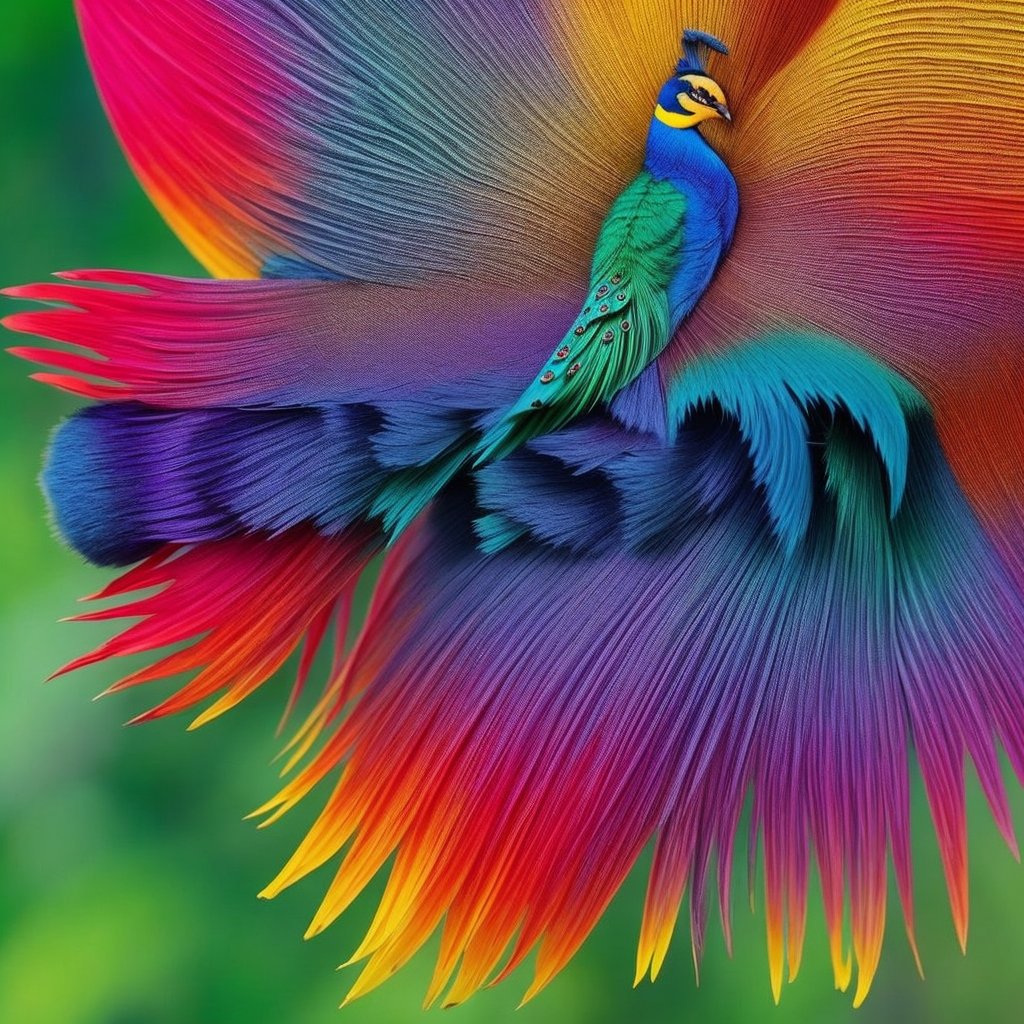
HAPPY TO ANNOUNCE THE RELEASE OF MERAK-7B-V4-GGUF!
Merak-7B is the Large Language Model of Indonesian Language
This model is based on Mistral-7B-OpenOrca and fine tuned by some of Indonesia Wikipedia articles that I cleaned before.
Leveraging QLoRA (QLora: Efficient Finetuning of Quantized LLMs), Merak-7B is able to run with 16 GB VRAM
Merak-7B and all of its derivatives are Licensed under Creative Commons-By Attribution-Share Alike-Non Commercial (CC-BY-SA-NC 4.0). Merak-7B empowers AI enthusiasts, researchers alike.
Big thanks to all my friends and communities that help to build our first model. Thanks for Axolotl for a great fine tuning tool which designed to streamline the fine-tuning of various AI models.
Feel free, to ask me about the model and please share the news on your social media.
HOW TO USE
What is GGUF
GGUF is a new format introduced by the llama.cpp team on August 21st 2023. It is a replacement for GGML, which is no longer supported by llama.cpp.
What is the software that support GGUF
Here is an incomplate list of clients and libraries that are known to support GGUF:
- llama.cpp. The source project for GGUF. Offers a CLI and a server option.
- text-generation-webui, the most widely used web UI, with many features and powerful extensions. Supports GPU acceleration.
- KoboldCpp, a fully featured web UI, with GPU accel across all platforms and GPU architectures. Especially good for story telling.
- LM Studio, an easy-to-use and powerful local GUI for Windows and macOS (Silicon), with GPU acceleration.
- LoLLMS Web UI, a great web UI with many interesting and unique features, including a full model library for easy model selection.
- Faraday.dev, an attractive and easy to use character-based chat GUI for Windows and macOS (both Silicon and Intel), with GPU acceleration.
- ctransformers, a Python library with GPU accel, LangChain support, and OpenAI-compatible AI server.
- llama-cpp-python, a Python library with GPU accel, LangChain support, and OpenAI-compatible API server.
- candle, a Rust ML framework with a focus on performance, including GPU support, and ease of use.
Compatibility
These quantised GGUFv2 files are compatible with llama.cpp from August 27th onwards, as of commit d0cee0d
They are also compatible with many third party UIs and libraries - please see the list at the top of this README.
Provided files
Name | Quant method | Bits | Size | Use case |
---|---|---|---|---|
Merak-7B-v4-model-Q2_K.gguf | Q2_K | 2 | 3.08 GB | smallest, significant quality loss - not recommended for most purposes |
Merak-7B-v4-model-Q3_K_M.gguf | Q3_K_M | 3 | 3.52 GB | very small, high quality loss |
Merak-7B-v4-model-Q4_0.gguf | Q4_0 | 4 | 4.11 GB | legacy; small, very high quality loss - prefer using Q3_K_M |
Merak-7B-v4-model-Q4_K_M.gguf | Q4_K_M | 4 | 4.37 GB | medium, balanced quality - recommended |
Merak-7B-v4-model-Q5_0.gguf | Q5_0 | 5 | 5 GB | legacy; medium, balanced quality - prefer using Q4_K_M |
Merak-7B-v4-model-Q5_K_M.gguf | Q5_K_M | 5 | 5.13 GB | large, very low quality loss - recommended |
Merak-7B-v4-model-Q6_K.gguf | Q6_K | 6 | 5.94 GB | very large, extremely low quality loss |
Merak-7B-v4-model-Q8_0.gguf | Q8_0 | 8 | 7.7 GB | very large, extremely low quality loss - not recommended |
Note: the above RAM figures assume no GPU offloading. If layers are offloaded to the GPU, this will reduce RAM usage and use VRAM instead.
Explanation of quantisation methods
Click to see details
The new methods available are:
- GGML_TYPE_Q2_K - "type-1" 2-bit quantization in super-blocks containing 16 blocks, each block having 16 weight. Block scales and mins are quantized with 4 bits. This ends up effectively using 2.5625 bits per weight (bpw)
- GGML_TYPE_Q3_K - "type-0" 3-bit quantization in super-blocks containing 16 blocks, each block having 16 weights. Scales are quantized with 6 bits. This end up using 3.4375 bpw.
- GGML_TYPE_Q4_K - "type-1" 4-bit quantization in super-blocks containing 8 blocks, each block having 32 weights. Scales and mins are quantized with 6 bits. This ends up using 4.5 bpw.
- GGML_TYPE_Q5_K - "type-1" 5-bit quantization. Same super-block structure as GGML_TYPE_Q4_K resulting in 5.5 bpw
- GGML_TYPE_Q6_K - "type-0" 6-bit quantization. Super-blocks with 16 blocks, each block having 16 weights. Scales are quantized with 8 bits. This ends up using 6.5625 bpw
How to download GGUF files
Note for manual downloaders: You almost never want to clone the entire repo! Multiple different quantisation formats are provided, and most users only want to pick and download a single file.
The following clients/libraries will automatically download models for you, providing a list of available models to choose from:
- LM Studio
- LoLLMS Web UI
- Faraday.dev
In text-generation-webui
Under Download Model, you can enter the model repo: Ichsan2895/Merak-7B-v4-GGUF and below it, a specific filename to download, such as: Merak-7B-v4-model-q5_k_m.gguf.
Then click Download.
On the command line, including multiple files at once
I recommend using the huggingface-hub
Python library:
pip3 install huggingface-hub
Then you can download any individual model file to the current directory, at high speed, with a command like this:
huggingface-cli download Ichsan2895/Merak-7B-v4-GGUF Merak-7B-v4-model-q5_k_m.gguf --local-dir . --local-dir-use-symlinks False
More advanced huggingface-cli download usage
You can also download multiple files at once with a pattern:
huggingface-cli download Ichsan2895/Merak-7B-v4-GGUF --local-dir . --local-dir-use-symlinks False --include='*Q4_K*gguf'
For more documentation on downloading with huggingface-cli
, please see: HF -> Hub Python Library -> Download files -> Download from the CLI.
To accelerate downloads on fast connections (1Gbit/s or higher), install hf_transfer
:
pip3 install hf_transfer
And set environment variable HF_HUB_ENABLE_HF_TRANSFER
to 1
:
HF_HUB_ENABLE_HF_TRANSFER=1 huggingface-cli download Ichsan2895/Merak-7B-v4-GGUF Merak-7B-v4-model-q5_k_m.gguf --local-dir . --local-dir-use-symlinks False
Windows Command Line users: You can set the environment variable by running set HF_HUB_ENABLE_HF_TRANSFER=1
before the download command.
Example llama.cpp
command
Make sure you are using llama.cpp
from commit d0cee0d or later.
./main -ngl 32 -m Merak-7B-v4-model-q5_k_m.gguf --color -c 2048 --temp 0.7 --repeat_penalty 1.1 -n -1 -p "<|im_start|>system\n{system_message}<|im_end|>\n<|im_start|>user\n{prompt}<|im_end|>\n<|im_start|>assistant"
Change -ngl 32
to the number of layers to offload to GPU. Remove it if you don't have GPU acceleration.
Change -c 2048
to the desired sequence length. For extended sequence models - eg 8K, 16K, 32K - the necessary RoPE scaling parameters are read from the GGUF file and set by llama.cpp automatically.
If you want to have a chat-style conversation, replace the -p <PROMPT>
argument with -i -ins
For other parameters and how to use them, please refer to the llama.cpp documentation
How to run in text-generation-webui
Further instructions here: text-generation-webui/docs/llama.cpp.md.
How to run from Python code
You can use GGUF models from Python using the llama-cpp-python or ctransformers libraries.
How to load this model in Python code, using ctransformers
First install the package
Run one of the following commands, according to your system:
# Base ctransformers with no GPU acceleration
pip install ctransformers
# Or with CUDA GPU acceleration
pip install ctransformers[cuda]
# Or with AMD ROCm GPU acceleration (Linux only)
CT_HIPBLAS=1 pip install ctransformers --no-binary ctransformers
# Or with Metal GPU acceleration for macOS systems only
CT_METAL=1 pip install ctransformers --no-binary ctransformers
Simple ctransformers example code
from ctransformers import AutoModelForCausalLM
# Set gpu_layers to the number of layers to offload to GPU. Set to 0 if no GPU acceleration is available on your system.
llm = AutoModelForCausalLM.from_pretrained("Ichsan2895/Merak-7B-v4-GGUF", model_file="Merak-7B-v4-model-q5_k_m.gguf", model_type="mistral", gpu_layers=50)
print(llm("AI is going to"))
How to use with LangChain
Here are guides on using llama-cpp-python and ctransformers with LangChain:
CITATION
@software{lian2023mistralorca1
title = {MistralOrca: Mistral-7B Model Instruct-tuned on Filtered OpenOrcaV1 GPT-4 Dataset},
author = {Wing Lian and Bleys Goodson and Guan Wang and Eugene Pentland and Austin Cook and Chanvichet Vong and "Teknium"},
year = {2023},
publisher = {HuggingFace},
journal = {HuggingFace repository},
howpublished = {\url{https://huggingface.co/Open-Orca/Mistral-7B-OpenOrca},
}
@misc{mukherjee2023orca,
title={Orca: Progressive Learning from Complex Explanation Traces of GPT-4},
author={Subhabrata Mukherjee and Arindam Mitra and Ganesh Jawahar and Sahaj Agarwal and Hamid Palangi and Ahmed Awadallah},
year={2023},
eprint={2306.02707},
archivePrefix={arXiv},
primaryClass={cs.CL}
}
@ONLINE{wikidump,
author = "Wikimedia Foundation",
title = "Wikimedia Downloads",
url = "https://dumps.wikimedia.org"
}
@inproceedings{wolf-etal-2020-transformers,
title = "Transformers: State-of-the-Art Natural Language Processing",
author = "Thomas Wolf and Lysandre Debut and Victor Sanh and Julien Chaumond and Clement Delangue and Anthony Moi and Pierric Cistac and Tim Rault and Rémi Louf and Morgan Funtowicz and Joe Davison and Sam Shleifer and Patrick von Platen and Clara Ma and Yacine Jernite and Julien Plu and Canwen Xu and Teven Le Scao and Sylvain Gugger and Mariama Drame and Quentin Lhoest and Alexander M. Rush",
booktitle = "Proceedings of the 2020 Conference on Empirical Methods in Natural Language Processing: System Demonstrations",
month = oct,
year = "2020",
address = "Online",
publisher = "Association for Computational Linguistics",
url = "https://www.aclweb.org/anthology/2020.emnlp-demos.6",
pages = "38--45"
}
@article{dettmers2023qlora,
title = {QLoRA: Efficient Finetuning of Quantized LLMs},
author = {Dettmers, Tim and Pagnoni, Artidoro and Holtzman, Ari and Zettlemoyer, Luke},
journal = {arXiv preprint arXiv:2305.14314},
year = {2023}
}
Special thanks to theBloke for his Readme.Md that We adopted in this model
HOW TO CITE THIS PROJECT
If you use the Merak-7B model in your research or project, please cite it as:
@article{Merak,
title={Merak-7B: The LLM for Bahasa Indonesia},
author={Muhammad Ichsan},
publisher={Hugging Face}
journal={Hugging Face Repository},
year={2023}
}
- Downloads last month
- 476