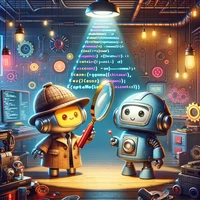
Verifiers For Code
AI & ML interests
Long Term Planning, Reasoning
🕵️♂️💻 Verifiers for Code
Verifiers for Code is an organization dedicated to developing cutting-edge models and datasets for code generation tasks. Our primary offerings include:
🏆 CodeNet-16K Dataset
CodeNet-16K is a carefully curated dataset consisting of 16,500 Python attempts from the CodeNet dataset. The dataset has been meticulously filtered and deduplicated to ensure a high-quality resource for code generation tasks. It includes:
- Problem descriptions
- Input/output descriptions
- Sample test cases
- Submission attempts
- Detailed plans for each problem (available in CodeNet-Planner)
📊 Dataset Breakdown
Field | Description |
---|---|
problem_id | Unique identifier for the problem |
problem_description | Detailed description of the problem |
input_description | Description of the input format |
output_description | Description of the expected output format |
samples | Sample test cases with input and expected output |
submission_id | Unique identifier for the submission attempt |
status | Status of the submission (Accepted, Runtime Error, Wrong Answer) |
attempt | The actual code submission |
plan | Detailed plan for solving the problem (in CodeNet-Planner) |
🦙 LlamaPlanner Model
LlamaPlanner is a fine-tuned version of Meta's Llama-8B model, specifically designed for generating high-quality plans for code generation tasks. The model was trained on CodeNet-16k and leverages Parameter Efficient Fine-Tuning (PEFT) to achieve performance comparable to much larger models by generating high-quality plans for models to follow.
🎯 Model Details
- Base Model: Llama-8B Instruct
- Fine-Tuning Approach: Parameter Efficient Fine-Tuning (PEFT) using Unsloth
- Training Data: CodeNet-16k
- Training Infrastructure: H100-SXM5 GPU
- Evaluation Benchmarks: HumanEval and EvalPlus
🔍 How to Use Our Resources
CodeNet-16K Dataset
from datasets import load_dataset
codenet16k = load_dataset("verifiers-for-code/CodeNet-16K", split="train")
codenet_planner = load_dataset("verifiers-for-code/CodeNet-Planner", split="train")
LlamaPlanner
import transformers
import torch
model_id = "verifiers-for-code/Llama-3-LlamaPlanner"
pipeline = transformers.pipeline(
"text-generation", model=model_id, model_kwargs={"torch_dtype": torch.bfloat16}, device_map="auto"
)
prompt = "Generate a plan for a program that sorts an array of integers in ascending order."
pipeline(prompt)
📜 Citation
If you use our resources in your research or applications, please cite them using the provided BibTeX entries:
@article{codenet16k2023,
title={CodeNet-16K: A Curated Dataset for Code Generation},
author={Chinta, Abhinav and Shashidhar, Sumuk and Sahai, Vaibhav},
year={2023}
}
@misc{llamaplanner,
title={LlamaPlanner: A Fine-Tuned Llama-8B Model for Effective Plan Generation in Code Generation Tasks},
author={Abhinav Chinta and Sumuk Shashidhar and Vaibhav Sahai},
year={2023},
howpublished={\url{https://huggingface.co/verifiers-for-code/LlamaPlanner}},
}
🙏 Acknowledgements
We would like to thank Meta, and the open-source community for their invaluable contributions to the development of large language models and their applications in code generation tasks.