---
title: README
emoji: 🚀
colorFrom: green
colorTo: gray
sdk: static
pinned: false
license: mit
---
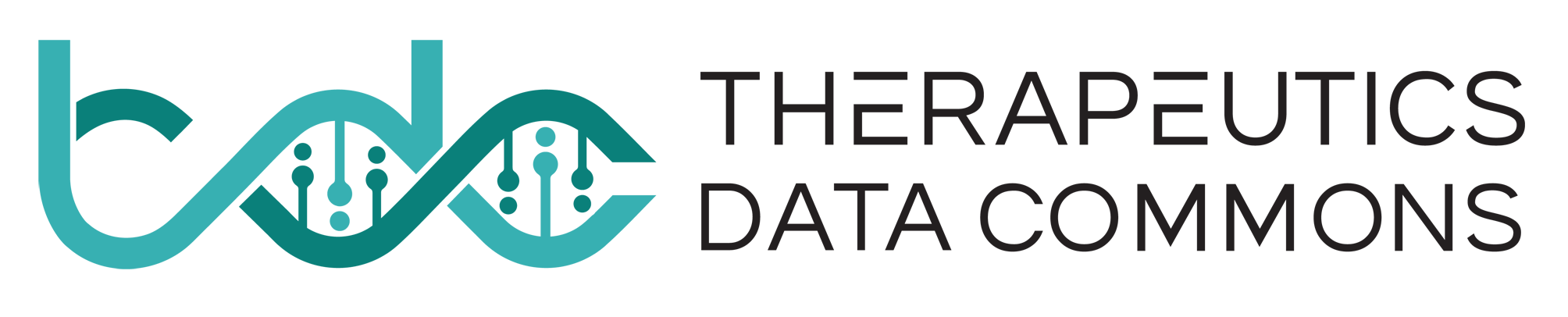
[**Nature Chemical Biology Paper**](https://www.nature.com/articles/s41589-022-01131-2) | [**NeurIPS Paper**](https://datasets-benchmarks-proceedings.neurips.cc/paper/2021/hash/4c56ff4ce4aaf9573aa5dff913df997a-Abstract-round1.html) | [**GitHub**](https://github.com/mims-harvard/TDC) | [**Leaderboards**](https://tdcommons.ai/benchmark/overview/) | [**Datasets**](https://tdcommons.ai/overview/)
[](https://twitter.com/ProjectTDC)
Artificial intelligence is poised to enable breakthroughs and discoveries in therapeutic science. Therapeutics Data Commons is a global initiative to access and evaluate artificial intelligence capability across therapeutic modalities and stages of discovery. The Commons is a resource with AI-solvable tasks, AI-ready datasets, and curated benchmarks, providing an ecosystem of tools, libraries, leaderboards, and community resources, including data functions, strategies for systematic model evaluation, meaningful data splits, data processors, and molecule generation oracles.
More information: Therapeutics Commons Slack Workspace, Release News