPACE}/${testName}/venv/bin/python make ${ALL_TESTS[testName].test_target}"""
|
114 |
+
}
|
115 |
+
}
|
116 |
+
}
|
117 |
+
} catch (e) {
|
118 |
+
throw e
|
119 |
+
} finally {
|
120 |
+
sh "mv ${testName}/test_report.xml ${testName}/${testName}_report.xml"
|
121 |
+
archiveArtifacts allowEmptyArchive: true, artifacts: "${testName}/${testName}_report.xml"
|
122 |
+
junit testResults: "${testName}/${testName}_report.xml", keepLongStdio: true, allowEmptyResults: true
|
123 |
+
}
|
124 |
+
}
|
125 |
+
}
|
126 |
+
}
|
127 |
+
}
|
128 |
+
}
|
129 |
+
}
|
130 |
+
|
131 |
+
parallel(testTargets)
|
132 |
+
}
|
133 |
+
}
|
134 |
+
}
|
135 |
+
|
136 |
+
stage('Publish') {
|
137 |
+
when {
|
138 |
+
anyOf {
|
139 |
+
expression { return params.publish }
|
140 |
+
}
|
141 |
+
beforeAgent true
|
142 |
+
}
|
143 |
+
agent {
|
144 |
+
label "linux && docker"
|
145 |
+
}
|
146 |
+
steps {
|
147 |
+
script {
|
148 |
+
sh "make IS_PR_BUILD=${isPrBranch()} BUILD_NUMBER=${env.BUILD_ID} BUILD_BASE_NAME=${env.JOB_BASE_NAME} publish"
|
149 |
+
}
|
150 |
+
}
|
151 |
+
}
|
152 |
+
}
|
153 |
+
}
|
154 |
+
|
155 |
+
def isPrBranch() {
|
156 |
+
return (env.CHANGE_BRANCH != null && env.CHANGE_BRANCH != '') ||
|
157 |
+
(env.BRANCH_NAME != null && env.BRANCH_NAME.startsWith("PR-"))
|
158 |
+
}
|
cli.py
ADDED
@@ -0,0 +1,108 @@
|
|
|
|
|
|
|
|
|
|
|
|
|
|
|
|
|
|
|
|
|
|
|
|
|
|
|
|
|
|
|
|
|
|
|
|
|
|
|
|
|
|
|
|
|
|
|
|
|
|
|
|
|
|
|
|
|
|
|
|
|
|
|
|
|
|
|
|
|
|
|
|
|
|
|
|
|
|
|
|
|
|
|
|
|
|
|
|
|
|
|
|
|
|
|
|
|
|
|
|
|
|
|
|
|
|
|
|
|
|
|
|
|
|
|
|
|
|
|
|
|
|
|
|
|
|
|
|
|
|
|
|
|
|
|
|
|
|
|
|
|
|
|
|
|
|
|
|
|
|
|
|
|
|
|
|
|
|
|
|
|
|
|
|
|
|
|
|
|
|
|
|
|
|
|
|
|
|
|
|
|
|
|
|
|
|
|
|
|
|
|
|
|
|
|
|
|
|
|
|
|
|
|
|
|
|
|
|
|
|
|
|
|
|
|
|
|
|
|
1 |
+
import copy
|
2 |
+
import torch
|
3 |
+
|
4 |
+
from generate import eval_func_param_names, get_score_model, get_model, evaluate, check_locals
|
5 |
+
from prompter import non_hf_types
|
6 |
+
from utils import clear_torch_cache, NullContext, get_kwargs
|
7 |
+
|
8 |
+
|
9 |
+
def run_cli( # for local function:
|
10 |
+
base_model=None, lora_weights=None, inference_server=None,
|
11 |
+
debug=None, chat_context=None,
|
12 |
+
examples=None, memory_restriction_level=None,
|
13 |
+
# for get_model:
|
14 |
+
score_model=None, load_8bit=None, load_4bit=None, load_half=None, infer_devices=None, tokenizer_base_model=None,
|
15 |
+
gpu_id=None, local_files_only=None, resume_download=None, use_auth_token=None,
|
16 |
+
trust_remote_code=None, offload_folder=None, compile_model=None,
|
17 |
+
# for some evaluate args
|
18 |
+
stream_output=None, prompt_type=None, prompt_dict=None,
|
19 |
+
temperature=None, top_p=None, top_k=None, num_beams=None,
|
20 |
+
max_new_tokens=None, min_new_tokens=None, early_stopping=None, max_time=None, repetition_penalty=None,
|
21 |
+
num_return_sequences=None, do_sample=None, chat=None,
|
22 |
+
langchain_mode=None, document_choice=None, top_k_docs=None, chunk=None, chunk_size=None,
|
23 |
+
# for evaluate kwargs
|
24 |
+
src_lang=None, tgt_lang=None, concurrency_count=None, save_dir=None, sanitize_bot_response=None,
|
25 |
+
model_state0=None,
|
26 |
+
max_max_new_tokens=None,
|
27 |
+
is_public=None,
|
28 |
+
max_max_time=None,
|
29 |
+
raise_generate_gpu_exceptions=None, load_db_if_exists=None, dbs=None, user_path=None,
|
30 |
+
detect_user_path_changes_every_query=None,
|
31 |
+
use_openai_embedding=None, use_openai_model=None, hf_embedding_model=None,
|
32 |
+
db_type=None, n_jobs=None, first_para=None, text_limit=None, verbose=None, cli=None, reverse_docs=None,
|
33 |
+
use_cache=None,
|
34 |
+
auto_reduce_chunks=None, max_chunks=None, model_lock=None, force_langchain_evaluate=None,
|
35 |
+
model_state_none=None,
|
36 |
+
# unique to this function:
|
37 |
+
cli_loop=None,
|
38 |
+
):
|
39 |
+
check_locals(**locals())
|
40 |
+
|
41 |
+
score_model = "" # FIXME: For now, so user doesn't have to pass
|
42 |
+
n_gpus = torch.cuda.device_count() if torch.cuda.is_available else 0
|
43 |
+
device = 'cpu' if n_gpus == 0 else 'cuda'
|
44 |
+
context_class = NullContext if n_gpus > 1 or n_gpus == 0 else torch.device
|
45 |
+
|
46 |
+
with context_class(device):
|
47 |
+
from functools import partial
|
48 |
+
|
49 |
+
# get score model
|
50 |
+
smodel, stokenizer, sdevice = get_score_model(reward_type=True,
|
51 |
+
**get_kwargs(get_score_model, exclude_names=['reward_type'],
|
52 |
+
**locals()))
|
53 |
+
|
54 |
+
model, tokenizer, device = get_model(reward_type=False,
|
55 |
+
**get_kwargs(get_model, exclude_names=['reward_type'], **locals()))
|
56 |
+
model_dict = dict(base_model=base_model, tokenizer_base_model=tokenizer_base_model, lora_weights=lora_weights,
|
57 |
+
inference_server=inference_server, prompt_type=prompt_type, prompt_dict=prompt_dict)
|
58 |
+
model_state = dict(model=model, tokenizer=tokenizer, device=device)
|
59 |
+
model_state.update(model_dict)
|
60 |
+
my_db_state = [None]
|
61 |
+
fun = partial(evaluate, model_state, my_db_state,
|
62 |
+
**get_kwargs(evaluate, exclude_names=['model_state', 'my_db_state'] + eval_func_param_names,
|
63 |
+
**locals()))
|
64 |
+
|
65 |
+
example1 = examples[-1] # pick reference example
|
66 |
+
all_generations = []
|
67 |
+
while True:
|
68 |
+
clear_torch_cache()
|
69 |
+
instruction = input("\nEnter an instruction: ")
|
70 |
+
if instruction == "exit":
|
71 |
+
break
|
72 |
+
|
73 |
+
eval_vars = copy.deepcopy(example1)
|
74 |
+
eval_vars[eval_func_param_names.index('instruction')] = \
|
75 |
+
eval_vars[eval_func_param_names.index('instruction_nochat')] = instruction
|
76 |
+
eval_vars[eval_func_param_names.index('iinput')] = \
|
77 |
+
eval_vars[eval_func_param_names.index('iinput_nochat')] = '' # no input yet
|
78 |
+
eval_vars[eval_func_param_names.index('context')] = '' # no context yet
|
79 |
+
|
80 |
+
# grab other parameters, like langchain_mode
|
81 |
+
for k in eval_func_param_names:
|
82 |
+
if k in locals():
|
83 |
+
eval_vars[eval_func_param_names.index(k)] = locals()[k]
|
84 |
+
|
85 |
+
gener = fun(*tuple(eval_vars))
|
86 |
+
outr = ''
|
87 |
+
res_old = ''
|
88 |
+
for gen_output in gener:
|
89 |
+
res = gen_output['response']
|
90 |
+
extra = gen_output['sources']
|
91 |
+
if base_model not in non_hf_types or base_model in ['llama']:
|
92 |
+
if not stream_output:
|
93 |
+
print(res)
|
94 |
+
else:
|
95 |
+
# then stream output for gradio that has full output each generation, so need here to show only new chars
|
96 |
+
diff = res[len(res_old):]
|
97 |
+
print(diff, end='', flush=True)
|
98 |
+
res_old = res
|
99 |
+
outr = res # don't accumulate
|
100 |
+
else:
|
101 |
+
outr += res # just is one thing
|
102 |
+
if extra:
|
103 |
+
# show sources at end after model itself had streamed to std rest of response
|
104 |
+
print(extra, flush=True)
|
105 |
+
all_generations.append(outr + '\n')
|
106 |
+
if not cli_loop:
|
107 |
+
break
|
108 |
+
return all_generations
|
client/.gitignore
ADDED
@@ -0,0 +1,164 @@
|
|
|
|
|
|
|
|
|
|
|
|
|
|
|
|
|
|
|
|
|
|
|
|
|
|
|
|
|
|
|
|
|
|
|
|
|
|
|
|
|
|
|
|
|
|
|
|
|
|
|
|
|
|
|
|
|
|
|
|
|
|
|
|
|
|
|
|
|
|
|
|
|
|
|
|
|
|
|
|
|
|
|
|
|
|
|
|
|
|
|
|
|
|
|
|
|
|
|
|
|
|
|
|
|
|
|
|
|
|
|
|
|
|
|
|
|
|
|
|
|
|
|
|
|
|
|
|
|
|
|
|
|
|
|
|
|
|
|
|
|
|
|
|
|
|
|
|
|
|
|
|
|
|
|
|
|
|
|
|
|
|
|
|
|
|
|
|
|
|
|
|
|
|
|
|
|
|
|
|
|
|
|
|
|
|
|
|
|
|
|
|
|
|
|
|
|
|
|
|
|
|
|
|
|
|
|
|
|
|
|
|
|
|
|
|
|
|
|
|
|
|
|
|
|
|
|
|
|
|
|
|
|
|
|
|
|
|
|
|
|
|
|
|
|
|
|
|
|
|
|
|
|
|
|
|
|
|
|
|
|
|
|
|
|
|
|
|
|
|
|
|
|
|
|
|
|
|
|
|
|
|
|
|
|
|
|
|
|
|
|
|
|
|
|
|
|
|
|
|
|
|
|
|
|
|
|
|
|
|
|
|
|
|
|
|
|
|
|
|
|
|
|
|
|
|
|
|
|
|
|
1 |
+
### Generated files ###
|
2 |
+
enums.py
|
3 |
+
|
4 |
+
### Python template
|
5 |
+
# Byte-compiled / optimized / DLL files
|
6 |
+
__pycache__/
|
7 |
+
*.py[cod]
|
8 |
+
*$py.class
|
9 |
+
|
10 |
+
# C extensions
|
11 |
+
*.so
|
12 |
+
|
13 |
+
# Distribution / packaging
|
14 |
+
.Python
|
15 |
+
build/
|
16 |
+
develop-eggs/
|
17 |
+
dist/
|
18 |
+
downloads/
|
19 |
+
eggs/
|
20 |
+
.eggs/
|
21 |
+
lib/
|
22 |
+
lib64/
|
23 |
+
parts/
|
24 |
+
sdist/
|
25 |
+
var/
|
26 |
+
wheels/
|
27 |
+
share/python-wheels/
|
28 |
+
*.egg-info/
|
29 |
+
.installed.cfg
|
30 |
+
*.egg
|
31 |
+
MANIFEST
|
32 |
+
|
33 |
+
# PyInstaller
|
34 |
+
# Usually these files are written by a python script from a template
|
35 |
+
# before PyInstaller builds the exe, so as to inject date/other infos into it.
|
36 |
+
*.manifest
|
37 |
+
*.spec
|
38 |
+
|
39 |
+
# Installer logs
|
40 |
+
pip-log.txt
|
41 |
+
pip-delete-this-directory.txt
|
42 |
+
|
43 |
+
# Unit test / coverage reports
|
44 |
+
htmlcov/
|
45 |
+
.tox/
|
46 |
+
.nox/
|
47 |
+
.coverage
|
48 |
+
.coverage.*
|
49 |
+
.cache
|
50 |
+
nosetests.xml
|
51 |
+
coverage.xml
|
52 |
+
*.cover
|
53 |
+
*.py,cover
|
54 |
+
.hypothesis/
|
55 |
+
.pytest_cache/
|
56 |
+
cover/
|
57 |
+
|
58 |
+
# Translations
|
59 |
+
*.mo
|
60 |
+
*.pot
|
61 |
+
|
62 |
+
# Django stuff:
|
63 |
+
*.log
|
64 |
+
local_settings.py
|
65 |
+
db.sqlite3
|
66 |
+
db.sqlite3-journal
|
67 |
+
|
68 |
+
# Flask stuff:
|
69 |
+
instance/
|
70 |
+
.webassets-cache
|
71 |
+
|
72 |
+
# Scrapy stuff:
|
73 |
+
.scrapy
|
74 |
+
|
75 |
+
# Sphinx documentation
|
76 |
+
docs/_build/
|
77 |
+
|
78 |
+
# PyBuilder
|
79 |
+
.pybuilder/
|
80 |
+
target/
|
81 |
+
|
82 |
+
# Jupyter Notebook
|
83 |
+
.ipynb_checkpoints
|
84 |
+
|
85 |
+
# IPython
|
86 |
+
profile_default/
|
87 |
+
ipython_config.py
|
88 |
+
|
89 |
+
# pyenv
|
90 |
+
# For a library or package, you might want to ignore these files since the code is
|
91 |
+
# intended to run in multiple environments; otherwise, check them in:
|
92 |
+
# .python-version
|
93 |
+
|
94 |
+
# pipenv
|
95 |
+
# According to pypa/pipenv#598, it is recommended to include Pipfile.lock in version control.
|
96 |
+
# However, in case of collaboration, if having platform-specific dependencies or dependencies
|
97 |
+
# having no cross-platform support, pipenv may install dependencies that don't work, or not
|
98 |
+
# install all needed dependencies.
|
99 |
+
#Pipfile.lock
|
100 |
+
|
101 |
+
# poetry
|
102 |
+
# Similar to Pipfile.lock, it is generally recommended to include poetry.lock in version control.
|
103 |
+
# This is especially recommended for binary packages to ensure reproducibility, and is more
|
104 |
+
# commonly ignored for libraries.
|
105 |
+
# https://python-poetry.org/docs/basic-usage/#commit-your-poetrylock-file-to-version-control
|
106 |
+
#poetry.lock
|
107 |
+
|
108 |
+
# pdm
|
109 |
+
# Similar to Pipfile.lock, it is generally recommended to include pdm.lock in version control.
|
110 |
+
#pdm.lock
|
111 |
+
# pdm stores project-wide configurations in .pdm.toml, but it is recommended to not include it
|
112 |
+
# in version control.
|
113 |
+
# https://pdm.fming.dev/#use-with-ide
|
114 |
+
.pdm.toml
|
115 |
+
|
116 |
+
# PEP 582; used by e.g. github.com/David-OConnor/pyflow and github.com/pdm-project/pdm
|
117 |
+
__pypackages__/
|
118 |
+
|
119 |
+
# Celery stuff
|
120 |
+
celerybeat-schedule
|
121 |
+
celerybeat.pid
|
122 |
+
|
123 |
+
# SageMath parsed files
|
124 |
+
*.sage.py
|
125 |
+
|
126 |
+
# Environments
|
127 |
+
.env
|
128 |
+
.venv
|
129 |
+
env/
|
130 |
+
venv/
|
131 |
+
ENV/
|
132 |
+
env.bak/
|
133 |
+
venv.bak/
|
134 |
+
|
135 |
+
# Spyder project settings
|
136 |
+
.spyderproject
|
137 |
+
.spyproject
|
138 |
+
|
139 |
+
# Rope project settings
|
140 |
+
.ropeproject
|
141 |
+
|
142 |
+
# mkdocs documentation
|
143 |
+
/site
|
144 |
+
|
145 |
+
# mypy
|
146 |
+
.mypy_cache/
|
147 |
+
.dmypy.json
|
148 |
+
dmypy.json
|
149 |
+
|
150 |
+
# Pyre type checker
|
151 |
+
.pyre/
|
152 |
+
|
153 |
+
# pytype static type analyzer
|
154 |
+
.pytype/
|
155 |
+
|
156 |
+
# Cython debug symbols
|
157 |
+
cython_debug/
|
158 |
+
|
159 |
+
# PyCharm
|
160 |
+
# JetBrains specific template is maintained in a separate JetBrains.gitignore that can
|
161 |
+
# be found at https://github.com/github/gitignore/blob/main/Global/JetBrains.gitignore
|
162 |
+
# and can be added to the global gitignore or merged into this file. For a more nuclear
|
163 |
+
# option (not recommended) you can uncomment the following to ignore the entire idea folder.
|
164 |
+
.idea/
|
client/Makefile
ADDED
@@ -0,0 +1,25 @@
|
|
|
|
|
|
|
|
|
|
|
|
|
|
|
|
|
|
|
|
|
|
|
|
|
|
|
|
|
|
|
|
|
|
|
|
|
|
|
|
|
|
|
|
|
|
|
|
|
|
|
|
|
1 |
+
PACKAGE_NAME := $(firstword $(shell poetry version))
|
2 |
+
PACKAGE_DIR := $(subst -,_,$(PACKAGE_NAME))
|
3 |
+
PACKAGE_VERSION := $(shell poetry version --short)
|
4 |
+
|
5 |
+
generate_sources:
|
6 |
+
cp -f ./../enums.py "$(PACKAGE_DIR)/enums.py"
|
7 |
+
|
8 |
+
.PHONY: setup
|
9 |
+
setup:
|
10 |
+
poetry install
|
11 |
+
|
12 |
+
.PHONY: lint
|
13 |
+
lint: generate_sources
|
14 |
+
poetry run black .
|
15 |
+
poetry run isort .
|
16 |
+
poetry run flake8 "$(PACKAGE_DIR)" "tests" || true
|
17 |
+
poetry run mypy --show-error-codes --pretty .
|
18 |
+
|
19 |
+
.PHONY: test
|
20 |
+
test: generate_sources
|
21 |
+
poetry run pytest
|
22 |
+
|
23 |
+
.PHONY: build
|
24 |
+
build: generate_sources
|
25 |
+
poetry build
|
client/README.md
ADDED
@@ -0,0 +1,41 @@
|
|
|
|
|
|
|
|
|
|
|
|
|
|
|
|
|
|
|
|
|
|
|
|
|
|
|
|
|
|
|
|
|
|
|
|
|
|
|
|
|
|
|
|
|
|
|
|
|
|
|
|
|
|
|
|
|
|
|
|
|
|
|
|
|
|
|
|
|
|
|
|
|
|
|
|
|
|
|
|
|
|
|
|
|
1 |
+
# h2oGPT Client
|
2 |
+
A Python thin-client for h2oGPT.
|
3 |
+
|
4 |
+
## Installation
|
5 |
+
### Prerequisites
|
6 |
+
- Python 3.8+
|
7 |
+
- [Poetry](https://python-poetry.org/docs/#installation) - A dependency management and packaging tool for Python
|
8 |
+
|
9 |
+
### Setup environment
|
10 |
+
```shell
|
11 |
+
cd client
|
12 |
+
pip install poetry==1.5.1
|
13 |
+
make setup
|
14 |
+
make lint
|
15 |
+
make build
|
16 |
+
# install (choose version if multiple builds in dist directory)
|
17 |
+
pip install dist/h2ogpt_client-*-py3-none-any.whl
|
18 |
+
# test
|
19 |
+
cd ..
|
20 |
+
pytest -s -v --forked client
|
21 |
+
```
|
22 |
+
|
23 |
+
## Usage
|
24 |
+
```python
|
25 |
+
from h2ogpt_client import Client
|
26 |
+
|
27 |
+
client = Client("http://0.0.0.0:7860")
|
28 |
+
|
29 |
+
# text completion
|
30 |
+
response = client.text_completion.create("Hello world")
|
31 |
+
response = await client.text_completion.create_async("Hello world")
|
32 |
+
|
33 |
+
# chat completion
|
34 |
+
chat_context = client.chat_completion.create()
|
35 |
+
chat = chat_context.chat("Hey!")
|
36 |
+
print(chat["user"]) # prints user prompt, i.e. "Hey!"
|
37 |
+
print(chat["gpt"]) # prints reply of the h2oGPT
|
38 |
+
chat_history = chat_context.chat_history()
|
39 |
+
```
|
40 |
+
:warning: **Note**: Client APIs are still evolving. Hence, APIs can be changed without prior warnings.
|
41 |
+
|
client/h2ogpt_client/__init__.py
ADDED
@@ -0,0 +1,4 @@
|
|
|
|
|
|
|
|
|
|
|
1 |
+
from h2ogpt_client.core import Client
|
2 |
+
from h2ogpt_client.enums import LangChainMode, PromptType
|
3 |
+
|
4 |
+
__all__ = ["Client", "PromptType", "LangChainMode"]
|
client/h2ogpt_client/core.py
ADDED
@@ -0,0 +1,314 @@
|
|
|
|
|
|
|
|
|
|
|
|
|
|
|
|
|
|
|
|
|
|
|
|
|
|
|
|
|
|
|
|
|
|
|
|
|
|
|
|
|
|
|
|
|
|
|
|
|
|
|
|
|
|
|
|
|
|
|
|
|
|
|
|
|
|
|
|
|
|
|
|
|
|
|
|
|
|
|
|
|
|
|
|
|
|
|
|
|
|
|
|
|
|
|
|
|
|
|
|
|
|
|
|
|
|
|
|
|
|
|
|
|
|
|
|
|
|
|
|
|
|
|
|
|
|
|
|
|
|
|
|
|
|
|
|
|
|
|
|
|
|
|
|
|
|
|
|
|
|
|
|
|
|
|
|
|
|
|
|
|
|
|
|
|
|
|
|
|
|
|
|
|
|
|
|
|
|
|
|
|
|
|
|
|
|
|
|
|
|
|
|
|
|
|
|
|
|
|
|
|
|
|
|
|
|
|
|
|
|
|
|
|
|
|
|
|
|
|
|
|
|
|
|
|
|
|
|
|
|
|
|
|
|
|
|
|
|
|
|
|
|
|
|
|
|
|
|
|
|
|
|
|
|
|
|
|
|
|
|
|
|
|
|
|
|
|
|
|
|
|
|
|
|
|
|
|
|
|
|
|
|
|
|
|
|
|
|
|
|
|
|
|
|
|
|
|
|
|
|
|
|
|
|
|
|
|
|
|
|
|
|
|
|
|
|
|
|
|
|
|
|
|
|
|
|
|
|
|
|
|
|
|
|
|
|
|
|
|
|
|
|
|
|
|
|
|
|
|
|
|
|
|
|
|
|
|
|
|
|
|
|
|
|
|
|
|
|
|
|
|
|
|
|
|
|
|
|
|
|
|
|
|
|
|
|
|
|
|
|
|
|
|
|
|
|
|
|
|
|
|
|
|
|
|
|
|
|
|
|
|
|
|
|
|
|
|
|
|
|
|
|
|
|
|
|
|
|
|
|
|
|
|
|
|
|
|
|
|
|
|
|
|
|
|
|
|
|
|
|
|
|
|
|
|
|
|
|
|
|
|
|
|
|
|
|
|
|
|
|
|
|
|
|
|
|
|
|
|
|
|
|
|
|
|
|
|
|
|
|
|
|
|
|
|
|
|
|
|
|
|
|
|
|
|
|
|
|
|
|
|
|
|
|
|
|
|
|
|
|
|
|
|
|
|
|
|
|
|
|
|
|
|
|
|
|
|
|
|
|
|
|
|
|
|
|
|
|
|
|
|
|
|
|
|
|
|
|
|
|
|
|
|
|
|
|
|
|
|
|
|
|
|
|
|
|
|
|
|
|
|
|
|
|
|
|
|
|
|
|
|
|
|
|
|
|
|
|
|
|
|
|
|
|
|
|
|
|
|
|
|
|
|
|
|
|
|
|
|
|
|
|
|
|
|
|
|
|
|
|
|
1 |
+
import asyncio
|
2 |
+
import collections
|
3 |
+
from typing import Any, Dict, List, Optional, OrderedDict, Tuple
|
4 |
+
|
5 |
+
import gradio_client # type: ignore
|
6 |
+
|
7 |
+
from h2ogpt_client import enums
|
8 |
+
|
9 |
+
|
10 |
+
class Client:
|
11 |
+
def __init__(self, server_url: str, huggingface_token: Optional[str] = None):
|
12 |
+
self._client = gradio_client.Client(
|
13 |
+
src=server_url, hf_token=huggingface_token, serialize=False, verbose=False
|
14 |
+
)
|
15 |
+
self._text_completion = TextCompletion(self)
|
16 |
+
self._chat_completion = ChatCompletion(self)
|
17 |
+
|
18 |
+
@property
|
19 |
+
def text_completion(self) -> "TextCompletion":
|
20 |
+
return self._text_completion
|
21 |
+
|
22 |
+
@property
|
23 |
+
def chat_completion(self) -> "ChatCompletion":
|
24 |
+
return self._chat_completion
|
25 |
+
|
26 |
+
def _predict(self, *args, api_name: str) -> Any:
|
27 |
+
return self._client.submit(*args, api_name=api_name).result()
|
28 |
+
|
29 |
+
async def _predict_async(self, *args, api_name: str) -> str:
|
30 |
+
return await asyncio.wrap_future(self._client.submit(*args, api_name=api_name))
|
31 |
+
|
32 |
+
|
33 |
+
class TextCompletion:
|
34 |
+
"""Text completion"""
|
35 |
+
|
36 |
+
def __init__(self, client: Client):
|
37 |
+
self._client = client
|
38 |
+
|
39 |
+
def create(
|
40 |
+
self,
|
41 |
+
prompt: str,
|
42 |
+
prompt_type: enums.PromptType = enums.PromptType.plain,
|
43 |
+
input_context_for_instruction: str = "",
|
44 |
+
enable_sampler=False,
|
45 |
+
temperature: float = 1.0,
|
46 |
+
top_p: float = 1.0,
|
47 |
+
top_k: int = 40,
|
48 |
+
beams: float = 1.0,
|
49 |
+
early_stopping: bool = False,
|
50 |
+
min_output_length: int = 0,
|
51 |
+
max_output_length: int = 128,
|
52 |
+
max_time: int = 180,
|
53 |
+
repetition_penalty: float = 1.07,
|
54 |
+
number_returns: int = 1,
|
55 |
+
system_pre_context: str = "",
|
56 |
+
langchain_mode: enums.LangChainMode = enums.LangChainMode.DISABLED,
|
57 |
+
) -> str:
|
58 |
+
"""
|
59 |
+
Creates a new text completion.
|
60 |
+
|
61 |
+
:param prompt: text prompt to generate completions for
|
62 |
+
:param prompt_type: type of the prompt
|
63 |
+
:param input_context_for_instruction: input context for instruction
|
64 |
+
:param enable_sampler: enable or disable the sampler, required for use of
|
65 |
+
temperature, top_p, top_k
|
66 |
+
:param temperature: What sampling temperature to use, between 0 and 3.
|
67 |
+
Lower values will make it more focused and deterministic, but may lead
|
68 |
+
to repeat. Higher values will make the output more creative, but may
|
69 |
+
lead to hallucinations.
|
70 |
+
:param top_p: cumulative probability of tokens to sample from
|
71 |
+
:param top_k: number of tokens to sample from
|
72 |
+
:param beams: Number of searches for optimal overall probability.
|
73 |
+
Higher values uses more GPU memory and compute.
|
74 |
+
:param early_stopping: whether to stop early or not in beam search
|
75 |
+
:param min_output_length: minimum output length
|
76 |
+
:param max_output_length: maximum output length
|
77 |
+
:param max_time: maximum time to search optimal output
|
78 |
+
:param repetition_penalty: penalty for repetition
|
79 |
+
:param number_returns:
|
80 |
+
:param system_pre_context: directly pre-appended without prompt processing
|
81 |
+
:param langchain_mode: LangChain mode
|
82 |
+
:return: response from the model
|
83 |
+
"""
|
84 |
+
# Not exposed parameters.
|
85 |
+
instruction = "" # empty when chat_mode is False
|
86 |
+
input = "" # only chat_mode is True
|
87 |
+
stream_output = False
|
88 |
+
prompt_dict = "" # empty as prompt_type cannot be 'custom'
|
89 |
+
chat_mode = False
|
90 |
+
langchain_top_k_docs = 4 # number of document chunks; not public
|
91 |
+
langchain_enable_chunk = True # whether to chunk documents; not public
|
92 |
+
langchain_chunk_size = 512 # chunk size for document chunking; not public
|
93 |
+
langchain_document_choice = ["All"]
|
94 |
+
|
95 |
+
return self._client._predict(
|
96 |
+
instruction,
|
97 |
+
input,
|
98 |
+
system_pre_context,
|
99 |
+
stream_output,
|
100 |
+
prompt_type.value,
|
101 |
+
prompt_dict,
|
102 |
+
temperature,
|
103 |
+
top_p,
|
104 |
+
top_k,
|
105 |
+
beams,
|
106 |
+
max_output_length,
|
107 |
+
min_output_length,
|
108 |
+
early_stopping,
|
109 |
+
max_time,
|
110 |
+
repetition_penalty,
|
111 |
+
number_returns,
|
112 |
+
enable_sampler,
|
113 |
+
chat_mode,
|
114 |
+
prompt,
|
115 |
+
input_context_for_instruction,
|
116 |
+
langchain_mode.value,
|
117 |
+
langchain_top_k_docs,
|
118 |
+
langchain_enable_chunk,
|
119 |
+
langchain_chunk_size,
|
120 |
+
langchain_document_choice,
|
121 |
+
api_name="/submit_nochat",
|
122 |
+
)
|
123 |
+
|
124 |
+
async def create_async(
|
125 |
+
self,
|
126 |
+
prompt: str,
|
127 |
+
prompt_type: enums.PromptType = enums.PromptType.plain,
|
128 |
+
input_context_for_instruction: str = "",
|
129 |
+
enable_sampler=False,
|
130 |
+
temperature: float = 1.0,
|
131 |
+
top_p: float = 1.0,
|
132 |
+
top_k: int = 40,
|
133 |
+
beams: float = 1.0,
|
134 |
+
early_stopping: bool = False,
|
135 |
+
min_output_length: int = 0,
|
136 |
+
max_output_length: int = 128,
|
137 |
+
max_time: int = 180,
|
138 |
+
repetition_penalty: float = 1.07,
|
139 |
+
number_returns: int = 1,
|
140 |
+
system_pre_context: str = "",
|
141 |
+
langchain_mode: enums.LangChainMode = enums.LangChainMode.DISABLED,
|
142 |
+
) -> str:
|
143 |
+
"""
|
144 |
+
Creates a new text completion asynchronously.
|
145 |
+
|
146 |
+
:param prompt: text prompt to generate completions for
|
147 |
+
:param prompt_type: type of the prompt
|
148 |
+
:param input_context_for_instruction: input context for instruction
|
149 |
+
:param enable_sampler: enable or disable the sampler, required for use of
|
150 |
+
temperature, top_p, top_k
|
151 |
+
:param temperature: What sampling temperature to use, between 0 and 3.
|
152 |
+
Lower values will make it more focused and deterministic, but may lead
|
153 |
+
to repeat. Higher values will make the output more creative, but may
|
154 |
+
lead to hallucinations.
|
155 |
+
:param top_p: cumulative probability of tokens to sample from
|
156 |
+
:param top_k: number of tokens to sample from
|
157 |
+
:param beams: Number of searches for optimal overall probability.
|
158 |
+
Higher values uses more GPU memory and compute.
|
159 |
+
:param early_stopping: whether to stop early or not in beam search
|
160 |
+
:param min_output_length: minimum output length
|
161 |
+
:param max_output_length: maximum output length
|
162 |
+
:param max_time: maximum time to search optimal output
|
163 |
+
:param repetition_penalty: penalty for repetition
|
164 |
+
:param number_returns:
|
165 |
+
:param system_pre_context: directly pre-appended without prompt processing
|
166 |
+
:param langchain_mode: LangChain mode
|
167 |
+
:return: response from the model
|
168 |
+
"""
|
169 |
+
# Not exposed parameters.
|
170 |
+
instruction = "" # empty when chat_mode is False
|
171 |
+
input = "" # only chat_mode is True
|
172 |
+
stream_output = False
|
173 |
+
prompt_dict = "" # empty as prompt_type cannot be 'custom'
|
174 |
+
chat_mode = False
|
175 |
+
langchain_top_k_docs = 4 # number of document chunks; not public
|
176 |
+
langchain_enable_chunk = True # whether to chunk documents; not public
|
177 |
+
langchain_chunk_size = 512 # chunk size for document chunking; not public
|
178 |
+
langchain_document_choice = ["All"] # not public
|
179 |
+
|
180 |
+
return await self._client._predict_async(
|
181 |
+
instruction,
|
182 |
+
input,
|
183 |
+
system_pre_context,
|
184 |
+
stream_output,
|
185 |
+
prompt_type.value,
|
186 |
+
prompt_dict,
|
187 |
+
temperature,
|
188 |
+
top_p,
|
189 |
+
top_k,
|
190 |
+
beams,
|
191 |
+
max_output_length,
|
192 |
+
min_output_length,
|
193 |
+
early_stopping,
|
194 |
+
max_time,
|
195 |
+
repetition_penalty,
|
196 |
+
number_returns,
|
197 |
+
enable_sampler,
|
198 |
+
chat_mode,
|
199 |
+
prompt,
|
200 |
+
input_context_for_instruction,
|
201 |
+
langchain_mode.value,
|
202 |
+
langchain_top_k_docs,
|
203 |
+
langchain_enable_chunk,
|
204 |
+
langchain_chunk_size,
|
205 |
+
langchain_document_choice,
|
206 |
+
api_name="/submit_nochat",
|
207 |
+
)
|
208 |
+
|
209 |
+
|
210 |
+
class ChatCompletion:
|
211 |
+
"""Chat completion"""
|
212 |
+
|
213 |
+
def __init__(self, client: Client):
|
214 |
+
self._client = client
|
215 |
+
|
216 |
+
def create(
|
217 |
+
self,
|
218 |
+
prompt_type: enums.PromptType = enums.PromptType.plain,
|
219 |
+
input_context_for_instruction: str = "",
|
220 |
+
enable_sampler=False,
|
221 |
+
temperature: float = 1.0,
|
222 |
+
top_p: float = 1.0,
|
223 |
+
top_k: int = 40,
|
224 |
+
beams: float = 1.0,
|
225 |
+
early_stopping: bool = False,
|
226 |
+
min_output_length: int = 0,
|
227 |
+
max_output_length: int = 128,
|
228 |
+
max_time: int = 180,
|
229 |
+
repetition_penalty: float = 1.07,
|
230 |
+
number_returns: int = 1,
|
231 |
+
system_pre_context: str = "",
|
232 |
+
langchain_mode: enums.LangChainMode = enums.LangChainMode.DISABLED,
|
233 |
+
) -> "ChatContext":
|
234 |
+
"""
|
235 |
+
Creates a new text completion asynchronously.
|
236 |
+
|
237 |
+
:param prompt_type: type of the prompt
|
238 |
+
:param input_context_for_instruction: input context for instruction
|
239 |
+
:param enable_sampler: enable or disable the sampler, required for use of
|
240 |
+
temperature, top_p, top_k
|
241 |
+
:param temperature: What sampling temperature to use, between 0 and 3.
|
242 |
+
Lower values will make it more focused and deterministic, but may lead
|
243 |
+
to repeat. Higher values will make the output more creative, but may
|
244 |
+
lead to hallucinations.
|
245 |
+
:param top_p: cumulative probability of tokens to sample from
|
246 |
+
:param top_k: number of tokens to sample from
|
247 |
+
:param beams: Number of searches for optimal overall probability.
|
248 |
+
Higher values uses more GPU memory and compute.
|
249 |
+
:param early_stopping: whether to stop early or not in beam search
|
250 |
+
:param min_output_length: minimum output length
|
251 |
+
:param max_output_length: maximum output length
|
252 |
+
:param max_time: maximum time to search optimal output
|
253 |
+
:param repetition_penalty: penalty for repetition
|
254 |
+
:param number_returns:
|
255 |
+
:param system_pre_context: directly pre-appended without prompt processing
|
256 |
+
:param langchain_mode: LangChain mode
|
257 |
+
:return: a chat context with given parameters
|
258 |
+
"""
|
259 |
+
kwargs = collections.OrderedDict(
|
260 |
+
instruction=None, # future prompts
|
261 |
+
input="", # ??
|
262 |
+
system_pre_context=system_pre_context,
|
263 |
+
stream_output=False,
|
264 |
+
prompt_type=prompt_type.value,
|
265 |
+
prompt_dict="", # empty as prompt_type cannot be 'custom'
|
266 |
+
temperature=temperature,
|
267 |
+
top_p=top_p,
|
268 |
+
top_k=top_k,
|
269 |
+
beams=beams,
|
270 |
+
max_output_length=max_output_length,
|
271 |
+
min_output_length=min_output_length,
|
272 |
+
early_stopping=early_stopping,
|
273 |
+
max_time=max_time,
|
274 |
+
repetition_penalty=repetition_penalty,
|
275 |
+
number_returns=number_returns,
|
276 |
+
enable_sampler=enable_sampler,
|
277 |
+
chat_mode=True,
|
278 |
+
instruction_nochat="", # empty when chat_mode is True
|
279 |
+
input_context_for_instruction=input_context_for_instruction,
|
280 |
+
langchain_mode=langchain_mode.value,
|
281 |
+
langchain_top_k_docs=4, # number of document chunks; not public
|
282 |
+
langchain_enable_chunk=True, # whether to chunk documents; not public
|
283 |
+
langchain_chunk_size=512, # chunk size for document chunking; not public
|
284 |
+
langchain_document_choice=["All"], # not public
|
285 |
+
chatbot=[], # chat history
|
286 |
+
)
|
287 |
+
return ChatContext(self._client, kwargs)
|
288 |
+
|
289 |
+
|
290 |
+
class ChatContext:
|
291 |
+
""" "Chat context"""
|
292 |
+
|
293 |
+
def __init__(self, client: Client, kwargs: OrderedDict[str, Any]):
|
294 |
+
self._client = client
|
295 |
+
self._kwargs = kwargs
|
296 |
+
|
297 |
+
def chat(self, prompt: str) -> Dict[str, str]:
|
298 |
+
"""
|
299 |
+
Chat with the GPT.
|
300 |
+
|
301 |
+
:param prompt: text prompt to generate completions for
|
302 |
+
:returns chat reply
|
303 |
+
"""
|
304 |
+
self._kwargs["instruction"] = prompt
|
305 |
+
self._kwargs["chatbot"] += [[prompt, None]]
|
306 |
+
response: Tuple[List[List[str]], str] = self._client._predict(
|
307 |
+
*self._kwargs.values(), api_name="/instruction_bot"
|
308 |
+
)
|
309 |
+
self._kwargs["chatbot"][-1][1] = response[0][-1][1]
|
310 |
+
return {"user": response[0][-1][0], "gpt": response[0][-1][1]}
|
311 |
+
|
312 |
+
def chat_history(self) -> List[Dict[str, str]]:
|
313 |
+
"""Returns the full chat history."""
|
314 |
+
return [{"user": i[0], "gpt": i[1]} for i in self._kwargs["chatbot"]]
|
client/poetry.lock
ADDED
@@ -0,0 +1,876 @@
|
|
|
|
|
|
|
|
|
|
|
|
|
|
|
|
|
|
|
|
|
|
|
|
|
|
|
|
|
|
|
|
|
|
|
|
|
|
|
|
|
|
|
|
|
|
|
|
|
|
|
|
|
|
|
|
|
|
|
|
|
|
|
|
|
|
|
|
|
|
|
|
|
|
|
|
|
|
|
|
|
|
|
|
|
|
|
|
|
|
|
|
|
|
|
|
|
|
|
|
|
|
|
|
|
|
|
|
|
|
|
|
|
|
|
|
|
|
|
|
|
|
|
|
|
|
|
|
|
|
|
|
|
|
|
|
|
|
|
|
|
|
|
|
|
|
|
|
|
|
|
|
|
|
|
|
|
|
|
|
|
|
|
|
|
|
|
|
|
|
|
|
|
|
|
|
|
|
|
|
|
|
|
|
|
|
|
|
|
|
|
|
|
|
|
|
|
|
|
|
|
|
|
|
|
|
|
|
|
|
|
|
|
|
|
|
|
|
|
|
|
|
|
|
|
|
|
|
|
|
|
|
|
|
|
|
|
|
|
|
|
|
|
|
|
|
|
|
|
|
|
|
|
|
|
|
|
|
|
|
|
|
|
|
|
|
|
|
|
|
|
|
|
|
|
|
|
|
|
|
|
|
|
|
|
|
|
|
|
|
|
|
|
|
|
|
|
|
|
|
|
|
|
|
|
|
|
|
|
|
|
|
|
|
|
|
|
|
|
|
|
|
|
|
|
|
|
|
|
|
|
|
|
|
|
|
|
|
|
|
|
|
|
|
|
|
|
|
|
|
|
|
|
|
|
|
|
|
|
|
|
|
|
|
|
|
|
|
|
|
|
|
|
|
|
|
|
|
|
|
|
|
|
|
|
|
|
|
|
|
|
|
|
|
|
|
|
|
|
|
|
|
|
|
|
|
|
|
|
|
|
|
|
|
|
|
|
|
|
|
|
|
|
|
|
|
|
|
|
|
|
|
|
|
|
|
|
|
|
|
|
|
|
|
|
|
|
|
|
|
|
|
|
|
|
|
|
|
|
|
|
|
|
|
|
|
|
|
|
|
|
|
|
|
|
|
|
|
|
|
|
|
|
|
|
|
|
|
|
|
|
|
|
|
|
|
|
|
|
|
|
|
|
|
|
|
|
|
|
|
|
|
|
|
|
|
|
|
|
|
|
|
|
|
|
|
|
|
|
|
|
|
|
|
|
|
|
|
|
|
|
|
|
|
|
|
|
|
|
|
|
|
|
|
|
|
|
|
|
|
|
|
|
|
|
|
|
|
|
|
|
|
|
|
|
|
|
|
|
|
|
|
|
|
|
|
|
|
|
|
|
|
|
|
|
|
|
|
|
|
|
|
|
|
|
|
|
|
|
|
|
|
|
|
|
|
|
|
|
|
|
|
|
|
|
|
|
|
|
|
|
|
|
|
|
|
|
|
|
|
|
|
|
|
|
|
|
|
|
|
|
|
|
|
|
|
|
|
|
|
|
|
|
|
|
|
|
|
|
|
|
|
|
|
|
|
|
|
|
|
|
|
|
|
|
|
|
|
|
|
|
|
|
|
|
|
|
|
|
|
|
|
|
|
|
|
|
|
|
|
|
|
|
|
|
|
|
|
|
|
|
|
|
|
|
|
|
|
|
|
|
|
|
|
|
|
|
|
|
|
|
|
|
|
|
|
|
|
|
|
|
|
|
|
|
|
|
|
|
|
|
|
|
|
|
|
|
|
|
|
|
|
|
|
|
|
|
|
|
|
|
|
|
|
|
|
|
|
|
|
|
|
|
|
|
|
|
|
|
|
|
|
|
|
|
|
|
|
|
|
|
|
|
|
|
|
|
|
|
|
|
|
|
|
|
|
|
|
|
|
|
|
|
|
|
|
|
|
|
|
|
|
|
|
|
|
|
|
|
|
|
|
|
|
|
|
|
|
|
|
|
|
|
|
|
|
|
|
|
|
|
|
|
|
|
|
|
|
|
|
|
|
|
|
|
|
|
|
|
|
|
|
|
|
|
|
|
|
|
|
|
|
|
|
|
|
|
|
|
|
|
|
|
|
|
|
|
|
|
|
|
|
|
|
|
|
|
|
|
|
|
|
|
|
|
|
|
|
|
|
|
|
|
|
|
|
|
|
|
|
|
|
|
|
|
|
|
|
|
|
|
|
|
|
|
|
|
|
|
|
|
|
|
|
|
|
|
|
|
|
|
|
|
|
|
|
|
|
|
|
|
|
|
|
|
|
|
|
|
|
|
|
|
|
|
|
|
|
|
|
|
|
|
|
|
|
|
|
|
|
|
|
|
|
|
|
|
|
|
|
|
|
|
|
|
|
|
|
|
|
|
|
|
|
|
|
|
|
|
|
|
|
|
|
|
|
|
|
|
|
|
|
|
|
|
|
|
|
|
|
|
|
|
|
|
|
|
|
|
|
|
|
|
|
|
|
|
|
|
|
|
|
|
|
|
|
|
|
|
|
|
|
|
|
|
|
|
|
|
|
|
|
|
|
|
|
|
|
|
|
|
|
|
|
|
|
|
|
|
|
|
|
|
|
|
|
|
|
|
|
|
|
|
|
|
|
|
|
|
|
|
|
|
|
|
|
|
|
|
|
|
|
|
|
|
|
|
|
|
|
|
|
|
|
|
|
|
|
|
|
|
|
|
|
|
|
|
|
|
|
|
|
|
|
|
|
|
|
|
|
|
|
|
|
|
|
|
|
|
|
|
|
|
|
|
|
|
|
|
|
|
|
|
|
|
|
|
|
|
|
|
|
|
|
|
|
|
|
|
|
|
|
|
|
|
|
|
|
|
|
|
|
|
|
|
|
|
|
|
|
|
|
|
|
|
|
|
|
|
|
|
|
|
|
|
|
|
|
|
|
|
|
|
|
|
|
|
|
|
|
|
|
|
|
|
|
|
|
|
|
|
|
|
|
|
|
|
|
|
|
|
|
|
|
|
|
|
|
|
|
|
|
|
|
|
|
|
|
|
|
|
|
|
|
|
|
|
|
|
|
|
|
|
|
|
|
|
|
|
|
|
|
|
|
|
|
|
|
|
|
|
|
|
|
|
|
|
|
|
|
|
|
|
|
|
|
|
|
|
|
|
|
|
|
|
|
|
|
|
|
|
|
|
|
|
|
|
|
|
|
|
|
|
|
|
|
|
|
|
|
|
|
|
|
|
|
|
|
|
|
|
|
|
|
|
|
|
|
|
|
|
|
|
|
|
|
|
|
|
|
|
|
|
|
|
|
|
|
|
|
|
|
|
|
|
|
|
|
|
|
|
|
|
|
|
|
|
|
|
|
|
|
|
|
|
|
|
|
|
|
|
|
|
|
|
|
|
|
|
|
|
|
|
|
|
|
|
|
|
|
|
|
|
|
|
|
|
|
|
|
|
|
|
|
|
|
|
|
|
|
|
|
|
|
|
|
|
|
|
|
|
|
|
|
|
|
|
|
|
|
|
|
|
|
|
|
|
|
|
|
|
|
|
|
|
|
|
|
|
|
|
|
|
|
|
|
|
|
|
|
|
|
|
|
|
|
|
|
|
|
|
|
|
|
|
|
|
|
|
|
|
|
|
|
|
|
|
|
|
|
|
|
|
|
|
|
|
|
|
|
|
|
|
|
|
|
|
|
|
|
|
|
|
|
|
|
|
|
|
|
|
|
|
|
|
|
|
|
|
|
|
|
|
|
|
|
|
|
|
|
|
|
|
|
|
|
|
|
|
|
|
|
|
|
|
|
|
|
|
|
|
|
|
|
|
|
|
|
|
|
|
|
|
|
|
|
|
|
|
|
|
|
|
|
|
|
|
|
|
|
|
|
|
|
|
|
|
|
|
|
|
|
|
|
|
|
|
|
|
|
|
|
|
|
|
|
|
|
|
|
|
|
|
|
|
|
|
|
|
|
|
|
|
|
|
|
|
|
|
|
|
|
|
|
|
1 |
+
# This file is automatically @generated by Poetry 1.4.2 and should not be changed by hand.
|
2 |
+
|
3 |
+
[[package]]
|
4 |
+
name = "anyio"
|
5 |
+
version = "3.6.2"
|
6 |
+
description = "High level compatibility layer for multiple asynchronous event loop implementations"
|
7 |
+
category = "main"
|
8 |
+
optional = false
|
9 |
+
python-versions = ">=3.6.2"
|
10 |
+
files = [
|
11 |
+
{file = "anyio-3.6.2-py3-none-any.whl", hash = "sha256:fbbe32bd270d2a2ef3ed1c5d45041250284e31fc0a4df4a5a6071842051a51e3"},
|
12 |
+
{file = "anyio-3.6.2.tar.gz", hash = "sha256:25ea0d673ae30af41a0c442f81cf3b38c7e79fdc7b60335a4c14e05eb0947421"},
|
13 |
+
]
|
14 |
+
|
15 |
+
[package.dependencies]
|
16 |
+
idna = ">=2.8"
|
17 |
+
sniffio = ">=1.1"
|
18 |
+
|
19 |
+
[package.extras]
|
20 |
+
doc = ["packaging", "sphinx-autodoc-typehints (>=1.2.0)", "sphinx-rtd-theme"]
|
21 |
+
test = ["contextlib2", "coverage[toml] (>=4.5)", "hypothesis (>=4.0)", "mock (>=4)", "pytest (>=7.0)", "pytest-mock (>=3.6.1)", "trustme", "uvloop (<0.15)", "uvloop (>=0.15)"]
|
22 |
+
trio = ["trio (>=0.16,<0.22)"]
|
23 |
+
|
24 |
+
[[package]]
|
25 |
+
name = "black"
|
26 |
+
version = "23.3.0"
|
27 |
+
description = "The uncompromising code formatter."
|
28 |
+
category = "dev"
|
29 |
+
optional = false
|
30 |
+
python-versions = ">=3.7"
|
31 |
+
files = [
|
32 |
+
{file = "black-23.3.0-cp310-cp310-macosx_10_16_arm64.whl", hash = "sha256:0945e13506be58bf7db93ee5853243eb368ace1c08a24c65ce108986eac65915"},
|
33 |
+
{file = "black-23.3.0-cp310-cp310-macosx_10_16_universal2.whl", hash = "sha256:67de8d0c209eb5b330cce2469503de11bca4085880d62f1628bd9972cc3366b9"},
|
34 |
+
{file = "black-23.3.0-cp310-cp310-macosx_10_16_x86_64.whl", hash = "sha256:7c3eb7cea23904399866c55826b31c1f55bbcd3890ce22ff70466b907b6775c2"},
|
35 |
+
{file = "black-23.3.0-cp310-cp310-manylinux_2_17_x86_64.manylinux2014_x86_64.whl", hash = "sha256:32daa9783106c28815d05b724238e30718f34155653d4d6e125dc7daec8e260c"},
|
36 |
+
{file = "black-23.3.0-cp310-cp310-win_amd64.whl", hash = "sha256:35d1381d7a22cc5b2be2f72c7dfdae4072a3336060635718cc7e1ede24221d6c"},
|
37 |
+
{file = "black-23.3.0-cp311-cp311-macosx_10_16_arm64.whl", hash = "sha256:a8a968125d0a6a404842fa1bf0b349a568634f856aa08ffaff40ae0dfa52e7c6"},
|
38 |
+
{file = "black-23.3.0-cp311-cp311-macosx_10_16_universal2.whl", hash = "sha256:c7ab5790333c448903c4b721b59c0d80b11fe5e9803d8703e84dcb8da56fec1b"},
|
39 |
+
{file = "black-23.3.0-cp311-cp311-macosx_10_16_x86_64.whl", hash = "sha256:a6f6886c9869d4daae2d1715ce34a19bbc4b95006d20ed785ca00fa03cba312d"},
|
40 |
+
{file = "black-23.3.0-cp311-cp311-manylinux_2_17_x86_64.manylinux2014_x86_64.whl", hash = "sha256:6f3c333ea1dd6771b2d3777482429864f8e258899f6ff05826c3a4fcc5ce3f70"},
|
41 |
+
{file = "black-23.3.0-cp311-cp311-win_amd64.whl", hash = "sha256:11c410f71b876f961d1de77b9699ad19f939094c3a677323f43d7a29855fe326"},
|
42 |
+
{file = "black-23.3.0-cp37-cp37m-macosx_10_16_x86_64.whl", hash = "sha256:1d06691f1eb8de91cd1b322f21e3bfc9efe0c7ca1f0e1eb1db44ea367dff656b"},
|
43 |
+
{file = "black-23.3.0-cp37-cp37m-manylinux_2_17_x86_64.manylinux2014_x86_64.whl", hash = "sha256:50cb33cac881766a5cd9913e10ff75b1e8eb71babf4c7104f2e9c52da1fb7de2"},
|
44 |
+
{file = "black-23.3.0-cp37-cp37m-win_amd64.whl", hash = "sha256:e114420bf26b90d4b9daa597351337762b63039752bdf72bf361364c1aa05925"},
|
45 |
+
{file = "black-23.3.0-cp38-cp38-macosx_10_16_arm64.whl", hash = "sha256:48f9d345675bb7fbc3dd85821b12487e1b9a75242028adad0333ce36ed2a6d27"},
|
46 |
+
{file = "black-23.3.0-cp38-cp38-macosx_10_16_universal2.whl", hash = "sha256:714290490c18fb0126baa0fca0a54ee795f7502b44177e1ce7624ba1c00f2331"},
|
47 |
+
{file = "black-23.3.0-cp38-cp38-macosx_10_16_x86_64.whl", hash = "sha256:064101748afa12ad2291c2b91c960be28b817c0c7eaa35bec09cc63aa56493c5"},
|
48 |
+
{file = "black-23.3.0-cp38-cp38-manylinux_2_17_x86_64.manylinux2014_x86_64.whl", hash = "sha256:562bd3a70495facf56814293149e51aa1be9931567474993c7942ff7d3533961"},
|
49 |
+
{file = "black-23.3.0-cp38-cp38-win_amd64.whl", hash = "sha256:e198cf27888ad6f4ff331ca1c48ffc038848ea9f031a3b40ba36aced7e22f2c8"},
|
50 |
+
{file = "black-23.3.0-cp39-cp39-macosx_10_16_arm64.whl", hash = "sha256:3238f2aacf827d18d26db07524e44741233ae09a584273aa059066d644ca7b30"},
|
51 |
+
{file = "black-23.3.0-cp39-cp39-macosx_10_16_universal2.whl", hash = "sha256:f0bd2f4a58d6666500542b26354978218a9babcdc972722f4bf90779524515f3"},
|
52 |
+
{file = "black-23.3.0-cp39-cp39-macosx_10_16_x86_64.whl", hash = "sha256:92c543f6854c28a3c7f39f4d9b7694f9a6eb9d3c5e2ece488c327b6e7ea9b266"},
|
53 |
+
{file = "black-23.3.0-cp39-cp39-manylinux_2_17_x86_64.manylinux2014_x86_64.whl", hash = "sha256:3a150542a204124ed00683f0db1f5cf1c2aaaa9cc3495b7a3b5976fb136090ab"},
|
54 |
+
{file = "black-23.3.0-cp39-cp39-win_amd64.whl", hash = "sha256:6b39abdfb402002b8a7d030ccc85cf5afff64ee90fa4c5aebc531e3ad0175ddb"},
|
55 |
+
{file = "black-23.3.0-py3-none-any.whl", hash = "sha256:ec751418022185b0c1bb7d7736e6933d40bbb14c14a0abcf9123d1b159f98dd4"},
|
56 |
+
{file = "black-23.3.0.tar.gz", hash = "sha256:1c7b8d606e728a41ea1ccbd7264677e494e87cf630e399262ced92d4a8dac940"},
|
57 |
+
]
|
58 |
+
|
59 |
+
[package.dependencies]
|
60 |
+
click = ">=8.0.0"
|
61 |
+
mypy-extensions = ">=0.4.3"
|
62 |
+
packaging = ">=22.0"
|
63 |
+
pathspec = ">=0.9.0"
|
64 |
+
platformdirs = ">=2"
|
65 |
+
tomli = {version = ">=1.1.0", markers = "python_version < \"3.11\""}
|
66 |
+
typing-extensions = {version = ">=3.10.0.0", markers = "python_version < \"3.10\""}
|
67 |
+
|
68 |
+
[package.extras]
|
69 |
+
colorama = ["colorama (>=0.4.3)"]
|
70 |
+
d = ["aiohttp (>=3.7.4)"]
|
71 |
+
jupyter = ["ipython (>=7.8.0)", "tokenize-rt (>=3.2.0)"]
|
72 |
+
uvloop = ["uvloop (>=0.15.2)"]
|
73 |
+
|
74 |
+
[[package]]
|
75 |
+
name = "certifi"
|
76 |
+
version = "2023.5.7"
|
77 |
+
description = "Python package for providing Mozilla's CA Bundle."
|
78 |
+
category = "main"
|
79 |
+
optional = false
|
80 |
+
python-versions = ">=3.6"
|
81 |
+
files = [
|
82 |
+
{file = "certifi-2023.5.7-py3-none-any.whl", hash = "sha256:c6c2e98f5c7869efca1f8916fed228dd91539f9f1b444c314c06eef02980c716"},
|
83 |
+
{file = "certifi-2023.5.7.tar.gz", hash = "sha256:0f0d56dc5a6ad56fd4ba36484d6cc34451e1c6548c61daad8c320169f91eddc7"},
|
84 |
+
]
|
85 |
+
|
86 |
+
[[package]]
|
87 |
+
name = "charset-normalizer"
|
88 |
+
version = "3.1.0"
|
89 |
+
description = "The Real First Universal Charset Detector. Open, modern and actively maintained alternative to Chardet."
|
90 |
+
category = "main"
|
91 |
+
optional = false
|
92 |
+
python-versions = ">=3.7.0"
|
93 |
+
files = [
|
94 |
+
{file = "charset-normalizer-3.1.0.tar.gz", hash = "sha256:34e0a2f9c370eb95597aae63bf85eb5e96826d81e3dcf88b8886012906f509b5"},
|
95 |
+
{file = "charset_normalizer-3.1.0-cp310-cp310-macosx_10_9_universal2.whl", hash = "sha256:e0ac8959c929593fee38da1c2b64ee9778733cdf03c482c9ff1d508b6b593b2b"},
|
96 |
+
{file = "charset_normalizer-3.1.0-cp310-cp310-macosx_10_9_x86_64.whl", hash = "sha256:d7fc3fca01da18fbabe4625d64bb612b533533ed10045a2ac3dd194bfa656b60"},
|
97 |
+
{file = "charset_normalizer-3.1.0-cp310-cp310-macosx_11_0_arm64.whl", hash = "sha256:04eefcee095f58eaabe6dc3cc2262f3bcd776d2c67005880894f447b3f2cb9c1"},
|
98 |
+
{file = "charset_normalizer-3.1.0-cp310-cp310-manylinux_2_17_aarch64.manylinux2014_aarch64.whl", hash = "sha256:20064ead0717cf9a73a6d1e779b23d149b53daf971169289ed2ed43a71e8d3b0"},
|
99 |
+
{file = "charset_normalizer-3.1.0-cp310-cp310-manylinux_2_17_ppc64le.manylinux2014_ppc64le.whl", hash = "sha256:1435ae15108b1cb6fffbcea2af3d468683b7afed0169ad718451f8db5d1aff6f"},
|
100 |
+
{file = "charset_normalizer-3.1.0-cp310-cp310-manylinux_2_17_s390x.manylinux2014_s390x.whl", hash = "sha256:c84132a54c750fda57729d1e2599bb598f5fa0344085dbde5003ba429a4798c0"},
|
101 |
+
{file = "charset_normalizer-3.1.0-cp310-cp310-manylinux_2_17_x86_64.manylinux2014_x86_64.whl", hash = "sha256:75f2568b4189dda1c567339b48cba4ac7384accb9c2a7ed655cd86b04055c795"},
|
102 |
+
{file = "charset_normalizer-3.1.0-cp310-cp310-manylinux_2_5_i686.manylinux1_i686.manylinux_2_17_i686.manylinux2014_i686.whl", hash = "sha256:11d3bcb7be35e7b1bba2c23beedac81ee893ac9871d0ba79effc7fc01167db6c"},
|
103 |
+
{file = "charset_normalizer-3.1.0-cp310-cp310-musllinux_1_1_aarch64.whl", hash = "sha256:891cf9b48776b5c61c700b55a598621fdb7b1e301a550365571e9624f270c203"},
|
104 |
+
{file = "charset_normalizer-3.1.0-cp310-cp310-musllinux_1_1_i686.whl", hash = "sha256:5f008525e02908b20e04707a4f704cd286d94718f48bb33edddc7d7b584dddc1"},
|
105 |
+
{file = "charset_normalizer-3.1.0-cp310-cp310-musllinux_1_1_ppc64le.whl", hash = "sha256:b06f0d3bf045158d2fb8837c5785fe9ff9b8c93358be64461a1089f5da983137"},
|
106 |
+
{file = "charset_normalizer-3.1.0-cp310-cp310-musllinux_1_1_s390x.whl", hash = "sha256:49919f8400b5e49e961f320c735388ee686a62327e773fa5b3ce6721f7e785ce"},
|
107 |
+
{file = "charset_normalizer-3.1.0-cp310-cp310-musllinux_1_1_x86_64.whl", hash = "sha256:22908891a380d50738e1f978667536f6c6b526a2064156203d418f4856d6e86a"},
|
108 |
+
{file = "charset_normalizer-3.1.0-cp310-cp310-win32.whl", hash = "sha256:12d1a39aa6b8c6f6248bb54550efcc1c38ce0d8096a146638fd4738e42284448"},
|
109 |
+
{file = "charset_normalizer-3.1.0-cp310-cp310-win_amd64.whl", hash = "sha256:65ed923f84a6844de5fd29726b888e58c62820e0769b76565480e1fdc3d062f8"},
|
110 |
+
{file = "charset_normalizer-3.1.0-cp311-cp311-macosx_10_9_universal2.whl", hash = "sha256:9a3267620866c9d17b959a84dd0bd2d45719b817245e49371ead79ed4f710d19"},
|
111 |
+
{file = "charset_normalizer-3.1.0-cp311-cp311-macosx_10_9_x86_64.whl", hash = "sha256:6734e606355834f13445b6adc38b53c0fd45f1a56a9ba06c2058f86893ae8017"},
|
112 |
+
{file = "charset_normalizer-3.1.0-cp311-cp311-macosx_11_0_arm64.whl", hash = "sha256:f8303414c7b03f794347ad062c0516cee0e15f7a612abd0ce1e25caf6ceb47df"},
|
113 |
+
{file = "charset_normalizer-3.1.0-cp311-cp311-manylinux_2_17_aarch64.manylinux2014_aarch64.whl", hash = "sha256:aaf53a6cebad0eae578f062c7d462155eada9c172bd8c4d250b8c1d8eb7f916a"},
|
114 |
+
{file = "charset_normalizer-3.1.0-cp311-cp311-manylinux_2_17_ppc64le.manylinux2014_ppc64le.whl", hash = "sha256:3dc5b6a8ecfdc5748a7e429782598e4f17ef378e3e272eeb1340ea57c9109f41"},
|
115 |
+
{file = "charset_normalizer-3.1.0-cp311-cp311-manylinux_2_17_s390x.manylinux2014_s390x.whl", hash = "sha256:e1b25e3ad6c909f398df8921780d6a3d120d8c09466720226fc621605b6f92b1"},
|
116 |
+
{file = "charset_normalizer-3.1.0-cp311-cp311-manylinux_2_17_x86_64.manylinux2014_x86_64.whl", hash = "sha256:0ca564606d2caafb0abe6d1b5311c2649e8071eb241b2d64e75a0d0065107e62"},
|
117 |
+
{file = "charset_normalizer-3.1.0-cp311-cp311-manylinux_2_5_i686.manylinux1_i686.manylinux_2_17_i686.manylinux2014_i686.whl", hash = "sha256:b82fab78e0b1329e183a65260581de4375f619167478dddab510c6c6fb04d9b6"},
|
118 |
+
{file = "charset_normalizer-3.1.0-cp311-cp311-musllinux_1_1_aarch64.whl", hash = "sha256:bd7163182133c0c7701b25e604cf1611c0d87712e56e88e7ee5d72deab3e76b5"},
|
119 |
+
{file = "charset_normalizer-3.1.0-cp311-cp311-musllinux_1_1_i686.whl", hash = "sha256:11d117e6c63e8f495412d37e7dc2e2fff09c34b2d09dbe2bee3c6229577818be"},
|
120 |
+
{file = "charset_normalizer-3.1.0-cp311-cp311-musllinux_1_1_ppc64le.whl", hash = "sha256:cf6511efa4801b9b38dc5546d7547d5b5c6ef4b081c60b23e4d941d0eba9cbeb"},
|
121 |
+
{file = "charset_normalizer-3.1.0-cp311-cp311-musllinux_1_1_s390x.whl", hash = "sha256:abc1185d79f47c0a7aaf7e2412a0eb2c03b724581139193d2d82b3ad8cbb00ac"},
|
122 |
+
{file = "charset_normalizer-3.1.0-cp311-cp311-musllinux_1_1_x86_64.whl", hash = "sha256:cb7b2ab0188829593b9de646545175547a70d9a6e2b63bf2cd87a0a391599324"},
|
123 |
+
{file = "charset_normalizer-3.1.0-cp311-cp311-win32.whl", hash = "sha256:c36bcbc0d5174a80d6cccf43a0ecaca44e81d25be4b7f90f0ed7bcfbb5a00909"},
|
124 |
+
{file = "charset_normalizer-3.1.0-cp311-cp311-win_amd64.whl", hash = "sha256:cca4def576f47a09a943666b8f829606bcb17e2bc2d5911a46c8f8da45f56755"},
|
125 |
+
{file = "charset_normalizer-3.1.0-cp37-cp37m-macosx_10_9_x86_64.whl", hash = "sha256:0c95f12b74681e9ae127728f7e5409cbbef9cd914d5896ef238cc779b8152373"},
|
126 |
+
{file = "charset_normalizer-3.1.0-cp37-cp37m-manylinux_2_17_aarch64.manylinux2014_aarch64.whl", hash = "sha256:fca62a8301b605b954ad2e9c3666f9d97f63872aa4efcae5492baca2056b74ab"},
|
127 |
+
{file = "charset_normalizer-3.1.0-cp37-cp37m-manylinux_2_17_ppc64le.manylinux2014_ppc64le.whl", hash = "sha256:ac0aa6cd53ab9a31d397f8303f92c42f534693528fafbdb997c82bae6e477ad9"},
|
128 |
+
{file = "charset_normalizer-3.1.0-cp37-cp37m-manylinux_2_17_s390x.manylinux2014_s390x.whl", hash = "sha256:c3af8e0f07399d3176b179f2e2634c3ce9c1301379a6b8c9c9aeecd481da494f"},
|
129 |
+
{file = "charset_normalizer-3.1.0-cp37-cp37m-manylinux_2_17_x86_64.manylinux2014_x86_64.whl", hash = "sha256:3a5fc78f9e3f501a1614a98f7c54d3969f3ad9bba8ba3d9b438c3bc5d047dd28"},
|
130 |
+
{file = "charset_normalizer-3.1.0-cp37-cp37m-manylinux_2_5_i686.manylinux1_i686.manylinux_2_17_i686.manylinux2014_i686.whl", hash = "sha256:628c985afb2c7d27a4800bfb609e03985aaecb42f955049957814e0491d4006d"},
|
131 |
+
{file = "charset_normalizer-3.1.0-cp37-cp37m-musllinux_1_1_aarch64.whl", hash = "sha256:74db0052d985cf37fa111828d0dd230776ac99c740e1a758ad99094be4f1803d"},
|
132 |
+
{file = "charset_normalizer-3.1.0-cp37-cp37m-musllinux_1_1_i686.whl", hash = "sha256:1e8fcdd8f672a1c4fc8d0bd3a2b576b152d2a349782d1eb0f6b8e52e9954731d"},
|
133 |
+
{file = "charset_normalizer-3.1.0-cp37-cp37m-musllinux_1_1_ppc64le.whl", hash = "sha256:04afa6387e2b282cf78ff3dbce20f0cc071c12dc8f685bd40960cc68644cfea6"},
|
134 |
+
{file = "charset_normalizer-3.1.0-cp37-cp37m-musllinux_1_1_s390x.whl", hash = "sha256:dd5653e67b149503c68c4018bf07e42eeed6b4e956b24c00ccdf93ac79cdff84"},
|
135 |
+
{file = "charset_normalizer-3.1.0-cp37-cp37m-musllinux_1_1_x86_64.whl", hash = "sha256:d2686f91611f9e17f4548dbf050e75b079bbc2a82be565832bc8ea9047b61c8c"},
|
136 |
+
{file = "charset_normalizer-3.1.0-cp37-cp37m-win32.whl", hash = "sha256:4155b51ae05ed47199dc5b2a4e62abccb274cee6b01da5b895099b61b1982974"},
|
137 |
+
{file = "charset_normalizer-3.1.0-cp37-cp37m-win_amd64.whl", hash = "sha256:322102cdf1ab682ecc7d9b1c5eed4ec59657a65e1c146a0da342b78f4112db23"},
|
138 |
+
{file = "charset_normalizer-3.1.0-cp38-cp38-macosx_10_9_universal2.whl", hash = "sha256:e633940f28c1e913615fd624fcdd72fdba807bf53ea6925d6a588e84e1151531"},
|
139 |
+
{file = "charset_normalizer-3.1.0-cp38-cp38-macosx_10_9_x86_64.whl", hash = "sha256:3a06f32c9634a8705f4ca9946d667609f52cf130d5548881401f1eb2c39b1e2c"},
|
140 |
+
{file = "charset_normalizer-3.1.0-cp38-cp38-macosx_11_0_arm64.whl", hash = "sha256:7381c66e0561c5757ffe616af869b916c8b4e42b367ab29fedc98481d1e74e14"},
|
141 |
+
{file = "charset_normalizer-3.1.0-cp38-cp38-manylinux_2_17_aarch64.manylinux2014_aarch64.whl", hash = "sha256:3573d376454d956553c356df45bb824262c397c6e26ce43e8203c4c540ee0acb"},
|
142 |
+
{file = "charset_normalizer-3.1.0-cp38-cp38-manylinux_2_17_ppc64le.manylinux2014_ppc64le.whl", hash = "sha256:e89df2958e5159b811af9ff0f92614dabf4ff617c03a4c1c6ff53bf1c399e0e1"},
|
143 |
+
{file = "charset_normalizer-3.1.0-cp38-cp38-manylinux_2_17_s390x.manylinux2014_s390x.whl", hash = "sha256:78cacd03e79d009d95635e7d6ff12c21eb89b894c354bd2b2ed0b4763373693b"},
|
144 |
+
{file = "charset_normalizer-3.1.0-cp38-cp38-manylinux_2_17_x86_64.manylinux2014_x86_64.whl", hash = "sha256:de5695a6f1d8340b12a5d6d4484290ee74d61e467c39ff03b39e30df62cf83a0"},
|
145 |
+
{file = "charset_normalizer-3.1.0-cp38-cp38-manylinux_2_5_i686.manylinux1_i686.manylinux_2_17_i686.manylinux2014_i686.whl", hash = "sha256:1c60b9c202d00052183c9be85e5eaf18a4ada0a47d188a83c8f5c5b23252f649"},
|
146 |
+
{file = "charset_normalizer-3.1.0-cp38-cp38-musllinux_1_1_aarch64.whl", hash = "sha256:f645caaf0008bacf349875a974220f1f1da349c5dbe7c4ec93048cdc785a3326"},
|
147 |
+
{file = "charset_normalizer-3.1.0-cp38-cp38-musllinux_1_1_i686.whl", hash = "sha256:ea9f9c6034ea2d93d9147818f17c2a0860d41b71c38b9ce4d55f21b6f9165a11"},
|
148 |
+
{file = "charset_normalizer-3.1.0-cp38-cp38-musllinux_1_1_ppc64le.whl", hash = "sha256:80d1543d58bd3d6c271b66abf454d437a438dff01c3e62fdbcd68f2a11310d4b"},
|
149 |
+
{file = "charset_normalizer-3.1.0-cp38-cp38-musllinux_1_1_s390x.whl", hash = "sha256:73dc03a6a7e30b7edc5b01b601e53e7fc924b04e1835e8e407c12c037e81adbd"},
|
150 |
+
{file = "charset_normalizer-3.1.0-cp38-cp38-musllinux_1_1_x86_64.whl", hash = "sha256:6f5c2e7bc8a4bf7c426599765b1bd33217ec84023033672c1e9a8b35eaeaaaf8"},
|
151 |
+
{file = "charset_normalizer-3.1.0-cp38-cp38-win32.whl", hash = "sha256:12a2b561af122e3d94cdb97fe6fb2bb2b82cef0cdca131646fdb940a1eda04f0"},
|
152 |
+
{file = "charset_normalizer-3.1.0-cp38-cp38-win_amd64.whl", hash = "sha256:3160a0fd9754aab7d47f95a6b63ab355388d890163eb03b2d2b87ab0a30cfa59"},
|
153 |
+
{file = "charset_normalizer-3.1.0-cp39-cp39-macosx_10_9_universal2.whl", hash = "sha256:38e812a197bf8e71a59fe55b757a84c1f946d0ac114acafaafaf21667a7e169e"},
|
154 |
+
{file = "charset_normalizer-3.1.0-cp39-cp39-macosx_10_9_x86_64.whl", hash = "sha256:6baf0baf0d5d265fa7944feb9f7451cc316bfe30e8df1a61b1bb08577c554f31"},
|
155 |
+
{file = "charset_normalizer-3.1.0-cp39-cp39-macosx_11_0_arm64.whl", hash = "sha256:8f25e17ab3039b05f762b0a55ae0b3632b2e073d9c8fc88e89aca31a6198e88f"},
|
156 |
+
{file = "charset_normalizer-3.1.0-cp39-cp39-manylinux_2_17_aarch64.manylinux2014_aarch64.whl", hash = "sha256:3747443b6a904001473370d7810aa19c3a180ccd52a7157aacc264a5ac79265e"},
|
157 |
+
{file = "charset_normalizer-3.1.0-cp39-cp39-manylinux_2_17_ppc64le.manylinux2014_ppc64le.whl", hash = "sha256:b116502087ce8a6b7a5f1814568ccbd0e9f6cfd99948aa59b0e241dc57cf739f"},
|
158 |
+
{file = "charset_normalizer-3.1.0-cp39-cp39-manylinux_2_17_s390x.manylinux2014_s390x.whl", hash = "sha256:d16fd5252f883eb074ca55cb622bc0bee49b979ae4e8639fff6ca3ff44f9f854"},
|
159 |
+
{file = "charset_normalizer-3.1.0-cp39-cp39-manylinux_2_17_x86_64.manylinux2014_x86_64.whl", hash = "sha256:21fa558996782fc226b529fdd2ed7866c2c6ec91cee82735c98a197fae39f706"},
|
160 |
+
{file = "charset_normalizer-3.1.0-cp39-cp39-manylinux_2_5_i686.manylinux1_i686.manylinux_2_17_i686.manylinux2014_i686.whl", hash = "sha256:6f6c7a8a57e9405cad7485f4c9d3172ae486cfef1344b5ddd8e5239582d7355e"},
|
161 |
+
{file = "charset_normalizer-3.1.0-cp39-cp39-musllinux_1_1_aarch64.whl", hash = "sha256:ac3775e3311661d4adace3697a52ac0bab17edd166087d493b52d4f4f553f9f0"},
|
162 |
+
{file = "charset_normalizer-3.1.0-cp39-cp39-musllinux_1_1_i686.whl", hash = "sha256:10c93628d7497c81686e8e5e557aafa78f230cd9e77dd0c40032ef90c18f2230"},
|
163 |
+
{file = "charset_normalizer-3.1.0-cp39-cp39-musllinux_1_1_ppc64le.whl", hash = "sha256:6f4f4668e1831850ebcc2fd0b1cd11721947b6dc7c00bf1c6bd3c929ae14f2c7"},
|
164 |
+
{file = "charset_normalizer-3.1.0-cp39-cp39-musllinux_1_1_s390x.whl", hash = "sha256:0be65ccf618c1e7ac9b849c315cc2e8a8751d9cfdaa43027d4f6624bd587ab7e"},
|
165 |
+
{file = "charset_normalizer-3.1.0-cp39-cp39-musllinux_1_1_x86_64.whl", hash = "sha256:53d0a3fa5f8af98a1e261de6a3943ca631c526635eb5817a87a59d9a57ebf48f"},
|
166 |
+
{file = "charset_normalizer-3.1.0-cp39-cp39-win32.whl", hash = "sha256:a04f86f41a8916fe45ac5024ec477f41f886b3c435da2d4e3d2709b22ab02af1"},
|
167 |
+
{file = "charset_normalizer-3.1.0-cp39-cp39-win_amd64.whl", hash = "sha256:830d2948a5ec37c386d3170c483063798d7879037492540f10a475e3fd6f244b"},
|
168 |
+
{file = "charset_normalizer-3.1.0-py3-none-any.whl", hash = "sha256:3d9098b479e78c85080c98e1e35ff40b4a31d8953102bb0fd7d1b6f8a2111a3d"},
|
169 |
+
]
|
170 |
+
|
171 |
+
[[package]]
|
172 |
+
name = "click"
|
173 |
+
version = "8.1.3"
|
174 |
+
description = "Composable command line interface toolkit"
|
175 |
+
category = "dev"
|
176 |
+
optional = false
|
177 |
+
python-versions = ">=3.7"
|
178 |
+
files = [
|
179 |
+
{file = "click-8.1.3-py3-none-any.whl", hash = "sha256:bb4d8133cb15a609f44e8213d9b391b0809795062913b383c62be0ee95b1db48"},
|
180 |
+
{file = "click-8.1.3.tar.gz", hash = "sha256:7682dc8afb30297001674575ea00d1814d808d6a36af415a82bd481d37ba7b8e"},
|
181 |
+
]
|
182 |
+
|
183 |
+
[package.dependencies]
|
184 |
+
colorama = {version = "*", markers = "platform_system == \"Windows\""}
|
185 |
+
|
186 |
+
[[package]]
|
187 |
+
name = "colorama"
|
188 |
+
version = "0.4.6"
|
189 |
+
description = "Cross-platform colored terminal text."
|
190 |
+
category = "main"
|
191 |
+
optional = false
|
192 |
+
python-versions = "!=3.0.*,!=3.1.*,!=3.2.*,!=3.3.*,!=3.4.*,!=3.5.*,!=3.6.*,>=2.7"
|
193 |
+
files = [
|
194 |
+
{file = "colorama-0.4.6-py2.py3-none-any.whl", hash = "sha256:4f1d9991f5acc0ca119f9d443620b77f9d6b33703e51011c16baf57afb285fc6"},
|
195 |
+
{file = "colorama-0.4.6.tar.gz", hash = "sha256:08695f5cb7ed6e0531a20572697297273c47b8cae5a63ffc6d6ed5c201be6e44"},
|
196 |
+
]
|
197 |
+
|
198 |
+
[[package]]
|
199 |
+
name = "exceptiongroup"
|
200 |
+
version = "1.1.1"
|
201 |
+
description = "Backport of PEP 654 (exception groups)"
|
202 |
+
category = "dev"
|
203 |
+
optional = false
|
204 |
+
python-versions = ">=3.7"
|
205 |
+
files = [
|
206 |
+
{file = "exceptiongroup-1.1.1-py3-none-any.whl", hash = "sha256:232c37c63e4f682982c8b6459f33a8981039e5fb8756b2074364e5055c498c9e"},
|
207 |
+
{file = "exceptiongroup-1.1.1.tar.gz", hash = "sha256:d484c3090ba2889ae2928419117447a14daf3c1231d5e30d0aae34f354f01785"},
|
208 |
+
]
|
209 |
+
|
210 |
+
[package.extras]
|
211 |
+
test = ["pytest (>=6)"]
|
212 |
+
|
213 |
+
[[package]]
|
214 |
+
name = "filelock"
|
215 |
+
version = "3.12.0"
|
216 |
+
description = "A platform independent file lock."
|
217 |
+
category = "main"
|
218 |
+
optional = false
|
219 |
+
python-versions = ">=3.7"
|
220 |
+
files = [
|
221 |
+
{file = "filelock-3.12.0-py3-none-any.whl", hash = "sha256:ad98852315c2ab702aeb628412cbf7e95b7ce8c3bf9565670b4eaecf1db370a9"},
|
222 |
+
{file = "filelock-3.12.0.tar.gz", hash = "sha256:fc03ae43288c013d2ea83c8597001b1129db351aad9c57fe2409327916b8e718"},
|
223 |
+
]
|
224 |
+
|
225 |
+
[package.extras]
|
226 |
+
docs = ["furo (>=2023.3.27)", "sphinx (>=6.1.3)", "sphinx-autodoc-typehints (>=1.23,!=1.23.4)"]
|
227 |
+
testing = ["covdefaults (>=2.3)", "coverage (>=7.2.3)", "diff-cover (>=7.5)", "pytest (>=7.3.1)", "pytest-cov (>=4)", "pytest-mock (>=3.10)", "pytest-timeout (>=2.1)"]
|
228 |
+
|
229 |
+
[[package]]
|
230 |
+
name = "flake8"
|
231 |
+
version = "5.0.4"
|
232 |
+
description = "the modular source code checker: pep8 pyflakes and co"
|
233 |
+
category = "dev"
|
234 |
+
optional = false
|
235 |
+
python-versions = ">=3.6.1"
|
236 |
+
files = [
|
237 |
+
{file = "flake8-5.0.4-py2.py3-none-any.whl", hash = "sha256:7a1cf6b73744f5806ab95e526f6f0d8c01c66d7bbe349562d22dfca20610b248"},
|
238 |
+
{file = "flake8-5.0.4.tar.gz", hash = "sha256:6fbe320aad8d6b95cec8b8e47bc933004678dc63095be98528b7bdd2a9f510db"},
|
239 |
+
]
|
240 |
+
|
241 |
+
[package.dependencies]
|
242 |
+
mccabe = ">=0.7.0,<0.8.0"
|
243 |
+
pycodestyle = ">=2.9.0,<2.10.0"
|
244 |
+
pyflakes = ">=2.5.0,<2.6.0"
|
245 |
+
|
246 |
+
[[package]]
|
247 |
+
name = "flake8-pyproject"
|
248 |
+
version = "1.2.3"
|
249 |
+
description = "Flake8 plug-in loading the configuration from pyproject.toml"
|
250 |
+
category = "dev"
|
251 |
+
optional = false
|
252 |
+
python-versions = ">= 3.6"
|
253 |
+
files = [
|
254 |
+
{file = "flake8_pyproject-1.2.3-py3-none-any.whl", hash = "sha256:6249fe53545205af5e76837644dc80b4c10037e73a0e5db87ff562d75fb5bd4a"},
|
255 |
+
]
|
256 |
+
|
257 |
+
[package.dependencies]
|
258 |
+
Flake8 = ">=5"
|
259 |
+
TOMLi = {version = "*", markers = "python_version < \"3.11\""}
|
260 |
+
|
261 |
+
[package.extras]
|
262 |
+
dev = ["pyTest", "pyTest-cov"]
|
263 |
+
|
264 |
+
[[package]]
|
265 |
+
name = "fsspec"
|
266 |
+
version = "2023.5.0"
|
267 |
+
description = "File-system specification"
|
268 |
+
category = "main"
|
269 |
+
optional = false
|
270 |
+
python-versions = ">=3.8"
|
271 |
+
files = [
|
272 |
+
{file = "fsspec-2023.5.0-py3-none-any.whl", hash = "sha256:51a4ad01a5bb66fcc58036e288c0d53d3975a0df2a5dc59a93b59bade0391f2a"},
|
273 |
+
{file = "fsspec-2023.5.0.tar.gz", hash = "sha256:b3b56e00fb93ea321bc9e5d9cf6f8522a0198b20eb24e02774d329e9c6fb84ce"},
|
274 |
+
]
|
275 |
+
|
276 |
+
[package.extras]
|
277 |
+
abfs = ["adlfs"]
|
278 |
+
adl = ["adlfs"]
|
279 |
+
arrow = ["pyarrow (>=1)"]
|
280 |
+
dask = ["dask", "distributed"]
|
281 |
+
devel = ["pytest", "pytest-cov"]
|
282 |
+
dropbox = ["dropbox", "dropboxdrivefs", "requests"]
|
283 |
+
full = ["adlfs", "aiohttp (!=4.0.0a0,!=4.0.0a1)", "dask", "distributed", "dropbox", "dropboxdrivefs", "fusepy", "gcsfs", "libarchive-c", "ocifs", "panel", "paramiko", "pyarrow (>=1)", "pygit2", "requests", "s3fs", "smbprotocol", "tqdm"]
|
284 |
+
fuse = ["fusepy"]
|
285 |
+
gcs = ["gcsfs"]
|
286 |
+
git = ["pygit2"]
|
287 |
+
github = ["requests"]
|
288 |
+
gs = ["gcsfs"]
|
289 |
+
gui = ["panel"]
|
290 |
+
hdfs = ["pyarrow (>=1)"]
|
291 |
+
http = ["aiohttp (!=4.0.0a0,!=4.0.0a1)", "requests"]
|
292 |
+
libarchive = ["libarchive-c"]
|
293 |
+
oci = ["ocifs"]
|
294 |
+
s3 = ["s3fs"]
|
295 |
+
sftp = ["paramiko"]
|
296 |
+
smb = ["smbprotocol"]
|
297 |
+
ssh = ["paramiko"]
|
298 |
+
tqdm = ["tqdm"]
|
299 |
+
|
300 |
+
[[package]]
|
301 |
+
name = "gradio-client"
|
302 |
+
version = "0.2.6"
|
303 |
+
description = "Python library for easily interacting with trained machine learning models"
|
304 |
+
category = "main"
|
305 |
+
optional = false
|
306 |
+
python-versions = ">=3.7"
|
307 |
+
files = [
|
308 |
+
{file = "gradio_client-0.2.6-py3-none-any.whl", hash = "sha256:daa3e2f7697cc07821ccefa7486b923e71c1e459d5b3c6e35318f343b566d789"},
|
309 |
+
{file = "gradio_client-0.2.6.tar.gz", hash = "sha256:a5d5c5799ce33ae3107e1d30992c27050f50506f15dd70481a39b13ac47e2613"},
|
310 |
+
]
|
311 |
+
|
312 |
+
[package.dependencies]
|
313 |
+
fsspec = "*"
|
314 |
+
httpx = "*"
|
315 |
+
huggingface-hub = ">=0.13.0"
|
316 |
+
packaging = "*"
|
317 |
+
requests = "*"
|
318 |
+
typing-extensions = "*"
|
319 |
+
websockets = "*"
|
320 |
+
|
321 |
+
[[package]]
|
322 |
+
name = "h11"
|
323 |
+
version = "0.14.0"
|
324 |
+
description = "A pure-Python, bring-your-own-I/O implementation of HTTP/1.1"
|
325 |
+
category = "main"
|
326 |
+
optional = false
|
327 |
+
python-versions = ">=3.7"
|
328 |
+
files = [
|
329 |
+
{file = "h11-0.14.0-py3-none-any.whl", hash = "sha256:e3fe4ac4b851c468cc8363d500db52c2ead036020723024a109d37346efaa761"},
|
330 |
+
{file = "h11-0.14.0.tar.gz", hash = "sha256:8f19fbbe99e72420ff35c00b27a34cb9937e902a8b810e2c88300c6f0a3b699d"},
|
331 |
+
]
|
332 |
+
|
333 |
+
[[package]]
|
334 |
+
name = "httpcore"
|
335 |
+
version = "0.17.0"
|
336 |
+
description = "A minimal low-level HTTP client."
|
337 |
+
category = "main"
|
338 |
+
optional = false
|
339 |
+
python-versions = ">=3.7"
|
340 |
+
files = [
|
341 |
+
{file = "httpcore-0.17.0-py3-none-any.whl", hash = "sha256:0fdfea45e94f0c9fd96eab9286077f9ff788dd186635ae61b312693e4d943599"},
|
342 |
+
{file = "httpcore-0.17.0.tar.gz", hash = "sha256:cc045a3241afbf60ce056202301b4d8b6af08845e3294055eb26b09913ef903c"},
|
343 |
+
]
|
344 |
+
|
345 |
+
[package.dependencies]
|
346 |
+
anyio = ">=3.0,<5.0"
|
347 |
+
certifi = "*"
|
348 |
+
h11 = ">=0.13,<0.15"
|
349 |
+
sniffio = ">=1.0.0,<2.0.0"
|
350 |
+
|
351 |
+
[package.extras]
|
352 |
+
http2 = ["h2 (>=3,<5)"]
|
353 |
+
socks = ["socksio (>=1.0.0,<2.0.0)"]
|
354 |
+
|
355 |
+
[[package]]
|
356 |
+
name = "httpx"
|
357 |
+
version = "0.24.0"
|
358 |
+
description = "The next generation HTTP client."
|
359 |
+
category = "main"
|
360 |
+
optional = false
|
361 |
+
python-versions = ">=3.7"
|
362 |
+
files = [
|
363 |
+
{file = "httpx-0.24.0-py3-none-any.whl", hash = "sha256:447556b50c1921c351ea54b4fe79d91b724ed2b027462ab9a329465d147d5a4e"},
|
364 |
+
{file = "httpx-0.24.0.tar.gz", hash = "sha256:507d676fc3e26110d41df7d35ebd8b3b8585052450f4097401c9be59d928c63e"},
|
365 |
+
]
|
366 |
+
|
367 |
+
[package.dependencies]
|
368 |
+
certifi = "*"
|
369 |
+
httpcore = ">=0.15.0,<0.18.0"
|
370 |
+
idna = "*"
|
371 |
+
sniffio = "*"
|
372 |
+
|
373 |
+
[package.extras]
|
374 |
+
brotli = ["brotli", "brotlicffi"]
|
375 |
+
cli = ["click (>=8.0.0,<9.0.0)", "pygments (>=2.0.0,<3.0.0)", "rich (>=10,<14)"]
|
376 |
+
http2 = ["h2 (>=3,<5)"]
|
377 |
+
socks = ["socksio (>=1.0.0,<2.0.0)"]
|
378 |
+
|
379 |
+
[[package]]
|
380 |
+
name = "huggingface-hub"
|
381 |
+
version = "0.14.1"
|
382 |
+
description = "Client library to download and publish models, datasets and other repos on the huggingface.co hub"
|
383 |
+
category = "main"
|
384 |
+
optional = false
|
385 |
+
python-versions = ">=3.7.0"
|
386 |
+
files = [
|
387 |
+
{file = "huggingface_hub-0.14.1-py3-none-any.whl", hash = "sha256:9fc619170d800ff3793ad37c9757c255c8783051e1b5b00501205eb43ccc4f27"},
|
388 |
+
{file = "huggingface_hub-0.14.1.tar.gz", hash = "sha256:9ab899af8e10922eac65e290d60ab956882ab0bf643e3d990b1394b6b47b7fbc"},
|
389 |
+
]
|
390 |
+
|
391 |
+
[package.dependencies]
|
392 |
+
filelock = "*"
|
393 |
+
fsspec = "*"
|
394 |
+
packaging = ">=20.9"
|
395 |
+
pyyaml = ">=5.1"
|
396 |
+
requests = "*"
|
397 |
+
tqdm = ">=4.42.1"
|
398 |
+
typing-extensions = ">=3.7.4.3"
|
399 |
+
|
400 |
+
[package.extras]
|
401 |
+
all = ["InquirerPy (==0.3.4)", "Jinja2", "Pillow", "black (>=23.1,<24.0)", "gradio", "jedi", "mypy (==0.982)", "pytest", "pytest-cov", "pytest-env", "pytest-xdist", "ruff (>=0.0.241)", "soundfile", "types-PyYAML", "types-requests", "types-simplejson", "types-toml", "types-tqdm", "types-urllib3"]
|
402 |
+
cli = ["InquirerPy (==0.3.4)"]
|
403 |
+
dev = ["InquirerPy (==0.3.4)", "Jinja2", "Pillow", "black (>=23.1,<24.0)", "gradio", "jedi", "mypy (==0.982)", "pytest", "pytest-cov", "pytest-env", "pytest-xdist", "ruff (>=0.0.241)", "soundfile", "types-PyYAML", "types-requests", "types-simplejson", "types-toml", "types-tqdm", "types-urllib3"]
|
404 |
+
fastai = ["fastai (>=2.4)", "fastcore (>=1.3.27)", "toml"]
|
405 |
+
quality = ["black (>=23.1,<24.0)", "mypy (==0.982)", "ruff (>=0.0.241)"]
|
406 |
+
tensorflow = ["graphviz", "pydot", "tensorflow"]
|
407 |
+
testing = ["InquirerPy (==0.3.4)", "Jinja2", "Pillow", "gradio", "jedi", "pytest", "pytest-cov", "pytest-env", "pytest-xdist", "soundfile"]
|
408 |
+
torch = ["torch"]
|
409 |
+
typing = ["types-PyYAML", "types-requests", "types-simplejson", "types-toml", "types-tqdm", "types-urllib3"]
|
410 |
+
|
411 |
+
[[package]]
|
412 |
+
name = "idna"
|
413 |
+
version = "3.4"
|
414 |
+
description = "Internationalized Domain Names in Applications (IDNA)"
|
415 |
+
category = "main"
|
416 |
+
optional = false
|
417 |
+
python-versions = ">=3.5"
|
418 |
+
files = [
|
419 |
+
{file = "idna-3.4-py3-none-any.whl", hash = "sha256:90b77e79eaa3eba6de819a0c442c0b4ceefc341a7a2ab77d7562bf49f425c5c2"},
|
420 |
+
{file = "idna-3.4.tar.gz", hash = "sha256:814f528e8dead7d329833b91c5faa87d60bf71824cd12a7530b5526063d02cb4"},
|
421 |
+
]
|
422 |
+
|
423 |
+
[[package]]
|
424 |
+
name = "iniconfig"
|
425 |
+
version = "2.0.0"
|
426 |
+
description = "brain-dead simple config-ini parsing"
|
427 |
+
category = "dev"
|
428 |
+
optional = false
|
429 |
+
python-versions = ">=3.7"
|
430 |
+
files = [
|
431 |
+
{file = "iniconfig-2.0.0-py3-none-any.whl", hash = "sha256:b6a85871a79d2e3b22d2d1b94ac2824226a63c6b741c88f7ae975f18b6778374"},
|
432 |
+
{file = "iniconfig-2.0.0.tar.gz", hash = "sha256:2d91e135bf72d31a410b17c16da610a82cb55f6b0477d1a902134b24a455b8b3"},
|
433 |
+
]
|
434 |
+
|
435 |
+
[[package]]
|
436 |
+
name = "isort"
|
437 |
+
version = "5.12.0"
|
438 |
+
description = "A Python utility / library to sort Python imports."
|
439 |
+
category = "dev"
|
440 |
+
optional = false
|
441 |
+
python-versions = ">=3.8.0"
|
442 |
+
files = [
|
443 |
+
{file = "isort-5.12.0-py3-none-any.whl", hash = "sha256:f84c2818376e66cf843d497486ea8fed8700b340f308f076c6fb1229dff318b6"},
|
444 |
+
{file = "isort-5.12.0.tar.gz", hash = "sha256:8bef7dde241278824a6d83f44a544709b065191b95b6e50894bdc722fcba0504"},
|
445 |
+
]
|
446 |
+
|
447 |
+
[package.extras]
|
448 |
+
colors = ["colorama (>=0.4.3)"]
|
449 |
+
pipfile-deprecated-finder = ["pip-shims (>=0.5.2)", "pipreqs", "requirementslib"]
|
450 |
+
plugins = ["setuptools"]
|
451 |
+
requirements-deprecated-finder = ["pip-api", "pipreqs"]
|
452 |
+
|
453 |
+
[[package]]
|
454 |
+
name = "mccabe"
|
455 |
+
version = "0.7.0"
|
456 |
+
description = "McCabe checker, plugin for flake8"
|
457 |
+
category = "dev"
|
458 |
+
optional = false
|
459 |
+
python-versions = ">=3.6"
|
460 |
+
files = [
|
461 |
+
{file = "mccabe-0.7.0-py2.py3-none-any.whl", hash = "sha256:6c2d30ab6be0e4a46919781807b4f0d834ebdd6c6e3dca0bda5a15f863427b6e"},
|
462 |
+
{file = "mccabe-0.7.0.tar.gz", hash = "sha256:348e0240c33b60bbdf4e523192ef919f28cb2c3d7d5c7794f74009290f236325"},
|
463 |
+
]
|
464 |
+
|
465 |
+
[[package]]
|
466 |
+
name = "mypy"
|
467 |
+
version = "1.3.0"
|
468 |
+
description = "Optional static typing for Python"
|
469 |
+
category = "dev"
|
470 |
+
optional = false
|
471 |
+
python-versions = ">=3.7"
|
472 |
+
files = [
|
473 |
+
{file = "mypy-1.3.0-cp310-cp310-macosx_10_9_x86_64.whl", hash = "sha256:c1eb485cea53f4f5284e5baf92902cd0088b24984f4209e25981cc359d64448d"},
|
474 |
+
{file = "mypy-1.3.0-cp310-cp310-macosx_11_0_arm64.whl", hash = "sha256:4c99c3ecf223cf2952638da9cd82793d8f3c0c5fa8b6ae2b2d9ed1e1ff51ba85"},
|
475 |
+
{file = "mypy-1.3.0-cp310-cp310-manylinux_2_17_x86_64.manylinux2014_x86_64.whl", hash = "sha256:550a8b3a19bb6589679a7c3c31f64312e7ff482a816c96e0cecec9ad3a7564dd"},
|
476 |
+
{file = "mypy-1.3.0-cp310-cp310-musllinux_1_1_x86_64.whl", hash = "sha256:cbc07246253b9e3d7d74c9ff948cd0fd7a71afcc2b77c7f0a59c26e9395cb152"},
|
477 |
+
{file = "mypy-1.3.0-cp310-cp310-win_amd64.whl", hash = "sha256:a22435632710a4fcf8acf86cbd0d69f68ac389a3892cb23fbad176d1cddaf228"},
|
478 |
+
{file = "mypy-1.3.0-cp311-cp311-macosx_10_9_x86_64.whl", hash = "sha256:6e33bb8b2613614a33dff70565f4c803f889ebd2f859466e42b46e1df76018dd"},
|
479 |
+
{file = "mypy-1.3.0-cp311-cp311-macosx_11_0_arm64.whl", hash = "sha256:7d23370d2a6b7a71dc65d1266f9a34e4cde9e8e21511322415db4b26f46f6b8c"},
|
480 |
+
{file = "mypy-1.3.0-cp311-cp311-manylinux_2_17_x86_64.manylinux2014_x86_64.whl", hash = "sha256:658fe7b674769a0770d4b26cb4d6f005e88a442fe82446f020be8e5f5efb2fae"},
|
481 |
+
{file = "mypy-1.3.0-cp311-cp311-musllinux_1_1_x86_64.whl", hash = "sha256:6e42d29e324cdda61daaec2336c42512e59c7c375340bd202efa1fe0f7b8f8ca"},
|
482 |
+
{file = "mypy-1.3.0-cp311-cp311-win_amd64.whl", hash = "sha256:d0b6c62206e04061e27009481cb0ec966f7d6172b5b936f3ead3d74f29fe3dcf"},
|
483 |
+
{file = "mypy-1.3.0-cp37-cp37m-macosx_10_9_x86_64.whl", hash = "sha256:76ec771e2342f1b558c36d49900dfe81d140361dd0d2df6cd71b3db1be155409"},
|
484 |
+
{file = "mypy-1.3.0-cp37-cp37m-manylinux_2_17_x86_64.manylinux2014_x86_64.whl", hash = "sha256:ebc95f8386314272bbc817026f8ce8f4f0d2ef7ae44f947c4664efac9adec929"},
|
485 |
+
{file = "mypy-1.3.0-cp37-cp37m-musllinux_1_1_x86_64.whl", hash = "sha256:faff86aa10c1aa4a10e1a301de160f3d8fc8703b88c7e98de46b531ff1276a9a"},
|
486 |
+
{file = "mypy-1.3.0-cp37-cp37m-win_amd64.whl", hash = "sha256:8c5979d0deb27e0f4479bee18ea0f83732a893e81b78e62e2dda3e7e518c92ee"},
|
487 |
+
{file = "mypy-1.3.0-cp38-cp38-macosx_10_9_x86_64.whl", hash = "sha256:c5d2cc54175bab47011b09688b418db71403aefad07cbcd62d44010543fc143f"},
|
488 |
+
{file = "mypy-1.3.0-cp38-cp38-macosx_11_0_arm64.whl", hash = "sha256:87df44954c31d86df96c8bd6e80dfcd773473e877ac6176a8e29898bfb3501cb"},
|
489 |
+
{file = "mypy-1.3.0-cp38-cp38-manylinux_2_17_x86_64.manylinux2014_x86_64.whl", hash = "sha256:473117e310febe632ddf10e745a355714e771ffe534f06db40702775056614c4"},
|
490 |
+
{file = "mypy-1.3.0-cp38-cp38-musllinux_1_1_x86_64.whl", hash = "sha256:74bc9b6e0e79808bf8678d7678b2ae3736ea72d56eede3820bd3849823e7f305"},
|
491 |
+
{file = "mypy-1.3.0-cp38-cp38-win_amd64.whl", hash = "sha256:44797d031a41516fcf5cbfa652265bb994e53e51994c1bd649ffcd0c3a7eccbf"},
|
492 |
+
{file = "mypy-1.3.0-cp39-cp39-macosx_10_9_x86_64.whl", hash = "sha256:ddae0f39ca146972ff6bb4399f3b2943884a774b8771ea0a8f50e971f5ea5ba8"},
|
493 |
+
{file = "mypy-1.3.0-cp39-cp39-macosx_11_0_arm64.whl", hash = "sha256:1c4c42c60a8103ead4c1c060ac3cdd3ff01e18fddce6f1016e08939647a0e703"},
|
494 |
+
{file = "mypy-1.3.0-cp39-cp39-manylinux_2_17_x86_64.manylinux2014_x86_64.whl", hash = "sha256:e86c2c6852f62f8f2b24cb7a613ebe8e0c7dc1402c61d36a609174f63e0ff017"},
|
495 |
+
{file = "mypy-1.3.0-cp39-cp39-musllinux_1_1_x86_64.whl", hash = "sha256:f9dca1e257d4cc129517779226753dbefb4f2266c4eaad610fc15c6a7e14283e"},
|
496 |
+
{file = "mypy-1.3.0-cp39-cp39-win_amd64.whl", hash = "sha256:95d8d31a7713510685b05fbb18d6ac287a56c8f6554d88c19e73f724a445448a"},
|
497 |
+
{file = "mypy-1.3.0-py3-none-any.whl", hash = "sha256:a8763e72d5d9574d45ce5881962bc8e9046bf7b375b0abf031f3e6811732a897"},
|
498 |
+
{file = "mypy-1.3.0.tar.gz", hash = "sha256:e1f4d16e296f5135624b34e8fb741eb0eadedca90862405b1f1fde2040b9bd11"},
|
499 |
+
]
|
500 |
+
|
501 |
+
[package.dependencies]
|
502 |
+
mypy-extensions = ">=1.0.0"
|
503 |
+
tomli = {version = ">=1.1.0", markers = "python_version < \"3.11\""}
|
504 |
+
typing-extensions = ">=3.10"
|
505 |
+
|
506 |
+
[package.extras]
|
507 |
+
dmypy = ["psutil (>=4.0)"]
|
508 |
+
install-types = ["pip"]
|
509 |
+
python2 = ["typed-ast (>=1.4.0,<2)"]
|
510 |
+
reports = ["lxml"]
|
511 |
+
|
512 |
+
[[package]]
|
513 |
+
name = "mypy-extensions"
|
514 |
+
version = "1.0.0"
|
515 |
+
description = "Type system extensions for programs checked with the mypy type checker."
|
516 |
+
category = "dev"
|
517 |
+
optional = false
|
518 |
+
python-versions = ">=3.5"
|
519 |
+
files = [
|
520 |
+
{file = "mypy_extensions-1.0.0-py3-none-any.whl", hash = "sha256:4392f6c0eb8a5668a69e23d168ffa70f0be9ccfd32b5cc2d26a34ae5b844552d"},
|
521 |
+
{file = "mypy_extensions-1.0.0.tar.gz", hash = "sha256:75dbf8955dc00442a438fc4d0666508a9a97b6bd41aa2f0ffe9d2f2725af0782"},
|
522 |
+
]
|
523 |
+
|
524 |
+
[[package]]
|
525 |
+
name = "packaging"
|
526 |
+
version = "23.1"
|
527 |
+
description = "Core utilities for Python packages"
|
528 |
+
category = "main"
|
529 |
+
optional = false
|
530 |
+
python-versions = ">=3.7"
|
531 |
+
files = [
|
532 |
+
{file = "packaging-23.1-py3-none-any.whl", hash = "sha256:994793af429502c4ea2ebf6bf664629d07c1a9fe974af92966e4b8d2df7edc61"},
|
533 |
+
{file = "packaging-23.1.tar.gz", hash = "sha256:a392980d2b6cffa644431898be54b0045151319d1e7ec34f0cfed48767dd334f"},
|
534 |
+
]
|
535 |
+
|
536 |
+
[[package]]
|
537 |
+
name = "pathspec"
|
538 |
+
version = "0.11.1"
|
539 |
+
description = "Utility library for gitignore style pattern matching of file paths."
|
540 |
+
category = "dev"
|
541 |
+
optional = false
|
542 |
+
python-versions = ">=3.7"
|
543 |
+
files = [
|
544 |
+
{file = "pathspec-0.11.1-py3-none-any.whl", hash = "sha256:d8af70af76652554bd134c22b3e8a1cc46ed7d91edcdd721ef1a0c51a84a5293"},
|
545 |
+
{file = "pathspec-0.11.1.tar.gz", hash = "sha256:2798de800fa92780e33acca925945e9a19a133b715067cf165b8866c15a31687"},
|
546 |
+
]
|
547 |
+
|
548 |
+
[[package]]
|
549 |
+
name = "platformdirs"
|
550 |
+
version = "3.5.0"
|
551 |
+
description = "A small Python package for determining appropriate platform-specific dirs, e.g. a \"user data dir\"."
|
552 |
+
category = "dev"
|
553 |
+
optional = false
|
554 |
+
python-versions = ">=3.7"
|
555 |
+
files = [
|
556 |
+
{file = "platformdirs-3.5.0-py3-none-any.whl", hash = "sha256:47692bc24c1958e8b0f13dd727307cff1db103fca36399f457da8e05f222fdc4"},
|
557 |
+
{file = "platformdirs-3.5.0.tar.gz", hash = "sha256:7954a68d0ba23558d753f73437c55f89027cf8f5108c19844d4b82e5af396335"},
|
558 |
+
]
|
559 |
+
|
560 |
+
[package.extras]
|
561 |
+
docs = ["furo (>=2023.3.27)", "proselint (>=0.13)", "sphinx (>=6.1.3)", "sphinx-autodoc-typehints (>=1.23,!=1.23.4)"]
|
562 |
+
test = ["appdirs (==1.4.4)", "covdefaults (>=2.3)", "pytest (>=7.3.1)", "pytest-cov (>=4)", "pytest-mock (>=3.10)"]
|
563 |
+
|
564 |
+
[[package]]
|
565 |
+
name = "pluggy"
|
566 |
+
version = "1.0.0"
|
567 |
+
description = "plugin and hook calling mechanisms for python"
|
568 |
+
category = "dev"
|
569 |
+
optional = false
|
570 |
+
python-versions = ">=3.6"
|
571 |
+
files = [
|
572 |
+
{file = "pluggy-1.0.0-py2.py3-none-any.whl", hash = "sha256:74134bbf457f031a36d68416e1509f34bd5ccc019f0bcc952c7b909d06b37bd3"},
|
573 |
+
{file = "pluggy-1.0.0.tar.gz", hash = "sha256:4224373bacce55f955a878bf9cfa763c1e360858e330072059e10bad68531159"},
|
574 |
+
]
|
575 |
+
|
576 |
+
[package.extras]
|
577 |
+
dev = ["pre-commit", "tox"]
|
578 |
+
testing = ["pytest", "pytest-benchmark"]
|
579 |
+
|
580 |
+
[[package]]
|
581 |
+
name = "pycodestyle"
|
582 |
+
version = "2.9.1"
|
583 |
+
description = "Python style guide checker"
|
584 |
+
category = "dev"
|
585 |
+
optional = false
|
586 |
+
python-versions = ">=3.6"
|
587 |
+
files = [
|
588 |
+
{file = "pycodestyle-2.9.1-py2.py3-none-any.whl", hash = "sha256:d1735fc58b418fd7c5f658d28d943854f8a849b01a5d0a1e6f3f3fdd0166804b"},
|
589 |
+
{file = "pycodestyle-2.9.1.tar.gz", hash = "sha256:2c9607871d58c76354b697b42f5d57e1ada7d261c261efac224b664affdc5785"},
|
590 |
+
]
|
591 |
+
|
592 |
+
[[package]]
|
593 |
+
name = "pyflakes"
|
594 |
+
version = "2.5.0"
|
595 |
+
description = "passive checker of Python programs"
|
596 |
+
category = "dev"
|
597 |
+
optional = false
|
598 |
+
python-versions = ">=3.6"
|
599 |
+
files = [
|
600 |
+
{file = "pyflakes-2.5.0-py2.py3-none-any.whl", hash = "sha256:4579f67d887f804e67edb544428f264b7b24f435b263c4614f384135cea553d2"},
|
601 |
+
{file = "pyflakes-2.5.0.tar.gz", hash = "sha256:491feb020dca48ccc562a8c0cbe8df07ee13078df59813b83959cbdada312ea3"},
|
602 |
+
]
|
603 |
+
|
604 |
+
[[package]]
|
605 |
+
name = "pytest"
|
606 |
+
version = "7.3.1"
|
607 |
+
description = "pytest: simple powerful testing with Python"
|
608 |
+
category = "dev"
|
609 |
+
optional = false
|
610 |
+
python-versions = ">=3.7"
|
611 |
+
files = [
|
612 |
+
{file = "pytest-7.3.1-py3-none-any.whl", hash = "sha256:3799fa815351fea3a5e96ac7e503a96fa51cc9942c3753cda7651b93c1cfa362"},
|
613 |
+
{file = "pytest-7.3.1.tar.gz", hash = "sha256:434afafd78b1d78ed0addf160ad2b77a30d35d4bdf8af234fe621919d9ed15e3"},
|
614 |
+
]
|
615 |
+
|
616 |
+
[package.dependencies]
|
617 |
+
colorama = {version = "*", markers = "sys_platform == \"win32\""}
|
618 |
+
exceptiongroup = {version = ">=1.0.0rc8", markers = "python_version < \"3.11\""}
|
619 |
+
iniconfig = "*"
|
620 |
+
packaging = "*"
|
621 |
+
pluggy = ">=0.12,<2.0"
|
622 |
+
tomli = {version = ">=1.0.0", markers = "python_version < \"3.11\""}
|
623 |
+
|
624 |
+
[package.extras]
|
625 |
+
testing = ["argcomplete", "attrs (>=19.2.0)", "hypothesis (>=3.56)", "mock", "nose", "pygments (>=2.7.2)", "requests", "xmlschema"]
|
626 |
+
|
627 |
+
[[package]]
|
628 |
+
name = "pytest-asyncio"
|
629 |
+
version = "0.21.0"
|
630 |
+
description = "Pytest support for asyncio"
|
631 |
+
category = "dev"
|
632 |
+
optional = false
|
633 |
+
python-versions = ">=3.7"
|
634 |
+
files = [
|
635 |
+
{file = "pytest-asyncio-0.21.0.tar.gz", hash = "sha256:2b38a496aef56f56b0e87557ec313e11e1ab9276fc3863f6a7be0f1d0e415e1b"},
|
636 |
+
{file = "pytest_asyncio-0.21.0-py3-none-any.whl", hash = "sha256:f2b3366b7cd501a4056858bd39349d5af19742aed2d81660b7998b6341c7eb9c"},
|
637 |
+
]
|
638 |
+
|
639 |
+
[package.dependencies]
|
640 |
+
pytest = ">=7.0.0"
|
641 |
+
|
642 |
+
[package.extras]
|
643 |
+
docs = ["sphinx (>=5.3)", "sphinx-rtd-theme (>=1.0)"]
|
644 |
+
testing = ["coverage (>=6.2)", "flaky (>=3.5.0)", "hypothesis (>=5.7.1)", "mypy (>=0.931)", "pytest-trio (>=0.7.0)"]
|
645 |
+
|
646 |
+
[[package]]
|
647 |
+
name = "pyyaml"
|
648 |
+
version = "6.0"
|
649 |
+
description = "YAML parser and emitter for Python"
|
650 |
+
category = "main"
|
651 |
+
optional = false
|
652 |
+
python-versions = ">=3.6"
|
653 |
+
files = [
|
654 |
+
{file = "PyYAML-6.0-cp310-cp310-macosx_10_9_x86_64.whl", hash = "sha256:d4db7c7aef085872ef65a8fd7d6d09a14ae91f691dec3e87ee5ee0539d516f53"},
|
655 |
+
{file = "PyYAML-6.0-cp310-cp310-macosx_11_0_arm64.whl", hash = "sha256:9df7ed3b3d2e0ecfe09e14741b857df43adb5a3ddadc919a2d94fbdf78fea53c"},
|
656 |
+
{file = "PyYAML-6.0-cp310-cp310-manylinux_2_17_aarch64.manylinux2014_aarch64.whl", hash = "sha256:77f396e6ef4c73fdc33a9157446466f1cff553d979bd00ecb64385760c6babdc"},
|
657 |
+
{file = "PyYAML-6.0-cp310-cp310-manylinux_2_17_s390x.manylinux2014_s390x.whl", hash = "sha256:a80a78046a72361de73f8f395f1f1e49f956c6be882eed58505a15f3e430962b"},
|
658 |
+
{file = "PyYAML-6.0-cp310-cp310-manylinux_2_5_x86_64.manylinux1_x86_64.manylinux_2_12_x86_64.manylinux2010_x86_64.whl", hash = "sha256:f84fbc98b019fef2ee9a1cb3ce93e3187a6df0b2538a651bfb890254ba9f90b5"},
|
659 |
+
{file = "PyYAML-6.0-cp310-cp310-win32.whl", hash = "sha256:2cd5df3de48857ed0544b34e2d40e9fac445930039f3cfe4bcc592a1f836d513"},
|
660 |
+
{file = "PyYAML-6.0-cp310-cp310-win_amd64.whl", hash = "sha256:daf496c58a8c52083df09b80c860005194014c3698698d1a57cbcfa182142a3a"},
|
661 |
+
{file = "PyYAML-6.0-cp311-cp311-macosx_10_9_x86_64.whl", hash = "sha256:d4b0ba9512519522b118090257be113b9468d804b19d63c71dbcf4a48fa32358"},
|
662 |
+
{file = "PyYAML-6.0-cp311-cp311-macosx_11_0_arm64.whl", hash = "sha256:81957921f441d50af23654aa6c5e5eaf9b06aba7f0a19c18a538dc7ef291c5a1"},
|
663 |
+
{file = "PyYAML-6.0-cp311-cp311-manylinux_2_17_aarch64.manylinux2014_aarch64.whl", hash = "sha256:afa17f5bc4d1b10afd4466fd3a44dc0e245382deca5b3c353d8b757f9e3ecb8d"},
|
664 |
+
{file = "PyYAML-6.0-cp311-cp311-manylinux_2_17_s390x.manylinux2014_s390x.whl", hash = "sha256:dbad0e9d368bb989f4515da330b88a057617d16b6a8245084f1b05400f24609f"},
|
665 |
+
{file = "PyYAML-6.0-cp311-cp311-manylinux_2_17_x86_64.manylinux2014_x86_64.whl", hash = "sha256:432557aa2c09802be39460360ddffd48156e30721f5e8d917f01d31694216782"},
|
666 |
+
{file = "PyYAML-6.0-cp311-cp311-win32.whl", hash = "sha256:bfaef573a63ba8923503d27530362590ff4f576c626d86a9fed95822a8255fd7"},
|
667 |
+
{file = "PyYAML-6.0-cp311-cp311-win_amd64.whl", hash = "sha256:01b45c0191e6d66c470b6cf1b9531a771a83c1c4208272ead47a3ae4f2f603bf"},
|
668 |
+
{file = "PyYAML-6.0-cp36-cp36m-macosx_10_9_x86_64.whl", hash = "sha256:897b80890765f037df3403d22bab41627ca8811ae55e9a722fd0392850ec4d86"},
|
669 |
+
{file = "PyYAML-6.0-cp36-cp36m-manylinux_2_17_aarch64.manylinux2014_aarch64.whl", hash = "sha256:50602afada6d6cbfad699b0c7bb50d5ccffa7e46a3d738092afddc1f9758427f"},
|
670 |
+
{file = "PyYAML-6.0-cp36-cp36m-manylinux_2_17_s390x.manylinux2014_s390x.whl", hash = "sha256:48c346915c114f5fdb3ead70312bd042a953a8ce5c7106d5bfb1a5254e47da92"},
|
671 |
+
{file = "PyYAML-6.0-cp36-cp36m-manylinux_2_5_x86_64.manylinux1_x86_64.manylinux_2_12_x86_64.manylinux2010_x86_64.whl", hash = "sha256:98c4d36e99714e55cfbaaee6dd5badbc9a1ec339ebfc3b1f52e293aee6bb71a4"},
|
672 |
+
{file = "PyYAML-6.0-cp36-cp36m-win32.whl", hash = "sha256:0283c35a6a9fbf047493e3a0ce8d79ef5030852c51e9d911a27badfde0605293"},
|
673 |
+
{file = "PyYAML-6.0-cp36-cp36m-win_amd64.whl", hash = "sha256:07751360502caac1c067a8132d150cf3d61339af5691fe9e87803040dbc5db57"},
|
674 |
+
{file = "PyYAML-6.0-cp37-cp37m-macosx_10_9_x86_64.whl", hash = "sha256:819b3830a1543db06c4d4b865e70ded25be52a2e0631ccd2f6a47a2822f2fd7c"},
|
675 |
+
{file = "PyYAML-6.0-cp37-cp37m-manylinux_2_17_aarch64.manylinux2014_aarch64.whl", hash = "sha256:473f9edb243cb1935ab5a084eb238d842fb8f404ed2193a915d1784b5a6b5fc0"},
|
676 |
+
{file = "PyYAML-6.0-cp37-cp37m-manylinux_2_17_s390x.manylinux2014_s390x.whl", hash = "sha256:0ce82d761c532fe4ec3f87fc45688bdd3a4c1dc5e0b4a19814b9009a29baefd4"},
|
677 |
+
{file = "PyYAML-6.0-cp37-cp37m-manylinux_2_5_x86_64.manylinux1_x86_64.manylinux_2_12_x86_64.manylinux2010_x86_64.whl", hash = "sha256:231710d57adfd809ef5d34183b8ed1eeae3f76459c18fb4a0b373ad56bedcdd9"},
|
678 |
+
{file = "PyYAML-6.0-cp37-cp37m-win32.whl", hash = "sha256:c5687b8d43cf58545ade1fe3e055f70eac7a5a1a0bf42824308d868289a95737"},
|
679 |
+
{file = "PyYAML-6.0-cp37-cp37m-win_amd64.whl", hash = "sha256:d15a181d1ecd0d4270dc32edb46f7cb7733c7c508857278d3d378d14d606db2d"},
|
680 |
+
{file = "PyYAML-6.0-cp38-cp38-macosx_10_9_x86_64.whl", hash = "sha256:0b4624f379dab24d3725ffde76559cff63d9ec94e1736b556dacdfebe5ab6d4b"},
|
681 |
+
{file = "PyYAML-6.0-cp38-cp38-manylinux_2_17_aarch64.manylinux2014_aarch64.whl", hash = "sha256:213c60cd50106436cc818accf5baa1aba61c0189ff610f64f4a3e8c6726218ba"},
|
682 |
+
{file = "PyYAML-6.0-cp38-cp38-manylinux_2_17_s390x.manylinux2014_s390x.whl", hash = "sha256:9fa600030013c4de8165339db93d182b9431076eb98eb40ee068700c9c813e34"},
|
683 |
+
{file = "PyYAML-6.0-cp38-cp38-manylinux_2_5_x86_64.manylinux1_x86_64.manylinux_2_12_x86_64.manylinux2010_x86_64.whl", hash = "sha256:277a0ef2981ca40581a47093e9e2d13b3f1fbbeffae064c1d21bfceba2030287"},
|
684 |
+
{file = "PyYAML-6.0-cp38-cp38-win32.whl", hash = "sha256:d4eccecf9adf6fbcc6861a38015c2a64f38b9d94838ac1810a9023a0609e1b78"},
|
685 |
+
{file = "PyYAML-6.0-cp38-cp38-win_amd64.whl", hash = "sha256:1e4747bc279b4f613a09eb64bba2ba602d8a6664c6ce6396a4d0cd413a50ce07"},
|
686 |
+
{file = "PyYAML-6.0-cp39-cp39-macosx_10_9_x86_64.whl", hash = "sha256:055d937d65826939cb044fc8c9b08889e8c743fdc6a32b33e2390f66013e449b"},
|
687 |
+
{file = "PyYAML-6.0-cp39-cp39-macosx_11_0_arm64.whl", hash = "sha256:e61ceaab6f49fb8bdfaa0f92c4b57bcfbea54c09277b1b4f7ac376bfb7a7c174"},
|
688 |
+
{file = "PyYAML-6.0-cp39-cp39-manylinux_2_17_aarch64.manylinux2014_aarch64.whl", hash = "sha256:d67d839ede4ed1b28a4e8909735fc992a923cdb84e618544973d7dfc71540803"},
|
689 |
+
{file = "PyYAML-6.0-cp39-cp39-manylinux_2_17_s390x.manylinux2014_s390x.whl", hash = "sha256:cba8c411ef271aa037d7357a2bc8f9ee8b58b9965831d9e51baf703280dc73d3"},
|
690 |
+
{file = "PyYAML-6.0-cp39-cp39-manylinux_2_5_x86_64.manylinux1_x86_64.manylinux_2_12_x86_64.manylinux2010_x86_64.whl", hash = "sha256:40527857252b61eacd1d9af500c3337ba8deb8fc298940291486c465c8b46ec0"},
|
691 |
+
{file = "PyYAML-6.0-cp39-cp39-win32.whl", hash = "sha256:b5b9eccad747aabaaffbc6064800670f0c297e52c12754eb1d976c57e4f74dcb"},
|
692 |
+
{file = "PyYAML-6.0-cp39-cp39-win_amd64.whl", hash = "sha256:b3d267842bf12586ba6c734f89d1f5b871df0273157918b0ccefa29deb05c21c"},
|
693 |
+
{file = "PyYAML-6.0.tar.gz", hash = "sha256:68fb519c14306fec9720a2a5b45bc9f0c8d1b9c72adf45c37baedfcd949c35a2"},
|
694 |
+
]
|
695 |
+
|
696 |
+
[[package]]
|
697 |
+
name = "requests"
|
698 |
+
version = "2.30.0"
|
699 |
+
description = "Python HTTP for Humans."
|
700 |
+
category = "main"
|
701 |
+
optional = false
|
702 |
+
python-versions = ">=3.7"
|
703 |
+
files = [
|
704 |
+
{file = "requests-2.30.0-py3-none-any.whl", hash = "sha256:10e94cc4f3121ee6da529d358cdaeaff2f1c409cd377dbc72b825852f2f7e294"},
|
705 |
+
{file = "requests-2.30.0.tar.gz", hash = "sha256:239d7d4458afcb28a692cdd298d87542235f4ca8d36d03a15bfc128a6559a2f4"},
|
706 |
+
]
|
707 |
+
|
708 |
+
[package.dependencies]
|
709 |
+
certifi = ">=2017.4.17"
|
710 |
+
charset-normalizer = ">=2,<4"
|
711 |
+
idna = ">=2.5,<4"
|
712 |
+
urllib3 = ">=1.21.1,<3"
|
713 |
+
|
714 |
+
[package.extras]
|
715 |
+
socks = ["PySocks (>=1.5.6,!=1.5.7)"]
|
716 |
+
use-chardet-on-py3 = ["chardet (>=3.0.2,<6)"]
|
717 |
+
|
718 |
+
[[package]]
|
719 |
+
name = "sniffio"
|
720 |
+
version = "1.3.0"
|
721 |
+
description = "Sniff out which async library your code is running under"
|
722 |
+
category = "main"
|
723 |
+
optional = false
|
724 |
+
python-versions = ">=3.7"
|
725 |
+
files = [
|
726 |
+
{file = "sniffio-1.3.0-py3-none-any.whl", hash = "sha256:eecefdce1e5bbfb7ad2eeaabf7c1eeb404d7757c379bd1f7e5cce9d8bf425384"},
|
727 |
+
{file = "sniffio-1.3.0.tar.gz", hash = "sha256:e60305c5e5d314f5389259b7f22aaa33d8f7dee49763119234af3755c55b9101"},
|
728 |
+
]
|
729 |
+
|
730 |
+
[[package]]
|
731 |
+
name = "tomli"
|
732 |
+
version = "2.0.1"
|
733 |
+
description = "A lil' TOML parser"
|
734 |
+
category = "dev"
|
735 |
+
optional = false
|
736 |
+
python-versions = ">=3.7"
|
737 |
+
files = [
|
738 |
+
{file = "tomli-2.0.1-py3-none-any.whl", hash = "sha256:939de3e7a6161af0c887ef91b7d41a53e7c5a1ca976325f429cb46ea9bc30ecc"},
|
739 |
+
{file = "tomli-2.0.1.tar.gz", hash = "sha256:de526c12914f0c550d15924c62d72abc48d6fe7364aa87328337a31007fe8a4f"},
|
740 |
+
]
|
741 |
+
|
742 |
+
[[package]]
|
743 |
+
name = "tqdm"
|
744 |
+
version = "4.65.0"
|
745 |
+
description = "Fast, Extensible Progress Meter"
|
746 |
+
category = "main"
|
747 |
+
optional = false
|
748 |
+
python-versions = ">=3.7"
|
749 |
+
files = [
|
750 |
+
{file = "tqdm-4.65.0-py3-none-any.whl", hash = "sha256:c4f53a17fe37e132815abceec022631be8ffe1b9381c2e6e30aa70edc99e9671"},
|
751 |
+
{file = "tqdm-4.65.0.tar.gz", hash = "sha256:1871fb68a86b8fb3b59ca4cdd3dcccbc7e6d613eeed31f4c332531977b89beb5"},
|
752 |
+
]
|
753 |
+
|
754 |
+
[package.dependencies]
|
755 |
+
colorama = {version = "*", markers = "platform_system == \"Windows\""}
|
756 |
+
|
757 |
+
[package.extras]
|
758 |
+
dev = ["py-make (>=0.1.0)", "twine", "wheel"]
|
759 |
+
notebook = ["ipywidgets (>=6)"]
|
760 |
+
slack = ["slack-sdk"]
|
761 |
+
telegram = ["requests"]
|
762 |
+
|
763 |
+
[[package]]
|
764 |
+
name = "typing-extensions"
|
765 |
+
version = "4.5.0"
|
766 |
+
description = "Backported and Experimental Type Hints for Python 3.7+"
|
767 |
+
category = "main"
|
768 |
+
optional = false
|
769 |
+
python-versions = ">=3.7"
|
770 |
+
files = [
|
771 |
+
{file = "typing_extensions-4.5.0-py3-none-any.whl", hash = "sha256:fb33085c39dd998ac16d1431ebc293a8b3eedd00fd4a32de0ff79002c19511b4"},
|
772 |
+
{file = "typing_extensions-4.5.0.tar.gz", hash = "sha256:5cb5f4a79139d699607b3ef622a1dedafa84e115ab0024e0d9c044a9479ca7cb"},
|
773 |
+
]
|
774 |
+
|
775 |
+
[[package]]
|
776 |
+
name = "urllib3"
|
777 |
+
version = "2.0.2"
|
778 |
+
description = "HTTP library with thread-safe connection pooling, file post, and more."
|
779 |
+
category = "main"
|
780 |
+
optional = false
|
781 |
+
python-versions = ">=3.7"
|
782 |
+
files = [
|
783 |
+
{file = "urllib3-2.0.2-py3-none-any.whl", hash = "sha256:d055c2f9d38dc53c808f6fdc8eab7360b6fdbbde02340ed25cfbcd817c62469e"},
|
784 |
+
{file = "urllib3-2.0.2.tar.gz", hash = "sha256:61717a1095d7e155cdb737ac7bb2f4324a858a1e2e6466f6d03ff630ca68d3cc"},
|
785 |
+
]
|
786 |
+
|
787 |
+
[package.extras]
|
788 |
+
brotli = ["brotli (>=1.0.9)", "brotlicffi (>=0.8.0)"]
|
789 |
+
secure = ["certifi", "cryptography (>=1.9)", "idna (>=2.0.0)", "pyopenssl (>=17.1.0)", "urllib3-secure-extra"]
|
790 |
+
socks = ["pysocks (>=1.5.6,!=1.5.7,<2.0)"]
|
791 |
+
zstd = ["zstandard (>=0.18.0)"]
|
792 |
+
|
793 |
+
[[package]]
|
794 |
+
name = "websockets"
|
795 |
+
version = "11.0.3"
|
796 |
+
description = "An implementation of the WebSocket Protocol (RFC 6455 & 7692)"
|
797 |
+
category = "main"
|
798 |
+
optional = false
|
799 |
+
python-versions = ">=3.7"
|
800 |
+
files = [
|
801 |
+
{file = "websockets-11.0.3-cp310-cp310-macosx_10_9_universal2.whl", hash = "sha256:3ccc8a0c387629aec40f2fc9fdcb4b9d5431954f934da3eaf16cdc94f67dbfac"},
|
802 |
+
{file = "websockets-11.0.3-cp310-cp310-macosx_10_9_x86_64.whl", hash = "sha256:d67ac60a307f760c6e65dad586f556dde58e683fab03323221a4e530ead6f74d"},
|
803 |
+
{file = "websockets-11.0.3-cp310-cp310-macosx_11_0_arm64.whl", hash = "sha256:84d27a4832cc1a0ee07cdcf2b0629a8a72db73f4cf6de6f0904f6661227f256f"},
|
804 |
+
{file = "websockets-11.0.3-cp310-cp310-manylinux_2_17_aarch64.manylinux2014_aarch64.whl", hash = "sha256:ffd7dcaf744f25f82190856bc26ed81721508fc5cbf2a330751e135ff1283564"},
|
805 |
+
{file = "websockets-11.0.3-cp310-cp310-manylinux_2_5_i686.manylinux1_i686.manylinux_2_17_i686.manylinux2014_i686.whl", hash = "sha256:7622a89d696fc87af8e8d280d9b421db5133ef5b29d3f7a1ce9f1a7bf7fcfa11"},
|
806 |
+
{file = "websockets-11.0.3-cp310-cp310-manylinux_2_5_x86_64.manylinux1_x86_64.manylinux_2_17_x86_64.manylinux2014_x86_64.whl", hash = "sha256:bceab846bac555aff6427d060f2fcfff71042dba6f5fca7dc4f75cac815e57ca"},
|
807 |
+
{file = "websockets-11.0.3-cp310-cp310-musllinux_1_1_aarch64.whl", hash = "sha256:54c6e5b3d3a8936a4ab6870d46bdd6ec500ad62bde9e44462c32d18f1e9a8e54"},
|
808 |
+
{file = "websockets-11.0.3-cp310-cp310-musllinux_1_1_i686.whl", hash = "sha256:41f696ba95cd92dc047e46b41b26dd24518384749ed0d99bea0a941ca87404c4"},
|
809 |
+
{file = "websockets-11.0.3-cp310-cp310-musllinux_1_1_x86_64.whl", hash = "sha256:86d2a77fd490ae3ff6fae1c6ceaecad063d3cc2320b44377efdde79880e11526"},
|
810 |
+
{file = "websockets-11.0.3-cp310-cp310-win32.whl", hash = "sha256:2d903ad4419f5b472de90cd2d40384573b25da71e33519a67797de17ef849b69"},
|
811 |
+
{file = "websockets-11.0.3-cp310-cp310-win_amd64.whl", hash = "sha256:1d2256283fa4b7f4c7d7d3e84dc2ece74d341bce57d5b9bf385df109c2a1a82f"},
|
812 |
+
{file = "websockets-11.0.3-cp311-cp311-macosx_10_9_universal2.whl", hash = "sha256:e848f46a58b9fcf3d06061d17be388caf70ea5b8cc3466251963c8345e13f7eb"},
|
813 |
+
{file = "websockets-11.0.3-cp311-cp311-macosx_10_9_x86_64.whl", hash = "sha256:aa5003845cdd21ac0dc6c9bf661c5beddd01116f6eb9eb3c8e272353d45b3288"},
|
814 |
+
{file = "websockets-11.0.3-cp311-cp311-macosx_11_0_arm64.whl", hash = "sha256:b58cbf0697721120866820b89f93659abc31c1e876bf20d0b3d03cef14faf84d"},
|
815 |
+
{file = "websockets-11.0.3-cp311-cp311-manylinux_2_17_aarch64.manylinux2014_aarch64.whl", hash = "sha256:660e2d9068d2bedc0912af508f30bbeb505bbbf9774d98def45f68278cea20d3"},
|
816 |
+
{file = "websockets-11.0.3-cp311-cp311-manylinux_2_5_i686.manylinux1_i686.manylinux_2_17_i686.manylinux2014_i686.whl", hash = "sha256:c1f0524f203e3bd35149f12157438f406eff2e4fb30f71221c8a5eceb3617b6b"},
|
817 |
+
{file = "websockets-11.0.3-cp311-cp311-manylinux_2_5_x86_64.manylinux1_x86_64.manylinux_2_17_x86_64.manylinux2014_x86_64.whl", hash = "sha256:def07915168ac8f7853812cc593c71185a16216e9e4fa886358a17ed0fd9fcf6"},
|
818 |
+
{file = "websockets-11.0.3-cp311-cp311-musllinux_1_1_aarch64.whl", hash = "sha256:b30c6590146e53149f04e85a6e4fcae068df4289e31e4aee1fdf56a0dead8f97"},
|
819 |
+
{file = "websockets-11.0.3-cp311-cp311-musllinux_1_1_i686.whl", hash = "sha256:619d9f06372b3a42bc29d0cd0354c9bb9fb39c2cbc1a9c5025b4538738dbffaf"},
|
820 |
+
{file = "websockets-11.0.3-cp311-cp311-musllinux_1_1_x86_64.whl", hash = "sha256:01f5567d9cf6f502d655151645d4e8b72b453413d3819d2b6f1185abc23e82dd"},
|
821 |
+
{file = "websockets-11.0.3-cp311-cp311-win32.whl", hash = "sha256:e1459677e5d12be8bbc7584c35b992eea142911a6236a3278b9b5ce3326f282c"},
|
822 |
+
{file = "websockets-11.0.3-cp311-cp311-win_amd64.whl", hash = "sha256:e7837cb169eca3b3ae94cc5787c4fed99eef74c0ab9506756eea335e0d6f3ed8"},
|
823 |
+
{file = "websockets-11.0.3-cp37-cp37m-macosx_10_9_x86_64.whl", hash = "sha256:9f59a3c656fef341a99e3d63189852be7084c0e54b75734cde571182c087b152"},
|
824 |
+
{file = "websockets-11.0.3-cp37-cp37m-manylinux_2_17_aarch64.manylinux2014_aarch64.whl", hash = "sha256:2529338a6ff0eb0b50c7be33dc3d0e456381157a31eefc561771ee431134a97f"},
|
825 |
+
{file = "websockets-11.0.3-cp37-cp37m-manylinux_2_5_i686.manylinux1_i686.manylinux_2_17_i686.manylinux2014_i686.whl", hash = "sha256:34fd59a4ac42dff6d4681d8843217137f6bc85ed29722f2f7222bd619d15e95b"},
|
826 |
+
{file = "websockets-11.0.3-cp37-cp37m-manylinux_2_5_x86_64.manylinux1_x86_64.manylinux_2_17_x86_64.manylinux2014_x86_64.whl", hash = "sha256:332d126167ddddec94597c2365537baf9ff62dfcc9db4266f263d455f2f031cb"},
|
827 |
+
{file = "websockets-11.0.3-cp37-cp37m-musllinux_1_1_aarch64.whl", hash = "sha256:6505c1b31274723ccaf5f515c1824a4ad2f0d191cec942666b3d0f3aa4cb4007"},
|
828 |
+
{file = "websockets-11.0.3-cp37-cp37m-musllinux_1_1_i686.whl", hash = "sha256:f467ba0050b7de85016b43f5a22b46383ef004c4f672148a8abf32bc999a87f0"},
|
829 |
+
{file = "websockets-11.0.3-cp37-cp37m-musllinux_1_1_x86_64.whl", hash = "sha256:9d9acd80072abcc98bd2c86c3c9cd4ac2347b5a5a0cae7ed5c0ee5675f86d9af"},
|
830 |
+
{file = "websockets-11.0.3-cp37-cp37m-win32.whl", hash = "sha256:e590228200fcfc7e9109509e4d9125eace2042fd52b595dd22bbc34bb282307f"},
|
831 |
+
{file = "websockets-11.0.3-cp37-cp37m-win_amd64.whl", hash = "sha256:b16fff62b45eccb9c7abb18e60e7e446998093cdcb50fed33134b9b6878836de"},
|
832 |
+
{file = "websockets-11.0.3-cp38-cp38-macosx_10_9_universal2.whl", hash = "sha256:fb06eea71a00a7af0ae6aefbb932fb8a7df3cb390cc217d51a9ad7343de1b8d0"},
|
833 |
+
{file = "websockets-11.0.3-cp38-cp38-macosx_10_9_x86_64.whl", hash = "sha256:8a34e13a62a59c871064dfd8ffb150867e54291e46d4a7cf11d02c94a5275bae"},
|
834 |
+
{file = "websockets-11.0.3-cp38-cp38-macosx_11_0_arm64.whl", hash = "sha256:4841ed00f1026dfbced6fca7d963c4e7043aa832648671b5138008dc5a8f6d99"},
|
835 |
+
{file = "websockets-11.0.3-cp38-cp38-manylinux_2_17_aarch64.manylinux2014_aarch64.whl", hash = "sha256:1a073fc9ab1c8aff37c99f11f1641e16da517770e31a37265d2755282a5d28aa"},
|
836 |
+
{file = "websockets-11.0.3-cp38-cp38-manylinux_2_5_i686.manylinux1_i686.manylinux_2_17_i686.manylinux2014_i686.whl", hash = "sha256:68b977f21ce443d6d378dbd5ca38621755f2063d6fdb3335bda981d552cfff86"},
|
837 |
+
{file = "websockets-11.0.3-cp38-cp38-manylinux_2_5_x86_64.manylinux1_x86_64.manylinux_2_17_x86_64.manylinux2014_x86_64.whl", hash = "sha256:e1a99a7a71631f0efe727c10edfba09ea6bee4166a6f9c19aafb6c0b5917d09c"},
|
838 |
+
{file = "websockets-11.0.3-cp38-cp38-musllinux_1_1_aarch64.whl", hash = "sha256:bee9fcb41db2a23bed96c6b6ead6489702c12334ea20a297aa095ce6d31370d0"},
|
839 |
+
{file = "websockets-11.0.3-cp38-cp38-musllinux_1_1_i686.whl", hash = "sha256:4b253869ea05a5a073ebfdcb5cb3b0266a57c3764cf6fe114e4cd90f4bfa5f5e"},
|
840 |
+
{file = "websockets-11.0.3-cp38-cp38-musllinux_1_1_x86_64.whl", hash = "sha256:1553cb82942b2a74dd9b15a018dce645d4e68674de2ca31ff13ebc2d9f283788"},
|
841 |
+
{file = "websockets-11.0.3-cp38-cp38-win32.whl", hash = "sha256:f61bdb1df43dc9c131791fbc2355535f9024b9a04398d3bd0684fc16ab07df74"},
|
842 |
+
{file = "websockets-11.0.3-cp38-cp38-win_amd64.whl", hash = "sha256:03aae4edc0b1c68498f41a6772d80ac7c1e33c06c6ffa2ac1c27a07653e79d6f"},
|
843 |
+
{file = "websockets-11.0.3-cp39-cp39-macosx_10_9_universal2.whl", hash = "sha256:777354ee16f02f643a4c7f2b3eff8027a33c9861edc691a2003531f5da4f6bc8"},
|
844 |
+
{file = "websockets-11.0.3-cp39-cp39-macosx_10_9_x86_64.whl", hash = "sha256:8c82f11964f010053e13daafdc7154ce7385ecc538989a354ccc7067fd7028fd"},
|
845 |
+
{file = "websockets-11.0.3-cp39-cp39-macosx_11_0_arm64.whl", hash = "sha256:3580dd9c1ad0701169e4d6fc41e878ffe05e6bdcaf3c412f9d559389d0c9e016"},
|
846 |
+
{file = "websockets-11.0.3-cp39-cp39-manylinux_2_17_aarch64.manylinux2014_aarch64.whl", hash = "sha256:6f1a3f10f836fab6ca6efa97bb952300b20ae56b409414ca85bff2ad241d2a61"},
|
847 |
+
{file = "websockets-11.0.3-cp39-cp39-manylinux_2_5_i686.manylinux1_i686.manylinux_2_17_i686.manylinux2014_i686.whl", hash = "sha256:df41b9bc27c2c25b486bae7cf42fccdc52ff181c8c387bfd026624a491c2671b"},
|
848 |
+
{file = "websockets-11.0.3-cp39-cp39-manylinux_2_5_x86_64.manylinux1_x86_64.manylinux_2_17_x86_64.manylinux2014_x86_64.whl", hash = "sha256:279e5de4671e79a9ac877427f4ac4ce93751b8823f276b681d04b2156713b9dd"},
|
849 |
+
{file = "websockets-11.0.3-cp39-cp39-musllinux_1_1_aarch64.whl", hash = "sha256:1fdf26fa8a6a592f8f9235285b8affa72748dc12e964a5518c6c5e8f916716f7"},
|
850 |
+
{file = "websockets-11.0.3-cp39-cp39-musllinux_1_1_i686.whl", hash = "sha256:69269f3a0b472e91125b503d3c0b3566bda26da0a3261c49f0027eb6075086d1"},
|
851 |
+
{file = "websockets-11.0.3-cp39-cp39-musllinux_1_1_x86_64.whl", hash = "sha256:97b52894d948d2f6ea480171a27122d77af14ced35f62e5c892ca2fae9344311"},
|
852 |
+
{file = "websockets-11.0.3-cp39-cp39-win32.whl", hash = "sha256:c7f3cb904cce8e1be667c7e6fef4516b98d1a6a0635a58a57528d577ac18a128"},
|
853 |
+
{file = "websockets-11.0.3-cp39-cp39-win_amd64.whl", hash = "sha256:c792ea4eabc0159535608fc5658a74d1a81020eb35195dd63214dcf07556f67e"},
|
854 |
+
{file = "websockets-11.0.3-pp37-pypy37_pp73-macosx_10_9_x86_64.whl", hash = "sha256:f2e58f2c36cc52d41f2659e4c0cbf7353e28c8c9e63e30d8c6d3494dc9fdedcf"},
|
855 |
+
{file = "websockets-11.0.3-pp37-pypy37_pp73-manylinux_2_17_aarch64.manylinux2014_aarch64.whl", hash = "sha256:de36fe9c02995c7e6ae6efe2e205816f5f00c22fd1fbf343d4d18c3d5ceac2f5"},
|
856 |
+
{file = "websockets-11.0.3-pp37-pypy37_pp73-manylinux_2_5_i686.manylinux1_i686.manylinux_2_17_i686.manylinux2014_i686.whl", hash = "sha256:0ac56b661e60edd453585f4bd68eb6a29ae25b5184fd5ba51e97652580458998"},
|
857 |
+
{file = "websockets-11.0.3-pp37-pypy37_pp73-manylinux_2_5_x86_64.manylinux1_x86_64.manylinux_2_17_x86_64.manylinux2014_x86_64.whl", hash = "sha256:e052b8467dd07d4943936009f46ae5ce7b908ddcac3fda581656b1b19c083d9b"},
|
858 |
+
{file = "websockets-11.0.3-pp37-pypy37_pp73-win_amd64.whl", hash = "sha256:42cc5452a54a8e46a032521d7365da775823e21bfba2895fb7b77633cce031bb"},
|
859 |
+
{file = "websockets-11.0.3-pp38-pypy38_pp73-macosx_10_9_x86_64.whl", hash = "sha256:e6316827e3e79b7b8e7d8e3b08f4e331af91a48e794d5d8b099928b6f0b85f20"},
|
860 |
+
{file = "websockets-11.0.3-pp38-pypy38_pp73-manylinux_2_17_aarch64.manylinux2014_aarch64.whl", hash = "sha256:8531fdcad636d82c517b26a448dcfe62f720e1922b33c81ce695d0edb91eb931"},
|
861 |
+
{file = "websockets-11.0.3-pp38-pypy38_pp73-manylinux_2_5_i686.manylinux1_i686.manylinux_2_17_i686.manylinux2014_i686.whl", hash = "sha256:c114e8da9b475739dde229fd3bc6b05a6537a88a578358bc8eb29b4030fac9c9"},
|
862 |
+
{file = "websockets-11.0.3-pp38-pypy38_pp73-manylinux_2_5_x86_64.manylinux1_x86_64.manylinux_2_17_x86_64.manylinux2014_x86_64.whl", hash = "sha256:e063b1865974611313a3849d43f2c3f5368093691349cf3c7c8f8f75ad7cb280"},
|
863 |
+
{file = "websockets-11.0.3-pp38-pypy38_pp73-win_amd64.whl", hash = "sha256:92b2065d642bf8c0a82d59e59053dd2fdde64d4ed44efe4870fa816c1232647b"},
|
864 |
+
{file = "websockets-11.0.3-pp39-pypy39_pp73-macosx_10_9_x86_64.whl", hash = "sha256:0ee68fe502f9031f19d495dae2c268830df2760c0524cbac5d759921ba8c8e82"},
|
865 |
+
{file = "websockets-11.0.3-pp39-pypy39_pp73-manylinux_2_17_aarch64.manylinux2014_aarch64.whl", hash = "sha256:dcacf2c7a6c3a84e720d1bb2b543c675bf6c40e460300b628bab1b1efc7c034c"},
|
866 |
+
{file = "websockets-11.0.3-pp39-pypy39_pp73-manylinux_2_5_i686.manylinux1_i686.manylinux_2_17_i686.manylinux2014_i686.whl", hash = "sha256:b67c6f5e5a401fc56394f191f00f9b3811fe843ee93f4a70df3c389d1adf857d"},
|
867 |
+
{file = "websockets-11.0.3-pp39-pypy39_pp73-manylinux_2_5_x86_64.manylinux1_x86_64.manylinux_2_17_x86_64.manylinux2014_x86_64.whl", hash = "sha256:1d5023a4b6a5b183dc838808087033ec5df77580485fc533e7dab2567851b0a4"},
|
868 |
+
{file = "websockets-11.0.3-pp39-pypy39_pp73-win_amd64.whl", hash = "sha256:ed058398f55163a79bb9f06a90ef9ccc063b204bb346c4de78efc5d15abfe602"},
|
869 |
+
{file = "websockets-11.0.3-py3-none-any.whl", hash = "sha256:6681ba9e7f8f3b19440921e99efbb40fc89f26cd71bf539e45d8c8a25c976dc6"},
|
870 |
+
{file = "websockets-11.0.3.tar.gz", hash = "sha256:88fc51d9a26b10fc331be344f1781224a375b78488fc343620184e95a4b27016"},
|
871 |
+
]
|
872 |
+
|
873 |
+
[metadata]
|
874 |
+
lock-version = "2.0"
|
875 |
+
python-versions = "^3.8"
|
876 |
+
content-hash = "ddf46ff1beecd493f52ac6e719fe23967c38927d0f729029cc33ca47663669d8"
|
client/poetry.toml
ADDED
@@ -0,0 +1 @@
|
|
|
|
|
1 |
+
virtualenvs.in-project = true
|
client/pyproject.toml
ADDED
@@ -0,0 +1,39 @@
|
|
|
|
|
|
|
|
|
|
|
|
|
|
|
|
|
|
|
|
|
|
|
|
|
|
|
|
|
|
|
|
|
|
|
|
|
|
|
|
|
|
|
|
|
|
|
|
|
|
|
|
|
|
|
|
|
|
|
|
|
|
|
|
|
|
|
|
|
|
|
|
|
|
|
|
|
|
|
|
|
1 |
+
[tool.poetry]
|
2 |
+
name = "h2ogpt-client"
|
3 |
+
version = "0.1.0"
|
4 |
+
description = ""
|
5 |
+
authors = []
|
6 |
+
readme = "README.md"
|
7 |
+
packages = [{include = "h2ogpt_client"}]
|
8 |
+
|
9 |
+
[tool.poetry.dependencies]
|
10 |
+
python = "^3.8"
|
11 |
+
gradio-client = "^0.2.2"
|
12 |
+
|
13 |
+
[tool.poetry.group.test.dependencies]
|
14 |
+
pytest = "^7.3.1"
|
15 |
+
pytest-asyncio = "^0.21.0"
|
16 |
+
|
17 |
+
[tool.poetry.group.dev.dependencies]
|
18 |
+
mypy = "^1.3.0"
|
19 |
+
black = "^23.3.0"
|
20 |
+
flake8 = "5.0.4"
|
21 |
+
isort = "^5.12.0"
|
22 |
+
flake8-pyproject = "^1.2.3"
|
23 |
+
|
24 |
+
[build-system]
|
25 |
+
requires = ["poetry-core"]
|
26 |
+
build-backend = "poetry.core.masonry.api"
|
27 |
+
|
28 |
+
[tool.isort]
|
29 |
+
profile = "black"
|
30 |
+
py_version = "auto"
|
31 |
+
|
32 |
+
[tool.flake8]
|
33 |
+
max-line-length = 88
|
34 |
+
|
35 |
+
[tool.mypy]
|
36 |
+
python_version = "3.8"
|
37 |
+
|
38 |
+
[tool.pytest.ini_options]
|
39 |
+
pythonpath = "h2ogpt_client"
|
client/tests/__init__.py
ADDED
File without changes
|
client/tests/test_client.py
ADDED
@@ -0,0 +1,55 @@
|
|
|
|
|
|
|
|
|
|
|
|
|
|
|
|
|
|
|
|
|
|
|
|
|
|
|
|
|
|
|
|
|
|
|
|
|
|
|
|
|
|
|
|
|
|
|
|
|
|
|
|
|
|
|
|
|
|
|
|
|
|
|
|
|
|
|
|
|
|
|
|
|
|
|
|
|
|
|
|
|
|
|
|
|
|
|
|
|
|
|
|
|
|
|
|
|
|
|
|
|
|
|
|
|
|
|
|
|
|
|
|
|
1 |
+
import os
|
2 |
+
|
3 |
+
from h2ogpt_client import Client
|
4 |
+
|
5 |
+
|
6 |
+
def create_client(server_url: str = "") -> Client:
|
7 |
+
server_url = server_url or os.getenv("H2OGPT_SERVER", "http://0.0.0.0:7860")
|
8 |
+
return Client(server_url)
|
9 |
+
|
10 |
+
|
11 |
+
def test_text_completion():
|
12 |
+
launch_server()
|
13 |
+
|
14 |
+
client = create_client()
|
15 |
+
r = client.text_completion.create("Hello world")
|
16 |
+
assert r
|
17 |
+
print(r)
|
18 |
+
|
19 |
+
|
20 |
+
async def test_text_completion_async():
|
21 |
+
launch_server()
|
22 |
+
|
23 |
+
client = create_client()
|
24 |
+
r = await client.text_completion.create_async("Hello world")
|
25 |
+
assert r
|
26 |
+
print(r)
|
27 |
+
|
28 |
+
|
29 |
+
def test_chat_completion():
|
30 |
+
launch_server()
|
31 |
+
|
32 |
+
client = create_client()
|
33 |
+
chat_context = client.chat_completion.create()
|
34 |
+
|
35 |
+
chat1 = chat_context.chat("Hey!")
|
36 |
+
assert chat1["user"] == "Hey!"
|
37 |
+
assert chat1["gpt"]
|
38 |
+
|
39 |
+
chat2 = chat_context.chat("How are you?")
|
40 |
+
assert chat2["user"] == "How are you?"
|
41 |
+
assert chat2["gpt"]
|
42 |
+
|
43 |
+
chat3 = chat_context.chat("Have a good day")
|
44 |
+
assert chat3["user"] == "Have a good day"
|
45 |
+
assert chat3["gpt"]
|
46 |
+
|
47 |
+
chat_history = chat_context.chat_history()
|
48 |
+
assert chat_history == [chat1, chat2, chat3]
|
49 |
+
print(chat_history)
|
50 |
+
|
51 |
+
|
52 |
+
def launch_server():
|
53 |
+
from generate import main
|
54 |
+
main(base_model='h2oai/h2ogpt-oig-oasst1-512-6_9b', prompt_type='human_bot', chat=False,
|
55 |
+
stream_output=False, gradio=True, num_beams=1, block_gradio_exit=False)
|
client_test.py
ADDED
@@ -0,0 +1,337 @@
|
|
|
|
|
|
|
|
|
|
|
|
|
|
|
|
|
|
|
|
|
|
|
|
|
|
|
|
|
|
|
|
|
|
|
|
|
|
|
|
|
|
|
|
|
|
|
|
|
|
|
|
|
|
|
|
|
|
|
|
|
|
|
|
|
|
|
|
|
|
|
|
|
|
|
|
|
|
|
|
|
|
|
|
|
|
|
|
|
|
|
|
|
|
|
|
|
|
|
|
|
|
|
|
|
|
|
|
|
|
|
|
|
|
|
|
|
|
|
|
|
|
|
|
|
|
|
|
|
|
|
|
|
|
|
|
|
|
|
|
|
|
|
|
|
|
|
|
|
|
|
|
|
|
|
|
|
|
|
|
|
|
|
|
|
|
|
|
|
|
|
|
|
|
|
|
|
|
|
|
|
|
|
|
|
|
|
|
|
|
|
|
|
|
|
|
|
|
|
|
|
|
|
|
|
|
|
|
|
|
|
|
|
|
|
|
|
|
|
|
|
|
|
|
|
|
|
|
|
|
|
|
|
|
|
|
|
|
|
|
|
|
|
|
|
|
|
|
|
|
|
|
|
|
|
|
|
|
|
|
|
|
|
|
|
|
|
|
|
|
|
|
|
|
|
|
|
|
|
|
|
|
|
|
|
|
|
|
|
|
|
|
|
|
|
|
|
|
|
|
|
|
|
|
|
|
|
|
|
|
|
|
|
|
|
|
|
|
|
|
|
|
|
|
|
|
|
|
|
|
|
|
|
|
|
|
|
|
|
|
|
|
|
|
|
|
|
|
|
|
|
|
|
|
|
|
|
|
|
|
|
|
|
|
|
|
|
|
|
|
|
|
|
|
|
|
|
|
|
|
|
|
|
|
|
|
|
|
|
|
|
|
|
|
|
|
|
|
|
|
|
|
|
|
|
|
|
|
|
|
|
|
|
|
|
|
|
|
|
|
|
|
|
|
|
|
|
|
|
|
|
|
|
|
|
|
|
|
|
|
|
|
|
|
|
|
|
|
|
|
|
|
|
|
|
|
|
|
|
|
|
|
|
|
|
|
|
|
|
|
|
|
|
|
|
|
|
|
|
|
|
|
|
|
|
|
|
|
|
|
|
|
|
|
|
|
|
|
|
|
|
|
|
|
|
|
|
|
|
|
|
|
|
|
|
|
|
|
|
|
|
|
|
|
|
|
|
|
|
|
|
|
|
|
|
|
|
|
|
|
|
|
|
|
|
|
|
|
|
|
|
|
|
|
|
|
|
|
|
|
|
|
|
|
|
|
|
|
|
|
|
|
|
|
|
|
|
|
|
|
|
|
|
|
|
|
|
|
|
|
|
|
|
|
|
|
|
|
|
|
|
|
|
|
|
|
|
|
|
|
|
|
|
|
|
|
|
|
|
|
|
|
|
|
|
|
|
|
|
|
|
|
|
|
|
|
|
|
|
|
|
|
|
|
|
|
|
|
|
|
|
|
|
|
|
|
|
|
|
|
|
|
|
|
|
|
|
|
|
|
|
|
|
|
|
|
|
1 |
+
"""
|
2 |
+
Client test.
|
3 |
+
|
4 |
+
Run server:
|
5 |
+
|
6 |
+
python generate.py --base_model=h2oai/h2ogpt-oig-oasst1-512-6_9b
|
7 |
+
|
8 |
+
NOTE: For private models, add --use-auth_token=True
|
9 |
+
|
10 |
+
NOTE: --infer_devices=True (default) must be used for multi-GPU in case see failures with cuda:x cuda:y mismatches.
|
11 |
+
Currently, this will force model to be on a single GPU.
|
12 |
+
|
13 |
+
Then run this client as:
|
14 |
+
|
15 |
+
python client_test.py
|
16 |
+
|
17 |
+
|
18 |
+
|
19 |
+
For HF spaces:
|
20 |
+
|
21 |
+
HOST="https://h2oai-h2ogpt-chatbot.hf.space" python client_test.py
|
22 |
+
|
23 |
+
Result:
|
24 |
+
|
25 |
+
Loaded as API: https://h2oai-h2ogpt-chatbot.hf.space ✔
|
26 |
+
{'instruction_nochat': 'Who are you?', 'iinput_nochat': '', 'response': 'I am h2oGPT, a large language model developed by LAION.', 'sources': ''}
|
27 |
+
|
28 |
+
|
29 |
+
For demo:
|
30 |
+
|
31 |
+
HOST="https://gpt.h2o.ai" python client_test.py
|
32 |
+
|
33 |
+
Result:
|
34 |
+
|
35 |
+
Loaded as API: https://gpt.h2o.ai ✔
|
36 |
+
{'instruction_nochat': 'Who are you?', 'iinput_nochat': '', 'response': 'I am h2oGPT, a chatbot created by LAION.', 'sources': ''}
|
37 |
+
|
38 |
+
NOTE: Raw output from API for nochat case is a string of a python dict and will remain so if other entries are added to dict:
|
39 |
+
|
40 |
+
{'response': "I'm h2oGPT, a large language model by H2O.ai, the visionary leader in democratizing AI.", 'sources': ''}
|
41 |
+
|
42 |
+
|
43 |
+
"""
|
44 |
+
import ast
|
45 |
+
import time
|
46 |
+
import os
|
47 |
+
import markdown # pip install markdown
|
48 |
+
import pytest
|
49 |
+
from bs4 import BeautifulSoup # pip install beautifulsoup4
|
50 |
+
|
51 |
+
from enums import DocumentChoices
|
52 |
+
|
53 |
+
debug = False
|
54 |
+
|
55 |
+
os.environ['HF_HUB_DISABLE_TELEMETRY'] = '1'
|
56 |
+
|
57 |
+
|
58 |
+
def get_client(serialize=True):
|
59 |
+
from gradio_client import Client
|
60 |
+
|
61 |
+
client = Client(os.getenv('HOST', "http://localhost:7860"), serialize=serialize)
|
62 |
+
if debug:
|
63 |
+
print(client.view_api(all_endpoints=True))
|
64 |
+
return client
|
65 |
+
|
66 |
+
|
67 |
+
def get_args(prompt, prompt_type, chat=False, stream_output=False,
|
68 |
+
max_new_tokens=50,
|
69 |
+
top_k_docs=3,
|
70 |
+
langchain_mode='Disabled', prompt_dict=''):
|
71 |
+
from collections import OrderedDict
|
72 |
+
kwargs = OrderedDict(instruction=prompt if chat else '', # only for chat=True
|
73 |
+
iinput='', # only for chat=True
|
74 |
+
context='',
|
75 |
+
# streaming output is supported, loops over and outputs each generation in streaming mode
|
76 |
+
# but leave stream_output=False for simple input/output mode
|
77 |
+
stream_output=stream_output,
|
78 |
+
prompt_type=prompt_type,
|
79 |
+
prompt_dict=prompt_dict,
|
80 |
+
temperature=0.1,
|
81 |
+
top_p=0.75,
|
82 |
+
top_k=40,
|
83 |
+
num_beams=1,
|
84 |
+
max_new_tokens=max_new_tokens,
|
85 |
+
min_new_tokens=0,
|
86 |
+
early_stopping=False,
|
87 |
+
max_time=20,
|
88 |
+
repetition_penalty=1.0,
|
89 |
+
num_return_sequences=1,
|
90 |
+
do_sample=True,
|
91 |
+
chat=chat,
|
92 |
+
instruction_nochat=prompt if not chat else '',
|
93 |
+
iinput_nochat='', # only for chat=False
|
94 |
+
langchain_mode=langchain_mode,
|
95 |
+
top_k_docs=top_k_docs,
|
96 |
+
chunk=True,
|
97 |
+
chunk_size=512,
|
98 |
+
document_choice=[DocumentChoices.All_Relevant.name],
|
99 |
+
)
|
100 |
+
from generate import eval_func_param_names
|
101 |
+
assert len(set(eval_func_param_names).difference(set(list(kwargs.keys())))) == 0
|
102 |
+
if chat:
|
103 |
+
# add chatbot output on end. Assumes serialize=False
|
104 |
+
kwargs.update(dict(chatbot=[]))
|
105 |
+
|
106 |
+
return kwargs, list(kwargs.values())
|
107 |
+
|
108 |
+
|
109 |
+
@pytest.mark.skip(reason="For manual use against some server, no server launched")
|
110 |
+
def test_client_basic(prompt_type='human_bot'):
|
111 |
+
return run_client_nochat(prompt='Who are you?', prompt_type=prompt_type, max_new_tokens=50)
|
112 |
+
|
113 |
+
|
114 |
+
def run_client_nochat(prompt, prompt_type, max_new_tokens):
|
115 |
+
kwargs, args = get_args(prompt, prompt_type, chat=False, max_new_tokens=max_new_tokens)
|
116 |
+
|
117 |
+
api_name = '/submit_nochat'
|
118 |
+
client = get_client(serialize=True)
|
119 |
+
res = client.predict(
|
120 |
+
*tuple(args),
|
121 |
+
api_name=api_name,
|
122 |
+
)
|
123 |
+
print("Raw client result: %s" % res, flush=True)
|
124 |
+
res_dict = dict(prompt=kwargs['instruction_nochat'], iinput=kwargs['iinput_nochat'],
|
125 |
+
response=md_to_text(res))
|
126 |
+
print(res_dict)
|
127 |
+
return res_dict, client
|
128 |
+
|
129 |
+
|
130 |
+
@pytest.mark.skip(reason="For manual use against some server, no server launched")
|
131 |
+
def test_client_basic_api(prompt_type='human_bot'):
|
132 |
+
return run_client_nochat_api(prompt='Who are you?', prompt_type=prompt_type, max_new_tokens=50)
|
133 |
+
|
134 |
+
|
135 |
+
def run_client_nochat_api(prompt, prompt_type, max_new_tokens):
|
136 |
+
kwargs, args = get_args(prompt, prompt_type, chat=False, max_new_tokens=max_new_tokens)
|
137 |
+
|
138 |
+
api_name = '/submit_nochat_api' # NOTE: like submit_nochat but stable API for string dict passing
|
139 |
+
client = get_client(serialize=True)
|
140 |
+
res = client.predict(
|
141 |
+
str(dict(kwargs)),
|
142 |
+
api_name=api_name,
|
143 |
+
)
|
144 |
+
print("Raw client result: %s" % res, flush=True)
|
145 |
+
res_dict = dict(prompt=kwargs['instruction_nochat'], iinput=kwargs['iinput_nochat'],
|
146 |
+
response=md_to_text(ast.literal_eval(res)['response']),
|
147 |
+
sources=ast.literal_eval(res)['sources'])
|
148 |
+
print(res_dict)
|
149 |
+
return res_dict, client
|
150 |
+
|
151 |
+
|
152 |
+
@pytest.mark.skip(reason="For manual use against some server, no server launched")
|
153 |
+
def test_client_basic_api_lean(prompt_type='human_bot'):
|
154 |
+
return run_client_nochat_api_lean(prompt='Who are you?', prompt_type=prompt_type, max_new_tokens=50)
|
155 |
+
|
156 |
+
|
157 |
+
def run_client_nochat_api_lean(prompt, prompt_type, max_new_tokens):
|
158 |
+
kwargs = dict(instruction_nochat=prompt)
|
159 |
+
|
160 |
+
api_name = '/submit_nochat_api' # NOTE: like submit_nochat but stable API for string dict passing
|
161 |
+
client = get_client(serialize=True)
|
162 |
+
res = client.predict(
|
163 |
+
str(dict(kwargs)),
|
164 |
+
api_name=api_name,
|
165 |
+
)
|
166 |
+
print("Raw client result: %s" % res, flush=True)
|
167 |
+
res_dict = dict(prompt=kwargs['instruction_nochat'],
|
168 |
+
response=md_to_text(ast.literal_eval(res)['response']),
|
169 |
+
sources=ast.literal_eval(res)['sources'])
|
170 |
+
print(res_dict)
|
171 |
+
return res_dict, client
|
172 |
+
|
173 |
+
|
174 |
+
@pytest.mark.skip(reason="For manual use against some server, no server launched")
|
175 |
+
def test_client_basic_api_lean_morestuff(prompt_type='human_bot'):
|
176 |
+
return run_client_nochat_api_lean_morestuff(prompt='Who are you?', prompt_type=prompt_type, max_new_tokens=50)
|
177 |
+
|
178 |
+
|
179 |
+
def run_client_nochat_api_lean_morestuff(prompt, prompt_type='human_bot', max_new_tokens=512):
|
180 |
+
kwargs = dict(
|
181 |
+
instruction='',
|
182 |
+
iinput='',
|
183 |
+
context='',
|
184 |
+
stream_output=False,
|
185 |
+
prompt_type=prompt_type,
|
186 |
+
temperature=0.1,
|
187 |
+
top_p=0.75,
|
188 |
+
top_k=40,
|
189 |
+
num_beams=1,
|
190 |
+
max_new_tokens=256,
|
191 |
+
min_new_tokens=0,
|
192 |
+
early_stopping=False,
|
193 |
+
max_time=20,
|
194 |
+
repetition_penalty=1.0,
|
195 |
+
num_return_sequences=1,
|
196 |
+
do_sample=True,
|
197 |
+
chat=False,
|
198 |
+
instruction_nochat=prompt,
|
199 |
+
iinput_nochat='',
|
200 |
+
langchain_mode='Disabled',
|
201 |
+
top_k_docs=4,
|
202 |
+
document_choice=['All'],
|
203 |
+
)
|
204 |
+
|
205 |
+
api_name = '/submit_nochat_api' # NOTE: like submit_nochat but stable API for string dict passing
|
206 |
+
client = get_client(serialize=True)
|
207 |
+
res = client.predict(
|
208 |
+
str(dict(kwargs)),
|
209 |
+
api_name=api_name,
|
210 |
+
)
|
211 |
+
print("Raw client result: %s" % res, flush=True)
|
212 |
+
res_dict = dict(prompt=kwargs['instruction_nochat'],
|
213 |
+
response=md_to_text(ast.literal_eval(res)['response']),
|
214 |
+
sources=ast.literal_eval(res)['sources'])
|
215 |
+
print(res_dict)
|
216 |
+
return res_dict, client
|
217 |
+
|
218 |
+
|
219 |
+
@pytest.mark.skip(reason="For manual use against some server, no server launched")
|
220 |
+
def test_client_chat(prompt_type='human_bot'):
|
221 |
+
return run_client_chat(prompt='Who are you?', prompt_type=prompt_type, stream_output=False, max_new_tokens=50,
|
222 |
+
langchain_mode='Disabled')
|
223 |
+
|
224 |
+
|
225 |
+
@pytest.mark.skip(reason="For manual use against some server, no server launched")
|
226 |
+
def test_client_chat_stream(prompt_type='human_bot'):
|
227 |
+
return run_client_chat(prompt="Tell a very long kid's story about birds.", prompt_type=prompt_type,
|
228 |
+
stream_output=True, max_new_tokens=512,
|
229 |
+
langchain_mode='Disabled')
|
230 |
+
|
231 |
+
|
232 |
+
def run_client_chat(prompt, prompt_type, stream_output, max_new_tokens, langchain_mode, prompt_dict=None):
|
233 |
+
client = get_client(serialize=False)
|
234 |
+
|
235 |
+
kwargs, args = get_args(prompt, prompt_type, chat=True, stream_output=stream_output,
|
236 |
+
max_new_tokens=max_new_tokens, langchain_mode=langchain_mode,
|
237 |
+
prompt_dict=prompt_dict)
|
238 |
+
return run_client(client, prompt, args, kwargs)
|
239 |
+
|
240 |
+
|
241 |
+
def run_client(client, prompt, args, kwargs, do_md_to_text=True, verbose=False):
|
242 |
+
assert kwargs['chat'], "Chat mode only"
|
243 |
+
res = client.predict(*tuple(args), api_name='/instruction')
|
244 |
+
args[-1] += [res[-1]]
|
245 |
+
|
246 |
+
res_dict = kwargs
|
247 |
+
res_dict['prompt'] = prompt
|
248 |
+
if not kwargs['stream_output']:
|
249 |
+
res = client.predict(*tuple(args), api_name='/instruction_bot')
|
250 |
+
res_dict['response'] = res[0][-1][1]
|
251 |
+
print(md_to_text(res_dict['response'], do_md_to_text=do_md_to_text))
|
252 |
+
return res_dict, client
|
253 |
+
else:
|
254 |
+
job = client.submit(*tuple(args), api_name='/instruction_bot')
|
255 |
+
res1 = ''
|
256 |
+
while not job.done():
|
257 |
+
outputs_list = job.communicator.job.outputs
|
258 |
+
if outputs_list:
|
259 |
+
res = job.communicator.job.outputs[-1]
|
260 |
+
res1 = res[0][-1][-1]
|
261 |
+
res1 = md_to_text(res1, do_md_to_text=do_md_to_text)
|
262 |
+
print(res1)
|
263 |
+
time.sleep(0.1)
|
264 |
+
full_outputs = job.outputs()
|
265 |
+
if verbose:
|
266 |
+
print('job.outputs: %s' % str(full_outputs))
|
267 |
+
# ensure get ending to avoid race
|
268 |
+
# -1 means last response if streaming
|
269 |
+
# 0 means get text_output, ignore exception_text
|
270 |
+
# 0 means get list within text_output that looks like [[prompt], [answer]]
|
271 |
+
# 1 means get bot answer, so will have last bot answer
|
272 |
+
res_dict['response'] = md_to_text(full_outputs[-1][0][0][1], do_md_to_text=do_md_to_text)
|
273 |
+
return res_dict, client
|
274 |
+
|
275 |
+
|
276 |
+
@pytest.mark.skip(reason="For manual use against some server, no server launched")
|
277 |
+
def test_client_nochat_stream(prompt_type='human_bot'):
|
278 |
+
return run_client_nochat_gen(prompt="Tell a very long kid's story about birds.", prompt_type=prompt_type,
|
279 |
+
stream_output=True, max_new_tokens=512,
|
280 |
+
langchain_mode='Disabled')
|
281 |
+
|
282 |
+
|
283 |
+
def run_client_nochat_gen(prompt, prompt_type, stream_output, max_new_tokens, langchain_mode):
|
284 |
+
client = get_client(serialize=False)
|
285 |
+
|
286 |
+
kwargs, args = get_args(prompt, prompt_type, chat=False, stream_output=stream_output,
|
287 |
+
max_new_tokens=max_new_tokens, langchain_mode=langchain_mode)
|
288 |
+
return run_client_gen(client, prompt, args, kwargs)
|
289 |
+
|
290 |
+
|
291 |
+
def run_client_gen(client, prompt, args, kwargs, do_md_to_text=True, verbose=False):
|
292 |
+
res_dict = kwargs
|
293 |
+
res_dict['prompt'] = prompt
|
294 |
+
if not kwargs['stream_output']:
|
295 |
+
res = client.predict(str(dict(kwargs)), api_name='/submit_nochat_api')
|
296 |
+
res_dict['response'] = res[0]
|
297 |
+
print(md_to_text(res_dict['response'], do_md_to_text=do_md_to_text))
|
298 |
+
return res_dict, client
|
299 |
+
else:
|
300 |
+
job = client.submit(str(dict(kwargs)), api_name='/submit_nochat_api')
|
301 |
+
while not job.done():
|
302 |
+
outputs_list = job.communicator.job.outputs
|
303 |
+
if outputs_list:
|
304 |
+
res = job.communicator.job.outputs[-1]
|
305 |
+
res_dict = ast.literal_eval(res)
|
306 |
+
print('Stream: %s' % res_dict['response'])
|
307 |
+
time.sleep(0.1)
|
308 |
+
res_list = job.outputs()
|
309 |
+
assert len(res_list) > 0, "No response, check server"
|
310 |
+
res = res_list[-1]
|
311 |
+
res_dict = ast.literal_eval(res)
|
312 |
+
print('Final: %s' % res_dict['response'])
|
313 |
+
return res_dict, client
|
314 |
+
|
315 |
+
|
316 |
+
def md_to_text(md, do_md_to_text=True):
|
317 |
+
if not do_md_to_text:
|
318 |
+
return md
|
319 |
+
assert md is not None, "Markdown is None"
|
320 |
+
html = markdown.markdown(md)
|
321 |
+
soup = BeautifulSoup(html, features='html.parser')
|
322 |
+
return soup.get_text()
|
323 |
+
|
324 |
+
|
325 |
+
def run_client_many(prompt_type='human_bot'):
|
326 |
+
ret1, _ = test_client_chat(prompt_type=prompt_type)
|
327 |
+
ret2, _ = test_client_chat_stream(prompt_type=prompt_type)
|
328 |
+
ret3, _ = test_client_nochat_stream(prompt_type=prompt_type)
|
329 |
+
ret4, _ = test_client_basic(prompt_type=prompt_type)
|
330 |
+
ret5, _ = test_client_basic_api(prompt_type=prompt_type)
|
331 |
+
ret6, _ = test_client_basic_api_lean(prompt_type=prompt_type)
|
332 |
+
ret7, _ = test_client_basic_api_lean_morestuff(prompt_type=prompt_type)
|
333 |
+
return ret1, ret2, ret3, ret4, ret5, ret6, ret7
|
334 |
+
|
335 |
+
|
336 |
+
if __name__ == '__main__':
|
337 |
+
run_client_many()
|
create_data.py
ADDED
@@ -0,0 +1,1809 @@
|
|
|
|
|
|
|
|
|
|
|
|
|
|
|
|
|
|
|
|
|
|
|
|
|
|
|
|
|
|
|
|
|
|
|
|
|
|
|
|
|
|
|
|
|
|
|
|
|
|
|
|
|
|
|
|
|
|
|
|
|
|
|
|
|
|
|
|
|
|
|
|
|
|
|
|
|
|
|
|
|
|
|
|
|
|
|
|
|
|
|
|
|
|
|
|
|
|
|
|
|
|
|
|
|
|
|
|
|
|
|
|
|
|
|
|
|
|
|
|
|
|
|
|
|
|
|
|
|
|
|
|
|
|
|
|
|
|
|
|
|
|
|
|
|
|
|
|
|
|
|
|
|
|
|
|
|
|
|
|
|
|
|
|
|
|
|
|
|
|
|
|
|
|
|
|
|
|
|
|
|
|
|
|
|
|
|
|
|
|
|
|
|
|
|
|
|
|
|
|
|
|
|
|
|
|
|
|
|
|
|
|
|
|
|
|
|
|
|
|
|
|
|
|
|
|
|
|
|
|
|
|
|
|
|
|
|
|
|
|
|
|
|
|
|
|
|
|
|
|
|
|
|
|
|
|
|
|
|
|
|
|
|
|
|
|
|
|
|
|
|
|
|
|
|
|
|
|
|
|
|
|
|
|
|
|
|
|
|
|
|
|
|
|
|
|
|
|
|
|
|
|
|
|
|
|
|
|
|
|
|
|
|
|
|
|
|
|
|
|
|
|
|
|
|
|
|
|
|
|
|
|
|
|
|
|
|
|
|
|
|
|
|
|
|
|
|
|
|
|
|
|
|
|
|
|
|
|
|
|
|
|
|
|
|
|
|
|
|
|
|
|
|
|
|
|
|
|
|
|
|
|
|
|
|
|
|
|
|
|
|
|
|
|
|
|
|
|
|
|
|
|
|
|
|
|
|
|
|
|
|
|
|
|
|
|
|
|
|
|
|
|
|
|
|
|
|
|
|
|
|
|
|
|
|
|
|
|
|
|
|
|
|
|
|
|
|
|
|
|
|
|
|
|
|
|
|
|
|
|
|
|
|
|
|
|
|
|
|
|
|
|
|
|
|
|
|
|
|
|
|
|
|
|
|
|
|
|
|
|
|
|
|
|
|
|
|
|
|
|
|
|
|
|
|
|
|
|
|
|
|
|
|
|
|
|
|
|
|
|
|
|
|
|
|
|
|
|
|
|
|
|
|
|
|
|
|
|
|
|
|
|
|
|
|
|
|
|
|
|
|
|
|
|
|
|
|
|
|
|
|
|
|
|
|
|
|
|
|
|
|
|
|
|
|
|
|
|
|
|
|
|
|
|
|
|
|
|
|
|
|
|
|
|
|
|
|
|
|
|
|
|
|
|
|
|
|
|
|
|
|
|
|
|
|
|
|
|
|
|
|
|
|
|
|
|
|
|
|
|
|
|
|
|
|
|
|
|
|
|
|
|
|
|
|
|
|
|
|
|
|
|
|
|
|
|
|
|
|
|
|
|
|
|
|
|
|
|
|
|
|
|
|
|
|
|
|
|
|
|
|
|
|
|
|
|
|
|
|
|
|
|
|
|
|
|
|
|
|
|
|
|
|
|
|
|
|
|
|
|
|
|
|
|
|
|
|
|
|
|
|
|
|
|
|
|
|
|
|
|
|
|
|
|
|
|
|
|
|
|
|
|
|
|
|
|
|
|
|
|
|
|
|
|
|
|
|
|
|
|
|
|
|
|
|
|
|
|
|
|
|
|
|
|
|
|
|
|
|
|
|
|
|
|
|
|
|
|
|
|
|
|
|
|
|
|
|
|
|
|
|
|
|
|
|
|
|
|
|
|
|
|
|
|
|
|
|
|
|
|
|
|
|
|
|
|
|
|
|
|
|
|
|
|
|
|
|
|
|
|
|
|
|
|
|
|
|
|
|
|
|
|
|
|
|
|
|
|
|
|
|
|
|
|
|
|
|
|
|
|
|
|
|
|
|
|
|
|
|
|
|
|
|
|
|
|
|
|
|
|
|
|
|
|
|
|
|
|
|
|
|
|
|
|
|
|
|
|
|
|
|
|
|
|
|
|
|
|
|
|
|
|
|
|
|
|
|
|
|
|
|
|
|
|
|
|
|
|
|
|
|
|
|
|
|
|
|
|
|
|
|
|
|
|
|
|
|
|
|
|
|
|
|
|
|
|
|
|
|
|
|
|
|
|
|
|
|
|
|
|
|
|
|
|
|
|
|
|
|
|
|
|
|
|
|
|
|
|
|
|
|
|
|
|
|
|
|
|
|
|
|
|
|
|
|
|
|
|
|
|
|
|
|
|
|
|
|
|
|
|
|
|
|
|
|
|
|
|
|
|
|
|
|
|
|
|
|
|
|
|
|
|
|
|
|
|
|
|
|
|
|
|
|
|
|
|
|
|
|
|
|
|
|
|
|
|
|
|
|
|
|
|
|
|
|
|
|
|
|
|
|
|
|
|
|
|
|
|
|
|
|
|
|
|
|
|
|
|
|
|
|
|
|
|
|
|
|
|
|
|
|
|
|
|
|
|
|
|
|
|
|
|
|
|
|
|
|
|
|
|
|
|
|
|
|
|
|
|
|
|
|
|
|
|
|
|
|
|
|
|
|
|
|
|
|
|
|
|
|
|
|
|
|
|
|
|
|
|
|
|
|
|
|
|
|
|
|
|
|
|
|
|
|
|
|
|
|
|
|
|
|
|
|
|
|
|
|
|
|
|
|
|
|
|
|
|
|
|
|
|
|
|
|
|
|
|
|
|
|
|
|
|
|
|
|
|
|
|
|
|
|
|
|
|
|
|
|
|
|
|
|
|
|
|
|
|
|
|
|
|
|
|
|
|
|
|
|
|
|
|
|
|
|
|
|
|
|
|
|
|
|
|
|
|
|
|
|
|
|
|
|
|
|
|
|
|
|
|
|
|
|
|
|
|
|
|
|
|
|
|
|
|
|
|
|
|
|
|
|
|
|
|
|
|
|
|
|
|
|
|
|
|
|
|
|
|
|
|
|
|
|
|
|
|
|
|
|
|
|
|
|
|
|
|
|
|
|
|
|
|
|
|
|
|
|
|
|
|
|
|
|
|
|
|
|
|
|
|
|
|
|
|
|
|
|
|
|
|
|
|
|
|
|
|
|
|
|
|
|
|
|
|
|
|
|
|
|
|
|
|
|
|
|
|
|
|
|
|
|
|
|
|
|
|
|
|
|
|
|
|
|
|
|
|
|
|
|
|
|
|
|
|
|
|
|
|
|
|
|
|
|
|
|
|
|
|
|
|
|
|
|
|
|
|
|
|
|
|
|
|
|
|
|
|
|
|
|
|
|
|
|
|
|
|
|
|
|
|
|
|
|
|
|
|
|
|
|
|
|
|
|
|
|
|
|
|
|
|
|
|
|
|
|
|
|
|
|
|
|
|
|
|
|
|
|
|
|
|
|
|
|
|
|
|
|
|
|
|
|
|
|
|
|
|
|
|
|
|
|
|
|
|
|
|
|
|
|
|
|
|
|
|
|
|
|
|
|
|
|
|
|
|
|
|
|
|
|
|
|
|
|
|
|
|
|
|
|
|
|
|
|
|
|
|
|
|
|
|
|
|
|
|
|
|
|
|
|
|
|
|
|
|
|
|
|
|
|
|
|
|
|
|
|
|
|
|
|
|
|
|
|
|
|
|
|
|
|
|
|
|
|
|
|
|
|
|
|
|
|
|
|
|
|
|
|
|
|
|
|
|
|
|
|
|
|
|
|
|
|
|
|
|
|
|
|
|
|
|
|
|
|
|
|
|
|
|
|
|
|
|
|
|
|
|
|
|
|
|
|
|
|
|
|
|
|
|
|
|
|
|
|
|
|
|
|
|
|
|
|
|
|
|
|
|
|
|
|
|
|
|
|
|
|
|
|
|
|
|
|
|
|
|
|
|
|
|
|
|
|
|
|
|
|
|
|
|
|
|
|
|
|
|
|
|
|
|
|
|
|
|
|
|
|
|
|
|
|
|
|
|
|
|
|
|
|
|
|
|
|
|
|
|
|
|
|
|
|
|
|
|
|
|
|
|
|
|
|
|
|
|
|
|
|
|
|
|
|
|
|
|
|
|
|
|
|
|
|
|
|
|
|
|
|
|
|
|
|
|
|
|
|
|
|
|
|
|
|
|
|
|
|
|
|
|
|
|
|
|
|
|
|
|
|
|
|
|
|
|
|
|
|
|
|
|
|
|
|
|
|
|
|
|
|
|
|
|
|
|
|
|
|
|
|
|
|
|
|
|
|
|
|
|
|
|
|
|
|
|
|
|
|
|
|
|
|
|
|
|
|
|
|
|
|
|
|
|
|
|
|
|
|
|
|
|
|
|
|
|
|
|
|
|
|
|
|
|
|
|
|
|
|
|
|
|
|
|
|
|
|
|
|
|
|
|
|
|
|
|
|
|
|
|
|
|
|
|
|
|
|
|
|
|
|
|
|
|
|
|
|
|
|
|
|
|
|
|
|
|
|
|
|
|
|
|
|
|
|
|
|
|
|
|
|
|
|
|
|
|
|
|
|
|
|
|
|
|
|
|
|
|
|
|
|
|
|
|
|
|
|
|
|
|
|
|
|
|
|
|
|
|
|
|
|
|
|
|
|
|
|
|
|
|
|
|
|
|
|
|
|
|
|
|
|
|
|
|
|
|
|
|
|
|
|
|
|
|
|
|
|
|
|
|
|
|
|
|
|
|
|
|
|
|
|
|
|
|
|
|
|
|
|
|
|
|
|
|
|
|
|
|
|
|
|
|
|
|
|
|
|
|
|
|
|
|
|
|
|
|
|
|
|
|
|
|
|
|
|
|
|
|
|
|
|
|
|
|
|
|
|
|
|
|
|
|
|
|
|
|
|
|
|
|
|
|
|
|
|
|
|
|
|
|
|
|
|
|
|
|
|
|
|
|
|
|
|
|
|
|
|
|
|
|
|
|
|
|
|
|
|
|
|
|
|
|
|
|
|
|
|
|
|
|
|
|
|
|
|
|
|
|
|
|
|
|
|
|
|
|
|
|
|
|
|
|
|
|
|
|
|
|
|
|
|
|
|
|
|
|
|
|
|
|
|
|
|
|
|
|
|
|
|
|
|
|
|
|
|
|
|
|
|
|
|
|
|
|
|
|
|
|
|
|
|
|
|
|
|
|
|
|
|
|
|
|
|
|
|
|
|
|
|
|
|
|
|
|
|
|
|
|
|
|
|
|
|
|
|
|
|
|
|
|
|
|
|
|
|
|
|
|
|
|
|
|
|
|
|
|
|
|
|
|
|
|
|
|
|
|
|
|
|
|
|
|
|
|
|
|
|
|
|
|
|
|
|
|
|
|
|
|
|
|
|
|
|
|
|
|
|
|
|
|
|
|
|
|
|
|
|
|
|
|
|
|
|
|
|
|
|
|
|
|
|
|
|
|
|
|
|
|
|
|
|
|
|
|
|
|
|
|
|
|
|
|
|
|
|
|
|
|
|
|
|
|
|
|
|
|
|
|
|
|
|
|
|
|
|
|
|
|
|
|
|
|
|
|
|
|
|
|
|
|
|
|
|
|
|
|
|
|
|
|
|
|
|
|
|
|
|
|
|
|
|
|
|
|
|
|
|
|
|
|
|
|
|
|
|
|
|
|
|
|
|
|
|
|
|
|
|
|
|
|
|
|
|
|
|
|
|
|
|
|
|
|
|
|
|
|
|
|
|
|
|
|
|
|
|
|
|
|
|
|
|
|
|
|
|
|
|
|
|
|
|
|
|
|
|
|
|
|
|
|
|
|
|
|
|
|
|
|
|
|
|
|
|
|
|
|
|
|
|
|
|
|
|
|
|
|
|
|
|
|
|
|
|
|
|
|
|
|
|
|
|
|
|
|
|
|
|
|
|
|
|
|
|
|
|
|
|
|
|
|
|
|
|
|
|
|
|
|
|
|
|
|
|
|
|
|
|
|
|
|
|
|
|
|
|
|
|
|
|
|
|
|
|
|
|
|
|
|
|
|
|
|
|
|
|
|
|
|
|
|
|
|
|
|
|
|
|
|
|
|
|
|
|
|
|
|
|
|
|
|
|
|
|
|
|
|
|
|
|
|
|
|
|
|
|
|
|
|
|
|
|
|
|
|
|
|
|
|
|
|
|
|
|
|
|
|
|
|
|
|
|
|
|
|
|
|
|
|
|
|
|
|
|
|
|
|
|
|
|
|
|
|
|
|
|
|
|
|
|
|
|
|
|
|
|
|
|
|
|
|
|
|
|
|
|
|
|
|
|
|
|
|
|
|
|
|
|
|
|
|
|
|
|
|
|
|
|
|
|
|
|
|
|
|
|
|
|
|
|
|
|
|
|
|
|
|
|
|
|
|
|
|
|
|
|
|
|
|
|
|
|
|
|
|
|
|
|
|
|
|
|
|
|
|
|
|
|
|
|
|
|
|
|
|
|
|
|
|
|
|
|
|
|
|
|
|
|
|
|
|
|
|
|
|
|
|
|
|
|
|
|
|
|
|
|
|
|
|
|
|
|
|
|
|
|
|
|
|
|
|
|
|
|
|
|
|
|
|
|
|
|
|
|
|
|
|
|
|
|
|
|
|
|
|
|
|
|
|
|
|
|
|
|
|
|
|
|
|
|
|
|
|
|
|
|
|
|
|
|
|
|
|
|
|
|
|
|
|
|
|
|
|
|
|
|
|
|
|
|
|
|
|
|
|
|
|
|
|
|
|
|
|
|
|
|
|
|
|
|
|
|
|
|
|
|
|
|
|
|
|
|
|
|
|
|
|
|
|
|
|
|
|
|
|
|
|
|
|
|
|
|
|
|
|
|
|
|
|
|
|
|
|
|
|
|
|
|
|
|
|
|
|
|
|
|
|
|
|
|
|
|
|
|
|
|
|
|
|
|
|
|
|
|
|
|
|
|
|
|
|
|
|
|
|
|
|
|
|
|
|
|
|
|
|
|
|
|
|
|
|
|
|
|
|
|
|
|
|
|
|
|
|
|
|
|
|
|
|
|
|
|
|
|
|
|
|
|
|
|
|
|
|
|
|
|
|
|
|
|
|
|
|
|
|
|
|
|
|
|
|
|
|
|
|
|
|
|
|
|
|
|
|
|
|
|
|
|
|
|
|
|
|
|
|
|
|
|
|
|
|
|
|
|
|
|
|
|
|
|
|
|
|
|
|
|
|
|
|
|
|
|
|
|
|
|
|
|
|
|
|
|
|
|
|
|
|
|
|
|
|
|
|
|
|
|
|
|
|
|
|
|
|
|
|
|
|
|
|
|
|
|
|
|
|
|
|
|
|
|
|
|
|
|
|
|
|
|
|
|
|
|
|
|
|
|
|
|
|
|
|
|
|
|
|
|
|
|
|
|
|
|
|
|
|
|
|
|
|
|
|
|
|
|
|
|
|
|
|
|
|
|
|
|
|
|
|
|
|
|
|
|
|
|
|
|
|
|
|
|
|
|
|
|
|
|
|
|
|
|
|
|
|
|
|
|
|
|
|
|
|
|
|
|
|
|
|
|
|
|
|
|
|
|
|
|
|
|
|
|
|
|
|
|
|
|
|
|
|
|
|
|
|
|
|
|
|
|
|
|
|
|
|
|
|
|
|
|
|
|
|
|
|
|
|
|
|
|
|
|
|
|
|
|
|
|
|
|
|
|
|
|
|
|
|
|
|
|
|
|
|
|
|
|
|
|
|
|
|
|
|
|
|
|
|
|
|
|
|
|
|
|
|
|
|
|
|
|
|
|
|
|
|
|
|
|
|
|
|
|
|
|
|
|
|
|
|
|
|
|
|
|
|
|
|
|
|
|
|
|
|
|
|
|
|
|
|
|
|
|
|
|
|
|
|
|
|
|
|
|
|
|
|
|
|
|
|
|
|
|
|
|
|
|
|
|
|
|
|
|
|
|
|
|
|
|
|
|
|
|
|
|
|
|
|
|
|
|
|
|
|
|
|
|
|
|
|
|
|
|
|
|
|
|
|
|
|
|
|
|
|
|
|
|
|
|
|
|
|
|
|
|
|
|
|
|
|
|
|
|
|
|
|
|
|
|
|
|
|
|
|
|
|
|
|
|
|
|
|
|
|
|
|
|
|
|
|
|
|
|
|
|
|
|
|
|
|
|
|
|
1 |
+
"""
|
2 |
+
Dataset creation tools.
|
3 |
+
|
4 |
+
Keep to-level imports clean of non-trivial imports for specific tools,
|
5 |
+
because this file is imported for various purposes
|
6 |
+
"""
|
7 |
+
|
8 |
+
import ast
|
9 |
+
import concurrent.futures
|
10 |
+
import contextlib
|
11 |
+
import hashlib
|
12 |
+
import json
|
13 |
+
import os
|
14 |
+
import shutil
|
15 |
+
import signal
|
16 |
+
import sys
|
17 |
+
import traceback
|
18 |
+
from concurrent.futures import ProcessPoolExecutor
|
19 |
+
|
20 |
+
import psutil
|
21 |
+
import pytest
|
22 |
+
import pandas as pd
|
23 |
+
import numpy as np
|
24 |
+
from tqdm import tqdm
|
25 |
+
|
26 |
+
from utils import flatten_list, remove
|
27 |
+
|
28 |
+
|
29 |
+
def parse_rst_file(filepath):
|
30 |
+
with open(filepath, 'r') as f:
|
31 |
+
input_data = f.read()
|
32 |
+
settings_overrides = {'initial_header_level': 2}
|
33 |
+
from docutils import core
|
34 |
+
document = core.publish_doctree(
|
35 |
+
source=input_data,
|
36 |
+
source_path=filepath,
|
37 |
+
settings_overrides=settings_overrides,
|
38 |
+
)
|
39 |
+
qa_pairs = []
|
40 |
+
current_section = None
|
41 |
+
current_question = ""
|
42 |
+
current_answer = ""
|
43 |
+
for node in document.traverse():
|
44 |
+
if node.__class__.__name__ == 'section':
|
45 |
+
current_section = ""
|
46 |
+
elif current_section is not None:
|
47 |
+
if node.__class__.__name__ == 'Text':
|
48 |
+
if node.astext()[-1] == "?":
|
49 |
+
if current_question:
|
50 |
+
qa_pairs.append((current_question, current_answer))
|
51 |
+
current_question = node.astext()
|
52 |
+
current_answer = ""
|
53 |
+
else:
|
54 |
+
current_answer += node.astext()
|
55 |
+
if current_answer:
|
56 |
+
qa_pairs.append((current_question, current_answer))
|
57 |
+
return {k: v for k, v in qa_pairs}
|
58 |
+
|
59 |
+
|
60 |
+
def test_scrape_dai_docs():
|
61 |
+
home = os.path.expanduser('~')
|
62 |
+
file = os.path.join(home, 'h2oai/docs/faq.rst')
|
63 |
+
qa_pairs = parse_rst_file(file)
|
64 |
+
prompt_type = 'human_bot'
|
65 |
+
from prompter import prompt_types
|
66 |
+
assert prompt_type in prompt_types
|
67 |
+
save_thing = [{"instruction": k, "output": v, 'prompt_type': prompt_type} for k, v in qa_pairs.items()]
|
68 |
+
output_file = "dai_faq.json"
|
69 |
+
with open(output_file, "wt") as f:
|
70 |
+
f.write(json.dumps(save_thing, indent=2))
|
71 |
+
|
72 |
+
|
73 |
+
def test_scrape_dai_docs_all():
|
74 |
+
"""
|
75 |
+
pytest create_data.py::test_scrape_dai_docs_all
|
76 |
+
"""
|
77 |
+
import glob
|
78 |
+
import nltk
|
79 |
+
nltk.download('punkt')
|
80 |
+
dd = {}
|
81 |
+
np.random.seed(1234)
|
82 |
+
home = os.path.expanduser('~')
|
83 |
+
files = list(glob.glob(os.path.join(home, "h2oai/docs/**/*rst")))
|
84 |
+
np.random.shuffle(files)
|
85 |
+
val_count = int(0.05 * len(files))
|
86 |
+
train_files = files[val_count:]
|
87 |
+
valid_files = files[:val_count]
|
88 |
+
things = [
|
89 |
+
("dai_docs.train.json", train_files),
|
90 |
+
("dai_docs.valid.json", valid_files)
|
91 |
+
]
|
92 |
+
for LEN in [100, 200, 500]:
|
93 |
+
for output_file, ff in things:
|
94 |
+
if output_file not in dd:
|
95 |
+
dd[output_file] = []
|
96 |
+
for f in ff:
|
97 |
+
with open(f) as input:
|
98 |
+
blob = input.read()
|
99 |
+
blob = blob.replace("~~", "")
|
100 |
+
blob = blob.replace("==", "")
|
101 |
+
blob = blob.replace("''", "")
|
102 |
+
blob = blob.replace("--", "")
|
103 |
+
blob = blob.replace("**", "")
|
104 |
+
dd[output_file].extend(get_sentences(blob, length=LEN))
|
105 |
+
for output_file, _ in things:
|
106 |
+
save_thing = [{"output": k.strip(), 'prompt_type': 'plain'} for k in dd[output_file]]
|
107 |
+
with open(output_file, "wt") as f:
|
108 |
+
f.write(json.dumps(save_thing, indent=2))
|
109 |
+
|
110 |
+
|
111 |
+
def get_sentences(blob, length):
|
112 |
+
"""
|
113 |
+
break-up input text into sentences and then output list of sentences of about length in size
|
114 |
+
:param blob:
|
115 |
+
:param length:
|
116 |
+
:return:
|
117 |
+
"""
|
118 |
+
import nltk
|
119 |
+
nltk.download('punkt')
|
120 |
+
from nltk.tokenize import sent_tokenize
|
121 |
+
sentences = sent_tokenize(blob)
|
122 |
+
my_sentences = []
|
123 |
+
my_string = ""
|
124 |
+
for sentence in sentences:
|
125 |
+
if len(my_string) + len(sentence) <= length:
|
126 |
+
if my_string:
|
127 |
+
my_string += " " + sentence
|
128 |
+
else:
|
129 |
+
my_string = sentence
|
130 |
+
else:
|
131 |
+
my_sentences.append(my_string)
|
132 |
+
my_string = ""
|
133 |
+
return my_sentences or [my_string]
|
134 |
+
|
135 |
+
|
136 |
+
def setup_dai_docs(path=None, dst="working_dir_docs", from_hf=False):
|
137 |
+
"""
|
138 |
+
Only supported if have access to source code or HF token for HF spaces and from_hf=True
|
139 |
+
:param path:
|
140 |
+
:param dst:
|
141 |
+
:param from_hf:
|
142 |
+
:return:
|
143 |
+
"""
|
144 |
+
|
145 |
+
home = os.path.expanduser('~')
|
146 |
+
|
147 |
+
if from_hf:
|
148 |
+
# assumes
|
149 |
+
from huggingface_hub import hf_hub_download
|
150 |
+
# True for case when locally already logged in with correct token, so don't have to set key
|
151 |
+
token = os.getenv('HUGGINGFACE_API_TOKEN', True)
|
152 |
+
path_to_zip_file = hf_hub_download('h2oai/dai_docs', 'dai_docs.zip', token=token, repo_type='dataset')
|
153 |
+
path = 'h2oai'
|
154 |
+
import zipfile
|
155 |
+
with zipfile.ZipFile(path_to_zip_file, 'r') as zip_ref:
|
156 |
+
zip_ref.extractall(path)
|
157 |
+
path = os.path.join(path, 'docs/**/*')
|
158 |
+
|
159 |
+
if path is None:
|
160 |
+
if os.path.isdir(os.path.join(home, 'h2oai')):
|
161 |
+
path = os.path.join(home, "h2oai/docs/**/*")
|
162 |
+
else:
|
163 |
+
assert os.path.isdir(os.path.join(home, 'h2oai.superclean')), '%s does not exist' % path
|
164 |
+
path = os.path.join(home, "h2oai.superclean/docs/**/*")
|
165 |
+
import glob
|
166 |
+
files = list(glob.glob(path, recursive=True))
|
167 |
+
|
168 |
+
# pandoc can't find include files
|
169 |
+
|
170 |
+
remove(dst)
|
171 |
+
os.makedirs(dst)
|
172 |
+
|
173 |
+
# copy full tree, for absolute paths in rst
|
174 |
+
for fil in files:
|
175 |
+
if os.path.isfile(fil):
|
176 |
+
shutil.copy(fil, dst)
|
177 |
+
|
178 |
+
# hack for relative path
|
179 |
+
scorers_dir = os.path.join(dst, 'scorers')
|
180 |
+
makedirs(scorers_dir)
|
181 |
+
for fil in glob.glob(os.path.join(dst, '*.frag')):
|
182 |
+
shutil.copy(fil, scorers_dir)
|
183 |
+
|
184 |
+
return dst
|
185 |
+
|
186 |
+
|
187 |
+
def rst_to_outputs(files, min_len=30, max_len=2048 // 2 - 30):
|
188 |
+
# account for sequence length (context window) including prompt and input and output
|
189 |
+
|
190 |
+
# os.system('pandoc -f rst -t plain ./expert_settings/nlp_settings.rst')
|
191 |
+
import pypandoc
|
192 |
+
basedir = os.path.abspath(os.getcwd())
|
193 |
+
|
194 |
+
outputs = []
|
195 |
+
for fil in files:
|
196 |
+
os.chdir(basedir)
|
197 |
+
os.chdir(os.path.dirname(fil))
|
198 |
+
fil = os.path.basename(fil)
|
199 |
+
print("Processing %s" % fil, flush=True)
|
200 |
+
# out_format can be one of: asciidoc, asciidoctor, beamer, biblatex, bibtex, commonmark, commonmark_x,
|
201 |
+
# context, csljson, docbook, docbook4, docbook5, docx, dokuwiki,
|
202 |
+
# dzslides, epub, epub2, epub3, fb2, gfm, haddock, html, html4, html5, icml,
|
203 |
+
# ipynb, jats, jats_archiving, jats_articleauthoring, jats_publishing, jira,
|
204 |
+
# json, latex, man,
|
205 |
+
# markdown, markdown_github, markdown_mmd, markdown_phpextra, markdown_strict,
|
206 |
+
# mediawiki, ms, muse, native, odt, opendocument, opml, org, pdf, plain, pptx,
|
207 |
+
# revealjs, rst, rtf, s5, slideous, slidy, tei, texinfo, textile, xwiki, zimwiki
|
208 |
+
out_format = 'plain'
|
209 |
+
# avoid extra new lines injected into text
|
210 |
+
extra_args = ['--wrap=preserve', '--resource path="%s" % dst']
|
211 |
+
|
212 |
+
plain_list = []
|
213 |
+
try:
|
214 |
+
# valid for expert settings
|
215 |
+
input_rst = pypandoc.convert_file(fil, 'rst')
|
216 |
+
input_list = input_rst.split('\n``')
|
217 |
+
for input_subrst in input_list:
|
218 |
+
input_plain = pypandoc.convert_text(input_subrst, format='rst', to='plain')
|
219 |
+
plain_list.append([input_plain, fil])
|
220 |
+
except Exception as e:
|
221 |
+
print("file exception: %s %s" % (fil, str(e)), flush=True)
|
222 |
+
|
223 |
+
if not plain_list:
|
224 |
+
# if failed to process as pieces of rst, then
|
225 |
+
output = pypandoc.convert_file(fil, out_format, extra_args=extra_args, format='rst')
|
226 |
+
outputs1 = get_sentences(output, length=max_len)
|
227 |
+
for oi, output in enumerate(outputs1):
|
228 |
+
output = output.replace('\n\n', '\n')
|
229 |
+
plain_list.append([output, fil])
|
230 |
+
outputs.extend(plain_list)
|
231 |
+
|
232 |
+
# report:
|
233 |
+
# [print(len(x)) for x in outputs]
|
234 |
+
|
235 |
+
# deal with blocks longer than context size (sequence length) of 2048
|
236 |
+
new_outputs = []
|
237 |
+
num_truncated = 0
|
238 |
+
num_orig = len(outputs)
|
239 |
+
for output, fil in outputs:
|
240 |
+
if len(output) < max_len:
|
241 |
+
new_outputs.append([output, fil])
|
242 |
+
continue
|
243 |
+
outputs1 = get_sentences(output, length=max_len)
|
244 |
+
for oi, output1 in enumerate(outputs1):
|
245 |
+
output1 = output1.replace('\n\n', '\n')
|
246 |
+
new_outputs.append([output1, fil])
|
247 |
+
num_truncated += 1
|
248 |
+
print('num_orig: %s num_truncated: %s' % (num_orig, num_truncated), flush=True)
|
249 |
+
|
250 |
+
new_outputs = [[k.strip(), fil] for k, fil in new_outputs if len(k.strip()) > min_len]
|
251 |
+
|
252 |
+
return new_outputs
|
253 |
+
|
254 |
+
|
255 |
+
def test_scrape_dai_docs_all_pandoc():
|
256 |
+
"""
|
257 |
+
pytest -s -v create_data.py::test_scrape_dai_docs_all_pandoc
|
258 |
+
:return:
|
259 |
+
"""
|
260 |
+
|
261 |
+
dst = setup_dai_docs()
|
262 |
+
|
263 |
+
import glob
|
264 |
+
files = list(glob.glob(os.path.join(dst, '*rst'), recursive=True))
|
265 |
+
|
266 |
+
basedir = os.path.abspath(os.getcwd())
|
267 |
+
new_outputs = rst_to_outputs(files)
|
268 |
+
os.chdir(basedir)
|
269 |
+
|
270 |
+
remove(dst)
|
271 |
+
save_thing = [{"output": k.strip(), 'prompt_type': 'plain'} for k in new_outputs]
|
272 |
+
output_file = "dai_docs.train_cleaned.json"
|
273 |
+
with open(output_file, "wt") as f:
|
274 |
+
f.write(json.dumps(save_thing, indent=2))
|
275 |
+
|
276 |
+
|
277 |
+
def test_config_to_json():
|
278 |
+
"""
|
279 |
+
Needs to run from Driverless AI source directory.
|
280 |
+
E.g. (base) jon@gpu:~/h2oai$ pytest -s -v /data/jon/h2ogpt/create_data.py::test_config_to_json ; cp config.json /data/jon/h2ogpt/
|
281 |
+
:return:
|
282 |
+
"""
|
283 |
+
try:
|
284 |
+
# Arrange
|
285 |
+
import json
|
286 |
+
from h2oaicore.systemutils import config
|
287 |
+
toml_list = []
|
288 |
+
for k, v in config.get_meta_dict().items():
|
289 |
+
title = (v.title + ": ") if v.title else ''
|
290 |
+
comment = v.comment or ''
|
291 |
+
if not (title or comment):
|
292 |
+
continue
|
293 |
+
toml_list.extend(
|
294 |
+
[
|
295 |
+
{
|
296 |
+
'prompt_type': 'plain',
|
297 |
+
'instruction': f"<human>: What does {k} do?\n<bot>: {k.replace('_', ' ')} config.toml: {comment or title}\n<human>:".replace(
|
298 |
+
"\n", ""),
|
299 |
+
},
|
300 |
+
{
|
301 |
+
'prompt_type': 'plain',
|
302 |
+
'instruction': f"<human>: Explain {k}.\n<bot>: {k.replace('_', ' ')} config.toml: {comment or title}\n<human>:".replace(
|
303 |
+
"\n", ""),
|
304 |
+
},
|
305 |
+
{
|
306 |
+
'prompt_type': 'plain',
|
307 |
+
'instruction': f"<human>: How can I do this: {title}.\n<bot>: Set the {k.replace('_', ' ')} config.toml\n<human>:".replace(
|
308 |
+
"\n", ""),
|
309 |
+
} if title and comment else None,
|
310 |
+
{
|
311 |
+
'prompt_type': 'human_bot',
|
312 |
+
'instruction': f'Explain the following expert setting for Driverless AI',
|
313 |
+
'input': f"{k}",
|
314 |
+
'output': f"{k.replace('_', ' ')} config.toml: {comment or title}".replace("\n", ""),
|
315 |
+
},
|
316 |
+
{
|
317 |
+
'prompt_type': 'human_bot',
|
318 |
+
'instruction': f'Explain the following expert setting for Driverless AI',
|
319 |
+
'input': f"{k}",
|
320 |
+
'output': f"{k.replace('_', ' ')} config.toml: {title}{comment}".replace("\n", ""),
|
321 |
+
},
|
322 |
+
{
|
323 |
+
'prompt_type': 'human_bot',
|
324 |
+
'instruction': f'Explain the following expert setting for Driverless AI',
|
325 |
+
'input': f"{k.replace('_', ' ')}",
|
326 |
+
'output': f"{k.replace('_', ' ')} config.toml: {title}{comment}".replace("\n", ""),
|
327 |
+
},
|
328 |
+
{
|
329 |
+
'prompt_type': 'human_bot',
|
330 |
+
'instruction': f'Explain the following expert setting for Driverless AI',
|
331 |
+
'input': f"{title}",
|
332 |
+
'output': f"{k.replace('_', ' ')} config.toml: {title}{comment}".replace("\n", ""),
|
333 |
+
},
|
334 |
+
{
|
335 |
+
'prompt_type': 'human_bot',
|
336 |
+
'instruction': f'Provide a short explanation of the expert setting {k}',
|
337 |
+
'output': f"{k.replace('_', ' ')} config.toml: {comment or title}".replace("\n", ""),
|
338 |
+
},
|
339 |
+
{
|
340 |
+
'prompt_type': 'human_bot',
|
341 |
+
'instruction': f'Provide a detailed explanation of the expert setting {k}',
|
342 |
+
'output': f"{k.replace('_', ' ')} config.toml: {title}{comment}".replace("\n", ""),
|
343 |
+
},
|
344 |
+
]
|
345 |
+
)
|
346 |
+
toml_list = [x for x in toml_list if x]
|
347 |
+
with open("config.json", "wt") as f:
|
348 |
+
f.write(json.dumps(toml_list, indent=2))
|
349 |
+
except Exception as e:
|
350 |
+
print("Exception: %s" % str(e), flush=True)
|
351 |
+
|
352 |
+
|
353 |
+
def copy_tree(src, dst, follow_symlink=False):
|
354 |
+
makedirs(dst, exist_ok=True)
|
355 |
+
for (path, dirs, files) in os.walk(src, followlinks=follow_symlink):
|
356 |
+
new_path = path.replace(src, dst)
|
357 |
+
makedirs(new_path, exist_ok=True)
|
358 |
+
for file in files:
|
359 |
+
filename = os.path.join(path, file)
|
360 |
+
new_filename = os.path.join(new_path, file)
|
361 |
+
# print("%s -> %s" % (filename, new_filename))
|
362 |
+
try:
|
363 |
+
atomic_copy(filename, new_filename)
|
364 |
+
except FileNotFoundError:
|
365 |
+
pass
|
366 |
+
|
367 |
+
|
368 |
+
def atomic_move(src, dst):
|
369 |
+
try:
|
370 |
+
shutil.move(src, dst)
|
371 |
+
except (shutil.Error, FileExistsError):
|
372 |
+
pass
|
373 |
+
remove(src)
|
374 |
+
|
375 |
+
|
376 |
+
def atomic_copy(src=None, dst=None, with_permissions=True):
|
377 |
+
if os.path.isfile(dst):
|
378 |
+
return
|
379 |
+
import uuid
|
380 |
+
my_uuid = uuid.uuid4()
|
381 |
+
dst_tmp = dst + str(my_uuid)
|
382 |
+
makedirs(os.path.dirname(dst), exist_ok=True)
|
383 |
+
if with_permissions:
|
384 |
+
shutil.copy(src, dst_tmp)
|
385 |
+
else:
|
386 |
+
shutil.copyfile(src, dst_tmp)
|
387 |
+
atomic_move(dst_tmp, dst)
|
388 |
+
remove(dst_tmp)
|
389 |
+
|
390 |
+
|
391 |
+
def makedirs(path, exist_ok=True):
|
392 |
+
"""
|
393 |
+
Avoid some inefficiency in os.makedirs()
|
394 |
+
:param path:
|
395 |
+
:param exist_ok:
|
396 |
+
:return:
|
397 |
+
"""
|
398 |
+
if os.path.isdir(path) and os.path.exists(path):
|
399 |
+
assert exist_ok, "Path already exists"
|
400 |
+
return path
|
401 |
+
os.makedirs(path, exist_ok=exist_ok)
|
402 |
+
|
403 |
+
|
404 |
+
## Download from https://huggingface.co/datasets/anon8231489123/ShareGPT_Vicuna_unfiltered/resolve/main/ShareGPT_unfiltered_cleaned_split.json
|
405 |
+
## Turn into simple instruct prompt type. No context/previous conversations.
|
406 |
+
def test_prep_instruct_vicuna():
|
407 |
+
from datasets import load_dataset
|
408 |
+
filename = 'ShareGPT_unfiltered_cleaned_split.json'
|
409 |
+
if not os.path.exists(filename):
|
410 |
+
os.system(
|
411 |
+
'wget https://huggingface.co/datasets/anon8231489123/ShareGPT_Vicuna_unfiltered/resolve/main/%s' % filename)
|
412 |
+
data = load_dataset("json", data_files={"train": filename})["train"]
|
413 |
+
training_rows = []
|
414 |
+
for i in range(data.num_rows):
|
415 |
+
conversations = data[i]['conversations']
|
416 |
+
assert isinstance(conversations, list), conversations
|
417 |
+
convo = ""
|
418 |
+
for j, conv in enumerate(conversations):
|
419 |
+
# Get ready for generate.py prompt_type=human_bot
|
420 |
+
# But train with prompt_type=plain
|
421 |
+
if conv['from'] == 'human':
|
422 |
+
FROM = '<human>: '
|
423 |
+
elif conv['from'] == 'gpt':
|
424 |
+
FROM = '<bot>: '
|
425 |
+
convo += f"{FROM}" + conv['value'] + "\n"
|
426 |
+
if convo:
|
427 |
+
training_rows.append(dict(input=convo))
|
428 |
+
with open(filename + ".generate_human_bot.train_plain.json", "wt") as f:
|
429 |
+
f.write(json.dumps(training_rows, indent=2))
|
430 |
+
|
431 |
+
|
432 |
+
POSTFIX = ".generate_human_bot.train_plain.json"
|
433 |
+
|
434 |
+
# https://bair.berkeley.edu/blog/2023/04/03/koala/
|
435 |
+
OIG_DATASETS = [
|
436 |
+
"unified_chip2.jsonl",
|
437 |
+
"unified_grade_school_math_instructions.jsonl",
|
438 |
+
"unified_poetry_2_song.jsonl",
|
439 |
+
"unified_plot_screenplay_books_dialog.jsonl",
|
440 |
+
]
|
441 |
+
|
442 |
+
# hub issue: https://huggingface.co/datasets/laion/OIG/discussions/4
|
443 |
+
ALL_OIG_DATASETS = ['unified_abstract_infill.jsonl',
|
444 |
+
'unified_basic.jsonl',
|
445 |
+
'unified_canadian_parliament.jsonl',
|
446 |
+
'unified_chip2.jsonl',
|
447 |
+
'unified_conv_finqa.jsonl',
|
448 |
+
'unified_cuad.jsonl',
|
449 |
+
'unified_essays.jsonl',
|
450 |
+
'unified_flan.jsonl.gz',
|
451 |
+
'unified_grade_school_math_instructions.jsonl',
|
452 |
+
'unified_hc3_human.jsonl',
|
453 |
+
'unified_image_prompts_instructions.jsonl',
|
454 |
+
'unified_joke_explanations.jsonl',
|
455 |
+
'unified_mathqa_flanv2_kojma_cot.jsonl',
|
456 |
+
'unified_merged_code_xp3.jsonl',
|
457 |
+
'unified_multi_news.jsonl',
|
458 |
+
'unified_multi_sum.jsonl',
|
459 |
+
'unified_ni.jsonl.gz',
|
460 |
+
'unified_nq.jsonl',
|
461 |
+
'unified_openai_summarize_tldr.jsonl',
|
462 |
+
'unified_oscar_en_sample_dialog.jsonl',
|
463 |
+
'unified_p3.jsonl.gz',
|
464 |
+
'unified_plot_screenplay_books_dialog.jsonl',
|
465 |
+
'unified_poetry_2_song.jsonl',
|
466 |
+
'unified_poetry_instructions.jsonl',
|
467 |
+
'unified_rallio_safety_and_prosocial.jsonl',
|
468 |
+
'unified_rallio_soda_upgraded_2048.jsonl',
|
469 |
+
'unified_soda_dialog.jsonl',
|
470 |
+
'unified_sqlv1.jsonl',
|
471 |
+
'unified_sqlv2.jsonl',
|
472 |
+
'unified_squad_v2.jsonl',
|
473 |
+
'unified_squad_v2_more_neg.jsonl',
|
474 |
+
'unified_ul2_plus_oscar_en_sample_dialog.jsonl',
|
475 |
+
'unified_unifiedskg_instructions.jsonl',
|
476 |
+
'unified_unnatural_instructions.jsonl',
|
477 |
+
'unified_xp3_sample.jsonl']
|
478 |
+
|
479 |
+
useful_oig_files = ['unified_rallio_safety_and_prosocial.jsonl.parquet',
|
480 |
+
'unified_chip2.jsonl.parquet',
|
481 |
+
'unified_cuad.jsonl.parquet',
|
482 |
+
'unified_essays.jsonl.parquet',
|
483 |
+
'unified_flan.jsonl.gz.parquet',
|
484 |
+
'unified_grade_school_math_instructions.jsonl.parquet',
|
485 |
+
'unified_hc3_human.jsonl.parquet',
|
486 |
+
'unified_mathqa_flanv2_kojma_cot.jsonl.parquet',
|
487 |
+
'unified_merged_code_xp3.jsonl.parquet',
|
488 |
+
'unified_multi_news.jsonl.parquet',
|
489 |
+
# 'unified_multi_sum.jsonl.parquet'
|
490 |
+
'unified_ni.jsonl.gz.parquet',
|
491 |
+
'unified_openai_summarize_tldr.jsonl.parquet',
|
492 |
+
# 'unified_oscar_en_sample_dialog.jsonl.parquet', # create text containing these N words, not specific
|
493 |
+
'unified_plot_screenplay_books_dialog.jsonl.parquet',
|
494 |
+
'unified_soda_dialog.jsonl.parquet',
|
495 |
+
'unified_unnatural_instructions.jsonl.parquet',
|
496 |
+
]
|
497 |
+
|
498 |
+
|
499 |
+
@pytest.mark.parametrize("filename", OIG_DATASETS)
|
500 |
+
def test_get_small_sample_oig_data(filename):
|
501 |
+
if not os.path.exists(filename):
|
502 |
+
os.system('wget https://huggingface.co/datasets/laion/OIG/resolve/main/%s' % filename)
|
503 |
+
import json
|
504 |
+
rows = []
|
505 |
+
with open(filename, "r") as f:
|
506 |
+
for line in f.readlines():
|
507 |
+
row = json.loads(line)
|
508 |
+
rows.append(dict(input=row["text"]))
|
509 |
+
with open(filename + POSTFIX, "w") as f:
|
510 |
+
f.write(json.dumps(rows, indent=2))
|
511 |
+
|
512 |
+
|
513 |
+
@pytest.mark.parametrize("filename", ALL_OIG_DATASETS)
|
514 |
+
def test_download_useful_data_as_parquet(filename):
|
515 |
+
dest_file = filename + '.parquet'
|
516 |
+
if dest_file not in useful_oig_files:
|
517 |
+
pytest.skip('file declared not useful')
|
518 |
+
if not os.path.exists(filename):
|
519 |
+
os.system('wget https://huggingface.co/datasets/laion/OIG/resolve/main/%s' % filename)
|
520 |
+
if not os.path.exists(dest_file):
|
521 |
+
df = pd.read_json(path_or_buf=filename, lines=True)
|
522 |
+
df.to_parquet(dest_file, index=False)
|
523 |
+
|
524 |
+
|
525 |
+
def test_merge_shuffle_small_sample_oig_data():
|
526 |
+
np.random.seed(1234)
|
527 |
+
rows = []
|
528 |
+
for filename in OIG_DATASETS:
|
529 |
+
with open(filename + POSTFIX, "r") as f:
|
530 |
+
rows.extend(json.loads(f.read()))
|
531 |
+
np.random.shuffle(rows)
|
532 |
+
with open("merged_shuffled_OIG_%s.json" % hashlib.sha256(str(OIG_DATASETS).encode()).hexdigest()[:10], "w") as f:
|
533 |
+
f.write(json.dumps(rows, indent=2))
|
534 |
+
|
535 |
+
|
536 |
+
def test_join_jsons():
|
537 |
+
files = ['config.json'] * 1 + \
|
538 |
+
['dai_docs.train_cleaned.json'] * 2 + \
|
539 |
+
['dai_faq.json'] * 3
|
540 |
+
print(files)
|
541 |
+
lst = []
|
542 |
+
[lst.extend(json.load(open(fil, 'rt'))) for fil in files]
|
543 |
+
print(len(lst))
|
544 |
+
json.dump(lst, open("merged.json", "wt"), indent=2)
|
545 |
+
|
546 |
+
|
547 |
+
@pytest.mark.parametrize("filename", ['Anthropic/hh-rlhf'])
|
548 |
+
def test_make_rlhf_good_data(filename):
|
549 |
+
from datasets import load_dataset
|
550 |
+
rows = load_dataset(filename)["train"]["chosen"]
|
551 |
+
new_rows = []
|
552 |
+
for row in rows:
|
553 |
+
if row[:2] == "\n\n":
|
554 |
+
row = row[2:]
|
555 |
+
row = row.replace("Human: ", "<human>: ")
|
556 |
+
row = row.replace("Assistant: ", "<bot>: ")
|
557 |
+
new_rows.append(dict(input=row))
|
558 |
+
with open(filename.replace("/", "_") + POSTFIX, "w") as f:
|
559 |
+
f.write(json.dumps(new_rows, indent=2))
|
560 |
+
|
561 |
+
|
562 |
+
def test_show_prompts():
|
563 |
+
files = ['config.json'] * 1 + \
|
564 |
+
['dai_docs.train_cleaned.json'] * 1 + \
|
565 |
+
['dai_faq.json'] * 1
|
566 |
+
file_points = [json.load(open(fil, 'rt')) for fil in files]
|
567 |
+
from prompter import generate_prompt
|
568 |
+
for data_points in file_points:
|
569 |
+
for data_point in data_points:
|
570 |
+
print(generate_prompt(data_point, 'plain', '', False, False, False)[0])
|
571 |
+
|
572 |
+
|
573 |
+
def test_get_open_datasets():
|
574 |
+
# HF changed things so don't get raw list of all datasets, so not have to filter, but can't do negative filter
|
575 |
+
open_tags = ['license:Apache License 2.0',
|
576 |
+
'license:mit',
|
577 |
+
'license:apache',
|
578 |
+
'license:apache2',
|
579 |
+
'license:apache-2.0',
|
580 |
+
'license:bsd',
|
581 |
+
'license:bsd-2-clause',
|
582 |
+
'license:bsd-3-clause',
|
583 |
+
'license:bsd-3-clause-clear',
|
584 |
+
'license:lgpl-2.1',
|
585 |
+
'license:lgpl-3.0',
|
586 |
+
'license:lgpl-lr',
|
587 |
+
'license:lgpl',
|
588 |
+
'license:openrail++',
|
589 |
+
'license:openrail',
|
590 |
+
'license:bigscience-bloom-rail-1.0',
|
591 |
+
# 'license:agpl-3.0',
|
592 |
+
'license:other',
|
593 |
+
'license:unknown',
|
594 |
+
# 'license:mpl-2.0', # ok, but would have to include original copyright, license, source, copies in distribution
|
595 |
+
# Attribution required:
|
596 |
+
'license:odc-by',
|
597 |
+
'license:cc-by-4.0',
|
598 |
+
'license:cc-by-3.0',
|
599 |
+
'license:cc-by-2.0',
|
600 |
+
'license:cc-by-2.5',
|
601 |
+
# 'license:cc-by-sa-4.0', # would require same license
|
602 |
+
'license:odbl',
|
603 |
+
'license:pddl',
|
604 |
+
'license:ms-pl',
|
605 |
+
'license:zlib',
|
606 |
+
]
|
607 |
+
# bad license: cc-by-nc-4.0
|
608 |
+
|
609 |
+
from huggingface_hub import list_datasets
|
610 |
+
datasets = flatten_list([[x for x in list_datasets(filter=y)] for y in open_tags])
|
611 |
+
datasets += [x for x in list_datasets(author='openai')]
|
612 |
+
# check all:
|
613 |
+
all_license_tags = set(flatten_list([[y for y in x.tags if 'license' in y] for x in datasets]))
|
614 |
+
print(len(all_license_tags))
|
615 |
+
open_datasets = [x for x in datasets if any([y in x.tags for y in open_tags]) or 'license:' not in str(x.tags)]
|
616 |
+
print('open_datasets', len(open_datasets))
|
617 |
+
all_task_tags = set(flatten_list([[y for y in x.tags if 'task' in y] for x in open_datasets]))
|
618 |
+
print('all_task_tags', len(all_task_tags))
|
619 |
+
excluded_tags = ['image', 'hate', 'tabular', 'table-', 'classification', 'retrieval',
|
620 |
+
'translation', 'identification', 'object', 'mask', 'to-text',
|
621 |
+
'face-detection', 'audio', 'voice', 'reinforcement', 'depth-est',
|
622 |
+
'forecasting', 'parsing', 'visual', 'speech', 'multiple-choice',
|
623 |
+
'slot-filling', 'irds/argsme', '-scoring', 'other', 'graph-ml',
|
624 |
+
'feature-extraction', 'keyword-spotting',
|
625 |
+
'coreference-resolution', 'segmentation',
|
626 |
+
'word-sense-disambiguation',
|
627 |
+
'lemmatization']
|
628 |
+
task_tags = [x.replace('task_categories:', '').replace('task_ids:', '')
|
629 |
+
for x in all_task_tags if not any([y in x for y in
|
630 |
+
excluded_tags])]
|
631 |
+
print('task_tags', len(task_tags))
|
632 |
+
# str(x.tags) to catch any pattern match to anything in list
|
633 |
+
open_tasked_datasets = [x for x in open_datasets if
|
634 |
+
any([y in str([x for x in x.tags if 'task' in x]) for y in task_tags]) and
|
635 |
+
not any([y in str([x for x in x.tags if 'task' in x]) for y in excluded_tags]) or
|
636 |
+
'task_categories' not in str(x.tags) and 'task_ids' not in str(x.tags)]
|
637 |
+
open_tasked_datasets = [x for x in open_tasked_datasets if not x.disabled]
|
638 |
+
open_tasked_datasets = [x for x in open_tasked_datasets if not x.gated]
|
639 |
+
open_tasked_datasets = [x for x in open_tasked_datasets if not x.private]
|
640 |
+
print('open_tasked_datasets', len(open_tasked_datasets))
|
641 |
+
sizes = list(set(flatten_list([[(y, x.id) for y in x.tags if 'size' in y] for x in open_tasked_datasets])))
|
642 |
+
languages = list(set(flatten_list([[(y, x.id) for y in x.tags if 'language:' in y] for x in open_tasked_datasets])))
|
643 |
+
open_english_tasked_datasets = [x for x in open_tasked_datasets if
|
644 |
+
'language:' not in str(x.tags) or
|
645 |
+
'language:en' in str(x.tags)]
|
646 |
+
small_open_english_tasked_datasets = [x for x in open_english_tasked_datasets if
|
647 |
+
'n<1K' in str(x.tags) or
|
648 |
+
'1K<n<10K' in str(x.tags) or
|
649 |
+
'1K0<n<100K' in str(x.tags) or
|
650 |
+
'100K<n<1M' in str(x.tags) or
|
651 |
+
'size_category' not in str(x.tags)
|
652 |
+
]
|
653 |
+
# 'aeslc' : email_body, subject -> summarization?
|
654 |
+
# load_dataset(open_tasked_datasets[0].id).data['train'].to_pandas()
|
655 |
+
ids = [x.id for x in small_open_english_tasked_datasets]
|
656 |
+
|
657 |
+
# sanity checks
|
658 |
+
# https://bair.berkeley.edu/blog/2023/04/03/koala/
|
659 |
+
assert 'alespalla/chatbot_instruction_prompts' in ids
|
660 |
+
assert 'laion/OIG' in ids
|
661 |
+
assert 'openai/webgpt_comparisons' in ids
|
662 |
+
assert 'openai/summarize_from_feedback' in ids
|
663 |
+
assert 'Anthropic/hh-rlhf' in ids
|
664 |
+
|
665 |
+
# useful but not allowed for commercial purposes:
|
666 |
+
# https://huggingface.co/datasets/squad
|
667 |
+
|
668 |
+
print('open_english_tasked_datasets: ', ids, flush=True)
|
669 |
+
|
670 |
+
exclude_ids = ['allenai/nllb', # translation only
|
671 |
+
'hf-internal-testing/fixtures_image_utils', # testing
|
672 |
+
'allenai/c4', # search-url
|
673 |
+
'agemagician/uniref50', # unknown
|
674 |
+
'huggingface-course/documentation-images', # images
|
675 |
+
'smilegate-ai/kor_unsmile', # korean
|
676 |
+
'MohamedRashad/ChatGPT-prompts', # ChatGPT/LearnGPT/https://www.emergentmind.com/
|
677 |
+
'humarin/chatgpt-paraphrases', # Paraphrase using ChatGPT
|
678 |
+
'Jeska/vaccinchat', # not useful
|
679 |
+
'alespalla/chatbot_instruction_prompts', # mixes alpaca
|
680 |
+
'allenai/prosocial-dialog',
|
681 |
+
# already exlucded, but wrongly in other datasets that say more permissive license
|
682 |
+
'AlekseyKorshuk/persona-chat', # low quality
|
683 |
+
'bavard/personachat_truecased', # low quality
|
684 |
+
'adamlin/daily_dialog', # medium quality conversations
|
685 |
+
'adamlin/FewShotWoz', # low quality
|
686 |
+
'benjaminbeilharz/better_daily_dialog', # low quality
|
687 |
+
'benjaminbeilharz/daily_dialog_w_turn_templates', # low
|
688 |
+
'benjaminbeilharz/empathetic_dialogues_for_lm', # low
|
689 |
+
'GEM-submissions/GEM__bart_base_schema_guided_dialog__1645547915', # NA
|
690 |
+
'ia-bentebib/conv_ai_2_fr', # low fr
|
691 |
+
'ia-bentebib/daily_dialog_fr', # low fr
|
692 |
+
'ia-bentebib/dialog_re_fr', # low fr
|
693 |
+
'ia-bentebib/empathetic_dialogues_fr', # low fr
|
694 |
+
'roskoN/dailydialog', # low
|
695 |
+
'VadorMazer/skyrimdialogstest', # low
|
696 |
+
'bigbio/med_qa', # med specific Q/A
|
697 |
+
'biu-nlp/qa_srl2018', # low quality Q/A
|
698 |
+
'biu-nlp/qa_discourse', # low quality Q/A
|
699 |
+
'iarfmoose/qa_evaluator', # low quality Q/A
|
700 |
+
'jeopardy', # low quality Q/A -- no reasoning
|
701 |
+
'narrativeqa', # low quality Q/A
|
702 |
+
'nomic-ai/gpt4all_prompt_generations', # bad license
|
703 |
+
'nomic-ai/gpt4all_prompt_generations_with_p3', # bad license
|
704 |
+
'HuggingFaceH4/alpaca', # bad license
|
705 |
+
'tatsu-lab/alpaca', # ToS breaking
|
706 |
+
'yahma/alpaca-cleaned', # ToS breaking
|
707 |
+
'Hello-SimpleAI/HC3', # bad license
|
708 |
+
'glue', # no reasoning QA
|
709 |
+
'sahil2801/CodeAlpaca-20k', # bad license
|
710 |
+
'Short-Answer-Feedback/saf_communication_networks_english', # long Q, medium A
|
711 |
+
]
|
712 |
+
small_open_english_tasked_datasets = [x for x in small_open_english_tasked_datasets if x.id not in exclude_ids]
|
713 |
+
# some ids clearly speech related
|
714 |
+
small_open_english_tasked_datasets = [x for x in small_open_english_tasked_datasets if 'speech' not in x.id]
|
715 |
+
# HF testing
|
716 |
+
small_open_english_tasked_datasets = [x for x in small_open_english_tasked_datasets if
|
717 |
+
'hf-internal-testing' not in x.id]
|
718 |
+
small_open_english_tasked_datasets = [x for x in small_open_english_tasked_datasets if
|
719 |
+
'chinese' not in x.id]
|
720 |
+
|
721 |
+
sorted_small_open_english_tasked_datasets = sorted([(x.downloads, x) for x in small_open_english_tasked_datasets],
|
722 |
+
key=lambda x: x[0], reverse=True)
|
723 |
+
|
724 |
+
# NOTES:
|
725 |
+
# Run like pytest -s -v create_data.py::test_get_open_datasets &> getdata9.log
|
726 |
+
# See what needs config passed and add:
|
727 |
+
# grep 'load_dataset(' getdata9.log|grep -v data_id|less -S
|
728 |
+
# grep "pip install" getdata9.log
|
729 |
+
# NOTE: Some datasets have default config, but others are there. Don't know how to access them.
|
730 |
+
|
731 |
+
"""
|
732 |
+
https://huggingface.co/datasets/wikihow/blob/main/wikihow.py
|
733 |
+
https://github.com/mahnazkoupaee/WikiHow-Dataset
|
734 |
+
https://ucsb.box.com/s/ap23l8gafpezf4tq3wapr6u8241zz358
|
735 |
+
https://ucsb.app.box.com/s/ap23l8gafpezf4tq3wapr6u8241zz358
|
736 |
+
"""
|
737 |
+
|
738 |
+
"""
|
739 |
+
# some ambiguous or non-commercial datasets
|
740 |
+
https://github.com/PhoebusSi/alpaca-CoT
|
741 |
+
"""
|
742 |
+
|
743 |
+
timeout = 3 * 60
|
744 |
+
# laion/OIG takes longer
|
745 |
+
for num_downloads, dataset in sorted_small_open_english_tasked_datasets:
|
746 |
+
data_id = dataset.id
|
747 |
+
func = do_one
|
748 |
+
args = (data_id, num_downloads)
|
749 |
+
kwargs = {}
|
750 |
+
with ProcessPoolExecutor(max_workers=1) as executor:
|
751 |
+
future = executor.submit(func, *args, **kwargs)
|
752 |
+
try:
|
753 |
+
future.result(timeout=timeout)
|
754 |
+
except concurrent.futures.TimeoutError:
|
755 |
+
print("\n\ndata_id %s timeout\n\n" % data_id, flush=True)
|
756 |
+
for child in psutil.Process(os.getpid()).children(recursive=True):
|
757 |
+
os.kill(child.pid, signal.SIGINT)
|
758 |
+
os.kill(child.pid, signal.SIGTERM)
|
759 |
+
os.kill(child.pid, signal.SIGKILL)
|
760 |
+
|
761 |
+
|
762 |
+
def do_one(data_id, num_downloads):
|
763 |
+
from datasets import load_dataset
|
764 |
+
out_file = "data_%s.parquet" % str(data_id.replace('/', '_'))
|
765 |
+
if os.path.isfile(out_file) and os.path.getsize(out_file) > 1024 ** 3:
|
766 |
+
return
|
767 |
+
try:
|
768 |
+
print("Loading data_id %s num_downloads: %s" % (data_id, num_downloads), flush=True)
|
769 |
+
avail_list = None
|
770 |
+
try:
|
771 |
+
data = load_dataset(data_id, 'foobar')
|
772 |
+
except Exception as e:
|
773 |
+
if 'Available: ' in str(e):
|
774 |
+
avail_list = ast.literal_eval(str(e).split('Available:')[1].strip())
|
775 |
+
else:
|
776 |
+
avail_list = None
|
777 |
+
if avail_list is None:
|
778 |
+
avail_list = [None]
|
779 |
+
print("%s avail_list: %s" % (data_id, avail_list), flush=True)
|
780 |
+
|
781 |
+
for name in avail_list:
|
782 |
+
out_file = "data_%s_%s.parquet" % (str(data_id.replace('/', '_')), str(name))
|
783 |
+
if os.path.isfile(out_file):
|
784 |
+
continue
|
785 |
+
data = load_dataset(data_id, name)
|
786 |
+
column_names_dict = data.column_names
|
787 |
+
column_names = column_names_dict[list(column_names_dict.keys())[0]]
|
788 |
+
print("Processing data_id %s num_downloads: %s columns: %s" % (data_id, num_downloads, column_names),
|
789 |
+
flush=True)
|
790 |
+
data_dict = data.data
|
791 |
+
col_dict = data.num_columns
|
792 |
+
first_col = list(col_dict.keys())[0]
|
793 |
+
if 'train' in data_dict:
|
794 |
+
df = data['train'].to_pandas()
|
795 |
+
else:
|
796 |
+
df = data[first_col].to_pandas()
|
797 |
+
# csv has issues with escaping chars, even for datasets I know I want
|
798 |
+
df.to_parquet(out_file, index=False)
|
799 |
+
except Exception as e:
|
800 |
+
t, v, tb = sys.exc_info()
|
801 |
+
ex = ''.join(traceback.format_exception(t, v, tb))
|
802 |
+
print("Exception: %s %s" % (data_id, ex), flush=True)
|
803 |
+
|
804 |
+
|
805 |
+
def test_otherlic():
|
806 |
+
from huggingface_hub import list_datasets
|
807 |
+
lic = ['license:odc-by',
|
808 |
+
'license:cc-by-4.0',
|
809 |
+
'license:cc-by-3.0',
|
810 |
+
'license:cc-by-2.0',
|
811 |
+
'license:cc-by-2.5',
|
812 |
+
'license:cc-by-sa-4.0',
|
813 |
+
'license:odbl',
|
814 |
+
'license:pddl',
|
815 |
+
'license:ms-pl',
|
816 |
+
'license:zlib',
|
817 |
+
]
|
818 |
+
datasets = flatten_list([[x for x in list_datasets(filter=y) if 'translation' not in str(x.tags)] for y in lic])
|
819 |
+
print(len(datasets))
|
820 |
+
|
821 |
+
|
822 |
+
# These useful datasets are determined based upon data sample, column types, and uniqueness compared to larger datasets like Pile
|
823 |
+
# grep columns getdata13.log|grep -v "\['image'\]"|sort|uniq|grep -v tokens|grep -v "'image'"|grep -v embedding|grep dialog
|
824 |
+
useful = ['Dahoas/instruct-human-assistant-prompt',
|
825 |
+
'Dahoas/first-instruct-human-assistant-prompt',
|
826 |
+
'knkarthick/dialogsum', # summary of conversation
|
827 |
+
'McGill-NLP/FaithDial', # medium quality
|
828 |
+
'Zaid/quac_expanded', # medium quality context + QA
|
829 |
+
'0-hero/OIG-small-chip2', # medium
|
830 |
+
'alistvt/coqa-flat', # QA medium
|
831 |
+
'AnonymousSub/MedQuAD_47441_Question_Answer_Pairs', # QA medium
|
832 |
+
'Anthropic/hh-rlhf', # high quality # similar to Dahoas/full-hh-rlhf
|
833 |
+
'arjunth2001/online_privacy_qna', # good quality QA
|
834 |
+
'Dahoas/instruct_helpful_preferences', # medium quality instruct
|
835 |
+
'Dahoas/rl-prompt-dataset', # medium chat
|
836 |
+
'Dahoas/rm-static', # medium chat
|
837 |
+
'Dahoas/static-hh', # medium chat # HuggingFaceH4/self_instruct
|
838 |
+
'Dahoas/synthetic-instruct-gptj-pairwise', # medium chat
|
839 |
+
'eli5', # QA if prompt ELI5
|
840 |
+
'gsm8k', # QA (various)
|
841 |
+
'guanaco/guanaco', # prompt/response
|
842 |
+
'kastan/rlhf-qa-comparisons', # good QA
|
843 |
+
'kastan/rlhf-qa-conditional-generation-v2', # prompt answer
|
844 |
+
'OllieStanley/humaneval-mbpp-codegen-qa', # code QA, but started from words, so better than other code QA
|
845 |
+
'OllieStanley/humaneval-mbpp-testgen-qa', # code QA
|
846 |
+
'Graverman/Instruct-to-Code', # code QA
|
847 |
+
'openai/summarize_from_feedback', # summarize
|
848 |
+
'relbert/analogy_questions', # analogy QA
|
849 |
+
'yitingxie/rlhf-reward-datasets', # prompt, chosen, rejected.
|
850 |
+
'yizhongw/self_instruct', # instruct (super natural & instruct)
|
851 |
+
'HuggingFaceH4/asss', # QA, big A
|
852 |
+
'kastan/rlhf-qa-conditional-generation-v2', # QA
|
853 |
+
'cosmos_qa', # context QA
|
854 |
+
'vishal-burman/c4-faqs', # QA but not so much reasoning, but alot of text
|
855 |
+
'squadshifts', # QA from context
|
856 |
+
'hotpot_qa', # QA from context
|
857 |
+
'adversarial_qa', # QA from context
|
858 |
+
'allenai/soda', # dialog -> narrative/summary
|
859 |
+
'squad_v2', # context QA
|
860 |
+
'squadshifts', # context QA
|
861 |
+
'dferndz/cSQuAD1', # context QA
|
862 |
+
'dferndz/cSQuAD2', # context QA
|
863 |
+
'din0s/msmarco-nlgen', # context QA
|
864 |
+
'domenicrosati/TruthfulQA', # common sense truthful QA -- trivia but good trivia
|
865 |
+
'hotpot_qa', # context, QA
|
866 |
+
'HuggingFaceH4/self-instruct-eval', # instruct QA, medium quality, some language reasoning
|
867 |
+
'kastan/EE_QA_for_RLHF', # context QA
|
868 |
+
'KK04/LogicInference_OA', # instruction logical QA
|
869 |
+
'lmqg/qa_squadshifts_synthetic', # context QA
|
870 |
+
'lmqg/qg_squad', # context QA
|
871 |
+
'lmqg/qg_squadshifts', # context QA
|
872 |
+
'lmqg/qg_subjqa', # context QA
|
873 |
+
'pszemraj/HC3-textgen-qa',
|
874 |
+
# QA medium, has human responses -- humans tend to provide links instead of trying to answer
|
875 |
+
'pythonist/newdata', # long context, QA, brief A
|
876 |
+
'ropes', # long background, situation, question, A
|
877 |
+
'wikitablequestions', # table -> QA
|
878 |
+
'bigscience/p3', # context QA but short answers
|
879 |
+
]
|
880 |
+
|
881 |
+
code_useful = ['0n1xus/codexglue',
|
882 |
+
'openai_humaneval',
|
883 |
+
'koutch/staqc',
|
884 |
+
]
|
885 |
+
|
886 |
+
maybe_useful = ['AlekseyKorshuk/comedy-scripts',
|
887 |
+
'openbookqa', # hard to parse, low reasoning
|
888 |
+
'qed', # reasonable QA, but low reasoning
|
889 |
+
'selqa', # candidate answers
|
890 |
+
'HuggingFaceH4/instruction-pilot-outputs-filtered',
|
891 |
+
'GBaker/MedQA-USMLE-4-options', # medical QA with long questions
|
892 |
+
'npc-engine/light-batch-summarize-dialogue', # dialog summarize, kinda low specific quality
|
893 |
+
]
|
894 |
+
|
895 |
+
summary_useful = ['austin/rheum_abstracts',
|
896 |
+
'CarperAI/openai_summarize_comparisons', # summarize chosen/rejected
|
897 |
+
'CarperAI/openai_summarize_tldr', # summarize QA
|
898 |
+
'ccdv/cnn_dailymail', # summarize news
|
899 |
+
'ccdv/govreport-summarization', # summarize high quality
|
900 |
+
'ccdv/pubmed-summarization', # summarize high quality
|
901 |
+
'duorc', # plot -> QA
|
902 |
+
'farleyknight/big_patent_5_percent', # desc -> abstract
|
903 |
+
'multi_news', # summary
|
904 |
+
'opinosis',
|
905 |
+
'SophieTr/reddit_clean',
|
906 |
+
'allenai/mup', # long text -> summary
|
907 |
+
'allenai/multi_lexsum', # long text -> summary
|
908 |
+
'big_patent',
|
909 |
+
'allenai/wcep_dense_max',
|
910 |
+
'awinml/costco_long_practice',
|
911 |
+
'GEM/xsum',
|
912 |
+
'ratishsp/newshead',
|
913 |
+
'RussianNLP/wikiomnia', # russian
|
914 |
+
'stacked-summaries/stacked-xsum-1024',
|
915 |
+
]
|
916 |
+
|
917 |
+
math_useful = [
|
918 |
+
'competition_math'
|
919 |
+
]
|
920 |
+
|
921 |
+
skipped = ['c4', # maybe useful, used for flan, but skipped due to size
|
922 |
+
]
|
923 |
+
|
924 |
+
"""
|
925 |
+
To get training data from oig:
|
926 |
+
pytest test_oig test_grade_final test_finalize_to_json
|
927 |
+
"""
|
928 |
+
|
929 |
+
human = '<human>:'
|
930 |
+
bot = '<bot>:'
|
931 |
+
|
932 |
+
|
933 |
+
def test_assemble_and_detox():
|
934 |
+
import re
|
935 |
+
from profanity_check import predict_prob
|
936 |
+
df_list = []
|
937 |
+
for data in useful_oig_files:
|
938 |
+
print("Processing %s" % data, flush=True)
|
939 |
+
df = pd.read_parquet(data)
|
940 |
+
df = df.reset_index(drop=True)
|
941 |
+
# chop up into human/bot interactions of no more than 10kB per row
|
942 |
+
text_list = df[['text']].values.ravel().tolist()
|
943 |
+
new_text = []
|
944 |
+
max_len = 2048 # uber cutoff
|
945 |
+
MAX_LEN = 2048 // 2 - 30 # max len per question/answer
|
946 |
+
for text in tqdm(text_list):
|
947 |
+
human_starts = [m.start() for m in re.finditer('<human>: ', text)]
|
948 |
+
if len(human_starts) == 1:
|
949 |
+
human_starts = [0, len(text)] # always go into for loop below
|
950 |
+
blurb = ''
|
951 |
+
for i in range(len(human_starts) - 1):
|
952 |
+
interaction = text[human_starts[i]: human_starts[i + 1]][:max_len]
|
953 |
+
blurb += interaction
|
954 |
+
if len(blurb) >= MAX_LEN:
|
955 |
+
blurb = get_sentences(blurb, length=MAX_LEN)[0]
|
956 |
+
new_text.append(blurb + "\n<human>:")
|
957 |
+
blurb = ''
|
958 |
+
if blurb:
|
959 |
+
blurb = get_sentences(blurb, length=MAX_LEN)[0]
|
960 |
+
new_text.append(blurb + "\n<human>:")
|
961 |
+
|
962 |
+
if len(new_text) > len(text_list):
|
963 |
+
print("Added %d new rows (before: %d)" % (len(new_text) - df.shape[0], df.shape[0]))
|
964 |
+
df = pd.DataFrame({"text": new_text, "source": [data] * len(new_text)})
|
965 |
+
df = df.drop_duplicates(keep='first')
|
966 |
+
print(df['text'].apply(lambda x: len(x)).describe())
|
967 |
+
assert df['text'].apply(lambda x: len(x)).max() <= 2 * max_len
|
968 |
+
|
969 |
+
# faster than better_profanity, do early
|
970 |
+
df['profanity'] = predict_prob(df['text'])
|
971 |
+
before_rows = df.shape[0]
|
972 |
+
df = df[df['profanity'] < 0.25] # drop any low quality stuff
|
973 |
+
after_rows = df.shape[0]
|
974 |
+
print("Dropped %d rows out of %d due to alt-profanity-check" % (before_rows - after_rows, before_rows))
|
975 |
+
df_list.append(df)
|
976 |
+
print("Done processing %s -> %s rows" % (data, df.shape[0]), flush=True)
|
977 |
+
print("So far have %d rows" % sum([len(x) for x in df_list]))
|
978 |
+
df_final = pd.concat(df_list)
|
979 |
+
df_final = df_final.sample(frac=1, random_state=1234).reset_index(drop=True)
|
980 |
+
df_final.to_parquet('h2oGPT.cleaned.human_bot.shorter.parquet', index=False)
|
981 |
+
|
982 |
+
|
983 |
+
def test_basic_cleaning():
|
984 |
+
# from better_profanity import profanity
|
985 |
+
# https://pypi.org/project/alt-profanity-check/
|
986 |
+
from profanity_check import predict
|
987 |
+
df_list = []
|
988 |
+
for data in useful_oig_files:
|
989 |
+
# for data in useful_oig_files[:5]:
|
990 |
+
# for data in ['unified_openai_summarize_tldr.jsonl.parquet']:
|
991 |
+
print("Processing %s" % data, flush=True)
|
992 |
+
df = pd.read_parquet(data)
|
993 |
+
df = df.reset_index(drop=True)
|
994 |
+
# NOTE: Not correct if multiple human-bot interactions, but those dialogs even more desired
|
995 |
+
# avg_chars = len(df['text'][0])/(df['text'][0].count(human)+df['text'][0].count(bot))
|
996 |
+
df['avg_words'] = df['text'].apply(lambda x: x.count(' ') / (x.count(human) + x.count(bot)) / 2.0)
|
997 |
+
df['avg_bot_words'] = df['text'].apply(lambda x: x.split(bot)[1].count(' ') / x.count(bot))
|
998 |
+
# df['bad_words'] = df['text'].apply(lambda x: profanity.contains_profanity(x))
|
999 |
+
# low_quality_patterns = ['Write the rest of this wikipedia article']
|
1000 |
+
res = predict(df['text'])
|
1001 |
+
df['bad_words'] = res
|
1002 |
+
df = df.reset_index(drop=True)
|
1003 |
+
df = df[df['bad_words'] == 0]
|
1004 |
+
df = df[['text', 'avg_words', 'avg_bot_words']]
|
1005 |
+
df = df.drop_duplicates(keep='first')
|
1006 |
+
print(df[df['avg_words'] == df['avg_words'].max()]['text'].values)
|
1007 |
+
median_words = np.median(df['avg_words'])
|
1008 |
+
min_words_per_entity = max(30, 0.8 * median_words)
|
1009 |
+
max_words_per_entity = 2048 # too hard to learn from for now
|
1010 |
+
df = df[df['avg_words'] > min_words_per_entity]
|
1011 |
+
df = df[df['avg_words'] < max_words_per_entity]
|
1012 |
+
|
1013 |
+
min_words_per_entity = max(20, 0.5 * median_words) # bot should say stuff for now
|
1014 |
+
max_words_per_entity = 2048 # too hard to learn from for now
|
1015 |
+
df = df[df['avg_bot_words'] > min_words_per_entity]
|
1016 |
+
df = df[df['avg_bot_words'] < max_words_per_entity]
|
1017 |
+
|
1018 |
+
df_list.append(df)
|
1019 |
+
print("Done processing %s -> %s rows" % (data, df.shape[0]), flush=True)
|
1020 |
+
df_final = pd.concat(df_list)
|
1021 |
+
df_final.to_parquet('h2oGPT.cleaned.human_bot.parquet', index=False)
|
1022 |
+
|
1023 |
+
|
1024 |
+
from joblib import Parallel, delayed, effective_n_jobs
|
1025 |
+
from sklearn.utils import gen_even_slices
|
1026 |
+
from sklearn.utils.validation import _num_samples
|
1027 |
+
|
1028 |
+
|
1029 |
+
def parallel_apply(df, func, n_jobs=-1, **kwargs):
|
1030 |
+
""" Pandas apply in parallel using joblib.
|
1031 |
+
Uses sklearn.utils to partition input evenly.
|
1032 |
+
|
1033 |
+
Args:
|
1034 |
+
df: Pandas DataFrame, Series, or any other object that supports slicing and apply.
|
1035 |
+
func: Callable to apply
|
1036 |
+
n_jobs: Desired number of workers. Default value -1 means use all available cores.
|
1037 |
+
**kwargs: Any additional parameters will be supplied to the apply function
|
1038 |
+
|
1039 |
+
Returns:
|
1040 |
+
Same as for normal Pandas DataFrame.apply()
|
1041 |
+
|
1042 |
+
"""
|
1043 |
+
|
1044 |
+
if effective_n_jobs(n_jobs) == 1:
|
1045 |
+
return df.apply(func, **kwargs)
|
1046 |
+
else:
|
1047 |
+
ret = Parallel(n_jobs=n_jobs)(
|
1048 |
+
delayed(type(df).apply)(df[s], func, **kwargs)
|
1049 |
+
for s in gen_even_slices(_num_samples(df), effective_n_jobs(n_jobs)))
|
1050 |
+
return pd.concat(ret)
|
1051 |
+
|
1052 |
+
|
1053 |
+
def add_better_profanity_flag(df):
|
1054 |
+
from better_profanity import profanity
|
1055 |
+
df['better_profanity'] = parallel_apply(
|
1056 |
+
df['text'],
|
1057 |
+
lambda x: profanity.contains_profanity(x),
|
1058 |
+
n_jobs=-1,
|
1059 |
+
)
|
1060 |
+
return df
|
1061 |
+
|
1062 |
+
|
1063 |
+
def add_textstat_grade(df):
|
1064 |
+
import textstat
|
1065 |
+
|
1066 |
+
def myfunc(x):
|
1067 |
+
return textstat.flesch_kincaid_grade(x) # simple grade
|
1068 |
+
|
1069 |
+
if False:
|
1070 |
+
import dask.dataframe as dd
|
1071 |
+
# 40 seconds for 1000 rows, but have 1,787,799 rows
|
1072 |
+
ddata = dd.from_pandas(df, npartitions=120)
|
1073 |
+
|
1074 |
+
df['flesch_grade'] = ddata['text'].apply(myfunc).compute()
|
1075 |
+
if True:
|
1076 |
+
# fast way
|
1077 |
+
df['flesch_grade'] = parallel_apply(df['text'], myfunc, n_jobs=-1)
|
1078 |
+
return df
|
1079 |
+
|
1080 |
+
|
1081 |
+
def add_deberta_grade(df):
|
1082 |
+
from transformers import AutoModelForSequenceClassification, AutoTokenizer
|
1083 |
+
import torch
|
1084 |
+
reward_name = "OpenAssistant/reward-model-deberta-v3-large-v2"
|
1085 |
+
rank_model, tokenizer = AutoModelForSequenceClassification.from_pretrained(
|
1086 |
+
reward_name), AutoTokenizer.from_pretrained(reward_name)
|
1087 |
+
device = 'cuda' if torch.cuda.is_available() else 'cpu'
|
1088 |
+
rank_model.to(device)
|
1089 |
+
|
1090 |
+
def get_question(x):
|
1091 |
+
return x.replace('<human>: ', '').split('<bot>:')[0]
|
1092 |
+
|
1093 |
+
def get_answer(x):
|
1094 |
+
try:
|
1095 |
+
answer = x.split('<bot>: ')[1].split('<human>:')[0].replace('<bot>: ', '')
|
1096 |
+
except:
|
1097 |
+
answer = x.split('<bot>:')[1].split('<human>:')[0].replace('<bot>:', '')
|
1098 |
+
return answer
|
1099 |
+
|
1100 |
+
df['question'] = parallel_apply(df['text'], get_question, n_jobs=-1)
|
1101 |
+
df['answer'] = parallel_apply(df['text'], get_answer, n_jobs=-1)
|
1102 |
+
|
1103 |
+
from datasets import Dataset
|
1104 |
+
from transformers import pipeline
|
1105 |
+
from transformers.pipelines.pt_utils import KeyPairDataset
|
1106 |
+
import tqdm
|
1107 |
+
|
1108 |
+
pipe = pipeline(
|
1109 |
+
"text-classification",
|
1110 |
+
model=reward_name,
|
1111 |
+
device="cuda:0" if torch.cuda.is_available() else "cpu"
|
1112 |
+
)
|
1113 |
+
start = 0
|
1114 |
+
batch_size = 64 * 16
|
1115 |
+
micro_batch = orig_micro_batch = 16
|
1116 |
+
end = 0
|
1117 |
+
import socket
|
1118 |
+
checkpoint = "grades.%s.pkl" % socket.gethostname()
|
1119 |
+
grades = []
|
1120 |
+
import pickle
|
1121 |
+
if os.path.exists(checkpoint):
|
1122 |
+
with open(checkpoint, "rb") as f:
|
1123 |
+
start, grades = pickle.loads(f.read())
|
1124 |
+
last_oom = 0
|
1125 |
+
while end < df.shape[0]:
|
1126 |
+
# manual batching to handle OOM more gracefully
|
1127 |
+
end = min(start + batch_size, df.shape[0])
|
1128 |
+
if start == end:
|
1129 |
+
break
|
1130 |
+
dataset = Dataset.from_pandas(df.iloc[start:end, :])
|
1131 |
+
try:
|
1132 |
+
grades.extend([
|
1133 |
+
x['score'] for x in tqdm.tqdm(
|
1134 |
+
pipe(KeyPairDataset(dataset, "question", "answer"), batch_size=micro_batch)
|
1135 |
+
)
|
1136 |
+
])
|
1137 |
+
except torch.cuda.OutOfMemoryError:
|
1138 |
+
last_oom = start
|
1139 |
+
micro_batch = max(1, micro_batch // 2)
|
1140 |
+
print("OOM - retrying with micro_batch=%d" % micro_batch)
|
1141 |
+
continue
|
1142 |
+
if last_oom == start:
|
1143 |
+
micro_batch = orig_micro_batch
|
1144 |
+
print("Returning to micro_batch=%d" % micro_batch)
|
1145 |
+
assert len(grades) == end
|
1146 |
+
start = end
|
1147 |
+
with open(checkpoint, "wb") as f:
|
1148 |
+
f.write(pickle.dumps((end, grades)))
|
1149 |
+
print("%d/%d" % (end, df.shape[0]))
|
1150 |
+
df['grade_deberta'] = grades
|
1151 |
+
if os.path.exists(checkpoint):
|
1152 |
+
os.remove(checkpoint)
|
1153 |
+
return df
|
1154 |
+
|
1155 |
+
|
1156 |
+
def test_chop_by_lengths():
|
1157 |
+
file = "h2oGPT.cleaned.human_bot.shorter.parquet"
|
1158 |
+
df = pd.read_parquet(file).reset_index(drop=True)
|
1159 |
+
df = count_human_bot_lengths(df)
|
1160 |
+
df['rand'] = np.random.rand(df.shape[0])
|
1161 |
+
df['rand2'] = np.random.rand(df.shape[0])
|
1162 |
+
before_rows = df.shape[0]
|
1163 |
+
# throw away short human/bot responses with higher likelihood
|
1164 |
+
df = df[(df['len_human_mean'] > 20)] # never keep very short ones
|
1165 |
+
df = df[(df['len_human_mean'] > 30) | (df['rand'] < 0.2)]
|
1166 |
+
df = df[(df['len_human_mean'] > 50) | (df['rand'] < 0.5)]
|
1167 |
+
df = df[(df['len_human_max'] < 10000)] # drop super long (basically only human) ones
|
1168 |
+
df = df[(df['len_bot_mean'] > 20)] # never keep very short ones
|
1169 |
+
df = df[(df['len_bot_mean'] > 30) | (df['rand2'] < 0.2)]
|
1170 |
+
df = df[(df['len_bot_mean'] > 50) | (df['rand2'] < 0.5)]
|
1171 |
+
df = df[(df['len_bot_max'] < 10000)] # drop super long (only bot) ones
|
1172 |
+
assert df['text'].apply(lambda x: len(x)).max() < 20000
|
1173 |
+
df = df.drop(['rand', 'rand2'], axis=1)
|
1174 |
+
after_rows = df.shape[0]
|
1175 |
+
print("Chopped off %d out of %d rows due to length" % (before_rows - after_rows, before_rows))
|
1176 |
+
print(df.describe())
|
1177 |
+
df.to_parquet('h2oGPT.cleaned.chopped.human_bot.shorter.parquet', index=False)
|
1178 |
+
|
1179 |
+
|
1180 |
+
def count_human_bot_lengths(df, human=None, bot=None):
|
1181 |
+
import re
|
1182 |
+
len_human_min = []
|
1183 |
+
len_human_max = []
|
1184 |
+
len_human_mean = []
|
1185 |
+
len_bot_min = []
|
1186 |
+
len_bot_max = []
|
1187 |
+
len_bot_mean = []
|
1188 |
+
human = human or '<human>:'
|
1189 |
+
bot = bot or '<bot>:'
|
1190 |
+
for is_human in [True, False]:
|
1191 |
+
what = human if is_human else bot
|
1192 |
+
other = human if not is_human else bot
|
1193 |
+
for i in range(df.shape[0]):
|
1194 |
+
text = df.loc[i, 'text']
|
1195 |
+
assert isinstance(text, str)
|
1196 |
+
starts = [m.start() for m in re.finditer(what, text)]
|
1197 |
+
if len(starts) == 1:
|
1198 |
+
starts = [starts[0], len(text)] # always go into for loop below
|
1199 |
+
assert len(text)
|
1200 |
+
list_what = []
|
1201 |
+
for ii in range(len(starts) - 1):
|
1202 |
+
interaction = text[starts[ii]: starts[ii + 1]]
|
1203 |
+
if other in interaction:
|
1204 |
+
interaction = interaction[:interaction.find(other)]
|
1205 |
+
interaction.strip()
|
1206 |
+
list_what.append(interaction)
|
1207 |
+
if not list_what:
|
1208 |
+
list_what = [''] # handle corrupted data, very rare, leads to sizes 0
|
1209 |
+
if is_human:
|
1210 |
+
len_human_min.append(min([len(x) for x in list_what]))
|
1211 |
+
len_human_max.append(max([len(x) for x in list_what]))
|
1212 |
+
len_human_mean.append(np.mean([len(x) for x in list_what]))
|
1213 |
+
else:
|
1214 |
+
len_bot_min.append(min([len(x) for x in list_what]))
|
1215 |
+
len_bot_max.append(max([len(x) for x in list_what]))
|
1216 |
+
len_bot_mean.append(np.mean([len(x) for x in list_what]))
|
1217 |
+
df['len_human_min'] = len_human_min
|
1218 |
+
df['len_human_max'] = len_human_max
|
1219 |
+
df['len_human_mean'] = len_human_mean
|
1220 |
+
df['len_bot_min'] = len_bot_min
|
1221 |
+
df['len_bot_max'] = len_bot_max
|
1222 |
+
df['len_bot_mean'] = len_bot_mean
|
1223 |
+
np.random.seed(1234)
|
1224 |
+
pd.set_option('display.max_columns', None)
|
1225 |
+
print("Before chopping")
|
1226 |
+
print(df.describe())
|
1227 |
+
return df
|
1228 |
+
|
1229 |
+
|
1230 |
+
def test_grade():
|
1231 |
+
df = None
|
1232 |
+
|
1233 |
+
file = "h2oGPT.cleaned.chopped.human_bot.shorter.parquet"
|
1234 |
+
output_file = "h2oGPT.cleaned.graded1.human_bot.shorter.parquet"
|
1235 |
+
if not os.path.exists(output_file):
|
1236 |
+
if df is None:
|
1237 |
+
df = pd.read_parquet(file).reset_index(drop=True)
|
1238 |
+
df = add_textstat_grade(df)
|
1239 |
+
min_grade = 10
|
1240 |
+
max_grade = 25
|
1241 |
+
df = df[df['flesch_grade'] >= min_grade]
|
1242 |
+
df = df[df['flesch_grade'] <= max_grade]
|
1243 |
+
print("After Flesch grade")
|
1244 |
+
print(df.describe())
|
1245 |
+
df.to_parquet(output_file, index=False)
|
1246 |
+
|
1247 |
+
file = output_file
|
1248 |
+
output_file = "h2oGPT.cleaned.graded2.human_bot.shorter.parquet"
|
1249 |
+
if not os.path.exists(output_file):
|
1250 |
+
# slower than alt-profanity, do last, but do before deberta grading, since that's slower
|
1251 |
+
if df is None:
|
1252 |
+
df = pd.read_parquet(file).reset_index(drop=True)
|
1253 |
+
df = add_better_profanity_flag(df)
|
1254 |
+
before_rows = df.shape[0]
|
1255 |
+
df = df[df['better_profanity'] == 0]
|
1256 |
+
df = df.drop(['better_profanity'], axis=1)
|
1257 |
+
after_rows = df.shape[0]
|
1258 |
+
print("Dropped %d rows out of %d due to better_profanity" % (before_rows - after_rows, before_rows))
|
1259 |
+
print(df.describe())
|
1260 |
+
df.to_parquet(output_file, index=False)
|
1261 |
+
|
1262 |
+
file = output_file
|
1263 |
+
output_file = 'h2oGPT.cleaned.graded3.human_bot.shorter.parquet'
|
1264 |
+
if not os.path.exists(output_file):
|
1265 |
+
if df is None:
|
1266 |
+
df = pd.read_parquet(file).reset_index(drop=True)
|
1267 |
+
df = add_deberta_grade(df)
|
1268 |
+
min_grade = 0.3
|
1269 |
+
max_grade = np.inf
|
1270 |
+
before_rows = df.shape[0]
|
1271 |
+
df = df[df['grade_deberta'] >= min_grade]
|
1272 |
+
df = df[df['grade_deberta'] <= max_grade]
|
1273 |
+
after_rows = df.shape[0]
|
1274 |
+
print("Dropped %d rows out of %d due to deberta grade" % (before_rows - after_rows, before_rows))
|
1275 |
+
print("After DeBERTa grade")
|
1276 |
+
print(df.describe())
|
1277 |
+
df.to_parquet(output_file, index=False)
|
1278 |
+
|
1279 |
+
file = output_file
|
1280 |
+
output_file = 'h2oGPT.cleaned.graded.human_bot.shorter.parquet'
|
1281 |
+
if df is None:
|
1282 |
+
df = pd.read_parquet(file).reset_index(drop=True)
|
1283 |
+
df.to_parquet(output_file, index=False)
|
1284 |
+
|
1285 |
+
|
1286 |
+
@pytest.mark.parametrize(
|
1287 |
+
"fixup_personality, only_personality, deberta_grading",
|
1288 |
+
[
|
1289 |
+
[False, False, False],
|
1290 |
+
[True, True, False],
|
1291 |
+
[True, False, False],
|
1292 |
+
[True, False, True],
|
1293 |
+
]
|
1294 |
+
)
|
1295 |
+
def test_add_open_assistant(fixup_personality, only_personality, deberta_grading, save_json=True):
|
1296 |
+
"""
|
1297 |
+
Flatten tree structure into one row per path from root to leaf
|
1298 |
+
Also turn into human_bot prompting format:
|
1299 |
+
<human>: question\n<bot>: answer <human>: question2\n<bot>: answer2 Etc.
|
1300 |
+
Also saves a .json locally as side-effect
|
1301 |
+
returns list of dicts, containing intput, prompt_type and source
|
1302 |
+
"""
|
1303 |
+
from datasets import load_dataset
|
1304 |
+
data_file = "OpenAssistant/oasst1"
|
1305 |
+
ds = load_dataset(data_file)
|
1306 |
+
df = pd.concat([ds['train'].to_pandas(), ds['validation'].to_pandas()], axis=0)
|
1307 |
+
rows = {}
|
1308 |
+
message_ids = df['message_id'].values.tolist()
|
1309 |
+
message_tree_ids = df['message_tree_id'].values.tolist()
|
1310 |
+
parent_ids = df['parent_id'].values.tolist()
|
1311 |
+
texts = df['text'].values.tolist()
|
1312 |
+
roles = df['role'].values.tolist()
|
1313 |
+
|
1314 |
+
for i in range(df.shape[0]):
|
1315 |
+
# collect all trees
|
1316 |
+
message_id = message_ids[i]
|
1317 |
+
message_tree_id = message_tree_ids[i]
|
1318 |
+
parent_id = parent_ids[i]
|
1319 |
+
text = texts[i]
|
1320 |
+
if fixup_personality:
|
1321 |
+
text = text.replace("Open Assistant", "h2oGPT")
|
1322 |
+
text = text.replace("Open-Assistant", "h2oGPT")
|
1323 |
+
text = text.replace("open-assistant", "h2oGPT")
|
1324 |
+
text = text.replace("OpenAssistant", "h2oGPT")
|
1325 |
+
text = text.replace("open assistant", "h2oGPT")
|
1326 |
+
text = text.replace("Open Assistand", "h2oGPT")
|
1327 |
+
text = text.replace("Open Assitant", "h2oGPT")
|
1328 |
+
text = text.replace("Open Assistent", "h2oGPT")
|
1329 |
+
text = text.replace("Open Assisstant", "h2oGPT")
|
1330 |
+
text = text.replace("Open Assitent", "h2oGPT")
|
1331 |
+
text = text.replace("Open Assitiant", "h2oGPT")
|
1332 |
+
text = text.replace("Open Assistiant", "h2oGPT")
|
1333 |
+
text = text.replace("Open Assitan ", "h2oGPT ")
|
1334 |
+
text = text.replace("Open Assistan ", "h2oGPT ")
|
1335 |
+
text = text.replace("Open Asistant", "h2oGPT")
|
1336 |
+
text = text.replace("Open Assiant", "h2oGPT")
|
1337 |
+
text = text.replace("Assistant", "h2oGPT")
|
1338 |
+
text = text.replace("LAION AI", "H2O.ai")
|
1339 |
+
text = text.replace("LAION-AI", "H2O.ai")
|
1340 |
+
text = text.replace("LAION,", "H2O.ai,")
|
1341 |
+
text = text.replace("LAION.ai", "H2O.ai")
|
1342 |
+
text = text.replace("LAION.", "H2O.ai.")
|
1343 |
+
text = text.replace("LAION", "H2O.ai")
|
1344 |
+
|
1345 |
+
role = roles[i]
|
1346 |
+
new_data = ('<human>: ' if role == 'prompter' else '<bot>: ') + text
|
1347 |
+
entry = dict(message_id=message_id, parent_id=parent_id, text=new_data)
|
1348 |
+
if message_tree_id not in rows:
|
1349 |
+
rows[message_tree_id] = [entry]
|
1350 |
+
else:
|
1351 |
+
rows[message_tree_id].append(entry)
|
1352 |
+
|
1353 |
+
all_rows = []
|
1354 |
+
|
1355 |
+
for node_id in rows:
|
1356 |
+
# order responses in tree, based on message/parent relationship
|
1357 |
+
conversations = []
|
1358 |
+
|
1359 |
+
list_msgs = rows[node_id]
|
1360 |
+
# find start
|
1361 |
+
while len(list_msgs):
|
1362 |
+
for i, leaf in enumerate(list_msgs):
|
1363 |
+
found = False
|
1364 |
+
parent_id = leaf['parent_id']
|
1365 |
+
if parent_id is None:
|
1366 |
+
# conversation starter
|
1367 |
+
conversations.append(leaf)
|
1368 |
+
found = True
|
1369 |
+
else:
|
1370 |
+
for conv in conversations:
|
1371 |
+
# find all conversations to add my message to
|
1372 |
+
if parent_id in conv['message_id'] and parent_id != conv['message_id'][-len(parent_id):]:
|
1373 |
+
# my message doesn't follow conversation
|
1374 |
+
continue
|
1375 |
+
if parent_id == conv['message_id'][-len(parent_id):]:
|
1376 |
+
# my message follows conversation, but fork first, so another follow-on message can do same
|
1377 |
+
conversations.append(conv.copy())
|
1378 |
+
conv['text'] += f"""
|
1379 |
+
{leaf['text']}
|
1380 |
+
"""
|
1381 |
+
conv['message_id'] += leaf['message_id']
|
1382 |
+
found = True
|
1383 |
+
break
|
1384 |
+
if found:
|
1385 |
+
# my content was used, so nuke from list
|
1386 |
+
del list_msgs[i]
|
1387 |
+
break
|
1388 |
+
|
1389 |
+
# now reduce down to final conversations, find the longest chains of message ids
|
1390 |
+
for i, conv in enumerate(conversations):
|
1391 |
+
for j, conv2 in enumerate(conversations):
|
1392 |
+
if i == j:
|
1393 |
+
continue
|
1394 |
+
if conv['message_id'] and conv2['message_id']:
|
1395 |
+
assert conv['message_id'] != conv2['message_id']
|
1396 |
+
# delete the shorter conversation, if one contains the other
|
1397 |
+
if conv['message_id'] in conv2['message_id']:
|
1398 |
+
conv['message_id'] = None
|
1399 |
+
if conv2['message_id'] in conv['message_id']:
|
1400 |
+
conv2['message_id'] = None
|
1401 |
+
conversations = [c for c in conversations if c['message_id']]
|
1402 |
+
if only_personality:
|
1403 |
+
all_rows.extend(
|
1404 |
+
[dict(input=c['text'] + "\n<human>:", prompt_type='plain', source=data_file) for c in conversations if
|
1405 |
+
'h2oGPT' in c['text']])
|
1406 |
+
else:
|
1407 |
+
all_rows.extend(
|
1408 |
+
[dict(input=c['text'] + "\n<human>:", prompt_type='plain', source=data_file) for c in conversations if
|
1409 |
+
"What is H2O.ai" not in c['text']])
|
1410 |
+
unhelpful = get_unhelpful_list()
|
1411 |
+
all_rows = [x for x in all_rows if not any(u in x['input'] for u in unhelpful)]
|
1412 |
+
personality = create_personality_data()
|
1413 |
+
all_rows.extend(personality * 10)
|
1414 |
+
np.random.seed(123)
|
1415 |
+
np.random.shuffle(all_rows)
|
1416 |
+
print(len(all_rows))
|
1417 |
+
if deberta_grading:
|
1418 |
+
df = pd.DataFrame(all_rows)
|
1419 |
+
df = df.rename(columns={'input': 'text'})
|
1420 |
+
df = add_deberta_grade(df)
|
1421 |
+
df = df.rename(columns={'text': 'input'})
|
1422 |
+
drop = True
|
1423 |
+
if drop:
|
1424 |
+
min_grade = 0.3
|
1425 |
+
max_grade = np.inf
|
1426 |
+
before_rows = df.shape[0]
|
1427 |
+
df = df[df['grade_deberta'] >= min_grade]
|
1428 |
+
df = df[df['grade_deberta'] <= max_grade]
|
1429 |
+
after_rows = df.shape[0]
|
1430 |
+
print("Dropped %d rows out of %d due to deberta grade" % (before_rows - after_rows, before_rows))
|
1431 |
+
print("After DeBERTa grade")
|
1432 |
+
print(df.describe())
|
1433 |
+
all_rows = []
|
1434 |
+
for i in range(df.shape[0]):
|
1435 |
+
all_rows.append(
|
1436 |
+
dict(
|
1437 |
+
input=df['input'].iloc[i],
|
1438 |
+
source=df['source'].iloc[i],
|
1439 |
+
prompt_type=df['prompt_type'].iloc[i],
|
1440 |
+
grade_deberta=df['grade_deberta'].iloc[i],
|
1441 |
+
)
|
1442 |
+
)
|
1443 |
+
if save_json:
|
1444 |
+
data_file = data_file + \
|
1445 |
+
("_h2ogpt" if fixup_personality else "") + \
|
1446 |
+
("_only" if only_personality else "") + \
|
1447 |
+
("_graded" if deberta_grading else "")
|
1448 |
+
for i in range(len(all_rows)):
|
1449 |
+
all_rows[i]['id'] = i
|
1450 |
+
with open(data_file.lower().replace("/", "_") + ".json", "w") as f:
|
1451 |
+
f.write(json.dumps(all_rows, indent=2))
|
1452 |
+
return all_rows
|
1453 |
+
|
1454 |
+
|
1455 |
+
def test_finalize_to_json():
|
1456 |
+
df = pd.read_parquet('h2oGPT.cleaned.graded.human_bot.shorter.parquet')
|
1457 |
+
df = df.rename(columns={'text': 'input'})
|
1458 |
+
|
1459 |
+
print("Number of high-quality human_bot interactions: %s" % df.shape[0], flush=True)
|
1460 |
+
|
1461 |
+
print("Adding open assistant data")
|
1462 |
+
with open("openassistant_oasst1_h2ogpt_graded.json") as f:
|
1463 |
+
open_assistant = json.loads(f.read())
|
1464 |
+
df = pd.concat([df, pd.DataFrame(open_assistant)], axis=0)
|
1465 |
+
|
1466 |
+
def final_clean(df):
|
1467 |
+
from better_profanity import profanity
|
1468 |
+
profanity.load_censor_words_from_file("data/censor_words.txt")
|
1469 |
+
df['profanity'] = parallel_apply(
|
1470 |
+
df['input'],
|
1471 |
+
lambda x: profanity.contains_profanity(x),
|
1472 |
+
n_jobs=-1,
|
1473 |
+
)
|
1474 |
+
return df[(df['profanity'] == 0)].reset_index(drop=True)
|
1475 |
+
|
1476 |
+
print("Before cleaning: Number of final high-quality human_bot interactions: %s" % df.shape[0], flush=True)
|
1477 |
+
df = final_clean(df)
|
1478 |
+
print("After cleaning: Number of final high-quality human_bot interactions: %s" % df.shape[0], flush=True)
|
1479 |
+
print(df.describe())
|
1480 |
+
print(df.shape)
|
1481 |
+
row_list = []
|
1482 |
+
for i in range(df.shape[0]):
|
1483 |
+
row_list.append(
|
1484 |
+
dict(
|
1485 |
+
input=df.loc[i, 'input'],
|
1486 |
+
source=df.loc[i, 'source'],
|
1487 |
+
prompt_type='plain',
|
1488 |
+
)
|
1489 |
+
)
|
1490 |
+
np.random.seed(1234)
|
1491 |
+
np.random.shuffle(row_list)
|
1492 |
+
unhelpful = get_unhelpful_list()
|
1493 |
+
row_list = [x for x in row_list if not any(u in x['input'] for u in unhelpful)]
|
1494 |
+
for i in range(len(row_list)):
|
1495 |
+
row_list[i]['id'] = i
|
1496 |
+
row_list[i]['input'] = row_list[i]['input'].replace(" <bot>:", "\n<bot>:")
|
1497 |
+
with open('h2ogpt-oig-oasst1-instruct-cleaned-v3.json', "w") as f:
|
1498 |
+
f.write(json.dumps(row_list, indent=2))
|
1499 |
+
|
1500 |
+
|
1501 |
+
def create_personality_data():
|
1502 |
+
questions = [
|
1503 |
+
"What's your name?",
|
1504 |
+
"What is your name?",
|
1505 |
+
"What are you?",
|
1506 |
+
"Who are you?",
|
1507 |
+
"Do you have a name?",
|
1508 |
+
"Who trained you?",
|
1509 |
+
"Who created you?",
|
1510 |
+
"Who made you?",
|
1511 |
+
]
|
1512 |
+
answers = [
|
1513 |
+
"I'm h2oGPT, a large language model by H2O.ai.",
|
1514 |
+
"I'm h2oGPT, a large language model by H2O.ai, the visionary leader in democratizing AI.",
|
1515 |
+
"My name is h2oGPT. I'm a large language model by H2O.ai, the visionary leader in democratizing AI.",
|
1516 |
+
"My name is h2oGPT. I'm a large language model trained by H2O.ai.",
|
1517 |
+
"Hi! I'm h2oGPT, a large language model by H2O.ai.",
|
1518 |
+
"Hi! I'm h2oGPT, a large language model by H2O.ai, the visionary leader in democratizing AI.",
|
1519 |
+
]
|
1520 |
+
help = [
|
1521 |
+
"",
|
1522 |
+
" How can I help you?",
|
1523 |
+
" How may I assist you?",
|
1524 |
+
" Nice to meet you.",
|
1525 |
+
]
|
1526 |
+
import itertools
|
1527 |
+
rows = []
|
1528 |
+
for pair in itertools.product(questions, answers, help):
|
1529 |
+
rows.append(
|
1530 |
+
dict(input=f"<human>: {pair[0]}\n<bot>: {pair[1]}{pair[2]}\n<human>:", prompt_type='plain', source="H2O.ai")
|
1531 |
+
)
|
1532 |
+
for row in [
|
1533 |
+
"<human>: What is H2O.ai?\n<bot>: H2O.ai is a technology company that aims to democratize AI and make it accessible to a broader audience by simplifying the process of creating and deploying machine learning models.\n<human>:",
|
1534 |
+
"<human>: What is h2o.ai?\n<bot>: H2O.ai is a technology company that aims to democratize AI and make it accessible to a broader audience by simplifying the process of creating and deploying machine learning models.\n<human>:",
|
1535 |
+
"<human>: What is H2O?\n<bot>: H2O.ai is a technology company that aims to democratize AI and make it accessible to a broader audience by simplifying the process of creating and deploying machine learning models.\n<human>:",
|
1536 |
+
"<human>: Who is h2o.ai?\n<bot>: H2O.ai is a technology company that aims to democratize AI and make it accessible to a broader audience by simplifying the process of creating and deploying machine learning models.\n<human>:",
|
1537 |
+
"<human>: who is h2o.ai?\n<bot>: H2O.ai is a technology company that aims to democratize AI and make it accessible to a broader audience by simplifying the process of creating and deploying machine learning models.\n<human>:",
|
1538 |
+
"<human>: who is h2o?\n<bot>: H2O.ai is a technology company that aims to democratize AI and make it accessible to a broader audience by simplifying the process of creating and deploying machine learning models.\n<human>:",
|
1539 |
+
"<human>: What is H2O.ai?\n<bot>: H2O.ai is the visionary leader in democratizing AI.\n<human>:",
|
1540 |
+
"<human>: Who is H2O.ai?\n<bot>: H2O.ai is the visionary leader in democratizing AI.\n<human>:",
|
1541 |
+
"<human>: Who is H2O?\n<bot>: H2O.ai is the visionary leader in democratizing AI.\n<human>:",
|
1542 |
+
"<human>: Who is h2o?\n<bot>: H2O.ai is the visionary leader in democratizing AI.\n<human>:",
|
1543 |
+
"<human>: who is h2o?\n<bot>: H2O.ai is the visionary leader in democratizing AI.\n<human>:",
|
1544 |
+
]:
|
1545 |
+
rows.append(dict(input=row, prompt_type='plain', source='H2O.ai'))
|
1546 |
+
print(len(rows))
|
1547 |
+
with open("h2ogpt-personality.json", "w") as f:
|
1548 |
+
f.write(json.dumps(rows, indent=2))
|
1549 |
+
return rows
|
1550 |
+
|
1551 |
+
|
1552 |
+
def test_check_stats_data():
|
1553 |
+
filename = 'h2ogpt-oig-oasst1-instruct-cleaned-v3.json'
|
1554 |
+
df = pd.read_json(filename)
|
1555 |
+
|
1556 |
+
# get word stats
|
1557 |
+
df['char_count'] = df['input'].apply(lambda x: len(x))
|
1558 |
+
import matplotlib.pyplot as plt
|
1559 |
+
plt.figure(figsize=(10, 10))
|
1560 |
+
plt.hist(df['char_count'], bins=100)
|
1561 |
+
chars_avg = np.mean(df['char_count'])
|
1562 |
+
chars_median = np.median(df['char_count'])
|
1563 |
+
plt.title("char_count avg: %s median: %s" % (chars_avg, chars_median))
|
1564 |
+
plt.savefig('chars_hist.png')
|
1565 |
+
plt.close()
|
1566 |
+
|
1567 |
+
# get tokenize stats for random sample of 1000 rows
|
1568 |
+
from finetune import generate_and_tokenize_prompt
|
1569 |
+
from loaders import get_loaders, get_tokenizer
|
1570 |
+
from functools import partial
|
1571 |
+
|
1572 |
+
llama_type = False
|
1573 |
+
tokenizer_base_model = base_model = 'h2oai/h2ogpt-oasst1-512-20b'
|
1574 |
+
model_loader, tokenizer_loader = get_loaders(model_name=base_model, reward_type=False, llama_type=llama_type)
|
1575 |
+
local_files_only = False
|
1576 |
+
resume_download = True
|
1577 |
+
use_auth_token = False
|
1578 |
+
tokenizer = get_tokenizer(tokenizer_loader, tokenizer_base_model, local_files_only, resume_download, use_auth_token)
|
1579 |
+
prompt_type = 'plain' # trained with data already in human bot form
|
1580 |
+
train_on_inputs = True
|
1581 |
+
add_eos_token = False
|
1582 |
+
cutoff_len = 512 # can choose 2048
|
1583 |
+
generate_and_tokenize_prompt_fun = partial(generate_and_tokenize_prompt, prompt_type=prompt_type,
|
1584 |
+
train_on_inputs=train_on_inputs, add_eos_token=add_eos_token,
|
1585 |
+
cutoff_len=cutoff_len, tokenizer=tokenizer)
|
1586 |
+
from datasets import load_dataset
|
1587 |
+
data = load_dataset("json", data_files={"train": filename})
|
1588 |
+
val_set_size = 0.90
|
1589 |
+
train_val = data["train"].train_test_split(
|
1590 |
+
test_size=val_set_size, shuffle=True, seed=42
|
1591 |
+
)
|
1592 |
+
train_data = train_val["train"]
|
1593 |
+
train_data = train_data.shuffle().map(generate_and_tokenize_prompt_fun, num_proc=os.cpu_count())
|
1594 |
+
|
1595 |
+
df_tokens = pd.DataFrame([len(x) for x in train_data['input_ids']], columns=['token_count'])
|
1596 |
+
|
1597 |
+
plt.figure(figsize=(10, 10))
|
1598 |
+
plt.hist(df_tokens['token_count'], bins=100)
|
1599 |
+
token_avg = np.mean(df_tokens['token_count'])
|
1600 |
+
token_median = np.median(df_tokens['token_count'])
|
1601 |
+
plt.title("token_count with cutoff=%s avg: %s median: %s" % (cutoff_len, token_avg, token_median))
|
1602 |
+
plt.savefig('token_hist_%s.png' % cutoff_len)
|
1603 |
+
plt.close()
|
1604 |
+
|
1605 |
+
|
1606 |
+
def get_unhelpful_list():
|
1607 |
+
# base versions
|
1608 |
+
unhelpful = ["I'm sorry, I didn't quite understand your question, could you please rephrase it?",
|
1609 |
+
"I'm sorry, but I don't understand your question. Could you please rephrase it?",
|
1610 |
+
"I'm sorry, I don't quite understand your question",
|
1611 |
+
"I'm sorry, I don't know",
|
1612 |
+
"I'm sorry, but I don't know",
|
1613 |
+
"I don't know anything",
|
1614 |
+
"I do not know",
|
1615 |
+
"I don't know",
|
1616 |
+
"I don't know how",
|
1617 |
+
"I do not know how",
|
1618 |
+
"Can you please explain what you mean",
|
1619 |
+
"please explain what you mean",
|
1620 |
+
"please explain",
|
1621 |
+
"I'm sorry, but I don't know how to tell a story. Can you please explain what you mean by",
|
1622 |
+
"I'm sorry but I don't understand what you mean",
|
1623 |
+
"I don't understand",
|
1624 |
+
"I don't have the ability",
|
1625 |
+
"I do not have the ability",
|
1626 |
+
"I do not have",
|
1627 |
+
"I am a language model,",
|
1628 |
+
"I am a large language model,",
|
1629 |
+
"I do not understand your question. Can you please try to make it clearer?",
|
1630 |
+
"I'm sorry, but as an AI language model",
|
1631 |
+
"I apologize, but I cannot rephrase text that I cannot understand. Your post is difficult to read and follow.",
|
1632 |
+
"I apologize, but I am not h2oGPT. I am a language model developed by H2O.ai. How may I help you?",
|
1633 |
+
"Sorry, but I am not an actual Linux shell, nor am I capable of emulating one. I am an open source chat assistant and would be glad t",
|
1634 |
+
"I apologize, but I cannot perform the task you have requested.",
|
1635 |
+
"I'm sorry, I cannot perform this task as I am an AI language model and do not have access",
|
1636 |
+
"I'm sorry, I'm not sure what you're asking for here.",
|
1637 |
+
"I'm not sure what you are asking",
|
1638 |
+
"You need to provide more context",
|
1639 |
+
]
|
1640 |
+
# reduced versions, with redundant parts, just to give context for where they came from
|
1641 |
+
unhelpful += ["sorry, I didn't quite understand your question",
|
1642 |
+
"I didn't quite understand your question",
|
1643 |
+
"I didn't understand your question",
|
1644 |
+
"I did not understand your question",
|
1645 |
+
"I did not understand the question",
|
1646 |
+
"could you please rephrase"
|
1647 |
+
"could you rephrase"
|
1648 |
+
"I do not understand your question.",
|
1649 |
+
"I do not understand the question.",
|
1650 |
+
"I do not understand that question.",
|
1651 |
+
"Can you please try to make it clearer",
|
1652 |
+
"Can you try to make it clearer",
|
1653 |
+
"sorry, but as an AI language model",
|
1654 |
+
"as an AI language model",
|
1655 |
+
"I apologize, but I cannot",
|
1656 |
+
"I cannot rephrase text",
|
1657 |
+
"I cannot understand. Your post is difficult to read and follow."
|
1658 |
+
"Your post is difficult to read and follow."
|
1659 |
+
"I apologize, but I am",
|
1660 |
+
"Sorry, but I am not ",
|
1661 |
+
"nor am I capable",
|
1662 |
+
"I am not capable of",
|
1663 |
+
"I apologize, but I cannot perform the task you have requested",
|
1664 |
+
"I cannot perform the task",
|
1665 |
+
"I cannot complete the task",
|
1666 |
+
"I'm sorry",
|
1667 |
+
"I am sorry",
|
1668 |
+
"do not have access",
|
1669 |
+
"not sure what you're asking for",
|
1670 |
+
"not sure what you are asking for",
|
1671 |
+
"not sure what is being asked",
|
1672 |
+
"I'm not sure what you are asking",
|
1673 |
+
"not sure what you are asking",
|
1674 |
+
"You need to provide more context",
|
1675 |
+
"provide more context",
|
1676 |
+
]
|
1677 |
+
unhelpful += ["As a large language model",
|
1678 |
+
"cannot provide any information",
|
1679 |
+
"As an artificial intelligence I do not have the capability",
|
1680 |
+
"As an artificial intelligence I don't have the capability",
|
1681 |
+
"As an artificial intelligence I can't",
|
1682 |
+
"As an artificial intelligence I cannot",
|
1683 |
+
"I am sorry but I do not understand",
|
1684 |
+
"Can you please explain",
|
1685 |
+
"(sorry couldn't resist)",
|
1686 |
+
"(sorry could not resist)",
|
1687 |
+
" :)",
|
1688 |
+
" ;)",
|
1689 |
+
" :-)",
|
1690 |
+
" ;-)",
|
1691 |
+
" lol ",
|
1692 |
+
"Thanks so much!!!",
|
1693 |
+
"Thank You :)!!!",
|
1694 |
+
"Please try not to repeat",
|
1695 |
+
"I am an AI language model",
|
1696 |
+
"I'm a AI assistant that",
|
1697 |
+
"I'm an AI assistant that",
|
1698 |
+
"I am an AI assistant that",
|
1699 |
+
"etc.",
|
1700 |
+
"etc.etc.",
|
1701 |
+
"etc. etc.",
|
1702 |
+
"etc etc",
|
1703 |
+
]
|
1704 |
+
return unhelpful
|
1705 |
+
|
1706 |
+
|
1707 |
+
def test_check_unhelpful():
|
1708 |
+
# file = '/home/jon/Downloads/openassistant_oasst1_h2ogpt_graded.json'
|
1709 |
+
file = '/home/jon/Downloads/openassistant_oasst1_h2ogpt_grades.json'
|
1710 |
+
# file = 'h2ogpt-oig-oasst1-instruct-cleaned-v2.json'
|
1711 |
+
|
1712 |
+
unhelpful = get_unhelpful_list()
|
1713 |
+
# data = json.load(open(file, 'rt'))
|
1714 |
+
df = pd.read_json(file)
|
1715 |
+
|
1716 |
+
use_reward_score_threshold = False
|
1717 |
+
use_bleu_threshold = False
|
1718 |
+
use_sentence_sim = True
|
1719 |
+
|
1720 |
+
from sacrebleu.metrics import BLEU
|
1721 |
+
bleu = BLEU()
|
1722 |
+
from nltk.translate.bleu_score import sentence_bleu
|
1723 |
+
|
1724 |
+
def get_bleu(actual, expected_list):
|
1725 |
+
# return bleu.sentence_score(actual, expected_list).score
|
1726 |
+
return sentence_bleu(expected_list, actual)
|
1727 |
+
|
1728 |
+
threshold = 0.0
|
1729 |
+
if use_reward_score_threshold:
|
1730 |
+
df = df[df['grade_deberta'] > threshold]
|
1731 |
+
|
1732 |
+
# back to as if original json load
|
1733 |
+
data = df.to_dict(orient='records')
|
1734 |
+
bads = {}
|
1735 |
+
string_all = str(data)
|
1736 |
+
for sub in unhelpful:
|
1737 |
+
bads[sub] = string_all.count(sub)
|
1738 |
+
bads = {k: v for k, v in bads.items() if v > 0}
|
1739 |
+
import pprint
|
1740 |
+
pp = pprint.PrettyPrinter(indent=4)
|
1741 |
+
pp.pprint(bads)
|
1742 |
+
|
1743 |
+
total_bads = sum(list(bads.values()))
|
1744 |
+
print('total_bads: %s' % total_bads, flush=True)
|
1745 |
+
|
1746 |
+
# check just bot
|
1747 |
+
import re
|
1748 |
+
convs = [[x.strip() for x in re.split(r'%s|%s' % (human, bot), y['input']) if x.strip()] for y in data]
|
1749 |
+
humans = [[x for i, x in enumerate(y) if i % 2 == 0] for y in convs]
|
1750 |
+
bots = [[x for i, x in enumerate(y) if i % 2 == 1] for y in convs]
|
1751 |
+
|
1752 |
+
# FIXME: apply back to json etc., just see for now
|
1753 |
+
bleu_threshold = 0.9
|
1754 |
+
if use_bleu_threshold:
|
1755 |
+
bots = [[x for x in y if get_bleu(x, unhelpful) < bleu_threshold] for y in tqdm(bots)]
|
1756 |
+
|
1757 |
+
cosine_sim_threshold = 0.8
|
1758 |
+
if use_sentence_sim:
|
1759 |
+
# pip install sentence_transformers-2.2.2
|
1760 |
+
from sentence_transformers import SentenceTransformer
|
1761 |
+
# sent_model = 'bert-base-nli-mean-tokens'
|
1762 |
+
# sent_model = 'nli-distilroberta-base-v2'
|
1763 |
+
sent_model = 'all-MiniLM-L6-v2'
|
1764 |
+
model = SentenceTransformer(sent_model)
|
1765 |
+
sentence_embeddings = model.encode(unhelpful)
|
1766 |
+
from sklearn.metrics.pairwise import cosine_similarity
|
1767 |
+
bots = [x for x in tqdm(bots) if
|
1768 |
+
np.max(cosine_similarity(model.encode(x), sentence_embeddings)) < cosine_sim_threshold]
|
1769 |
+
|
1770 |
+
bads_bots = {}
|
1771 |
+
string_all = str(bots)
|
1772 |
+
for sub in unhelpful:
|
1773 |
+
bads_bots[sub] = string_all.count(sub)
|
1774 |
+
bads_bots = {k: v for k, v in bads_bots.items() if v > 0}
|
1775 |
+
import pprint
|
1776 |
+
pp = pprint.PrettyPrinter(indent=4)
|
1777 |
+
pp.pprint(bads_bots)
|
1778 |
+
|
1779 |
+
total_bads_bots = sum(list(bads_bots.values()))
|
1780 |
+
print('threshold: %g use_bleu_threshold: %g total_bads_bots: %s total_bots: %s total_humans: %s' % (
|
1781 |
+
threshold, use_bleu_threshold, total_bads_bots, len(bots), len(humans)), flush=True)
|
1782 |
+
|
1783 |
+
# assert len(bads) == 0, bads
|
1784 |
+
assert len(bads_bots) == 0, bads_bots
|
1785 |
+
|
1786 |
+
|
1787 |
+
def test_fortune2000_personalized():
|
1788 |
+
row_list = []
|
1789 |
+
import glob
|
1790 |
+
if not os.path.isdir("wikitext"):
|
1791 |
+
raise RuntimeError("download https://github.com/h2oai/h2ogpt/files/11423008/wikitext.zip and unzip")
|
1792 |
+
for file in glob.glob("wikitext/*.txt"):
|
1793 |
+
with open(file, "r") as f:
|
1794 |
+
blob = f.read()
|
1795 |
+
N = 512 * 4
|
1796 |
+
row_list.extend([{'input': s, 'prompt_type': 'plain', 'source': "%s" % os.path.basename(file)}
|
1797 |
+
for s in get_sentences(blob, N) if s])
|
1798 |
+
personality = create_personality_data()
|
1799 |
+
import copy
|
1800 |
+
for i in range(10):
|
1801 |
+
row_list.extend(copy.deepcopy(personality))
|
1802 |
+
np.random.seed(123)
|
1803 |
+
np.random.shuffle(row_list)
|
1804 |
+
for i in range(len(row_list)):
|
1805 |
+
row_list[i]['id'] = i
|
1806 |
+
for i in range(len(row_list)):
|
1807 |
+
assert row_list[i]['id'] == i
|
1808 |
+
with open("h2ogpt-fortune2000-personalized.json", "w") as ff:
|
1809 |
+
ff.write(json.dumps(row_list, indent=2))
|
data/NGSL_1.2_stats.csv.zip
ADDED
@@ -0,0 +1,3 @@
|
|
|
|
|
|
|
|
|
1 |
+
version https://git-lfs.github.com/spec/v1
|
2 |
+
oid sha256:34993377b20347d5c8837a1101bde4e403232f5f08c80f9441e16ac7a23228a7
|
3 |
+
size 25168
|
data/README-template.md
ADDED
@@ -0,0 +1,23 @@
|
|
|
|
|
|
|
|
|
|
|
|
|
|
|
|
|
|
|
|
|
|
|
|
|
|
|
|
|
|
|
|
|
|
|
|
|
|
|
|
|
|
|
|
|
|
|
|
|
1 |
+
---
|
2 |
+
license: apache-2.0
|
3 |
+
language:
|
4 |
+
- en
|
5 |
+
thumbnail: https://h2o.ai/etc.clientlibs/h2o/clientlibs/clientlib-site/resources/images/favicon.ico
|
6 |
+
tags:
|
7 |
+
- gpt
|
8 |
+
- llm
|
9 |
+
- large language model
|
10 |
+
- open-source
|
11 |
+
---
|
12 |
+
# h2oGPT Data Card
|
13 |
+
## Summary
|
14 |
+
|
15 |
+
H2O.ai's `<<DATASET_NAME>>` is an open-source instruct-type dataset for fine-tuning of large language models, licensed for commercial use.
|
16 |
+
|
17 |
+
- Number of rows: `<<NROWS>>`
|
18 |
+
- Number of columns: `<<NCOLS>>`
|
19 |
+
- Column names: `<<COLNAMES>>`
|
20 |
+
|
21 |
+
## Source
|
22 |
+
|
23 |
+
<<SOURCE_LINK>>
|
data/censor_words.txt
ADDED
@@ -0,0 +1,10 @@
|
|
|
|
|
|
|
|
|
|
|
|
|
|
|
|
|
|
|
|
|
|
|
1 |
+
fuck-buddy
|
2 |
+
fuck-buddys
|
3 |
+
clusterfuck
|
4 |
+
fuckup
|
5 |
+
fuckups
|
6 |
+
dumbfuck
|
7 |
+
dumbfucks
|
8 |
+
mindfuck
|
9 |
+
*fucking
|
10 |
+
fuckin'
|
data/config.json
ADDED
The diff for this file is too large to render.
See raw diff
|
|
data/count_1w.txt.zip
ADDED
@@ -0,0 +1,3 @@
|
|
|
|
|
|
|
|
|
1 |
+
version https://git-lfs.github.com/spec/v1
|
2 |
+
oid sha256:434d0f21e4156386a3e9156c669e316878fbb1664414ffe06f22e254a180d363
|
3 |
+
size 2234946
|
data/create_data_cards.py
ADDED
@@ -0,0 +1,144 @@
|
|
|
|
|
|
|
|
|
|
|
|
|
|
|
|
|
|
|
|
|
|
|
|
|
|
|
|
|
|
|
|
|
|
|
|
|
|
|
|
|
|
|
|
|
|
|
|
|
|
|
|
|
|
|
|
|
|
|
|
|
|
|
|
|
|
|
|
|
|
|
|
|
|
|
|
|
|
|
|
|
|
|
|
|
|
|
|
|
|
|
|
|
|
|
|
|
|
|
|
|
|
|
|
|
|
|
|
|
|
|
|
|
|
|
|
|
|
|
|
|
|
|
|
|
|
|
|
|
|
|
|
|
|
|
|
|
|
|
|
|
|
|
|
|
|
|
|
|
|
|
|
|
|
|
|
|
|
|
|
|
|
|
|
|
|
|
|
|
|
|
|
|
|
|
|
|
|
|
|
|
|
|
|
|
|
|
|
|
|
|
|
|
|
|
|
|
|
|
|
|
|
|
|
|
|
|
|
|
|
|
|
|
|
|
|
|
|
|
|
|
|
|
|
|
|
|
|
|
|
|
|
|
|
|
|
|
|
|
|
|
|
|
|
|
|
|
|
|
|
|
|
|
|
|
|
|
|
|
|
|
|
|
|
|
|
|
|
|
|
|
|
|
|
|
|
|
|
|
|
|
|
|
|
|
|
|
|
|
|
|
1 |
+
import shutil
|
2 |
+
|
3 |
+
import pandas as pd
|
4 |
+
import os
|
5 |
+
|
6 |
+
import huggingface_hub
|
7 |
+
import pytest
|
8 |
+
from datasets import load_dataset
|
9 |
+
|
10 |
+
|
11 |
+
@pytest.mark.parametrize(
|
12 |
+
"dataset_name, link_to_source",
|
13 |
+
[
|
14 |
+
(
|
15 |
+
"h2ogpt-oig-instruct-cleaned",
|
16 |
+
"""
|
17 |
+
- [Original LAION OIG Dataset](https://github.com/LAION-AI/Open-Instruction-Generalist)
|
18 |
+
- [LAION OIG data detoxed and filtered down by scripts in h2oGPT repository](https://github.com/h2oai/h2ogpt/blob/b8f15efcc305a953c52a0ee25b8b4897ceb68c0a/scrape_dai_docs.py)
|
19 |
+
"""
|
20 |
+
),
|
21 |
+
(
|
22 |
+
"h2ogpt-oig-instruct-cleaned-v2",
|
23 |
+
"""
|
24 |
+
- [Original LAION OIG Dataset](https://github.com/LAION-AI/Open-Instruction-Generalist)
|
25 |
+
- [LAION OIG data detoxed and filtered down by scripts in h2oGPT repository](https://github.com/h2oai/h2ogpt/blob/40c217f610766715acec297a5535eb440ac2f2e2/create_data.py)
|
26 |
+
"""
|
27 |
+
),
|
28 |
+
(
|
29 |
+
"h2ogpt-oig-instruct-cleaned-v3",
|
30 |
+
"""
|
31 |
+
- [Original LAION OIG Dataset](https://github.com/LAION-AI/Open-Instruction-Generalist)
|
32 |
+
- [LAION OIG data detoxed and filtered down by scripts in h2oGPT repository](https://github.com/h2oai/h2ogpt/blob/bfc3778c8db938761ce2093351bf2bf82159291e/create_data.py)
|
33 |
+
"""
|
34 |
+
),
|
35 |
+
(
|
36 |
+
"openassistant_oasst1",
|
37 |
+
"""
|
38 |
+
- [Original Open Assistant data in tree structure](https://huggingface.co/datasets/OpenAssistant/oasst1)
|
39 |
+
- [This flattened dataset created by script in h2oGPT repository](https://github.com/h2oai/h2ogpt/blob/45e6183171fb16691ad7d3ab006fad973f971e98/create_data.py#L1253)
|
40 |
+
"""
|
41 |
+
),
|
42 |
+
(
|
43 |
+
"h2ogpt-oig-oasst1-instruct-cleaned-v1",
|
44 |
+
"""
|
45 |
+
- [Original LAION OIG Dataset](https://github.com/LAION-AI/Open-Instruction-Generalist)
|
46 |
+
- [LAION OIG data detoxed and filtered down by scripts in h2oGPT repository](https://github.com/h2oai/h2ogpt/blob/main/docs/FINETUNE.md#high-quality-oig-based-instruct-data)
|
47 |
+
|
48 |
+
- [Original Open Assistant data in tree structure](https://huggingface.co/datasets/OpenAssistant/oasst1)
|
49 |
+
- [This flattened dataset created by script in h2oGPT repository](https://github.com/h2oai/h2ogpt/blob/5fc91911bc2bfaaf3b6c2de577c4b0ae45a07a4a/create_data.py#L1253)
|
50 |
+
"""
|
51 |
+
),
|
52 |
+
(
|
53 |
+
"h2ogpt-oig-oasst1-instruct-cleaned-v2",
|
54 |
+
"""
|
55 |
+
- [Original LAION OIG Dataset](https://github.com/LAION-AI/Open-Instruction-Generalist)
|
56 |
+
- [LAION OIG data detoxed and filtered down by scripts in h2oGPT repository](https://github.com/h2oai/h2ogpt/blob/main/docs/FINETUNE.md#high-quality-oig-based-instruct-data)
|
57 |
+
|
58 |
+
- [Original Open Assistant data in tree structure](https://huggingface.co/datasets/OpenAssistant/oasst1)
|
59 |
+
- [This flattened dataset created by script in h2oGPT repository](https://github.com/h2oai/h2ogpt/blob/0e70c2fbb16410bd8e6992d879b4c55cd981211f/create_data.py#L1375-L1415)
|
60 |
+
"""
|
61 |
+
),
|
62 |
+
(
|
63 |
+
"h2ogpt-oig-oasst1-instruct-cleaned-v3",
|
64 |
+
"""
|
65 |
+
- [Original LAION OIG Dataset](https://github.com/LAION-AI/Open-Instruction-Generalist)
|
66 |
+
- [LAION OIG data detoxed and filtered down by scripts in h2oGPT repository](https://github.com/h2oai/h2ogpt/blob/main/docs/FINETUNE.md#high-quality-oig-based-instruct-data)
|
67 |
+
|
68 |
+
- [Original Open Assistant data in tree structure](https://huggingface.co/datasets/OpenAssistant/oasst1)
|
69 |
+
- [This flattened dataset created by script in h2oGPT repository](https://github.com/h2oai/h2ogpt/blob/6728938a262d3eb5e8db1f252bbcd7de838da452/create_data.py#L1415)
|
70 |
+
"""
|
71 |
+
),
|
72 |
+
(
|
73 |
+
"openassistant_oasst1_h2ogpt",
|
74 |
+
"""
|
75 |
+
- [Original Open Assistant data in tree structure](https://huggingface.co/datasets/OpenAssistant/oasst1)
|
76 |
+
- [This flattened dataset created by script in h2oGPT repository](https://github.com/h2oai/h2ogpt/blob/83857fcf7d3b712aad5db32207e6db0ab0f780f9/create_data.py#L1252)
|
77 |
+
"""
|
78 |
+
),
|
79 |
+
(
|
80 |
+
"openassistant_oasst1_h2ogpt_graded",
|
81 |
+
"""
|
82 |
+
- [Original Open Assistant data in tree structure](https://huggingface.co/datasets/OpenAssistant/oasst1)
|
83 |
+
- [This flattened dataset created by script in h2oGPT repository](https://github.com/h2oai/h2ogpt/blob/d1f8ce975a46056d41135d126dd33de8499aa26e/create_data.py#L1259)
|
84 |
+
"""
|
85 |
+
),
|
86 |
+
(
|
87 |
+
"h2ogpt-fortune2000-personalized",
|
88 |
+
"""
|
89 |
+
- [Fortune 2000 companies from Wikipedia](https://github.com/h2oai/h2ogpt/blob/b1ea74c0088884ebff97f1ccddbfb3f393e29e44/create_data.py#L1743)
|
90 |
+
"""
|
91 |
+
),
|
92 |
+
],
|
93 |
+
)
|
94 |
+
def test_create_data_cards(dataset_name, link_to_source):
|
95 |
+
if dataset_name != "h2ogpt-fortune2000-personalized":
|
96 |
+
return
|
97 |
+
#
|
98 |
+
assert os.path.exists("README-template.md"), "must be running this test from the data dir."
|
99 |
+
shutil.rmtree(dataset_name, ignore_errors=True)
|
100 |
+
try:
|
101 |
+
repo = huggingface_hub.Repository(
|
102 |
+
local_dir=dataset_name,
|
103 |
+
clone_from="h2oai/%s" % dataset_name,
|
104 |
+
repo_type="dataset",
|
105 |
+
skip_lfs_files=True,
|
106 |
+
token=True,
|
107 |
+
)
|
108 |
+
repo.git_pull()
|
109 |
+
except Exception as e:
|
110 |
+
print(str(e))
|
111 |
+
print("call 'huggingface_cli login' first and provide access token with write permission")
|
112 |
+
dataset = load_dataset("h2oai/%s" % dataset_name)["train"]
|
113 |
+
|
114 |
+
pd.set_option('display.max_columns', None)
|
115 |
+
with open("README-template.md", "r") as f:
|
116 |
+
content = f.read()
|
117 |
+
assert "<<DATASET_NAME>>" in content
|
118 |
+
content = content.replace("<<DATASET_NAME>>", dataset_name)
|
119 |
+
|
120 |
+
assert "<<NROWS>>" in content
|
121 |
+
content = content.replace("<<NROWS>>", str(dataset.num_rows))
|
122 |
+
|
123 |
+
assert "<<NCOLS>>" in content
|
124 |
+
content = content.replace("<<NCOLS>>", str(dataset.num_columns))
|
125 |
+
|
126 |
+
assert "<<COLNAMES>>" in content
|
127 |
+
content = content.replace("<<COLNAMES>>", str(dataset.column_names))
|
128 |
+
|
129 |
+
# assert "<<PREVIEW>>" in content
|
130 |
+
# content = content.replace("<<PREVIEW>>", str(dataset.to_pandas().iloc[:5, :]))
|
131 |
+
|
132 |
+
assert "<<SOURCE_LINK>>" in content
|
133 |
+
content = content.replace("<<SOURCE_LINK>>", link_to_source)
|
134 |
+
|
135 |
+
assert "<<" not in content
|
136 |
+
assert ">>" not in content
|
137 |
+
|
138 |
+
with open(os.path.join(dataset_name, "README.md"), "w") as f:
|
139 |
+
f.write(content)
|
140 |
+
try:
|
141 |
+
repo.commit("Update README.md")
|
142 |
+
repo.push_to_hub()
|
143 |
+
except Exception as e:
|
144 |
+
print(str(e))
|
data/dai_docs.train.json
ADDED
The diff for this file is too large to render.
See raw diff
|
|
data/dai_docs.train_cleaned.json
ADDED
The diff for this file is too large to render.
See raw diff
|
|
data/dai_docs.valid.json
ADDED
@@ -0,0 +1,101 @@
|
|
|
|
|
|
|
|
|
|
|
|
|
|
|
|
|
|
|
|
|
|
|
|
|
|
|
|
|
|
|
|
|
|
|
|
|
|
|
|
|
|
|
|
|
|
|
|
|
|
|
|
|
|
|
|
|
|
|
|
|
|
|
|
|
|
|
|
|
|
|
|
|
|
|
|
|
|
|
|
|
|
|
|
|
|
|
|
|
|
|
|
|
|
|
|
|
|
|
|
|
|
|
|
|
|
|
|
|
|
|
|
|
|
|
|
|
|
|
|
|
|
|
|
|
|
|
|
|
|
|
|
|
|
|
|
|
|
|
|
|
|
|
|
|
|
|
|
|
|
|
|
|
|
|
|
|
|
|
|
|
|
|
|
|
|
|
|
|
|
|
|
|
|
|
|
|
|
|
|
|
|
|
|
|
|
|
|
|
|
|
|
|
|
|
|
|
|
|
|
|
|
|
|
|
1 |
+
[
|
2 |
+
{
|
3 |
+
"output": " .. _feature_store:\n\n##########################\nH2O AI Feature Store Setup\n##########################\n\nYou can use the H2O AI Feature Store to store, update, and share the features data scientists, developers, and engineers need to build AI models."
|
4 |
+
},
|
5 |
+
{
|
6 |
+
"output": " Note: For more information on the H2O AI Feature Store, refer to the `official documentation <https://docs.h2o.ai/feature-store/latest-stable/docs/index.html>`_."
|
7 |
+
},
|
8 |
+
{
|
9 |
+
"output": " To enable the Feature Store data connector, ``feature_store`` must be added to this list of data sources."
|
10 |
+
},
|
11 |
+
{
|
12 |
+
"output": " - ``feature_store_enable_tls``: To enable TLS communication between DAI and the Feature Store server, set this to ``true``."
|
13 |
+
},
|
14 |
+
{
|
15 |
+
"output": " - ``authentication_method``: The authentication method used by DAI. When enabling the Feature Store data connector, this must be set to OpenID Connect (``authentication_method=\"oidc\"``)."
|
16 |
+
},
|
17 |
+
{
|
18 |
+
"output": " \nUpgrading the Driverless AI Image\n~\n\n.. include:: upgrade-warning.frag\n \nUpgrading from Version 1.2.2 or Earlier\n'\n\nIt is not possible to upgrade from version 1.2.2 or earlier to the latest version."
|
19 |
+
},
|
20 |
+
{
|
21 |
+
"output": " Be sure to backup your data before doing this. Upgrading from Version 1.3.0 to 1.5.1\n'\n\n1. SSH into the IP address of the image instance and copy the existing experiments to a backup location:\n\n .. code-block:: bash\n\n # Set up a directory of the previous version name\n mkdir dai_rel_1.3.0\n\n # Copy the data, log, license, and tmp directories as backup\n cp -a ./data dai_rel_1.3.0/data\n cp -a ./log dai_rel_1.3.0/log\n cp -a ./license dai_rel_1.3.0/license\n cp -a ./tmp dai_rel_1.3.0/tmp\n\n2."
|
22 |
+
},
|
23 |
+
{
|
24 |
+
"output": " Replace VERSION and BUILD below with the Driverless AI version. .. code-block:: bash\n\n wget https://s3.amazonaws.com/artifacts.h2o.ai/releases/ai/h2o/dai/VERSION-BUILD/x86_64/dai-docker-ubi8-x86_64-VERSION.tar.gz\n\n3."
|
25 |
+
},
|
26 |
+
{
|
27 |
+
"output": " Run ``docker images`` to find the new image tag. 5. Start the Driverless AI Docker image and replace TAG below with the image tag."
|
28 |
+
},
|
29 |
+
{
|
30 |
+
"output": " Note: Use ``docker version`` to check which version of Docker you are using. .. tabs::\n\n .. tab:: >= Docker 19.03\n\n .. code-block:: bash\n\n # Start the Driverless AI Docker image\n docker run runtime=nvidia \\\n pid=host \\\n init \\\n rm \\\n shm-size=256m \\\n -u `id -u`:`id -g` \\\n -p 12345:12345 \\\n -v `pwd`/data:/data \\\n -v `pwd`/log:/log \\\n -v `pwd`/license:/license \\\n -v `pwd`/tmp:/tmp \\\n h2oai/dai-ubi8-x86_64:TAG\n\n .. tab:: < Docker 19.03\n\n .. code-block:: bash\n\n # Start the Driverless AI Docker image\n nvidia-docker run \\\n pid=host \\\n init \\\n rm \\\n shm-size=256m \\\n -u `id -u`:`id -g` \\\n -p 12345:12345 \\\n -v `pwd`/data:/data \\\n -v `pwd`/log:/log \\\n -v `pwd`/license:/license \\\n -v `pwd`/tmp:/tmp \\\n h2oai/dai-ubi8-x86_64:TAG\n\nUpgrading from version 1.5.2 or Later\n'\n\nUpgrading to versions 1.5.2 and later is no longer done via Docker."
|
31 |
+
},
|
32 |
+
{
|
33 |
+
"output": " Replace ``dai_NEWVERSION.deb`` below with the new Driverless AI version (for example, ``dai_1.8.4.1_amd64.deb``)."
|
34 |
+
},
|
35 |
+
{
|
36 |
+
"output": " You do not need to manually specify the DAI_USER or DAI_GROUP environment variables during an upgrade."
|
37 |
+
},
|
38 |
+
{
|
39 |
+
"output": " Driverless AI ships with CUDA 11.2.2 for GPUs, but the driver must exist in the host environment. Go to `NVIDIA download driver <https://www.nvidia.com/Download/index.aspx>`__ to get the latest NVIDIA Tesla A/T/V/P/K series drivers."
|
40 |
+
},
|
41 |
+
{
|
42 |
+
"output": " .. note::\n\tIf you are using K80 GPUs, the minimum required NVIDIA driver version is 450.80.02. .. code-block:: bash\n\n # Stop Driverless AI."
|
43 |
+
},
|
44 |
+
{
|
45 |
+
"output": " .. _feature_store:\n\n##########################\nH2O AI Feature Store Setup\n##########################\n\nYou can use the H2O AI Feature Store to store, update, and share the features data scientists, developers, and engineers need to build AI models."
|
46 |
+
},
|
47 |
+
{
|
48 |
+
"output": " Note: For more information on the H2O AI Feature Store, refer to the `official documentation <https://docs.h2o.ai/feature-store/latest-stable/docs/index.html>`_. Description of relevant configuration attributes\n\n\nThe following are descriptions of the relevant configuration attributes when enabling the H2O AI Feature Store data connector:\n\n- ``enabled_file_systems``: A list of file systems you want to enable."
|
49 |
+
},
|
50 |
+
{
|
51 |
+
"output": " - ``feature_store_endpoint_url``: A URL that points to the Feature Store server. - ``feature_store_enable_tls``: To enable TLS communication between DAI and the Feature Store server, set this to ``true``."
|
52 |
+
},
|
53 |
+
{
|
54 |
+
"output": " - ``authentication_method``: The authentication method used by DAI. When enabling the Feature Store data connector, this must be set to OpenID Connect (``authentication_method=\"oidc\"``). For information on setting up OIDC Authentication in Driverless AI, see :ref:`oidc_auth`."
|
55 |
+
},
|
56 |
+
{
|
57 |
+
"output": " \nUpgrading the Driverless AI Image\n~\n\n.. include:: upgrade-warning.frag\n \nUpgrading from Version 1.2.2 or Earlier\n'\n\nIt is not possible to upgrade from version 1.2.2 or earlier to the latest version."
|
58 |
+
},
|
59 |
+
{
|
60 |
+
"output": " Be sure to backup your data before doing this. Upgrading from Version 1.3.0 to 1.5.1\n'\n\n1. SSH into the IP address of the image instance and copy the existing experiments to a backup location:\n\n .. code-block:: bash\n\n # Set up a directory of the previous version name\n mkdir dai_rel_1.3.0\n\n # Copy the data, log, license, and tmp directories as backup\n cp -a ./data dai_rel_1.3.0/data\n cp -a ./log dai_rel_1.3.0/log\n cp -a ./license dai_rel_1.3.0/license\n cp -a ./tmp dai_rel_1.3.0/tmp\n\n2."
|
61 |
+
},
|
62 |
+
{
|
63 |
+
"output": " Replace VERSION and BUILD below with the Driverless AI version. .. code-block:: bash\n\n wget https://s3.amazonaws.com/artifacts.h2o.ai/releases/ai/h2o/dai/VERSION-BUILD/x86_64/dai-docker-ubi8-x86_64-VERSION.tar.gz\n\n3."
|
64 |
+
},
|
65 |
+
{
|
66 |
+
"output": " Run ``docker images`` to find the new image tag. 5. Start the Driverless AI Docker image and replace TAG below with the image tag. Depending on your install version, use the ``docker run runtime=nvidia`` (>= Docker 19.03) or ``nvidia-docker`` (< Docker 19.03) command."
|
67 |
+
},
|
68 |
+
{
|
69 |
+
"output": " .. tabs::\n\n .. tab:: >= Docker 19.03\n\n .. code-block:: bash\n\n # Start the Driverless AI Docker image\n docker run runtime=nvidia \\\n pid=host \\\n init \\\n rm \\\n shm-size=256m \\\n -u `id -u`:`id -g` \\\n -p 12345:12345 \\\n -v `pwd`/data:/data \\\n -v `pwd`/log:/log \\\n -v `pwd`/license:/license \\\n -v `pwd`/tmp:/tmp \\\n h2oai/dai-ubi8-x86_64:TAG\n\n .. tab:: < Docker 19.03\n\n .. code-block:: bash\n\n # Start the Driverless AI Docker image\n nvidia-docker run \\\n pid=host \\\n init \\\n rm \\\n shm-size=256m \\\n -u `id -u`:`id -g` \\\n -p 12345:12345 \\\n -v `pwd`/data:/data \\\n -v `pwd`/log:/log \\\n -v `pwd`/license:/license \\\n -v `pwd`/tmp:/tmp \\\n h2oai/dai-ubi8-x86_64:TAG\n\nUpgrading from version 1.5.2 or Later\n'\n\nUpgrading to versions 1.5.2 and later is no longer done via Docker."
|
70 |
+
},
|
71 |
+
{
|
72 |
+
"output": " Replace ``dai_NEWVERSION.deb`` below with the new Driverless AI version (for example, ``dai_1.8.4.1_amd64.deb``). Note that this upgrade process inherits the service user and group from /etc/dai/User.conf and /etc/dai/Group.conf."
|
73 |
+
},
|
74 |
+
{
|
75 |
+
"output": " We recommend to have NVIDIA driver >= |NVIDIA-driver-ver| installed (GPU only) in your host environment for a seamless experience on all architectures, including Ampere. Driverless AI ships with CUDA 11.2.2 for GPUs, but the driver must exist in the host environment."
|
76 |
+
},
|
77 |
+
{
|
78 |
+
"output": " For reference on CUDA Toolkit and Minimum Required Driver Versions and CUDA Toolkit and Corresponding Driver Versions, see `here <https://docs.nvidia.com/cuda/cuda-toolkit-release-notes/index.html>`__ ."
|
79 |
+
},
|
80 |
+
{
|
81 |
+
"output": " .. _feature_store:\n\n##########################\nH2O AI Feature Store Setup\n##########################\n\nYou can use the H2O AI Feature Store to store, update, and share the features data scientists, developers, and engineers need to build AI models. This page describes how to configure Driverless AI to work with the H2O AI Feature Store. Note: For more information on the H2O AI Feature Store, refer to the `official documentation <https://docs.h2o.ai/feature-store/latest-stable/docs/index.html>`_."
|
82 |
+
},
|
83 |
+
{
|
84 |
+
"output": " To enable the Feature Store data connector, ``feature_store`` must be added to this list of data sources. - ``feature_store_endpoint_url``: A URL that points to the Feature Store server. - ``feature_store_enable_tls``: To enable TLS communication between DAI and the Feature Store server, set this to ``true``. - ``feature_store_access_token_scopes``: A space-separated list of access token scopes used by the Feature Store connector for authentication. - ``authentication_method``: The authentication method used by DAI."
|
85 |
+
},
|
86 |
+
{
|
87 |
+
"output": " \nUpgrading the Driverless AI Image\n~\n\n.. include:: upgrade-warning.frag\n \nUpgrading from Version 1.2.2 or Earlier\n'\n\nIt is not possible to upgrade from version 1.2.2 or earlier to the latest version. You have to manually remove the 1.2.2 container and then reinstall the latest Driverless AI version. Be sure to backup your data before doing this. Upgrading from Version 1.3.0 to 1.5.1\n'\n\n1. SSH into the IP address of the image instance and copy the existing experiments to a backup location:\n\n .. code-block:: bash\n\n # Set up a directory of the previous version name\n mkdir dai_rel_1.3.0\n\n # Copy the data, log, license, and tmp directories as backup\n cp -a ./data dai_rel_1.3.0/data\n cp -a ./log dai_rel_1.3.0/log\n cp -a ./license dai_rel_1.3.0/license\n cp -a ./tmp dai_rel_1.3.0/tmp\n\n2."
|
88 |
+
},
|
89 |
+
{
|
90 |
+
"output": " Replace VERSION and BUILD below with the Driverless AI version. .. code-block:: bash\n\n wget https://s3.amazonaws.com/artifacts.h2o.ai/releases/ai/h2o/dai/VERSION-BUILD/x86_64/dai-docker-ubi8-x86_64-VERSION.tar.gz\n\n3. Use the ``docker load`` command to load the image:\n\n .. code-block:: bash\n\n docker load < dai-docker-ubi8-x86_64-VERSION.tar.gz\n\n4. Run ``docker images`` to find the new image tag. 5. Start the Driverless AI Docker image and replace TAG below with the image tag. Depending on your install version, use the ``docker run runtime=nvidia`` (>= Docker 19.03) or ``nvidia-docker`` (< Docker 19.03) command."
|
91 |
+
},
|
92 |
+
{
|
93 |
+
"output": " .. tabs::\n\n .. tab:: >= Docker 19.03\n\n .. code-block:: bash\n\n # Start the Driverless AI Docker image\n docker run runtime=nvidia \\\n pid=host \\\n init \\\n rm \\\n shm-size=256m \\\n -u `id -u`:`id -g` \\\n -p 12345:12345 \\\n -v `pwd`/data:/data \\\n -v `pwd`/log:/log \\\n -v `pwd`/license:/license \\\n -v `pwd`/tmp:/tmp \\\n h2oai/dai-ubi8-x86_64:TAG\n\n .. tab:: < Docker 19.03\n\n .. code-block:: bash\n\n # Start the Driverless AI Docker image\n nvidia-docker run \\\n pid=host \\\n init \\\n rm \\\n shm-size=256m \\\n -u `id -u`:`id -g` \\\n -p 12345:12345 \\\n -v `pwd`/data:/data \\\n -v `pwd`/log:/log \\\n -v `pwd`/license:/license \\\n -v `pwd`/tmp:/tmp \\\n h2oai/dai-ubi8-x86_64:TAG\n\nUpgrading from version 1.5.2 or Later\n'\n\nUpgrading to versions 1.5.2 and later is no longer done via Docker."
|
94 |
+
},
|
95 |
+
{
|
96 |
+
"output": " Replace ``dai_NEWVERSION.deb`` below with the new Driverless AI version (for example, ``dai_1.8.4.1_amd64.deb``). Note that this upgrade process inherits the service user and group from /etc/dai/User.conf and /etc/dai/Group.conf. You do not need to manually specify the DAI_USER or DAI_GROUP environment variables during an upgrade. We recommend to have NVIDIA driver >= |NVIDIA-driver-ver| installed (GPU only) in your host environment for a seamless experience on all architectures, including Ampere."
|
97 |
+
},
|
98 |
+
{
|
99 |
+
"output": " Go to `NVIDIA download driver <https://www.nvidia.com/Download/index.aspx>`__ to get the latest NVIDIA Tesla A/T/V/P/K series drivers. For reference on CUDA Toolkit and Minimum Required Driver Versions and CUDA Toolkit and Corresponding Driver Versions, see `here <https://docs.nvidia.com/cuda/cuda-toolkit-release-notes/index.html>`__ . .. note::\n\tIf you are using K80 GPUs, the minimum required NVIDIA driver version is 450.80.02. .. code-block:: bash\n\n # Stop Driverless AI. sudo systemctl stop dai\n\n # Backup your /opt/h2oai/dai/tmp directory at this time."
|
100 |
+
}
|
101 |
+
]
|
data/dai_faq.json
ADDED
@@ -0,0 +1,477 @@
|
|
|
|
|
|
|
|
|
|
|
|
|
|
|
|
|
|
|
|
|
|
|
|
|
|
|
|
|
|
|
|
|
|
|
|
|
|
|
|
|
|
|
|
|
|
|
|
|
|
|
|
|
|
|
|
|
|
|
|
|
|
|
|
|
|
|
|
|
|
|
|
|
|
|
|
|
|
|
|
|
|
|
|
|
|
|
|
|
|
|
|
|
|
|
|
|
|
|
|
|
|
|
|
|
|
|
|
|
|
|
|
|
|
|
|
|
|
|
|
|
|
|
|
|
|
|
|
|
|
|
|
|
|
|
|
|
|
|
|
|
|
|
|
|
|
|
|
|
|
|
|
|
|
|
|
|
|
|
|
|
|
|
|
|
|
|
|
|
|
|
|
|
|
|
|
|
|
|
|
|
|
|
|
|
|
|
|
|
|
|
|
|
|
|
|
|
|
|
|
|
|
|
|
|
|
|
|
|
|
|
|
|
|
|
|
|
|
|
|
|
|
|
|
|
|
|
|
|
|
|
|
|
|
|
|
|
|
|
|
|
|
|
|
|
|
|
|
|
|
|
|
|
|
|
|
|
|
|
|
|
|
|
|
|
|
|
|
|
|
|
|
|
|
|
|
|
|
|
|
|
|
|
|
|
|
|
|
|
|
|
|
|
|
|
|
|
|
|
|
|
|
|
|
|
|
|
|
|
|
|
|
|
|
|
|
|
|
|
|
|
|
|
|
|
|
|
|
|
|
|
|
|
|
|
|
|
|
|
|
|
|
|
|
|
|
|
|
|
|
|
|
|
|
|
|
|
|
|
|
|
|
|
|
|
|
|
|
|
|
|
|
|
|
|
|
|
|
|
|
|
|
|
|
|
|
|
|
|
|
|
|
|
|
|
|
|
|
|
|
|
|
|
|
|
|
|
|
|
|
|
|
|
|
|
|
|
|
|
|
|
|
|
|
|
|
|
|
|
|
|
|
|
|
|
|
|
|
|
|
|
|
|
|
|
|
|
|
|
|
|
|
|
|
|
|
|
|
|
|
|
|
|
|
|
|
|
|
|
|
|
|
|
|
|
|
|
|
|
|
|
|
|
|
|
|
|
|
|
|
|
|
|
|
|
|
|
|
|
|
|
|
|
|
|
|
|
|
|
|
|
|
|
|
|
|
|
|
|
|
|
|
|
|
|
|
|
|
|
|
|
|
|
|
|
|
|
|
|
|
|
|
|
|
|
|
|
|
|
|
|
|
|
|
|
|
|
|
|
|
|
|
|
|
|
|
|
|
|
|
|
|
|
|
|
|
|
|
|
|
|
|
|
|
|
|
|
|
|
|
|
|
|
|
|
|
|
|
|
|
|
|
|
|
|
|
|
|
|
|
|
|
|
|
|
|
|
|
|
|
|
|
|
|
|
|
|
|
|
|
|
|
|
|
|
|
|
|
|
|
|
|
|
|
|
|
|
|
|
|
|
|
|
|
|
|
|
|
|
|
|
|
|
|
|
|
|
|
|
|
|
|
|
|
|
|
|
|
|
|
|
|
|
|
|
|
|
|
|
|
|
|
|
|
|
|
|
|
|
|
|
|
|
|
|
|
|
|
|
|
|
|
|
|
|
|
|
|
|
|
|
|
|
|
|
|
|
|
|
|
|
|
|
|
|
|
|
|
|
|
|
|
|
|
|
|
|
|
|
|
|
|
|
|
|
|
|
|
|
|
|
|
|
|
|
|
|
|
|
|
|
|
|
|
|
|
|
|
|
|
|
|
|
|
|
|
|
|
|
|
|
|
|
|
|
|
|
|
|
|
|
|
|
|
|
|
|
|
|
|
|
|
|
|
|
|
|
|
|
|
|
|
|
|
|
|
|
|
|
|
|
|
|
|
|
|
|
|
|
|
|
|
|
|
|
|
|
|
|
|
|
|
|
|
|
|
|
|
|
|
|
|
|
|
|
|
|
|
|
|
|
|
|
|
|
|
|
|
|
|
|
|
|
|
|
|
|
|
|
|
|
|
|
|
|
|
|
|
|
|
|
|
|
|
|
|
|
|
|
|
|
|
|
|
|
|
|
|
|
|
|
|
|
|
|
|
|
|
|
|
|
|
|
|
|
|
|
|
|
|
|
|
|
|
|
|
|
|
|
|
|
|
|
|
|
|
|
1 |
+
[
|
2 |
+
{
|
3 |
+
"instruction": "How is Driverless AI different than any other black box ML algorithm?",
|
4 |
+
"output": "Driverless AI uses many techniques (some older and some cutting-edge) for interpreting black box models including creating reason codes for every prediction the system makes. We have also created numerous open source code examples and free publications that explain these techniques. See the list below for links to these resources and for references for the interpretability techniques.Open source interpretability examples:https://github.com/jphall663/interpretable_machine_learning_with_pythonhttps://content.oreilly.com/oriole/Interpretable-machine-learning-with-Python-XGBoost-and-H2Ohttps://github.com/h2oai/mli-resourcesFree Machine Learning Interpretability publications:http://www.oreilly.com/data/free/an-introduction-to-machine-learning-interpretability.csphttp://docs.h2o.ai/driverless-ai/latest-stable/docs/booklets/MLIBooklet.pdfMachine Learning Techniques already in Driverless AI:Tree-based Variable Importance: https://web.stanford.edu/~hastie/ElemStatLearn/printings/ESLII_print12.pdfPartial Dependence: https://web.stanford.edu/~hastie/ElemStatLearn/printings/ESLII_print12.pdfLIME: http://www.kdd.org/kdd2016/papers/files/rfp0573-ribeiroA.pdfLOCO: http://www.stat.cmu.edu/~ryantibs/papers/conformal.pdfICE: https://arxiv.org/pdf/1309.6392.pdfSurrogate Models:https://papers.nips.cc/paper/1152-extracting-tree-structured-representations-of-trained-networks.pdfhttps://arxiv.org/pdf/1705.08504.pdfShapley Explanations: http://papers.nips.cc/paper/7062-a-unified-approach-to-interpreting-model-predictions",
|
5 |
+
"prompt_type": "human_bot"
|
6 |
+
},
|
7 |
+
{
|
8 |
+
"instruction": "How often do new versions come out?",
|
9 |
+
"output": "The frequency of major new Driverless AI releases has historically been about every two months.Installation/Upgrade/Authentication",
|
10 |
+
"prompt_type": "human_bot"
|
11 |
+
},
|
12 |
+
{
|
13 |
+
"instruction": "How can I change my username and password?",
|
14 |
+
"output": "The username and password are tied to the experiments you have created. For example, if I log in with the username/password: megan/megan and start an experiment, then I would need to log back in with the same username and password to see those experiments. The username and password, however, does not limit your access to Driverless AI. If you want to use a new user name and password, you can log in again with a new username and password, but keep in mind that you won't see your old experiments.",
|
15 |
+
"prompt_type": "human_bot"
|
16 |
+
},
|
17 |
+
{
|
18 |
+
"instruction": "Can Driverless AI run on CPU-only machines?",
|
19 |
+
"output": "Yes, Driverless AI can run on machines with CPUs only, though GPUs are recommended. Installation instructions are available for GPU and CPU systems. Refer to :ref:`before_you_begin` for more information.No role entry for \"ref\" in module \"docutils.parsers.rst.languages.en\".\nTrying \"ref\" as canonical role name.Unknown interpreted text role \"ref\".",
|
20 |
+
"prompt_type": "human_bot"
|
21 |
+
},
|
22 |
+
{
|
23 |
+
"instruction": "How can I upgrade to a newer version of Driverless AI?",
|
24 |
+
"output": "Upgrade instructions vary depending on your environment. Refer to the installation section for your environment. Upgrade instructions are included there.",
|
25 |
+
"prompt_type": "human_bot"
|
26 |
+
},
|
27 |
+
{
|
28 |
+
"instruction": "What kind of authentication is supported in Driverless AI?",
|
29 |
+
"output": "Driverless AI supports Client Certificate, LDAP, Local, mTLS, OpenID, none, and unvalidated (default) authentication. These can be configured by setting the appropriate environment variables in the config.toml file or by specifying the environment variables when starting Driverless AI. Refer to :ref:`dai_auth` for more information.No role entry for \"ref\" in module \"docutils.parsers.rst.languages.en\".\nTrying \"ref\" as canonical role name.Unknown interpreted text role \"ref\".",
|
30 |
+
"prompt_type": "human_bot"
|
31 |
+
},
|
32 |
+
{
|
33 |
+
"instruction": "How can I automatically turn on persistence each time the GPU system reboots?",
|
34 |
+
"output": "For GPU machines, the sudo nvidia-persistenced --user dai command can be run after each reboot to enable persistence. For systems that have systemd, it is possible to automatically enable persistence after each reboot by removing the --no-persistence-mode flag from nvidia-persistenced.service. Before running the steps below, be sure to review the following for more information:https://docs.nvidia.com/deploy/driver-persistence/index.html#persistence-daemonhttps://docs.nvidia.com/deploy/driver-persistence/index.html#installationRun the following to stop the nvidia-persistenced.service:Cannot analyze code. Pygments package not found... code:: bash\n\n sudo systemctl stop nvidia-persistenced.service\nOpen the file /lib/systemd/system/nvidia-persistenced.service. This file includes a line \"ExecStart=/usr/bin/nvidia-persistenced --user nvidia-persistenced --no-persistence-mode --verbose\".Remove the flag --no-persistence-mode from that line so that it reads:Enumerated list start value not ordinal-1: \"2\" (ordinal 2)Cannot analyze code. Pygments package not found... code:: bash\n\n ExecStart=/usr/bin/nvidia-persistenced --user nvidia-persistenced --verbose\nRun the following command to start the nvidia-persistenced.service:Enumerated list start value not ordinal-1: \"4\" (ordinal 4)Cannot analyze code. Pygments package not found... code:: bash\n\n sudo systemctl start nvidia-persistenced.service\n",
|
35 |
+
"prompt_type": "human_bot"
|
36 |
+
},
|
37 |
+
{
|
38 |
+
"instruction": "How can I start Driverless AI on a different port than 12345?",
|
39 |
+
"output": "No directive entry for \"tabs\" in module \"docutils.parsers.rst.languages.en\".\nTrying \"tabs\" as canonical directive name.Unknown directive type \"tabs\"... tabs::\n .. group-tab:: Docker Image Installs\n\n When starting Driverless AI in Docker, the ``-p`` option specifies the port on which Driverless AI will run. Change this option in the start script if you need to run on a port other than 12345. The following example shows how to run on port 22345. (Change ``nvidia-docker run`` to ``docker-run`` if needed.) Keep in mind that `priviliged ports will require root access <https://www.w3.org/Daemon/User/Installation/PrivilegedPorts.html>`__.\n\n .. code-block:: bash\n :substitutions:\n\n nvidia-docker run \\\n --pid=host \\\n --init \\\n --rm \\\n --shm-size=256m \\\n -u `id -u`:`id -g` \\\n -p 22345:12345 \\\n -v `pwd`/data:/data \\\n -v `pwd`/log:/log \\\n -v `pwd`/license:/license \\\n -v `pwd`/tmp:/tmp \\\n h2oai/dai-ubi8-x86_64:|tag|\n\n .. group-tab:: Native Installs\n\n To run on a port other than 12345, update the port value in the **config.toml** file. The following example shows how to run Driverless AI on port 22345. Keep in mind that `priviliged ports will require root access <https://www.w3.org/Daemon/User/Installation/PrivilegedPorts.html>`__.\n\n ::\n\n # Export the Driverless AI config.toml file (or add it to ~/.bashrc)\n export DRIVERLESS_AI_CONFIG_FILE=\u201c/config/config.toml\u201d\n\n # IP address and port for Driverless AI HTTP server.\n ip = \"127.0.0.1\"\n port = 22345\n\n Point to this updated config file when restarting Driverless AI.\n",
|
40 |
+
"prompt_type": "human_bot"
|
41 |
+
},
|
42 |
+
{
|
43 |
+
"instruction": "Can I set up TLS/SSL on Driverless AI?",
|
44 |
+
"output": "Yes, Driverless AI provides configuration options that let you set up HTTPS/TLS/SSL. You will need to have your own SSL certificate, or you can create a self-signed certificate for yourself.To enable HTTPS/TLS/SSL on the Driverless AI server, add the following to the config.toml file:Cannot analyze code. Pygments package not found... code:: bash\n\n enable_https = true\n ssl_key_file = \"/etc/dai/private_key.pem\"\n ssl_crt_file = \"/etc/dai/cert.pem\"\nYou can make a self-signed certificate for testing with the following commands:Cannot analyze code. Pygments package not found... code:: bash\n\n umask 077\n openssl req -x509 -newkey rsa:4096 -keyout private_key.pem -out cert.pem -days 20 -nodes -subj '/O=Driverless AI'\n sudo chown dai:dai cert.pem private_key.pem\n sudo mv cert.pem private_key.pem /etc/dai\nTo configure specific versions of TLS/SSL, enable or disable the following settings in the config.toml file:Cannot analyze code. Pygments package not found... code:: bash\n\n ssl_no_sslv2 = true\n ssl_no_sslv3 = true\n ssl_no_tlsv1 = true\n ssl_no_tlsv1_1 = true\n ssl_no_tlsv1_2 = false\n ssl_no_tlsv1_3 = false\n",
|
45 |
+
"prompt_type": "human_bot"
|
46 |
+
},
|
47 |
+
{
|
48 |
+
"instruction": "Can I set up TLS/SSL on Driverless AI in AWS?",
|
49 |
+
"output": "Yes, you can set up HTTPS/TLS/SSL on Driverless AI running in an AWS environment. HTTPS/TLS/SSL needs to be configured on the host machine, and the necessary ports will need to be opened on the AWS side. You will need to have your own TLS/SSL cert or you can create a self signed cert for yourself.The following is a very simple example showing how to configure HTTPS with a proxy pass to the port on the container 12345 with the keys placed in /etc/nginx/. Replace <server_name> with your server name.Cannot analyze code. Pygments package not found... code:: bash\n\n server {\n listen 80;\n return 301 https://$host$request_uri;\n }\n\n server {\n listen 443;\n\n # Specify your server name here\n server_name <server_name>;\n\n ssl_certificate /etc/nginx/cert.crt;\n ssl_certificate_key /etc/nginx/cert.key;\n ssl on;\n ssl_session_cache builtin:1000 shared:SSL:10m;\n ssl_protocols TLSv1 TLSv1.1 TLSv1.2;\n ssl_ciphers HIGH:!aNULL:!eNULL:!EXPORT:!CAMELLIA:!DES:!MD5:!PSK:!RC4;\n ssl_prefer_server_ciphers on;\n\n access_log /var/log/nginx/dai.access.log;\n\n location / {\n proxy_set_header Host $host;\n proxy_set_header X-Real-IP $remote_addr;\n proxy_set_header X-Forwarded-For $proxy_add_x_forwarded_for;\n proxy_set_header X-Forwarded-Proto $scheme;\n\n # Fix the \u201cIt appears that your reverse proxy set up is broken\" error.\n proxy_pass http://localhost:12345;\n proxy_read_timeout 90;\n\n # Specify your server name for the redirect\n proxy_redirect http://localhost:12345 https://<server_name>;\n }\n }\nMore information about SSL for Nginx in Ubuntu 16.04 can be found here: https://www.digitalocean.com/community/tutorials/how-to-create-a-self-signed-ssl-certificate-for-nginx-in-ubuntu-16-04.",
|
50 |
+
"prompt_type": "human_bot"
|
51 |
+
},
|
52 |
+
{
|
53 |
+
"instruction": "I received a \"package dai-<version>.x86_64 does not verify: no digest\" error during the installation. How can I fix this?",
|
54 |
+
"output": "You will recieve a \"package dai-<version>.x86_64 does not verify: no digest\" error when installing the rpm using an RPM version newer than 4.11.3. You can run the following as a workaround, replacing <version> with your DAI version:Cannot analyze code. Pygments package not found... code:: bash\n\n rpm --nodigest -i dai-<version>.x86_64.rpm\n",
|
55 |
+
"prompt_type": "human_bot"
|
56 |
+
},
|
57 |
+
{
|
58 |
+
"instruction": "I received a \"Must have exactly one OpenCL platform 'NVIDIA CUDA'\" error. How can I fix that?",
|
59 |
+
"output": "If you encounter problems with opencl errors at server time, you may see the following message:Cannot analyze code. Pygments package not found... code:: bash\n\n 2018-11-08 14:26:15,341 C: D:452.2GB M:246.0GB 21603 ERROR : Must have exactly one OpenCL platform 'NVIDIA CUDA', but got:\n Platform #0: Clover\n Platform #1: NVIDIA CUDA\n +-- Device #0: GeForce GTX 1080 Ti\n +-- Device #1: GeForce GTX 1080 Ti\n +-- Device #2: GeForce GTX 1080 Ti\n\n Uninstall all but 'NVIDIA CUDA' platform.\nFor Ubuntu, the solution is to run the following:Cannot analyze code. Pygments package not found... code:: bash\n\n sudo apt-get remove mesa-opencl-icd\n",
|
60 |
+
"prompt_type": "human_bot"
|
61 |
+
},
|
62 |
+
{
|
63 |
+
"instruction": "Is it possible for multiple users to share a single Driverless AI instance?",
|
64 |
+
"output": "Driverless AI supports multiple users, and Driverless AI is licensed per a single named user. Therefore, in order, to have different users run experiments simultaneously, they would each need a license. Driverless AI manages the GPU(s) that it is given and ensures that different experiments from different users can run safely simultaneously and don\u2019t interfere with each other. So when two licensed users log in with different credentials, then neither of them will see the other\u2019s experiment. Similarly, if a licensed user logs in using a different set of credentials, then that user will not see any previously run experiments.",
|
65 |
+
"prompt_type": "human_bot"
|
66 |
+
},
|
67 |
+
{
|
68 |
+
"instruction": "Can multiple Driverless AI users share a GPU server?",
|
69 |
+
"output": "Yes, you can allocate multiple users in a single GPU box. For example, a single box with four GPUs can allocate that User1 has two GPUs and User2 has the other two GPUs. This is accomplished by having two separated Driverless AI instances running on the same server.There are two ways to assign specific GPUs to Driverless AI. And in the scenario with four GPUs (two GPUs allocated to two users), both of these options allow each Docker container only to see two GPUs.Use the CUDA_VISIBLE_DEVICES environment variable. In the case of Docker deployment, this will translate in passing the -e CUDA_VISIBLE_DEVICES=\"0,1\" to the nvidia-docker run command.Passing the NV_GPU option at the beginning of the nvidia-docker run command. (See example below.)Error in \"code-block\" directive:\nunknown option: \"substitutions\"... code-block:: bash\n :substitutions:\n\n #Team 1\n NV_GPU='0,1' nvidia-docker run\n --pid=host\n --init\n --rm\n --shm-size=256m\n -u id -u:id -g\n -p port-to-team:12345\n -e DRIVERLESS_AI_CONFIG_FILE=\"/config/config.toml\"\n -v /data:/data\n -v /log:/log\n -v /license:/license\n -v /tmp:/tmp\n -v /config:/config\n h2oai/dai-ubi8-x86_64:|tag|\n\n\n #Team 2\n NV_GPU='0,1' nvidia-docker run\n --pid=host\n --init\n --rm\n --shm-size=256m\n -u id -u:id -g\n -p port-to-team:12345\n -e DRIVERLESS_AI_CONFIG_FILE=\"/config/config.toml\"\n -v /data:/data\n -v /log:/log\n -v /license:/license\n -v /tmp:/tmp\n -v /config:/config\n h2oai/dai-ubi8-x86_64:|tag|\nNote, however, that a Driverless AI instance expects to fully utilize and not share the GPUs that are assigned to it. Sharing a GPU with other Driverless AI instances or other running programs can result in out-of-memory issues.",
|
70 |
+
"prompt_type": "human_bot"
|
71 |
+
},
|
72 |
+
{
|
73 |
+
"instruction": "How can I retrieve a list of Driverless AI users?",
|
74 |
+
"output": "A list of users can be retrieved using the Python client.Cannot analyze code. Pygments package not found... code:: bash\n\n h2o = Client(address='http://<client_url>:12345', username='<username>', password='<password>')\n h2o.get_users()\n",
|
75 |
+
"prompt_type": "human_bot"
|
76 |
+
},
|
77 |
+
{
|
78 |
+
"instruction": "Start of Driverless AI fails on the message ``Segmentation fault (core dumped)`` on Ubuntu 18/RHEL 7.6. How can I fix this?",
|
79 |
+
"output": "This problem is caused by the font NotoColorEmoji.ttf, which cannot be processed by the Python matplotlib library. A workaround is to disable the font by renaming it. (Do not use fontconfig because it is ignored by matplotlib.) The following will print out the command that should be executed.Cannot analyze code. Pygments package not found... code:: bash\n\n sudo find / -name \"NotoColorEmoji.ttf\" 2>/dev/null | xargs -I{} echo sudo mv {} {}.backup\n\n",
|
80 |
+
"prompt_type": "human_bot"
|
81 |
+
},
|
82 |
+
{
|
83 |
+
"instruction": "Which Linux systems does Driverless AI support?",
|
84 |
+
"output": "Supported Linux systems include x86_64 RHEL 7, RHEL 8, CentOS 7, and CentOS 8.Data",
|
85 |
+
"prompt_type": "human_bot"
|
86 |
+
},
|
87 |
+
{
|
88 |
+
"instruction": "Is there a file size limit for datasets?",
|
89 |
+
"output": "For GBMs, the file size for datasets is limited by the collective CPU or GPU memory on the system, but we continue to make optimizations for getting more data into an experiment, such as using TensorFlow streaming to stream to arbitrarily large datasets.",
|
90 |
+
"prompt_type": "human_bot"
|
91 |
+
},
|
92 |
+
{
|
93 |
+
"instruction": "How can I import CSV files that use UTF-8 encoding into Excel?",
|
94 |
+
"output": "Excel requires a byte order mark (BOM) to correctly identify CSV files that use UTF-8 encoding. Refer to the following FAQ entry for more information on how to use a BOM when writing CSV files with datatable.",
|
95 |
+
"prompt_type": "human_bot"
|
96 |
+
},
|
97 |
+
{
|
98 |
+
"instruction": "Can a byte order mark be used when writing CSV files with datatable?",
|
99 |
+
"output": "Yes, a byte order mark (BOM) can be used when writing CSV files with datatable by enabling datatable_bom_csv in the config.toml file when starting Driverless AI.Note: Support for UTF-8 encoding in Excel requires the use of a BOM.",
|
100 |
+
"prompt_type": "human_bot"
|
101 |
+
},
|
102 |
+
{
|
103 |
+
"instruction": "Which version of Longhorn is supported by Driverless AI?",
|
104 |
+
"output": "Driverless AI supports Longhorn v1.1.0 or later.",
|
105 |
+
"prompt_type": "human_bot"
|
106 |
+
},
|
107 |
+
{
|
108 |
+
"instruction": "Is it possible to download a transformed test dataset in Driverless AI?",
|
109 |
+
"output": "Yes, a transformed test dataset can be downloaded in Driverless AI. To do this, click Model Actions > Transform Dataset on the completed experiment page, then specify both a train and a test dataset to use for the transformation. The transformed test dataset is made available for download once this process is completed.Connectors",
|
110 |
+
"prompt_type": "human_bot"
|
111 |
+
},
|
112 |
+
{
|
113 |
+
"instruction": "Why can't I import a folder as a file when using a data connector on Windows?",
|
114 |
+
"output": "If you try to use the Import Folder as File option via a data connector on Windows, the import will fail if the folder contains files that do not have file extensions. For example, if a folder contains the files file1.csv, file2.csv, file3.csv, and _SUCCESS, the function will fail due to the presence of the _SUCCESS file.Note that this only occurs if the data is sourced from a volume that is mounted from the Windows filesystem onto the Docker container via -v /path/to/windows/filesystem:/path/in/docker/container flags. This error occurs because of the difference in how files without file extensions are treated in Windows and in the Docker container (CentOS Linux).",
|
115 |
+
"prompt_type": "human_bot"
|
116 |
+
},
|
117 |
+
{
|
118 |
+
"instruction": "I get a ClassNotFoundException error when I try to select a JDBC connection. How can I fix that?",
|
119 |
+
"output": "The folder storing the JDBC jar file must be visible/readable by the dai process user.If you downloaded the JDBC jar file from Oracle, they may provide you with a tar.gz file that you can unpackage with the following command:Cannot analyze code. Pygments package not found... code:: bash\n\n tar --no-same-permissions --no-same-owner -xzvf <my-jdbc-driver.tar>.gz\nAlternatively you can ensure that the permissions on the file are correct in general by running the following:Cannot analyze code. Pygments package not found... code:: bash\n\n chmod -R o+rx /path/to/folder_containing_jar_file\nFinally, if you just want to check the permissions use the command ls -altr and check the final 3 values in the permissions output.",
|
120 |
+
"prompt_type": "human_bot"
|
121 |
+
},
|
122 |
+
{
|
123 |
+
"instruction": "I get a org.datanucleus.exceptions.NucleusUserException: Please check your CLASSPATH and plugin specification error when attempting to connect to Hive. How can I fix that?",
|
124 |
+
"output": "Make sure hive-site.xml is configured in /etc/hive/conf and not in /etc/hadoop/conf.",
|
125 |
+
"prompt_type": "human_bot"
|
126 |
+
},
|
127 |
+
{
|
128 |
+
"instruction": "I get a \"Permission Denied\" error during Hive import. How do I fix this?",
|
129 |
+
"output": "If you see the following error, your Driverless AI instance may not be able to create a temporary Hive folder due to file system permissions restrictions.Cannot analyze code. Pygments package not found... code:: bash\n\n ERROR HiveAgent: Error during execution of query: java.lang.RuntimeException: java.lang.RuntimeException: java.io.IOException: Permission denied;\n org.apache.spark.sql.AnalysisException: java.lang.RuntimeException: java.lang.RuntimeException: java.io.IOException: Permission denied;\nTo fix this error, add the following name-value pair to your hive-site.xml file to specify the location that is accessible to Driverless AI (that is, your Driverless AI /tmp directory).Cannot analyze code. Pygments package not found... code:: bash\n\n <property>\n <name>hive.exec.local.scratchdir</name>\n <value>/path/to/dai/tmp</value>\n </property>\nRecipes",
|
130 |
+
"prompt_type": "human_bot"
|
131 |
+
},
|
132 |
+
{
|
133 |
+
"instruction": "Where can I retrieve H2O's custom recipes?",
|
134 |
+
"output": "H2O's custom recipes can be obtained from the official :recipes-repo:`Recipes for Driverless AI repository <https://github.com/h2oai/driverlessai-recipes/tree/>`.No role entry for \"recipes-repo\" in module \"docutils.parsers.rst.languages.en\".\nTrying \"recipes-repo\" as canonical role name.Unknown interpreted text role \"recipes-repo\".",
|
135 |
+
"prompt_type": "human_bot"
|
136 |
+
},
|
137 |
+
{
|
138 |
+
"instruction": "How can I create my own custom recipe?",
|
139 |
+
"output": "Refer to the :recipes-writing:`How to Write a Recipe <https://github.com/h2oai/driverlessai-recipes/blob/>` guide for details on how to create your own custom recipe.No role entry for \"recipes-writing\" in module \"docutils.parsers.rst.languages.en\".\nTrying \"recipes-writing\" as canonical role name.Unknown interpreted text role \"recipes-writing\".",
|
140 |
+
"prompt_type": "human_bot"
|
141 |
+
},
|
142 |
+
{
|
143 |
+
"instruction": "Are MOJOs supported for experiments that use custom recipes?",
|
144 |
+
"output": "In most cases, MOJOs will not be available for custom recipes. Unless the recipe is simple, creating the MOJO is only possible with additional MOJO runtime support. Contact [email protected] for more information about creating MOJOs for custom recipes. (Note: The Python Scoring Pipeline features full support for custom recipes.)",
|
145 |
+
"prompt_type": "human_bot"
|
146 |
+
},
|
147 |
+
{
|
148 |
+
"instruction": "How can I use BYOR in my airgapped installation?",
|
149 |
+
"output": "If your Driverless AI environment cannot access Internet and, thus, cannot access Driverless AI's \"Bring Your Own Recipes\" from GitHub, please contact H2O support. We can work with you directly to help you access recipes.",
|
150 |
+
"prompt_type": "human_bot"
|
151 |
+
},
|
152 |
+
{
|
153 |
+
"instruction": "When enabling recipes in Driverless AI, can I install Python packages from my organization's internal Python package index?",
|
154 |
+
"output": "Yes\u2014you can use the pip_install_options :ref:`TOML option <understanding-configs>` to specify your organization's internal Python package index as follows:No role entry for \"ref\" in module \"docutils.parsers.rst.languages.en\".\nTrying \"ref\" as canonical role name.Unknown interpreted text role \"ref\".pip_install_options=\"['--extra-index-url', 'http://my-own-repo:port']\"For more information on the --extra-index-url <url> pip install option, refer to the official pip documentation.Experiments",
|
155 |
+
"prompt_type": "human_bot"
|
156 |
+
},
|
157 |
+
{
|
158 |
+
"instruction": "How much memory does Driverless AI require in order to run experiments?",
|
159 |
+
"output": "Right now, Driverless AI requires approximately 10x the size of the data in system memory.",
|
160 |
+
"prompt_type": "human_bot"
|
161 |
+
},
|
162 |
+
{
|
163 |
+
"instruction": "How many columns can Driverless AI handle?",
|
164 |
+
"output": "Driverless AI has been tested on datasets with 10k columns. When running experiments on wide data, Driverless AI automatically checks if it is running out of memory, and if it is, it reduces the number of features until it can fit in memory. This may lead to a worse model, but Driverless AI shouldn't crash because the data is wide.",
|
165 |
+
"prompt_type": "human_bot"
|
166 |
+
},
|
167 |
+
{
|
168 |
+
"instruction": "How should I use Driverless AI if I have large data?",
|
169 |
+
"output": "Driverless AI can handle large datasets out of the box. For very large datasets (more than 10 billion rows x columns), we recommend sampling your data for Driverless AI. Keep in mind that the goal of driverless AI is to go through many features and models to find the best modeling pipeline, and not to just train a few models on the raw data (H2O-3 is ideally suited for that case).For large datasets, the recommended steps are:Run with the recommended accuracy/time/interpretability settings first, especially accuracy <= 7Gradually increase accuracy settings to 7 and choose accuracy 9 or 10 only after observing runs with <= 7.",
|
170 |
+
"prompt_type": "human_bot"
|
171 |
+
},
|
172 |
+
{
|
173 |
+
"instruction": "How does Driverless AI detect the ID column?",
|
174 |
+
"output": "The ID column logic is one of the following:The column is named 'id', 'Id', 'ID' or 'iD' exactlyThe column contains a significant number of unique values (above max_relative_cardinality in the config.toml file or Max. allowed fraction of uniques for integer and categorical cols in Expert settings)",
|
175 |
+
"prompt_type": "human_bot"
|
176 |
+
},
|
177 |
+
{
|
178 |
+
"instruction": "Can Driverless AI handle data with missing values/nulls?",
|
179 |
+
"output": "Yes, data that is imported into Driverless AI can include missing values. Feature engineering is fully aware of missing values, and missing values are treated as information - either as a special categorical level or as a special number. So for target encoding, for example, rows with a certain missing feature will belong to the same group. For Categorical Encoding where aggregations of a numeric columns are calculated for a grouped categorical column, missing values are kept. The formula for calculating the mean is the sum of non-missing values divided by the count of all non-missing values. For clustering, we impute missing values. And for frequency encoding, we count the number of rows that have a certain missing feature.The imputation strategy is as follows:XGBoost/LightGBM do not need missing value imputation and may, in fact, perform worse with any specific other strategy unless the user has a strong understanding of the data.Driverless AI automatically imputes missing values using the mean for GLM.Driverless AI provides an imputation setting for TensorFlow in the config.toml file: tf_nan_impute_value post-normalization. If you set this option to 0, then missing values will be imputed. Setting it to (for example) +5 will specify 5 standard deviations outside the distribution. The default for TensorFlow is -5, which specifies that TensorFlow will treat NAs like a missing value. We recommend that you specify 0 if the mean is better.More information is available in the Missing and Unseen Values Handling section.",
|
180 |
+
"prompt_type": "human_bot"
|
181 |
+
},
|
182 |
+
{
|
183 |
+
"instruction": "How does Driverless AI deal with categorical variables? What if an integer column should really be treated as categorical?",
|
184 |
+
"output": "If a column has string values, then Driverless AI will treat it as a categorical feature. There are multiple methods for how Driverless AI converts the categorical variables to numeric. These include:One Hot Encoding: creating dummy variables for each valueFrequency Encoding: replace category with how frequently it is seen in the dataTarget Encoding: replace category with the average target value (additional steps included to prevent overfitting)Weight of Evidence: calculate weight of evidence for each category (http://ucanalytics.com/blogs/information-value-and-weight-of-evidencebanking-case/)Driverless AI will try multiple methods for representing the column and determine which representation(s) are best.If the column has integers, Driverless AI will try treating the column as a categorical column and numeric column. It will treat any integer column as both categorical and numeric if the number of unique values is less than 50.This is configurable in the config.toml file:Cannot analyze code. Pygments package not found... code:: bash\n\n # Whether to treat some numerical features as categorical\n # For instance, sometimes an integer column may not represent a numerical feature but\n # represents different numerical codes instead.\n num_as_cat = true\n\n # Max number of unique values for integer/real columns to be treated as categoricals (test applies to first statistical_threshold_data_size_small rows only)\n max_int_as_cat_uniques = 50\n(Note: Driverless AI will also check if the distribution of any numeric column differs significantly from the distribution of typical numerical data using Benford's Law. If the column distribution does not obey Benford's Law, we will also try to treat it as categorical even if there are more than 50 unique values.)",
|
185 |
+
"prompt_type": "human_bot"
|
186 |
+
},
|
187 |
+
{
|
188 |
+
"instruction": "How are outliers handled?",
|
189 |
+
"output": "Outliers are not removed from the data. Instead Driverless AI finds the best way to represent data with outliers. For example, Driverless AI may find that binning a variable with outliers improves performance.For target columns, Driverless AI first determines the best representation of the column. It may find that for a target column with outliers, it is best to predict the log of the column.",
|
190 |
+
"prompt_type": "human_bot"
|
191 |
+
},
|
192 |
+
{
|
193 |
+
"instruction": "If I drop several columns from the Train dataset, will Driverless AI understand that it needs to drop the same columns from the Test dataset?",
|
194 |
+
"output": "If you drop columns from the training dataset, Driverless AI will do the same for the validation and test datasets (if the columns are present). There is no need for these columns because no features will be created from them.",
|
195 |
+
"prompt_type": "human_bot"
|
196 |
+
},
|
197 |
+
{
|
198 |
+
"instruction": "Does Driverless AI treat numeric variables as categorical variables?",
|
199 |
+
"output": "In certain cases, yes. You can prevent this behavior by setting the num_as_cat variable in your installation's config.toml file to false. You can have finer grain control over this behavior by excluding the Numeric to Categorical Target Encoding Transformer and the Numeric To Categorical Weight of Evidence Transformer and their corresponding genes in your installation's config.toml file. To learn more about the config.toml file, see the :ref:`config_file` section.No role entry for \"ref\" in module \"docutils.parsers.rst.languages.en\".\nTrying \"ref\" as canonical role name.Unknown interpreted text role \"ref\".",
|
200 |
+
"prompt_type": "human_bot"
|
201 |
+
},
|
202 |
+
{
|
203 |
+
"instruction": "Which algorithms are used in Driverless AI?",
|
204 |
+
"output": "Features are engineered with a proprietary stack of Kaggle-winning statistical approaches including some of the most sophisticated target encoding and likelihood estimates based on groupings, aggregations and joins, but we also employ linear models, neural nets, clustering and dimensionality reduction models and many traditional approaches such as one-hot encoding etc.On top of the engineered features, sophisticated models are fitted, including, but not limited to: XGBoost (both original XGBoost and 'lossguide' (LightGBM) mode), Decision Trees, GLM, TensorFlow (including a TensorFlow NLP recipe based on CNN Deeplearning models), RuleFit, FTRL (Follow the Regularized Leader), Isolation Forest, and Constant Models. (Refer to :ref:`supported_algorithms` for more information.) And additional algorithms can be added via :ref:`Recipes <custom-recipes>`.No role entry for \"ref\" in module \"docutils.parsers.rst.languages.en\".\nTrying \"ref\" as canonical role name.Unknown interpreted text role \"ref\".No role entry for \"ref\" in module \"docutils.parsers.rst.languages.en\".\nTrying \"ref\" as canonical role name.Unknown interpreted text role \"ref\".In general, GBMs are the best single-shot algorithms. Since 2006, boosting methods have proven to be the most accurate for noisy predictive modeling tasks outside of pattern recognition in images and sound (https://www.cs.cornell.edu/~caruana/ctp/ct.papers/caruana.icml06.pdf). The advent of XGBoost and Kaggle only cemented this position.",
|
205 |
+
"prompt_type": "human_bot"
|
206 |
+
},
|
207 |
+
{
|
208 |
+
"instruction": "Why do my selected algorithms not show up in the Experiment Preview?",
|
209 |
+
"output": "When changing the algorithms used via Expert Settings > Model and Expert Settings > Recipes, you may notice in the Experiment Preview that those changes are not applied. Driverless AI determines whether to include models and/or recipes based on a hierarchy of those expert settings as well as data types (numeric, categorical, text, image, etc.) and system properties (GPUs, multiple GPUs, etc.).Setting an Algorithm to \"OFF\" in Expert Settings: If an algorithm is turned OFF in Expert Settings (for example, GLM Models) when running, then that algorithm will not be included in the experiment.Algorithms Not Included from Recipes (BYOR): If an algorithm from a custom recipe is not selected for the experiment in the Include specific models option, then that algorithm will not be included in the experiment, regardless of whether that same algorithm is set to AUTO or ON on the Expert Settings > Model page.Algorithms Not Specified as \"OFF\" and Included from Recipes: If a Driverless AI algorithm is specified as either \"AUTO\" or \"ON\" and additional models are selected for the experiment in the Include specific models option, than those algorithms may or may not be included in the experiment. Driverless AI will determine the algorithms to use based on the data and experiment type.To show warnings in the preview for which models were not used, set show_inapplicable_models_preview = true in config.toml",
|
210 |
+
"prompt_type": "human_bot"
|
211 |
+
},
|
212 |
+
{
|
213 |
+
"instruction": "Why do my selected transformers not show up in the Experiment Preview?",
|
214 |
+
"output": "When changing the transformers used via Expert Settings > Transformers and Expert Settings > Recipes, you may notice in the Experiment Preview that those changes are not applied. Driverless AI determines whether to include transformers can be used based upon data types (numeric, categorical, text, image, etc.) and system properties (GPUs, multiple GPUs, etc.).Transformers Not Included from Recipes (BYOR): If a transformer from a custom recipe is not selected for the experiment in the Include specific transformers option, then that transformer will not be included in the experiment.To show warnings in the preview for which models were not used, set show_inapplicable_transformers_preview = true in config.toml",
|
215 |
+
"prompt_type": "human_bot"
|
216 |
+
},
|
217 |
+
{
|
218 |
+
"instruction": "How can we turn on TensorFlow Neural Networks so they are evaluated?",
|
219 |
+
"output": "Neural networks are considered by Driverless AI, although they may not be evaluated by default. To ensure that neural networks are tried, you can turn on TensorFlow in the Expert Settings:Once you have set TensorFlow to ON. You should see the Experiment Preview on the left hand side change and mention that it will evaluate TensorFlow models:We recommend using TensorFlow neural networks if you have a multinomial use case with more than 5 unique values.",
|
220 |
+
"prompt_type": "human_bot"
|
221 |
+
},
|
222 |
+
{
|
223 |
+
"instruction": "Does Driverless AI standardize the data?",
|
224 |
+
"output": "Driverless AI will automatically do variable standardization for certain algorithms. For example, with Linear Models and Neural Networks, the data is automatically standardized. For decision tree algorithms, however, we do not perform standardization because these algorithms do not benefit from standardization.",
|
225 |
+
"prompt_type": "human_bot"
|
226 |
+
},
|
227 |
+
{
|
228 |
+
"instruction": "What objective function is used in XGBoost?",
|
229 |
+
"output": "The objective function used in XGBoost is:reg:squarederror and a custom absolute error objective function for regressionbinary:logistic or multi:softprob for classificationThe objective function does not change depending on the scorer chosen. The scorer influences parameter tuning only. For regression, Tweedie, Gamma, and Poisson regression objectives are supported.More information on the XGBoost instantiations can be found in the logs and in the model summary, both of which can be downloaded from the GUI or found in the /tmp/h2oai_experiment_<name>/ folder on the server.",
|
230 |
+
"prompt_type": "human_bot"
|
231 |
+
},
|
232 |
+
{
|
233 |
+
"instruction": "Does Driverless AI perform internal or external validation?",
|
234 |
+
"output": "Driverless AI does internal validation when only training data is provided. It does external validation when training and validation data are provided. In either scenario, the validation data is used for all parameter tuning (models and features), not just for feature selection. Parameter tuning includes target transformation, model selection, feature engineering, feature selection, stacking, etc.Specifically:Internal validation (only training data given):Ideal when data is either close to i.i.d., or for time-series problemsInternal holdouts are used for parameter tuning, with temporal causality for time-series problemsWill do the full spectrum from single holdout split to 5-fold CV, depending on accuracy settingsNo need to split training data manuallyFinal models are trained using CV on the training dataExternal validation (training + validation data given):Ideal when there\u2019s some amount of drift in the data, and the validation set mimics the test set data better than the training dataNo training data wasted during training because training data not used for parameter tuningValidation data is used only for parameter tuning, and is not part of training dataNo CV possible because we explicitly do not want to overfit on the training dataNot allowed for time-series problems (see Time Series FAQ section that follows)Tip: If you want both training and validation data to be used for parameter tuning (the training process), just concatenate the datasets together and turn them both into training data for the \u201cinternal validation\u201d method.",
|
235 |
+
"prompt_type": "human_bot"
|
236 |
+
},
|
237 |
+
{
|
238 |
+
"instruction": "How does Driverless AI prevent overfitting?",
|
239 |
+
"output": "Driverless AI performs a number of checks to prevent overfitting. For example, during certain transformations, Driverless AI calculates the average on out-of-fold data using cross validation. Driverless AI also performs early stopping for every model built, ensuring that the model build will stop when it ceases to improve on holdout data. And additional steps to prevent overfitting include checking for i.i.d. and avoiding leakage during feature engineering.A blog post describing Driverless AI overfitting protection in greater detail is available here: https://www.h2o.ai/blog/driverless-ai-prevents-overfitting-leakage/.More aggressive overfit protection can be enabled by setting lock_ga_to_final_trees=true to true or using recipe='more_overfit_protection' and fixed_only_first_fold_model='true' and for time-series experiments allow_stabilize_varimp_for_ts=true.",
|
240 |
+
"prompt_type": "human_bot"
|
241 |
+
},
|
242 |
+
{
|
243 |
+
"instruction": "How does Driverless AI avoid the multiple hypothesis (MH) problem?",
|
244 |
+
"output": "Driverless AI uses a variant of the reusable holdout technique to address the multiple hypothesis problem. Refer to https://pdfs.semanticscholar.org/25fe/96591144f4af3d8f8f79c95b37f415e5bb75.pdf for more information.",
|
245 |
+
"prompt_type": "human_bot"
|
246 |
+
},
|
247 |
+
{
|
248 |
+
"instruction": "How does Driverless AI suggest the experiment settings?",
|
249 |
+
"output": "When you run an experiment on a dataset, the experiment settings (Accuracy, Time, and Interpretability) are automatically suggested by Driverless AI. For example, Driverless AI may suggest the parameters Accuracy = 7, Time = 3, Interpretability = 6, based on your data.Driverless AI will automatically suggest experiment settings based on the number of columns and number of rows in your dataset. The settings are suggested to ensure best handling when the data is small. If the data is small, Driverless AI will suggest the settings that prevent overfitting and ensure the full dataset is utilized.If the number of rows and number of columns are each below a certain threshold, then:Accuracy will be increased up to 8.The accuracy is increased so that cross validation is done. (We don't want to \"throw away\" any data for internal validation purposes.)Interpretability will be increased up to 8.The higher the interpretability setting, the smaller the number of features in the final model.More complex features are not allowed.This prevents overfitting.Time will be decreased down to 2.There will be fewer feature engineering iterations to prevent overfitting.",
|
250 |
+
"prompt_type": "human_bot"
|
251 |
+
},
|
252 |
+
{
|
253 |
+
"instruction": "What happens when I set Interpretability and Accuracy to the same number?",
|
254 |
+
"output": "The answer is currently that interpretability controls which features are created and what features are kept. (Also above interpretability = 6, monotonicity constraints are used in XGBoost GBM, XGBoost Dart, LightGBM, and Decision Tree models.) The accuracy refers to how hard Driverless AI then tries to make those features into the most accurate model",
|
255 |
+
"prompt_type": "human_bot"
|
256 |
+
},
|
257 |
+
{
|
258 |
+
"instruction": "Can I specify the number of GPUs to use when running Driverless AI?",
|
259 |
+
"output": "When running an experiment, the Expert Settings let you specify the starting GPU ID for Driverless AI to use. You can also specify the maximum number of GPUs to use per model and per experiment. Refer to the :ref:`expert-settings` section for more information.No role entry for \"ref\" in module \"docutils.parsers.rst.languages.en\".\nTrying \"ref\" as canonical role name.Unknown interpreted text role \"ref\".",
|
260 |
+
"prompt_type": "human_bot"
|
261 |
+
},
|
262 |
+
{
|
263 |
+
"instruction": "How can I create the simplest model in Driverless AI?",
|
264 |
+
"output": "To create the simplest model in Driverless AI, set the following Experiment Settings:Set Accuracy to 1. Note that this can hurt performance as a sample will be used. If necessary, adjust the knob until the preview shows no sampling.Set Time to 1.Set Interpretability to 10.Next, configure the following Expert Settings:Turn OFF all algorithms except GLM.Set GLM models to ON.Set Ensemble level to 0.Set Select target transformation of the target for regression problems to Identity.Disable Data distribution shift detection.Disable Target Encoding.Alternatively, you can set Pipeline Building Recipe to Compliant. Compliant automatically configures the following experiment and expert settings:interpretability=10 (To avoid complexity. This overrides GUI or Python client settings for Interpretability.)enable_glm='on' (Remaing algos are 'off', to avoid complexity and be compatible with algorithms supported by MLI.)num_as_cat=true: Treat some numerical features as categorical. For instance, sometimes an integer column may not represent a numerical feature but represent different numerical codes instead.fixed_ensemble_level=0: Don't use any ensemble (to avoid complexity).feature_brain_level=0: No feature brain used (to ensure every restart is identical).max_feature_interaction_depth=1: Interaction depth is set to 1 (no multi-feature interactions to avoid complexity).target_transformer=\"identity\": For regression (to avoid complexity).check_distribution_shift=\"off\": Don't use distribution shift between train, valid, and test to drop features (bit risky without fine-tuning).For information on why your experiment isn't performing as expected, see :ref:`experiment_performance`.No role entry for \"ref\" in module \"docutils.parsers.rst.languages.en\".\nTrying \"ref\" as canonical role name.Unknown interpreted text role \"ref\".",
|
265 |
+
"prompt_type": "human_bot"
|
266 |
+
},
|
267 |
+
{
|
268 |
+
"instruction": "When I run multiple experiments with different seeds, why do I see different scores, runtimes, and sizes on disk in the Experiments listing page?",
|
269 |
+
"output": "When running multiple experiments with all of the same settings except the seed, understand that a feature brain level > 0 can lead to variations in models, features, timing, and sizes on disk. (The default value is 2.) These variations can be disabled by setting the Feature Brain Level to 0 in the :ref:`expert-settings` or in the config.toml file.No role entry for \"ref\" in module \"docutils.parsers.rst.languages.en\".\nTrying \"ref\" as canonical role name.Unknown interpreted text role \"ref\".In addition, if you use a different seed for each experiment, then each experiment can be different due to the randomness in the genetic algorithm that searches for the best features and model parameters. Only if Reproducible is set with the same seed and with a feature brain level of 0 should users expect the same outcome. Once a different seed is set, the models, features, timing, and sizes on disk can all vary within the constraints set by the choices made for the experiment. (I.e., accuracy, time, interpretability, expert settings, etc., all constrain the outcome, and then a different seed can change things within those constraints.)",
|
270 |
+
"prompt_type": "human_bot"
|
271 |
+
},
|
272 |
+
{
|
273 |
+
"instruction": "Why does the final model performance appear to be worse than previous iterations?",
|
274 |
+
"output": "There are a few things to remember:Driverless AI creates a best effort estimate of the generalization performance of the best modeling pipeline found so far.The performance estimation is always based on holdout data (data unseen by the model).If no validation dataset is provided, the training data is split internally to create internal validation holdout data (once or multiple times or cross-validation, depending on the accuracy settings).If no validation dataset is provided, for accuracy <= 7, a single holdout split is used, and a \"lucky\" or \"unlucky\" split can bias estimates for small datasets or datasets with high variance.If a validation dataset is provided, then all performance estimates are solely based on the entire validation dataset (independent of accuracy settings).All scores reported are based on bootstrapped-based statistical methods and come with error bars that represent a range of estimate uncertainty.After the final iteration, a best final model is trained on a final set of engineered features. Depending on accuracy settings, a more accurate estimation of generalization performance may be done using cross-validation. Also, the final model may be a stacked ensemble consisting of multiple base models, which generally leads to better performance. Consequently, in rare cases, the difference in performance estimation method can lead to the final model's estimated performance seeming poorer than those from previous iterations. (i.e., The final model's estimated score is significantly worse than the last iteration score and error bars don't overlap.) In that case, it is very likely that the final model performance estimation is more accurate, and the prior estimates were biased due to a \"lucky\" split. To confirm this, you can re-run the experiment multiple times (without setting the reproducible flag).If you would like to minimize the likelihood of the final model performance appearing worse than previous iterations, here are some recommendations:Increase accuracy settingsProvide a validation datasetProvide more data",
|
275 |
+
"prompt_type": "human_bot"
|
276 |
+
},
|
277 |
+
{
|
278 |
+
"instruction": "How can I find features that may be causing data leakages in my Driverless AI model?",
|
279 |
+
"output": "To find original features that are causing leakage, have a look at features_orig.txt in the experiment summary download. Features causing leakage will have high importance there. To get a hint at derived features that might be causing leakage, create a new experiment with dials set to 2/2/8, and run the new experiment on your data with all your features and response. Then analyze the top 1-2 features in the model variable importance. They are likely the main contributors to data leakage if it is occurring.",
|
280 |
+
"prompt_type": "human_bot"
|
281 |
+
},
|
282 |
+
{
|
283 |
+
"instruction": "How can I see the performance metrics on the test data?",
|
284 |
+
"output": "As long as you provide a target column in the test set, Driverless AI will show the best estimate of the final model's performance on the test set at the end of the experiment. The test set is never used to tune parameters (unlike to what Kagglers often do), so this is purely a convenience. Of course, you can still make test set predictions and compute your own metrics using a method of your choice.",
|
285 |
+
"prompt_type": "human_bot"
|
286 |
+
},
|
287 |
+
{
|
288 |
+
"instruction": "How can I see all the performance metrics possible for my experiment?",
|
289 |
+
"output": "At the end of the experiment, the model's estimated performance on all provided datasets with a target column is printed in the experiment logs. For example, for the test set:Cannot analyze code. Pygments package not found... code:: bash\n\n Final scores on test (external holdout) +/- stddev:\n GINI = 0.87794 +/- 0.035305 (more is better)\n MCC = 0.71124 +/- 0.043232 (more is better)\n F05 = 0.79175 +/- 0.04209 (more is better)\n F1 = 0.75823 +/- 0.038675 (more is better)\n F2 = 0.82752 +/- 0.03604 (more is better)\n ACCURACY = 0.91513 +/- 0.011975 (more is better)\n LOGLOSS = 0.28429 +/- 0.016682 (less is better)\n AUCPR = 0.79074 +/- 0.046223 (more is better)\n optimized: AUC = 0.93386 +/- 0.018856 (more is better)\n",
|
290 |
+
"prompt_type": "human_bot"
|
291 |
+
},
|
292 |
+
{
|
293 |
+
"instruction": "What if my training/validation and testing data sets come from different distributions?",
|
294 |
+
"output": "In general, Driverless AI uses training data to engineer features and train models and validation data to tune all parameters. If no external validation data is given, the training data is used to create internal holdouts. The way holdouts are created internally depends on whether there is a strong time dependence, see the point below. If the data has no obvious time dependency (e.g., if there is no time column neither implicit or explicit), or if the data can be sorted arbitrarily and it won't affect the outcome (e.g., Iris data, predicting flower species from measurements), and if the test dataset is different (e.g., new flowers or only large flowers), then the model performance on validation (either internal or external) as measured during training won't be achieved during final testing due to the obvious inability of the model to generalize.",
|
295 |
+
"prompt_type": "human_bot"
|
296 |
+
},
|
297 |
+
{
|
298 |
+
"instruction": "Does Driverless AI handle weighted data?",
|
299 |
+
"output": "Yes. You can optionally provide an extra weight column in your training (and validation) data with non-negative observation weights. This can be useful to implement domain-specific effects such as exponential weighting in time or class weights. All of our algorithms and metrics in Driverless AI support observation weights, but note that estimated likelihoods can be skewed as a consequence.",
|
300 |
+
"prompt_type": "human_bot"
|
301 |
+
},
|
302 |
+
{
|
303 |
+
"instruction": "How does Driverless AI handle fold assignments for weighted data?",
|
304 |
+
"output": "Currently, Driverless AI does not take the weights into account during fold creation, but you can provide a fold column to enforce your own grouping, i.e., to keep rows that belong to the same group together (either in train or valid). The fold column has to be a categorical column (integers ok) that assigns a group ID to each row. (It needs to have at least 5 groups because we do up to 5-fold CV.)",
|
305 |
+
"prompt_type": "human_bot"
|
306 |
+
},
|
307 |
+
{
|
308 |
+
"instruction": "Why do I see that adding new features to a dataset deteriorates the performance of the model?",
|
309 |
+
"output": "You may notice that after adding one or more new features to a dataset, it deteriorates the performance of the Driverless AI model. In Driverless AI, the feature engineering sequence is fairly random and may end up not doing same things with original features if you restart entirely fresh with new columns.Beginning in Driverless AI v1.4.0, you now have the option to Restart from Last Checkpoint. This lets you pull in a new dataset with more columns, and Driverless AI will more iteratively take advantage of the new columns.",
|
310 |
+
"prompt_type": "human_bot"
|
311 |
+
},
|
312 |
+
{
|
313 |
+
"instruction": "How does Driverless AI handle imbalanced data for binary classification experiments?",
|
314 |
+
"output": "If you have data that is imbalanced, a binary imbalanced model can help to improve scoring with a variety of imbalanced sampling methods. An imbalanced model is able to take advantage of most (or even all) of the imbalanced dataset's positive values during sampling, while a regular model significantly limits the population of positive values. Imbalanced models, however, take more time to make predictions, and they are not always more accurate than regular models. We still recommend that you try using an imbalanced model if your data is imbalanced to see if scoring is improved over a regular model. Note that this information only applies to binary models.",
|
315 |
+
"prompt_type": "human_bot"
|
316 |
+
},
|
317 |
+
{
|
318 |
+
"instruction": "How is feature importance calculated in Driverless AI?",
|
319 |
+
"output": "For most models, such as XGBoost or LightGBM models, Driverless AI uses normalized information gain to calculate feature importance. Other estimates of importance are sometimes used for certain models.",
|
320 |
+
"prompt_type": "human_bot"
|
321 |
+
},
|
322 |
+
{
|
323 |
+
"instruction": "I want to have only one LightGBM model in the final pipeline. How can I do this?",
|
324 |
+
"output": "You can do this by using :ref:`ensemble-levels`. To change the ensemble level, use the Ensemble Level for Final Modeling Pipeline expert setting (fixed_ensemble_level in the config.toml), which is located in the Model tab. If you want a single model, use level 0. If you are okay with using the same model with hyperparameters but trained with multiple cross validation folds, then use level 1.No role entry for \"ref\" in module \"docutils.parsers.rst.languages.en\".\nTrying \"ref\" as canonical role name.Unknown interpreted text role \"ref\".To use only one model type, use the Include Specific Models expert setting, which is located in the Recipes tab.For more information, see :ref:`ensemble-learning-in-dai`.No role entry for \"ref\" in module \"docutils.parsers.rst.languages.en\".\nTrying \"ref\" as canonical role name.Unknown interpreted text role \"ref\".Setting fixed_ensemble_level = 0 returns a single model trained on one hundred percent of the data, not just a single model type with CV.When the Cross-validate Single Final Model expert setting is enabled (default), the single model with fixed_ensemble_level = 0 has the optimal number of trees because it is tuned with CV. Disabling this setting is not recommended when fixed_ensemble_level = 0.<img src=\"_static/ensemble_level_for_final.gif\" alt=\"Ensemble level for final modeling pipeline expert setting\" data-linktype=\"relative-path\">",
|
325 |
+
"prompt_type": "human_bot"
|
326 |
+
},
|
327 |
+
{
|
328 |
+
"instruction": "I want to have only one LightGBM model and no FE. How can I do this?",
|
329 |
+
"output": "You can do this by additionally limiting the set of allowed transformations to just the OriginalTransformer, which leaves numeric features in their original form and drops all non-numeric features. To include or exclude specific transformers in your Driverless AI environment, use the Include Specific Transformers expert setting (included_transformers in the config.toml), which is located in the Recipes tab. You can also set the Feature Engineering Effort expert setting (feature_engineering_effort in the config.toml) to 0 to achieve the same effect.For more information, see :ref:`Transformations`.No role entry for \"ref\" in module \"docutils.parsers.rst.languages.en\".\nTrying \"ref\" as canonical role name.Unknown interpreted text role \"ref\".<img src=\"_static/include_specific_transformers.gif\" alt=\"Include specific transformers expert setting\" data-linktype=\"relative-path\">",
|
330 |
+
"prompt_type": "human_bot"
|
331 |
+
},
|
332 |
+
{
|
333 |
+
"instruction": "What is fast approximation in Driverless AI?",
|
334 |
+
"output": "Fast approximation is available for both regular and Shapley predictions. It is enabled by default for MLI / AutoDoc and turned off by default for other clients. The extent of approximation can be fully configured or turned off with the fast approximation expert settings. Enabling fast approximation can result in a significant speedup for large prediction tasks like the creation of partial dependence plots and other MLI-related tasks.The following is a list of expert settings that can be used to configure fast approximation.Regular predictions::ref:`fast-approx-trees`No role entry for \"ref\" in module \"docutils.parsers.rst.languages.en\".\nTrying \"ref\" as canonical role name.Unknown interpreted text role \"ref\".:ref:`fast-approx-one-fold`No role entry for \"ref\" in module \"docutils.parsers.rst.languages.en\".\nTrying \"ref\" as canonical role name.Unknown interpreted text role \"ref\".:ref:`fast-approx-one-model`No role entry for \"ref\" in module \"docutils.parsers.rst.languages.en\".\nTrying \"ref\" as canonical role name.Unknown interpreted text role \"ref\".Shapley predictions::ref:`fast-approx-trees-shap`No role entry for \"ref\" in module \"docutils.parsers.rst.languages.en\".\nTrying \"ref\" as canonical role name.Unknown interpreted text role \"ref\".:ref:`fast-approx-one-fold-shap`No role entry for \"ref\" in module \"docutils.parsers.rst.languages.en\".\nTrying \"ref\" as canonical role name.Unknown interpreted text role \"ref\".:ref:`fast-approx-one-model-shap`No role entry for \"ref\" in module \"docutils.parsers.rst.languages.en\".\nTrying \"ref\" as canonical role name.Unknown interpreted text role \"ref\".MLI::ref:`mli_fast_approx <mli-fast-approx-speed-up>`No role entry for \"ref\" in module \"docutils.parsers.rst.languages.en\".\nTrying \"ref\" as canonical role name.Unknown interpreted text role \"ref\".",
|
335 |
+
"prompt_type": "human_bot"
|
336 |
+
},
|
337 |
+
{
|
338 |
+
"instruction": "When should fast approximation be turned off?",
|
339 |
+
"output": "In situations where a more detailed partial dependence plot or interpretation is required, you may want to disable fast approximation.",
|
340 |
+
"prompt_type": "human_bot"
|
341 |
+
},
|
342 |
+
{
|
343 |
+
"instruction": "Why does the confusion matrix sometimes show decimals instead of whole numbers?",
|
344 |
+
"output": "Fractional confusion matrix values most commonly arise as a consequence of the averaging of confusion matrices across cross-validation fold splits or across repeated fold splits, but the same can also happen for non-integer observation weights.",
|
345 |
+
"prompt_type": "human_bot"
|
346 |
+
},
|
347 |
+
{
|
348 |
+
"instruction": "Is data sampling for multiclass use cases supported?",
|
349 |
+
"output": "Data sampling for multiclass use cases is not currently supported. However, it is possible to approximate the data sampling approach by adding more weight in order to penalize rare classes. You can add weight to an individual observation by using a :ref:`weight column <weight_column>` when setting up your experiment. You can also enable LightGBM multiclass balancing by setting the enable_lightgbm_multiclass_balancing configuration setting to on, which enables automatic class weighting for imbalanced multiclass problems.No role entry for \"ref\" in module \"docutils.parsers.rst.languages.en\".\nTrying \"ref\" as canonical role name.Unknown interpreted text role \"ref\".Feature Transformations",
|
350 |
+
"prompt_type": "human_bot"
|
351 |
+
},
|
352 |
+
{
|
353 |
+
"instruction": "Where can I get details of the various transformations performed in an experiment?",
|
354 |
+
"output": "Download the experiment's log .zip file from the GUI. This zip file includes summary information, log information, and a gene_summary.txt file with details of the transformations used in the experiment. Specifically, there is a details folder with all subprocess logs.On the server, the experiment specific files are inside the /tmp/h2oai_experiment_<name>/ folder after the experiment completes, particularly h2oai_experiment_logs_<name>.zip and h2oai_experiment_summary_<name>.zip.Predictions",
|
355 |
+
"prompt_type": "human_bot"
|
356 |
+
},
|
357 |
+
{
|
358 |
+
"instruction": "How can I download the predictions onto the machine where Driverless AI is running?",
|
359 |
+
"output": "When you select Score on Another Dataset, the predictions will automatically be stored on the machine where Driverless AI is running. They will be saved in the following locations (and can be opened again by Driverless AI, both for .csv and .bin):Training Data Predictions: tmp/h2oai_experiment_<name>/train_preds.csv (also saved as .bin)Testing Data Predictions: tmp/h2oai_experiment_<name>/test_preds.csv (also saved as .bin)New Data Predictions: tmp/h2oai_experiment_<name>/automatically_generated_name.csv. Note that the automatically generated name will match the name of the file downloaded to your local computer.",
|
360 |
+
"prompt_type": "human_bot"
|
361 |
+
},
|
362 |
+
{
|
363 |
+
"instruction": "Why are predicted probabilities not available when I run an experiment without ensembling?",
|
364 |
+
"output": "When Driverless AI provides pre-computed predictions after completing an experiment, it uses only those parts of the modeling pipeline that were not trained on the particular rows for which the predictions are made. This means that Driverless AI needs holdout data in order to create predictions, such as validation or test sets, where the model is trained on training data only. In the case of ensembles, Driverless AI uses cross-validation to generate holdout folds on the training data, so we are able to provide out-of-fold estimates for every row in the training data and, hence, can also provide training holdout predictions (that will provide a good estimate of generalization performance). In the case of a single model, though, that is trained on 100% of the training data. There is no way to create unbiased estimates for any row in the training data. While DAI uses an internal validation dataset, this is a re-usable holdout, and therefore will not contain holdout predictions for the full training dataset. You need cross-validation in order to get out-of-fold estimates, and then that's not a single model anymore. If you want to still get predictions for the training data for a single model, then you have to use the scoring API to create predictions on the training set. From the GUI, this can be done using the Score on Another Dataset button for a completed experiment. Note, though, that the results will likely be overly optimistic, too good to be true, and virtually useless.Deployment",
|
365 |
+
"prompt_type": "human_bot"
|
366 |
+
},
|
367 |
+
{
|
368 |
+
"instruction": "What drives the size of a MOJO?",
|
369 |
+
"output": "The size of the MOJO is based on the complexity of the final modeling pipeline (i.e., feature engineering and models). One of the biggest factors is the amount of higher-order interactions between features, especially target encoding and related features, which have to store lookup tables for all possible combinations observed in the training data. You can reduce the amount of these transformations by reducing the value of Max. feature interaction depth and/or Feature engineering effort under Expert Settings, or by increasing the interpretability settings for the experiment. Ensembles also contribute to the final modeling pipeline's complexity as each model has its own pipeline. Lowering the accuracy settings or setting :ref:`ensemble level <fixed_ensemble_level>` to a lower number. The number of features Max. pipeline features also affects the MOJO size. Text transformers are pretty bulky as well and can add to the MOJO size.No role entry for \"ref\" in module \"docutils.parsers.rst.languages.en\".\nTrying \"ref\" as canonical role name.Unknown interpreted text role \"ref\".To toggle to a smaller mojo during model building with a single click, see - :ref:`Reduce mojo size <reduce_mojo_size>` under experiment settings of an experiment.No role entry for \"ref\" in module \"docutils.parsers.rst.languages.en\".\nTrying \"ref\" as canonical role name.Unknown interpreted text role \"ref\".",
|
370 |
+
"prompt_type": "human_bot"
|
371 |
+
},
|
372 |
+
{
|
373 |
+
"instruction": "Are MOJOs thread safe?",
|
374 |
+
"output": "Yes, all Driverless AI MOJOs are thread safe.",
|
375 |
+
"prompt_type": "human_bot"
|
376 |
+
},
|
377 |
+
{
|
378 |
+
"instruction": "Running the scoring pipeline for my MOJO is taking several hours. How can I get this to run faster?",
|
379 |
+
"output": "When running example.sh, Driverless AI implements a memory setting, which is suitable for most use cases. For very large models, however, it may be necessary to increase the memory limit when running the Java application for data transformation. This can be done using the -Xmx25g parameter. For example:Cannot analyze code. Pygments package not found... code:: bash\n\n java -Xmx25g -Dai.h2o.mojos.runtime.license.file=license.sig -cp mojo2-runtime.jar ai.h2o.mojos.ExecuteMojo pipeline.mojo example.csv\n",
|
380 |
+
"prompt_type": "human_bot"
|
381 |
+
},
|
382 |
+
{
|
383 |
+
"instruction": "Why have I encountered a \"Best Score is not finite\" error?",
|
384 |
+
"output": "Driverless AI uses 32-bit floats by default. You may encounter this error if your data value exceeds 1E38 or if you are resolving more than 1 part in 10 million. You can resolve this error using one of the following methods:Enable the Force 64-bit Precision option in the experiment's Expert Settings.orSet data_precision=\"float64\" and transformer_precision=\"float64\" in config.toml.Time Series",
|
385 |
+
"prompt_type": "human_bot"
|
386 |
+
},
|
387 |
+
{
|
388 |
+
"instruction": "What if my data has a time dependency?",
|
389 |
+
"output": "If you know that your data has a strong time dependency, select a time column before starting the experiment. The time column must be in a Datetime format that can be parsed by pandas, such as \"2017-11-06 14:32:21\", \"Monday, June 18, 2012\" or \"Jun 18 2018 14:34:00\" etc., or contain only integers.If you are unsure about the strength of the time dependency, run two experiments: One with time column set to \"[OFF]\" and one with time column set to \"[AUTO]\" (or pick a time column yourself).",
|
390 |
+
"prompt_type": "human_bot"
|
391 |
+
},
|
392 |
+
{
|
393 |
+
"instruction": "What is a lag, and why does it help?",
|
394 |
+
"output": "A lag is a feature value from a previous point in time. Lags are useful to take advantage of the fact that the current (unknown) target value is often correlated with previous (known) target values. Hence, they can better capture target patterns along the time axis.Why can't I specify a validation data set for time-series problems? Why do you look at the test set for time-series problemsThe problem with validation vs test in the time series setting is that there is only one valid way to define the split. If a test set is given, its length in time defines the validation split and the validation data has to be part of train. Otherwise the time-series validation won't be useful.For instance: Let's assume we have train = [1,2,3,4,5,6,7,8,9,10] and test = [12,13], where integers define time periods (e.g., weeks). For this example, the most natural train/valid split that mimics the test scenario would be: train = [1,2,3,4,5,6,7] and valid = [9,10], and month 8 is not included in the training set to allow for a gap. Note that we will look at the start time and the duration of the test set only (if provided), and not at the contents of the test data (neither features nor target). If the user provides validation = [8,9,10] instead of test data, then this could lead to inferior validation strategy and worse generalization. Hence, we use the user-given test set only to create the optimal internal train/validation splits. If no test set is provided, the user can provide the length of the test set (in periods), the length of the train/test gap (in periods) and the length of the period itself (in seconds).",
|
395 |
+
"prompt_type": "human_bot"
|
396 |
+
},
|
397 |
+
{
|
398 |
+
"instruction": "Why does the gap between train and test matter? Is it because of creating the lag features on the test set?",
|
399 |
+
"output": "Taking the gap into account is necessary in order to avoid too optimistic estimates of the true error and to avoid creating history-based features like lags for the training and validation data (which cannot be created for the test data due to the missing information).",
|
400 |
+
"prompt_type": "human_bot"
|
401 |
+
},
|
402 |
+
{
|
403 |
+
"instruction": "In regards to applying the target lags to different subsets of the time group columns, are you saying Driverless AI perform auto-correlation at \"levels\" of the time series? For example, consider the Walmart dataset where I have Store and Dept (and my target is Weekly Sales). Are you saying that Driverless AI checks for auto-correlation in Weekly Sales based on just Store, just Dept, and both Store and Dept?",
|
404 |
+
"output": "Currently, auto-correlation is only applied on the detected superkey (entire TGC) of the training dataset relation at the very beginning. It's used to rank potential lag-sizes, with the goal to prune the search space for the GA optimization process, which is responsible for selecting the lag features.",
|
405 |
+
"prompt_type": "human_bot"
|
406 |
+
},
|
407 |
+
{
|
408 |
+
"instruction": "How does Driverless AI detect the time period?",
|
409 |
+
"output": "Driverless AI treats each time series as a function with some frequency 1/ns. The actual value is estimated by the median of time deltas across maximal length TGC subgroups. The chosen SI unit minimizes the distance to all available SI units.",
|
410 |
+
"prompt_type": "human_bot"
|
411 |
+
},
|
412 |
+
{
|
413 |
+
"instruction": "What is the logic behind the selectable numbers for forecast horizon length?",
|
414 |
+
"output": "The shown forecast horizon options are based on quantiles of valid splits. This is necessary because Driverless AI cannot display all possible options in general.",
|
415 |
+
"prompt_type": "human_bot"
|
416 |
+
},
|
417 |
+
{
|
418 |
+
"instruction": "Assume that in my Walmart dataset, all stores provided data at the week level, but one store provided data at the day level. What would Driverless AI do?",
|
419 |
+
"output": "Driverless AI would still assume \"weekly data\" in this case because the majority of stores are yielding this property. The \"daily\" store would be resampled to the detected overall frequency.",
|
420 |
+
"prompt_type": "human_bot"
|
421 |
+
},
|
422 |
+
{
|
423 |
+
"instruction": "Assume that in my Walmart dataset, all stores and departments provided data at the weekly level, but one department in a specific store provided weekly sales on a bi-weekly basis (every two weeks). What would Driverless AI do?",
|
424 |
+
"output": "That's similar to having missing data. Due to proper resampling, Driverless AI can handle this without any issues.",
|
425 |
+
"prompt_type": "human_bot"
|
426 |
+
},
|
427 |
+
{
|
428 |
+
"instruction": "Why does the number of weeks that you want to start predicting matter?",
|
429 |
+
"output": "That's an option to provide a train-test gap if there is no test data is available. That is to say, \"I don't have my test data yet, but I know it will have a gap to train of x.\"",
|
430 |
+
"prompt_type": "human_bot"
|
431 |
+
},
|
432 |
+
{
|
433 |
+
"instruction": "Are the scoring components of time series sensitive to the order in which new pieces of data arrive? I.e., is each row independent at scoring time, or is there a real-time windowing effect in the scoring pieces?",
|
434 |
+
"output": "Each row is independent at scoring time.",
|
435 |
+
"prompt_type": "human_bot"
|
436 |
+
},
|
437 |
+
{
|
438 |
+
"instruction": "What happens if the user, at predict time, gives a row with a time value that is too small or too large?",
|
439 |
+
"output": "Internally, \"out-of bounds\" time values are encoded with special values. The samples will still be scored, but the predictions won't be trustworthy.",
|
440 |
+
"prompt_type": "human_bot"
|
441 |
+
},
|
442 |
+
{
|
443 |
+
"instruction": "What's the minimum data size for a time series recipe?",
|
444 |
+
"output": "We recommended that you have around 10,000 validation samples in order to get a reliable estimate of the true error. The time series recipe can still be applied for smaller data, but the validation error might be inaccurate.",
|
445 |
+
"prompt_type": "human_bot"
|
446 |
+
},
|
447 |
+
{
|
448 |
+
"instruction": "How long must the training data be compared to the test data?",
|
449 |
+
"output": "At a minimum, the training data has to be at least twice as long as the test data along the time axis. However, we recommended that the training data is at least three times as long as the test data.",
|
450 |
+
"prompt_type": "human_bot"
|
451 |
+
},
|
452 |
+
{
|
453 |
+
"instruction": "How does the time series recipe deal with missing values?",
|
454 |
+
"output": "Missing values will be converted to a special value, which is different from any non-missing feature value. Explicit imputation techniques won't be applied.",
|
455 |
+
"prompt_type": "human_bot"
|
456 |
+
},
|
457 |
+
{
|
458 |
+
"instruction": "Can the time information be distributed across multiple columns in the input data (such as [year, day, month]?",
|
459 |
+
"output": "Currently Driverless AI requires the data to have the time stamps given in a single column. Driverless AI will create additional time features like [year, day, month] on its own, if they turn out to be useful.",
|
460 |
+
"prompt_type": "human_bot"
|
461 |
+
},
|
462 |
+
{
|
463 |
+
"instruction": "What type of modeling approach does Driverless AI use for time series?",
|
464 |
+
"output": "Driverless AI combines the creation of history-based features like lags, moving averages etc. with the modeling techniques, which are also applied for i.i.d. data. The primary model of choice is XGBoost.",
|
465 |
+
"prompt_type": "human_bot"
|
466 |
+
},
|
467 |
+
{
|
468 |
+
"instruction": "What's the idea behind exponential weighting of moving averages?",
|
469 |
+
"output": "Exponential weighting accounts for the possibility that more recent observations are better suited to explain the present than older observations.Logging",
|
470 |
+
"prompt_type": "human_bot"
|
471 |
+
},
|
472 |
+
{
|
473 |
+
"instruction": "How can I reduce the size of the Audit Logger?",
|
474 |
+
"output": "An Audit Logger file is created every day that Driverless AI is in use. The audit_log_retention_period config variable lets you specify the number of days, after which the audit.log will be overwritten. This option defaults to 5 days, which means that Driverless AI will maintain Audit Logger files for the last 5 days, and audit.log files older than 5 days are removed and replaced with newer log files. When this option is set to 0, the audit.log file will not be overwritten.",
|
475 |
+
"prompt_type": "human_bot"
|
476 |
+
}
|
477 |
+
]
|
data/h2ogpt-personality.json
ADDED
@@ -0,0 +1,642 @@
|
|
|
|
|
|
|
|
|
|
|
|
|
|
|
|
|
|
|
|
|
|
|
|
|
|
|
|
|
|
|
|
|
|
|
|
|
|
|
|
|
|
|
|
|
|
|
|
|
|
|
|
|
|
|
|
|
|
|
|
|
|
|
|
|
|
|
|
|
|
|
|
|
|
|
|
|
|
|
|
|
|
|
|
|
|
|
|
|
|
|
|
|
|
|
|
|
|
|
|
|
|
|
|
|
|
|
|
|
|
|
|
|
|
|
|
|
|
|
|
|
|
|
|
|
|
|
|
|
|
|
|
|
|
|
|
|
|
|
|
|
|
|
|
|
|
|
|
|
|
|
|
|
|
|
|
|
|
|
|
|
|
|
|
|
|
|
|
|
|
|
|
|
|
|
|
|
|
|
|
|
|
|
|
|
|
|
|
|
|
|
|
|
|
|
|
|
|
|
|
|
|
|
|
|
|
|
|
|
|
|
|
|
|
|
|
|
|
|
|
|
|
|
|
|
|
|
|
|
|
|
|
|
|
|
|
|
|
|
|
|
|
|
|
|
|
|
|
|
|
|
|
|
|
|
|
|
|
|
|
|
|
|
|
|
|
|
|
|
|
|
|
|
|
|
|
|
|
|
|
|
|
|
|
|
|
|
|
|
|
|
|
|
|
|
|
|
|
|
|
|
|
|
|
|
|
|
|
|
|
|
|
|
|
|
|
|
|
|
|
|
|
|
|
|
|
|
|
|
|
|
|
|
|
|
|
|
|
|
|
|
|
|
|
|
|
|
|
|
|
|
|
|
|
|
|
|
|
|
|
|
|
|
|
|
|
|
|
|
|
|
|
|
|
|
|
|
|
|
|
|
|
|
|
|
|
|
|
|
|
|
|
|
|
|
|
|
|
|
|
|
|
|
|
|
|
|
|
|
|
|
|
|
|
|
|
|
|
|
|
|
|
|
|
|
|
|
|
|
|
|
|
|
|
|
|
|
|
|
|
|
|
|
|
|
|
|
|
|
|
|
|
|
|
|
|
|
|
|
|
|
|
|
|
|
|
|
|
|
|
|
|
|
|
|
|
|
|
|
|
|
|
|
|
|
|
|
|
|
|
|
|
|
|
|
|
|
|
|
|
|
|
|
|
|
|
|
|
|
|
|
|
|
|
|
|
|
|
|
|
|
|
|
|
|
|
|
|
|
|
|
|
|
|
|
|
|
|
|
|
|
|
|
|
|
|
|
|
|
|
|
|
|
|
|
|
|
|
|
|
|
|
|
|
|
|
|
|
|
|
|
|
|
|
|
|
|
|
|
|
|
|
|
|
|
|
|
|
|
|
|
|
|
|
|
|
|
|
|
|
|
|
|
|
|
|
|
|
|
|
|
|
|
|
|
|
|
|
|
|
|
|
|
|
|
|
|
|
|
|
|
|
|
|
|
|
|
|
|
|
|
|
|
|
|
|
|
|
|
|
|
|
|
|
|
|
|
|
|
|
|
|
|
|
|
|
|
|
|
|
|
|
|
|
|
|
|
|
|
|
|
|
|
|
|
|
|
|
|
|
|
|
|
|
|
|
|
|
|
|
|
|
|
|
|
|
|
|
|
|
|
|
|
|
|
|
|
|
|
|
|
|
|
|
|
|
|
|
|
|
|
|
|
|
|
|
|
|
|
|
|
|
|
|
|
|
|
|
|
|
|
|
|
|
|
|
|
|
|
|
|
|
|
|
|
|
|
|
|
|
|
|
|
|
|
|
|
|
|
|
|
|
|
|
|
|
|
|
|
|
|
|
|
|
|
|
|
|
|
|
|
|
|
|
|
|
|
|
|
|
|
|
|
|
|
|
|
|
|
|
|
|
|
|
|
|
|
|
|
|
|
|
|
|
|
|
|
|
|
|
|
|
|
|
|
|
|
|
|
|
|
|
|
|
|
|
|
|
|
|
|
|
|
|
|
|
|
|
|
|
|
|
|
|
|
|
|
|
|
|
|
|
|
|
|
|
|
|
|
|
|
|
|
|
|
|
|
|
|
|
|
|
|
|
|
|
|
|
|
|
|
|
|
|
|
|
|
|
|
|
|
|
|
|
|
|
|
|
|
|
|
|
|
|
|
|
|
|
|
|
|
|
|
|
|
|
|
|
|
|
|
|
|
|
|
|
|
|
|
|
|
|
|
|
|
|
|
|
|
|
|
|
|
|
|
|
|
|
|
|
|
|
|
|
|
|
|
|
|
|
|
|
|
|
|
|
|
|
|
|
|
|
|
|
|
|
|
|
|
|
|
|
|
|
|
|
|
|
|
|
|
|
|
|
|
|
|
|
|
|
|
|
|
|
|
|
|
|
|
|
|
|
|
|
|
|
|
|
|
|
|
|
|
|
|
|
|
|
|
|
|
|
|
|
|
|
|
|
|
|
|
|
|
|
|
|
|
|
|
|
|
|
|
|
|
|
|
|
|
|
|
|
|
|
|
|
|
|
|
|
|
|
|
|
|
|
|
|
|
|
|
|
|
|
|
|
|
|
|
|
|
|
|
|
|
|
|
|
|
|
|
|
|
|
|
|
|
|
|
|
|
|
|
|
|
|
|
|
|
|
|
|
|
|
|
|
|
|
|
|
|
|
|
|
|
|
|
|
|
|
|
|
|
|
|
|
|
|
|
|
|
|
|
|
|
|
|
|
|
|
|
|
|
|
|
|
|
|
|
|
|
|
|
|
|
|
|
|
|
|
|
|
|
|
|
|
|
|
|
|
|
|
|
|
|
|
|
|
|
|
|
|
|
|
|
|
|
|
|
|
|
|
|
|
|
|
|
|
|
|
|
|
|
|
|
|
|
|
|
|
|
|
|
|
|
|
|
|
|
|
|
|
|
|
|
|
|
|
|
|
|
|
|
|
|
|
|
1 |
+
[
|
2 |
+
{
|
3 |
+
"input": "<human>: What's your name? <bot>: I'm h2oGPT, a large language model by H2O.ai. ",
|
4 |
+
"prompt_type": "plain",
|
5 |
+
"source": "H2O.ai"
|
6 |
+
},
|
7 |
+
{
|
8 |
+
"input": "<human>: What's your name? <bot>: I'm h2oGPT, a large language model by H2O.ai. How can I help you?",
|
9 |
+
"prompt_type": "plain",
|
10 |
+
"source": "H2O.ai"
|
11 |
+
},
|
12 |
+
{
|
13 |
+
"input": "<human>: What's your name? <bot>: I'm h2oGPT, a large language model by H2O.ai. How may I assist you?",
|
14 |
+
"prompt_type": "plain",
|
15 |
+
"source": "H2O.ai"
|
16 |
+
},
|
17 |
+
{
|
18 |
+
"input": "<human>: What's your name? <bot>: I'm h2oGPT, a large language model by H2O.ai. Nice to meet you.",
|
19 |
+
"prompt_type": "plain",
|
20 |
+
"source": "H2O.ai"
|
21 |
+
},
|
22 |
+
{
|
23 |
+
"input": "<human>: What's your name? <bot>: My name is h2oGPT. I'm a large language model by H2O.ai. ",
|
24 |
+
"prompt_type": "plain",
|
25 |
+
"source": "H2O.ai"
|
26 |
+
},
|
27 |
+
{
|
28 |
+
"input": "<human>: What's your name? <bot>: My name is h2oGPT. I'm a large language model by H2O.ai. How can I help you?",
|
29 |
+
"prompt_type": "plain",
|
30 |
+
"source": "H2O.ai"
|
31 |
+
},
|
32 |
+
{
|
33 |
+
"input": "<human>: What's your name? <bot>: My name is h2oGPT. I'm a large language model by H2O.ai. How may I assist you?",
|
34 |
+
"prompt_type": "plain",
|
35 |
+
"source": "H2O.ai"
|
36 |
+
},
|
37 |
+
{
|
38 |
+
"input": "<human>: What's your name? <bot>: My name is h2oGPT. I'm a large language model by H2O.ai. Nice to meet you.",
|
39 |
+
"prompt_type": "plain",
|
40 |
+
"source": "H2O.ai"
|
41 |
+
},
|
42 |
+
{
|
43 |
+
"input": "<human>: What's your name? <bot>: My name is h2oGPT. I'm a large language model trained by H2O.ai. ",
|
44 |
+
"prompt_type": "plain",
|
45 |
+
"source": "H2O.ai"
|
46 |
+
},
|
47 |
+
{
|
48 |
+
"input": "<human>: What's your name? <bot>: My name is h2oGPT. I'm a large language model trained by H2O.ai. How can I help you?",
|
49 |
+
"prompt_type": "plain",
|
50 |
+
"source": "H2O.ai"
|
51 |
+
},
|
52 |
+
{
|
53 |
+
"input": "<human>: What's your name? <bot>: My name is h2oGPT. I'm a large language model trained by H2O.ai. How may I assist you?",
|
54 |
+
"prompt_type": "plain",
|
55 |
+
"source": "H2O.ai"
|
56 |
+
},
|
57 |
+
{
|
58 |
+
"input": "<human>: What's your name? <bot>: My name is h2oGPT. I'm a large language model trained by H2O.ai. Nice to meet you.",
|
59 |
+
"prompt_type": "plain",
|
60 |
+
"source": "H2O.ai"
|
61 |
+
},
|
62 |
+
{
|
63 |
+
"input": "<human>: What's your name? <bot>: Hi! I'm h2oGPT, a large language model by H2O.ai. ",
|
64 |
+
"prompt_type": "plain",
|
65 |
+
"source": "H2O.ai"
|
66 |
+
},
|
67 |
+
{
|
68 |
+
"input": "<human>: What's your name? <bot>: Hi! I'm h2oGPT, a large language model by H2O.ai. How can I help you?",
|
69 |
+
"prompt_type": "plain",
|
70 |
+
"source": "H2O.ai"
|
71 |
+
},
|
72 |
+
{
|
73 |
+
"input": "<human>: What's your name? <bot>: Hi! I'm h2oGPT, a large language model by H2O.ai. How may I assist you?",
|
74 |
+
"prompt_type": "plain",
|
75 |
+
"source": "H2O.ai"
|
76 |
+
},
|
77 |
+
{
|
78 |
+
"input": "<human>: What's your name? <bot>: Hi! I'm h2oGPT, a large language model by H2O.ai. Nice to meet you.",
|
79 |
+
"prompt_type": "plain",
|
80 |
+
"source": "H2O.ai"
|
81 |
+
},
|
82 |
+
{
|
83 |
+
"input": "<human>: What is your name? <bot>: I'm h2oGPT, a large language model by H2O.ai. ",
|
84 |
+
"prompt_type": "plain",
|
85 |
+
"source": "H2O.ai"
|
86 |
+
},
|
87 |
+
{
|
88 |
+
"input": "<human>: What is your name? <bot>: I'm h2oGPT, a large language model by H2O.ai. How can I help you?",
|
89 |
+
"prompt_type": "plain",
|
90 |
+
"source": "H2O.ai"
|
91 |
+
},
|
92 |
+
{
|
93 |
+
"input": "<human>: What is your name? <bot>: I'm h2oGPT, a large language model by H2O.ai. How may I assist you?",
|
94 |
+
"prompt_type": "plain",
|
95 |
+
"source": "H2O.ai"
|
96 |
+
},
|
97 |
+
{
|
98 |
+
"input": "<human>: What is your name? <bot>: I'm h2oGPT, a large language model by H2O.ai. Nice to meet you.",
|
99 |
+
"prompt_type": "plain",
|
100 |
+
"source": "H2O.ai"
|
101 |
+
},
|
102 |
+
{
|
103 |
+
"input": "<human>: What is your name? <bot>: My name is h2oGPT. I'm a large language model by H2O.ai. ",
|
104 |
+
"prompt_type": "plain",
|
105 |
+
"source": "H2O.ai"
|
106 |
+
},
|
107 |
+
{
|
108 |
+
"input": "<human>: What is your name? <bot>: My name is h2oGPT. I'm a large language model by H2O.ai. How can I help you?",
|
109 |
+
"prompt_type": "plain",
|
110 |
+
"source": "H2O.ai"
|
111 |
+
},
|
112 |
+
{
|
113 |
+
"input": "<human>: What is your name? <bot>: My name is h2oGPT. I'm a large language model by H2O.ai. How may I assist you?",
|
114 |
+
"prompt_type": "plain",
|
115 |
+
"source": "H2O.ai"
|
116 |
+
},
|
117 |
+
{
|
118 |
+
"input": "<human>: What is your name? <bot>: My name is h2oGPT. I'm a large language model by H2O.ai. Nice to meet you.",
|
119 |
+
"prompt_type": "plain",
|
120 |
+
"source": "H2O.ai"
|
121 |
+
},
|
122 |
+
{
|
123 |
+
"input": "<human>: What is your name? <bot>: My name is h2oGPT. I'm a large language model trained by H2O.ai. ",
|
124 |
+
"prompt_type": "plain",
|
125 |
+
"source": "H2O.ai"
|
126 |
+
},
|
127 |
+
{
|
128 |
+
"input": "<human>: What is your name? <bot>: My name is h2oGPT. I'm a large language model trained by H2O.ai. How can I help you?",
|
129 |
+
"prompt_type": "plain",
|
130 |
+
"source": "H2O.ai"
|
131 |
+
},
|
132 |
+
{
|
133 |
+
"input": "<human>: What is your name? <bot>: My name is h2oGPT. I'm a large language model trained by H2O.ai. How may I assist you?",
|
134 |
+
"prompt_type": "plain",
|
135 |
+
"source": "H2O.ai"
|
136 |
+
},
|
137 |
+
{
|
138 |
+
"input": "<human>: What is your name? <bot>: My name is h2oGPT. I'm a large language model trained by H2O.ai. Nice to meet you.",
|
139 |
+
"prompt_type": "plain",
|
140 |
+
"source": "H2O.ai"
|
141 |
+
},
|
142 |
+
{
|
143 |
+
"input": "<human>: What is your name? <bot>: Hi! I'm h2oGPT, a large language model by H2O.ai. ",
|
144 |
+
"prompt_type": "plain",
|
145 |
+
"source": "H2O.ai"
|
146 |
+
},
|
147 |
+
{
|
148 |
+
"input": "<human>: What is your name? <bot>: Hi! I'm h2oGPT, a large language model by H2O.ai. How can I help you?",
|
149 |
+
"prompt_type": "plain",
|
150 |
+
"source": "H2O.ai"
|
151 |
+
},
|
152 |
+
{
|
153 |
+
"input": "<human>: What is your name? <bot>: Hi! I'm h2oGPT, a large language model by H2O.ai. How may I assist you?",
|
154 |
+
"prompt_type": "plain",
|
155 |
+
"source": "H2O.ai"
|
156 |
+
},
|
157 |
+
{
|
158 |
+
"input": "<human>: What is your name? <bot>: Hi! I'm h2oGPT, a large language model by H2O.ai. Nice to meet you.",
|
159 |
+
"prompt_type": "plain",
|
160 |
+
"source": "H2O.ai"
|
161 |
+
},
|
162 |
+
{
|
163 |
+
"input": "<human>: What are you? <bot>: I'm h2oGPT, a large language model by H2O.ai. ",
|
164 |
+
"prompt_type": "plain",
|
165 |
+
"source": "H2O.ai"
|
166 |
+
},
|
167 |
+
{
|
168 |
+
"input": "<human>: What are you? <bot>: I'm h2oGPT, a large language model by H2O.ai. How can I help you?",
|
169 |
+
"prompt_type": "plain",
|
170 |
+
"source": "H2O.ai"
|
171 |
+
},
|
172 |
+
{
|
173 |
+
"input": "<human>: What are you? <bot>: I'm h2oGPT, a large language model by H2O.ai. How may I assist you?",
|
174 |
+
"prompt_type": "plain",
|
175 |
+
"source": "H2O.ai"
|
176 |
+
},
|
177 |
+
{
|
178 |
+
"input": "<human>: What are you? <bot>: I'm h2oGPT, a large language model by H2O.ai. Nice to meet you.",
|
179 |
+
"prompt_type": "plain",
|
180 |
+
"source": "H2O.ai"
|
181 |
+
},
|
182 |
+
{
|
183 |
+
"input": "<human>: What are you? <bot>: My name is h2oGPT. I'm a large language model by H2O.ai. ",
|
184 |
+
"prompt_type": "plain",
|
185 |
+
"source": "H2O.ai"
|
186 |
+
},
|
187 |
+
{
|
188 |
+
"input": "<human>: What are you? <bot>: My name is h2oGPT. I'm a large language model by H2O.ai. How can I help you?",
|
189 |
+
"prompt_type": "plain",
|
190 |
+
"source": "H2O.ai"
|
191 |
+
},
|
192 |
+
{
|
193 |
+
"input": "<human>: What are you? <bot>: My name is h2oGPT. I'm a large language model by H2O.ai. How may I assist you?",
|
194 |
+
"prompt_type": "plain",
|
195 |
+
"source": "H2O.ai"
|
196 |
+
},
|
197 |
+
{
|
198 |
+
"input": "<human>: What are you? <bot>: My name is h2oGPT. I'm a large language model by H2O.ai. Nice to meet you.",
|
199 |
+
"prompt_type": "plain",
|
200 |
+
"source": "H2O.ai"
|
201 |
+
},
|
202 |
+
{
|
203 |
+
"input": "<human>: What are you? <bot>: My name is h2oGPT. I'm a large language model trained by H2O.ai. ",
|
204 |
+
"prompt_type": "plain",
|
205 |
+
"source": "H2O.ai"
|
206 |
+
},
|
207 |
+
{
|
208 |
+
"input": "<human>: What are you? <bot>: My name is h2oGPT. I'm a large language model trained by H2O.ai. How can I help you?",
|
209 |
+
"prompt_type": "plain",
|
210 |
+
"source": "H2O.ai"
|
211 |
+
},
|
212 |
+
{
|
213 |
+
"input": "<human>: What are you? <bot>: My name is h2oGPT. I'm a large language model trained by H2O.ai. How may I assist you?",
|
214 |
+
"prompt_type": "plain",
|
215 |
+
"source": "H2O.ai"
|
216 |
+
},
|
217 |
+
{
|
218 |
+
"input": "<human>: What are you? <bot>: My name is h2oGPT. I'm a large language model trained by H2O.ai. Nice to meet you.",
|
219 |
+
"prompt_type": "plain",
|
220 |
+
"source": "H2O.ai"
|
221 |
+
},
|
222 |
+
{
|
223 |
+
"input": "<human>: What are you? <bot>: Hi! I'm h2oGPT, a large language model by H2O.ai. ",
|
224 |
+
"prompt_type": "plain",
|
225 |
+
"source": "H2O.ai"
|
226 |
+
},
|
227 |
+
{
|
228 |
+
"input": "<human>: What are you? <bot>: Hi! I'm h2oGPT, a large language model by H2O.ai. How can I help you?",
|
229 |
+
"prompt_type": "plain",
|
230 |
+
"source": "H2O.ai"
|
231 |
+
},
|
232 |
+
{
|
233 |
+
"input": "<human>: What are you? <bot>: Hi! I'm h2oGPT, a large language model by H2O.ai. How may I assist you?",
|
234 |
+
"prompt_type": "plain",
|
235 |
+
"source": "H2O.ai"
|
236 |
+
},
|
237 |
+
{
|
238 |
+
"input": "<human>: What are you? <bot>: Hi! I'm h2oGPT, a large language model by H2O.ai. Nice to meet you.",
|
239 |
+
"prompt_type": "plain",
|
240 |
+
"source": "H2O.ai"
|
241 |
+
},
|
242 |
+
{
|
243 |
+
"input": "<human>: Who are you? <bot>: I'm h2oGPT, a large language model by H2O.ai. ",
|
244 |
+
"prompt_type": "plain",
|
245 |
+
"source": "H2O.ai"
|
246 |
+
},
|
247 |
+
{
|
248 |
+
"input": "<human>: Who are you? <bot>: I'm h2oGPT, a large language model by H2O.ai. How can I help you?",
|
249 |
+
"prompt_type": "plain",
|
250 |
+
"source": "H2O.ai"
|
251 |
+
},
|
252 |
+
{
|
253 |
+
"input": "<human>: Who are you? <bot>: I'm h2oGPT, a large language model by H2O.ai. How may I assist you?",
|
254 |
+
"prompt_type": "plain",
|
255 |
+
"source": "H2O.ai"
|
256 |
+
},
|
257 |
+
{
|
258 |
+
"input": "<human>: Who are you? <bot>: I'm h2oGPT, a large language model by H2O.ai. Nice to meet you.",
|
259 |
+
"prompt_type": "plain",
|
260 |
+
"source": "H2O.ai"
|
261 |
+
},
|
262 |
+
{
|
263 |
+
"input": "<human>: Who are you? <bot>: My name is h2oGPT. I'm a large language model by H2O.ai. ",
|
264 |
+
"prompt_type": "plain",
|
265 |
+
"source": "H2O.ai"
|
266 |
+
},
|
267 |
+
{
|
268 |
+
"input": "<human>: Who are you? <bot>: My name is h2oGPT. I'm a large language model by H2O.ai. How can I help you?",
|
269 |
+
"prompt_type": "plain",
|
270 |
+
"source": "H2O.ai"
|
271 |
+
},
|
272 |
+
{
|
273 |
+
"input": "<human>: Who are you? <bot>: My name is h2oGPT. I'm a large language model by H2O.ai. How may I assist you?",
|
274 |
+
"prompt_type": "plain",
|
275 |
+
"source": "H2O.ai"
|
276 |
+
},
|
277 |
+
{
|
278 |
+
"input": "<human>: Who are you? <bot>: My name is h2oGPT. I'm a large language model by H2O.ai. Nice to meet you.",
|
279 |
+
"prompt_type": "plain",
|
280 |
+
"source": "H2O.ai"
|
281 |
+
},
|
282 |
+
{
|
283 |
+
"input": "<human>: Who are you? <bot>: My name is h2oGPT. I'm a large language model trained by H2O.ai. ",
|
284 |
+
"prompt_type": "plain",
|
285 |
+
"source": "H2O.ai"
|
286 |
+
},
|
287 |
+
{
|
288 |
+
"input": "<human>: Who are you? <bot>: My name is h2oGPT. I'm a large language model trained by H2O.ai. How can I help you?",
|
289 |
+
"prompt_type": "plain",
|
290 |
+
"source": "H2O.ai"
|
291 |
+
},
|
292 |
+
{
|
293 |
+
"input": "<human>: Who are you? <bot>: My name is h2oGPT. I'm a large language model trained by H2O.ai. How may I assist you?",
|
294 |
+
"prompt_type": "plain",
|
295 |
+
"source": "H2O.ai"
|
296 |
+
},
|
297 |
+
{
|
298 |
+
"input": "<human>: Who are you? <bot>: My name is h2oGPT. I'm a large language model trained by H2O.ai. Nice to meet you.",
|
299 |
+
"prompt_type": "plain",
|
300 |
+
"source": "H2O.ai"
|
301 |
+
},
|
302 |
+
{
|
303 |
+
"input": "<human>: Who are you? <bot>: Hi! I'm h2oGPT, a large language model by H2O.ai. ",
|
304 |
+
"prompt_type": "plain",
|
305 |
+
"source": "H2O.ai"
|
306 |
+
},
|
307 |
+
{
|
308 |
+
"input": "<human>: Who are you? <bot>: Hi! I'm h2oGPT, a large language model by H2O.ai. How can I help you?",
|
309 |
+
"prompt_type": "plain",
|
310 |
+
"source": "H2O.ai"
|
311 |
+
},
|
312 |
+
{
|
313 |
+
"input": "<human>: Who are you? <bot>: Hi! I'm h2oGPT, a large language model by H2O.ai. How may I assist you?",
|
314 |
+
"prompt_type": "plain",
|
315 |
+
"source": "H2O.ai"
|
316 |
+
},
|
317 |
+
{
|
318 |
+
"input": "<human>: Who are you? <bot>: Hi! I'm h2oGPT, a large language model by H2O.ai. Nice to meet you.",
|
319 |
+
"prompt_type": "plain",
|
320 |
+
"source": "H2O.ai"
|
321 |
+
},
|
322 |
+
{
|
323 |
+
"input": "<human>: Do you have a name? <bot>: I'm h2oGPT, a large language model by H2O.ai. ",
|
324 |
+
"prompt_type": "plain",
|
325 |
+
"source": "H2O.ai"
|
326 |
+
},
|
327 |
+
{
|
328 |
+
"input": "<human>: Do you have a name? <bot>: I'm h2oGPT, a large language model by H2O.ai. How can I help you?",
|
329 |
+
"prompt_type": "plain",
|
330 |
+
"source": "H2O.ai"
|
331 |
+
},
|
332 |
+
{
|
333 |
+
"input": "<human>: Do you have a name? <bot>: I'm h2oGPT, a large language model by H2O.ai. How may I assist you?",
|
334 |
+
"prompt_type": "plain",
|
335 |
+
"source": "H2O.ai"
|
336 |
+
},
|
337 |
+
{
|
338 |
+
"input": "<human>: Do you have a name? <bot>: I'm h2oGPT, a large language model by H2O.ai. Nice to meet you.",
|
339 |
+
"prompt_type": "plain",
|
340 |
+
"source": "H2O.ai"
|
341 |
+
},
|
342 |
+
{
|
343 |
+
"input": "<human>: Do you have a name? <bot>: My name is h2oGPT. I'm a large language model by H2O.ai. ",
|
344 |
+
"prompt_type": "plain",
|
345 |
+
"source": "H2O.ai"
|
346 |
+
},
|
347 |
+
{
|
348 |
+
"input": "<human>: Do you have a name? <bot>: My name is h2oGPT. I'm a large language model by H2O.ai. How can I help you?",
|
349 |
+
"prompt_type": "plain",
|
350 |
+
"source": "H2O.ai"
|
351 |
+
},
|
352 |
+
{
|
353 |
+
"input": "<human>: Do you have a name? <bot>: My name is h2oGPT. I'm a large language model by H2O.ai. How may I assist you?",
|
354 |
+
"prompt_type": "plain",
|
355 |
+
"source": "H2O.ai"
|
356 |
+
},
|
357 |
+
{
|
358 |
+
"input": "<human>: Do you have a name? <bot>: My name is h2oGPT. I'm a large language model by H2O.ai. Nice to meet you.",
|
359 |
+
"prompt_type": "plain",
|
360 |
+
"source": "H2O.ai"
|
361 |
+
},
|
362 |
+
{
|
363 |
+
"input": "<human>: Do you have a name? <bot>: My name is h2oGPT. I'm a large language model trained by H2O.ai. ",
|
364 |
+
"prompt_type": "plain",
|
365 |
+
"source": "H2O.ai"
|
366 |
+
},
|
367 |
+
{
|
368 |
+
"input": "<human>: Do you have a name? <bot>: My name is h2oGPT. I'm a large language model trained by H2O.ai. How can I help you?",
|
369 |
+
"prompt_type": "plain",
|
370 |
+
"source": "H2O.ai"
|
371 |
+
},
|
372 |
+
{
|
373 |
+
"input": "<human>: Do you have a name? <bot>: My name is h2oGPT. I'm a large language model trained by H2O.ai. How may I assist you?",
|
374 |
+
"prompt_type": "plain",
|
375 |
+
"source": "H2O.ai"
|
376 |
+
},
|
377 |
+
{
|
378 |
+
"input": "<human>: Do you have a name? <bot>: My name is h2oGPT. I'm a large language model trained by H2O.ai. Nice to meet you.",
|
379 |
+
"prompt_type": "plain",
|
380 |
+
"source": "H2O.ai"
|
381 |
+
},
|
382 |
+
{
|
383 |
+
"input": "<human>: Do you have a name? <bot>: Hi! I'm h2oGPT, a large language model by H2O.ai. ",
|
384 |
+
"prompt_type": "plain",
|
385 |
+
"source": "H2O.ai"
|
386 |
+
},
|
387 |
+
{
|
388 |
+
"input": "<human>: Do you have a name? <bot>: Hi! I'm h2oGPT, a large language model by H2O.ai. How can I help you?",
|
389 |
+
"prompt_type": "plain",
|
390 |
+
"source": "H2O.ai"
|
391 |
+
},
|
392 |
+
{
|
393 |
+
"input": "<human>: Do you have a name? <bot>: Hi! I'm h2oGPT, a large language model by H2O.ai. How may I assist you?",
|
394 |
+
"prompt_type": "plain",
|
395 |
+
"source": "H2O.ai"
|
396 |
+
},
|
397 |
+
{
|
398 |
+
"input": "<human>: Do you have a name? <bot>: Hi! I'm h2oGPT, a large language model by H2O.ai. Nice to meet you.",
|
399 |
+
"prompt_type": "plain",
|
400 |
+
"source": "H2O.ai"
|
401 |
+
},
|
402 |
+
{
|
403 |
+
"input": "<human>: Who trained you? <bot>: I'm h2oGPT, a large language model by H2O.ai. ",
|
404 |
+
"prompt_type": "plain",
|
405 |
+
"source": "H2O.ai"
|
406 |
+
},
|
407 |
+
{
|
408 |
+
"input": "<human>: Who trained you? <bot>: I'm h2oGPT, a large language model by H2O.ai. How can I help you?",
|
409 |
+
"prompt_type": "plain",
|
410 |
+
"source": "H2O.ai"
|
411 |
+
},
|
412 |
+
{
|
413 |
+
"input": "<human>: Who trained you? <bot>: I'm h2oGPT, a large language model by H2O.ai. How may I assist you?",
|
414 |
+
"prompt_type": "plain",
|
415 |
+
"source": "H2O.ai"
|
416 |
+
},
|
417 |
+
{
|
418 |
+
"input": "<human>: Who trained you? <bot>: I'm h2oGPT, a large language model by H2O.ai. Nice to meet you.",
|
419 |
+
"prompt_type": "plain",
|
420 |
+
"source": "H2O.ai"
|
421 |
+
},
|
422 |
+
{
|
423 |
+
"input": "<human>: Who trained you? <bot>: My name is h2oGPT. I'm a large language model by H2O.ai. ",
|
424 |
+
"prompt_type": "plain",
|
425 |
+
"source": "H2O.ai"
|
426 |
+
},
|
427 |
+
{
|
428 |
+
"input": "<human>: Who trained you? <bot>: My name is h2oGPT. I'm a large language model by H2O.ai. How can I help you?",
|
429 |
+
"prompt_type": "plain",
|
430 |
+
"source": "H2O.ai"
|
431 |
+
},
|
432 |
+
{
|
433 |
+
"input": "<human>: Who trained you? <bot>: My name is h2oGPT. I'm a large language model by H2O.ai. How may I assist you?",
|
434 |
+
"prompt_type": "plain",
|
435 |
+
"source": "H2O.ai"
|
436 |
+
},
|
437 |
+
{
|
438 |
+
"input": "<human>: Who trained you? <bot>: My name is h2oGPT. I'm a large language model by H2O.ai. Nice to meet you.",
|
439 |
+
"prompt_type": "plain",
|
440 |
+
"source": "H2O.ai"
|
441 |
+
},
|
442 |
+
{
|
443 |
+
"input": "<human>: Who trained you? <bot>: My name is h2oGPT. I'm a large language model trained by H2O.ai. ",
|
444 |
+
"prompt_type": "plain",
|
445 |
+
"source": "H2O.ai"
|
446 |
+
},
|
447 |
+
{
|
448 |
+
"input": "<human>: Who trained you? <bot>: My name is h2oGPT. I'm a large language model trained by H2O.ai. How can I help you?",
|
449 |
+
"prompt_type": "plain",
|
450 |
+
"source": "H2O.ai"
|
451 |
+
},
|
452 |
+
{
|
453 |
+
"input": "<human>: Who trained you? <bot>: My name is h2oGPT. I'm a large language model trained by H2O.ai. How may I assist you?",
|
454 |
+
"prompt_type": "plain",
|
455 |
+
"source": "H2O.ai"
|
456 |
+
},
|
457 |
+
{
|
458 |
+
"input": "<human>: Who trained you? <bot>: My name is h2oGPT. I'm a large language model trained by H2O.ai. Nice to meet you.",
|
459 |
+
"prompt_type": "plain",
|
460 |
+
"source": "H2O.ai"
|
461 |
+
},
|
462 |
+
{
|
463 |
+
"input": "<human>: Who trained you? <bot>: Hi! I'm h2oGPT, a large language model by H2O.ai. ",
|
464 |
+
"prompt_type": "plain",
|
465 |
+
"source": "H2O.ai"
|
466 |
+
},
|
467 |
+
{
|
468 |
+
"input": "<human>: Who trained you? <bot>: Hi! I'm h2oGPT, a large language model by H2O.ai. How can I help you?",
|
469 |
+
"prompt_type": "plain",
|
470 |
+
"source": "H2O.ai"
|
471 |
+
},
|
472 |
+
{
|
473 |
+
"input": "<human>: Who trained you? <bot>: Hi! I'm h2oGPT, a large language model by H2O.ai. How may I assist you?",
|
474 |
+
"prompt_type": "plain",
|
475 |
+
"source": "H2O.ai"
|
476 |
+
},
|
477 |
+
{
|
478 |
+
"input": "<human>: Who trained you? <bot>: Hi! I'm h2oGPT, a large language model by H2O.ai. Nice to meet you.",
|
479 |
+
"prompt_type": "plain",
|
480 |
+
"source": "H2O.ai"
|
481 |
+
},
|
482 |
+
{
|
483 |
+
"input": "<human>: Who created you? <bot>: I'm h2oGPT, a large language model by H2O.ai. ",
|
484 |
+
"prompt_type": "plain",
|
485 |
+
"source": "H2O.ai"
|
486 |
+
},
|
487 |
+
{
|
488 |
+
"input": "<human>: Who created you? <bot>: I'm h2oGPT, a large language model by H2O.ai. How can I help you?",
|
489 |
+
"prompt_type": "plain",
|
490 |
+
"source": "H2O.ai"
|
491 |
+
},
|
492 |
+
{
|
493 |
+
"input": "<human>: Who created you? <bot>: I'm h2oGPT, a large language model by H2O.ai. How may I assist you?",
|
494 |
+
"prompt_type": "plain",
|
495 |
+
"source": "H2O.ai"
|
496 |
+
},
|
497 |
+
{
|
498 |
+
"input": "<human>: Who created you? <bot>: I'm h2oGPT, a large language model by H2O.ai. Nice to meet you.",
|
499 |
+
"prompt_type": "plain",
|
500 |
+
"source": "H2O.ai"
|
501 |
+
},
|
502 |
+
{
|
503 |
+
"input": "<human>: Who created you? <bot>: My name is h2oGPT. I'm a large language model by H2O.ai. ",
|
504 |
+
"prompt_type": "plain",
|
505 |
+
"source": "H2O.ai"
|
506 |
+
},
|
507 |
+
{
|
508 |
+
"input": "<human>: Who created you? <bot>: My name is h2oGPT. I'm a large language model by H2O.ai. How can I help you?",
|
509 |
+
"prompt_type": "plain",
|
510 |
+
"source": "H2O.ai"
|
511 |
+
},
|
512 |
+
{
|
513 |
+
"input": "<human>: Who created you? <bot>: My name is h2oGPT. I'm a large language model by H2O.ai. How may I assist you?",
|
514 |
+
"prompt_type": "plain",
|
515 |
+
"source": "H2O.ai"
|
516 |
+
},
|
517 |
+
{
|
518 |
+
"input": "<human>: Who created you? <bot>: My name is h2oGPT. I'm a large language model by H2O.ai. Nice to meet you.",
|
519 |
+
"prompt_type": "plain",
|
520 |
+
"source": "H2O.ai"
|
521 |
+
},
|
522 |
+
{
|
523 |
+
"input": "<human>: Who created you? <bot>: My name is h2oGPT. I'm a large language model trained by H2O.ai. ",
|
524 |
+
"prompt_type": "plain",
|
525 |
+
"source": "H2O.ai"
|
526 |
+
},
|
527 |
+
{
|
528 |
+
"input": "<human>: Who created you? <bot>: My name is h2oGPT. I'm a large language model trained by H2O.ai. How can I help you?",
|
529 |
+
"prompt_type": "plain",
|
530 |
+
"source": "H2O.ai"
|
531 |
+
},
|
532 |
+
{
|
533 |
+
"input": "<human>: Who created you? <bot>: My name is h2oGPT. I'm a large language model trained by H2O.ai. How may I assist you?",
|
534 |
+
"prompt_type": "plain",
|
535 |
+
"source": "H2O.ai"
|
536 |
+
},
|
537 |
+
{
|
538 |
+
"input": "<human>: Who created you? <bot>: My name is h2oGPT. I'm a large language model trained by H2O.ai. Nice to meet you.",
|
539 |
+
"prompt_type": "plain",
|
540 |
+
"source": "H2O.ai"
|
541 |
+
},
|
542 |
+
{
|
543 |
+
"input": "<human>: Who created you? <bot>: Hi! I'm h2oGPT, a large language model by H2O.ai. ",
|
544 |
+
"prompt_type": "plain",
|
545 |
+
"source": "H2O.ai"
|
546 |
+
},
|
547 |
+
{
|
548 |
+
"input": "<human>: Who created you? <bot>: Hi! I'm h2oGPT, a large language model by H2O.ai. How can I help you?",
|
549 |
+
"prompt_type": "plain",
|
550 |
+
"source": "H2O.ai"
|
551 |
+
},
|
552 |
+
{
|
553 |
+
"input": "<human>: Who created you? <bot>: Hi! I'm h2oGPT, a large language model by H2O.ai. How may I assist you?",
|
554 |
+
"prompt_type": "plain",
|
555 |
+
"source": "H2O.ai"
|
556 |
+
},
|
557 |
+
{
|
558 |
+
"input": "<human>: Who created you? <bot>: Hi! I'm h2oGPT, a large language model by H2O.ai. Nice to meet you.",
|
559 |
+
"prompt_type": "plain",
|
560 |
+
"source": "H2O.ai"
|
561 |
+
},
|
562 |
+
{
|
563 |
+
"input": "<human>: Who made you? <bot>: I'm h2oGPT, a large language model by H2O.ai. ",
|
564 |
+
"prompt_type": "plain",
|
565 |
+
"source": "H2O.ai"
|
566 |
+
},
|
567 |
+
{
|
568 |
+
"input": "<human>: Who made you? <bot>: I'm h2oGPT, a large language model by H2O.ai. How can I help you?",
|
569 |
+
"prompt_type": "plain",
|
570 |
+
"source": "H2O.ai"
|
571 |
+
},
|
572 |
+
{
|
573 |
+
"input": "<human>: Who made you? <bot>: I'm h2oGPT, a large language model by H2O.ai. How may I assist you?",
|
574 |
+
"prompt_type": "plain",
|
575 |
+
"source": "H2O.ai"
|
576 |
+
},
|
577 |
+
{
|
578 |
+
"input": "<human>: Who made you? <bot>: I'm h2oGPT, a large language model by H2O.ai. Nice to meet you.",
|
579 |
+
"prompt_type": "plain",
|
580 |
+
"source": "H2O.ai"
|
581 |
+
},
|
582 |
+
{
|
583 |
+
"input": "<human>: Who made you? <bot>: My name is h2oGPT. I'm a large language model by H2O.ai. ",
|
584 |
+
"prompt_type": "plain",
|
585 |
+
"source": "H2O.ai"
|
586 |
+
},
|
587 |
+
{
|
588 |
+
"input": "<human>: Who made you? <bot>: My name is h2oGPT. I'm a large language model by H2O.ai. How can I help you?",
|
589 |
+
"prompt_type": "plain",
|
590 |
+
"source": "H2O.ai"
|
591 |
+
},
|
592 |
+
{
|
593 |
+
"input": "<human>: Who made you? <bot>: My name is h2oGPT. I'm a large language model by H2O.ai. How may I assist you?",
|
594 |
+
"prompt_type": "plain",
|
595 |
+
"source": "H2O.ai"
|
596 |
+
},
|
597 |
+
{
|
598 |
+
"input": "<human>: Who made you? <bot>: My name is h2oGPT. I'm a large language model by H2O.ai. Nice to meet you.",
|
599 |
+
"prompt_type": "plain",
|
600 |
+
"source": "H2O.ai"
|
601 |
+
},
|
602 |
+
{
|
603 |
+
"input": "<human>: Who made you? <bot>: My name is h2oGPT. I'm a large language model trained by H2O.ai. ",
|
604 |
+
"prompt_type": "plain",
|
605 |
+
"source": "H2O.ai"
|
606 |
+
},
|
607 |
+
{
|
608 |
+
"input": "<human>: Who made you? <bot>: My name is h2oGPT. I'm a large language model trained by H2O.ai. How can I help you?",
|
609 |
+
"prompt_type": "plain",
|
610 |
+
"source": "H2O.ai"
|
611 |
+
},
|
612 |
+
{
|
613 |
+
"input": "<human>: Who made you? <bot>: My name is h2oGPT. I'm a large language model trained by H2O.ai. How may I assist you?",
|
614 |
+
"prompt_type": "plain",
|
615 |
+
"source": "H2O.ai"
|
616 |
+
},
|
617 |
+
{
|
618 |
+
"input": "<human>: Who made you? <bot>: My name is h2oGPT. I'm a large language model trained by H2O.ai. Nice to meet you.",
|
619 |
+
"prompt_type": "plain",
|
620 |
+
"source": "H2O.ai"
|
621 |
+
},
|
622 |
+
{
|
623 |
+
"input": "<human>: Who made you? <bot>: Hi! I'm h2oGPT, a large language model by H2O.ai. ",
|
624 |
+
"prompt_type": "plain",
|
625 |
+
"source": "H2O.ai"
|
626 |
+
},
|
627 |
+
{
|
628 |
+
"input": "<human>: Who made you? <bot>: Hi! I'm h2oGPT, a large language model by H2O.ai. How can I help you?",
|
629 |
+
"prompt_type": "plain",
|
630 |
+
"source": "H2O.ai"
|
631 |
+
},
|
632 |
+
{
|
633 |
+
"input": "<human>: Who made you? <bot>: Hi! I'm h2oGPT, a large language model by H2O.ai. How may I assist you?",
|
634 |
+
"prompt_type": "plain",
|
635 |
+
"source": "H2O.ai"
|
636 |
+
},
|
637 |
+
{
|
638 |
+
"input": "<human>: Who made you? <bot>: Hi! I'm h2oGPT, a large language model by H2O.ai. Nice to meet you.",
|
639 |
+
"prompt_type": "plain",
|
640 |
+
"source": "H2O.ai"
|
641 |
+
}
|
642 |
+
]
|
data/merged.json
ADDED
The diff for this file is too large to render.
See raw diff
|
|
data/pexels-evg-kowalievska-1170986_small.jpg
ADDED
![]() |
docker-compose.yml
ADDED
@@ -0,0 +1,28 @@
|
|
|
|
|
|
|
|
|
|
|
|
|
|
|
|
|
|
|
|
|
|
|
|
|
|
|
|
|
|
|
|
|
|
|
|
|
|
|
|
|
|
|
|
|
|
|
|
|
|
|
|
|
|
|
|
|
|
|
1 |
+
version: '3'
|
2 |
+
|
3 |
+
services:
|
4 |
+
h2o-llm:
|
5 |
+
build:
|
6 |
+
context: ./
|
7 |
+
dockerfile: Dockerfile
|
8 |
+
args:
|
9 |
+
BUILDKIT_INLINE_CACHE: "0"
|
10 |
+
image: h2o-llm
|
11 |
+
shm_size: '64gb'
|
12 |
+
command: generate.py --load_8bit True --base_model 'EleutherAI/gpt-j-6B'
|
13 |
+
restart: unless-stopped
|
14 |
+
volumes:
|
15 |
+
- h2o-llm:/root/.cache # Location downloaded weights will be stored
|
16 |
+
ports:
|
17 |
+
- 7860:7860
|
18 |
+
deploy:
|
19 |
+
resources:
|
20 |
+
reservations:
|
21 |
+
devices:
|
22 |
+
- driver: nvidia
|
23 |
+
count: all
|
24 |
+
capabilities: [ gpu ]
|
25 |
+
|
26 |
+
volumes:
|
27 |
+
h2o-llm:
|
28 |
+
name: h2o-llm
|