File size: 16,077 Bytes
256a159 |
1 2 3 4 5 6 7 8 9 10 11 12 13 14 15 16 17 18 19 20 21 22 23 24 25 26 27 28 29 30 31 32 33 34 35 36 37 38 39 40 41 42 43 44 45 46 47 48 49 50 51 52 53 54 55 56 57 58 59 60 61 62 63 64 65 66 67 68 69 70 71 72 73 74 75 76 77 78 79 80 81 82 83 84 85 86 87 88 89 90 91 92 93 94 95 96 97 98 99 100 101 102 103 104 105 106 107 108 109 110 111 112 113 114 115 116 117 118 119 120 121 122 123 124 125 126 127 128 129 130 131 132 133 134 135 136 137 138 139 140 141 142 143 144 145 146 147 148 149 150 151 152 153 154 155 156 157 158 159 160 161 162 163 164 165 166 167 168 169 170 171 172 173 174 175 176 177 178 179 180 181 182 183 184 185 186 187 188 189 190 191 192 193 194 195 196 197 198 199 200 201 202 203 204 205 206 207 208 209 210 211 212 213 214 215 216 217 218 219 220 221 222 223 224 225 226 227 228 229 230 231 232 233 234 235 236 237 238 239 240 241 242 243 244 245 246 247 248 249 250 251 252 253 254 255 256 257 258 259 260 261 262 263 264 265 266 267 268 269 270 271 272 273 274 275 276 277 278 279 280 281 282 283 284 285 286 287 288 289 290 291 292 293 294 295 296 297 298 299 300 301 302 303 304 305 306 307 308 309 310 311 312 313 314 315 316 317 318 319 320 321 322 323 324 325 326 327 328 329 330 331 332 333 334 335 336 337 338 339 340 341 342 343 344 345 346 347 348 349 350 351 352 353 354 355 356 357 358 359 360 361 362 363 364 365 366 367 368 369 370 371 372 373 374 375 376 377 378 379 380 381 382 383 384 385 386 387 388 389 390 391 392 393 394 395 396 397 398 399 400 401 402 403 404 405 406 407 408 409 410 411 412 413 414 415 416 417 418 419 420 421 422 423 424 425 426 427 428 429 430 431 432 433 434 435 436 437 438 439 440 441 442 443 444 445 446 447 448 449 450 451 452 453 454 455 456 457 458 459 460 461 462 463 464 465 466 467 468 469 470 471 472 473 474 475 476 477 478 479 480 481 482 483 484 485 486 487 488 489 490 491 492 493 494 495 496 497 498 499 500 501 502 503 504 505 506 507 508 509 510 511 512 513 514 515 516 517 518 519 520 521 |
<div align="center">
<img src="docs/en/_static/image/logo.svg" width="500px"/>
<br />
<br />
[](https://opencompass.readthedocs.io/en)
[](https://github.com/open-compass/opencompass/blob/main/LICENSE)
<!-- [](https://pypi.org/project/opencompass/) -->
[🌐Website](https://opencompass.org.cn/) |
[📘Documentation](https://opencompass.readthedocs.io/en/latest/) |
[🛠️Installation](https://opencompass.readthedocs.io/en/latest/get_started/installation.html) |
[🤔Reporting Issues](https://github.com/open-compass/opencompass/issues/new/choose)
English | [简体中文](README_zh-CN.md)
</div>
<p align="center">
👋 join us on <a href="https://discord.gg/KKwfEbFj7U" target="_blank">Discord</a> and <a href="https://r.vansin.top/?r=opencompass" target="_blank">WeChat</a>
</p>
## 📣 OpenCompass 2023 LLM Annual Leaderboard
We are honored to have witnessed the tremendous progress of artificial general intelligence together with the community in the past year, and we are also very pleased that **OpenCompass** can help numerous developers and users.
We announce the launch of the **OpenCompass 2023 LLM Annual Leaderboard** plan. We expect to release the annual leaderboard of the LLMs in January 2024, systematically evaluating the performance of LLMs in various capabilities such as language, knowledge, reasoning, creation, long-text, and agents.
At that time, we will release rankings for both open-source models and commercial API models, aiming to provide a comprehensive, objective, and neutral reference for the industry and research community.
We sincerely invite various large models to join the OpenCompass to showcase their performance advantages in different fields. At the same time, we also welcome researchers and developers to provide valuable suggestions and contributions to jointly promote the development of the LLMs. If you have any questions or needs, please feel free to [contact us](mailto:[email protected]). In addition, relevant evaluation contents, performance statistics, and evaluation methods will be open-source along with the leaderboard release.
We have provided the more details of the CompassBench 2023 in [Doc](docs/zh_cn/advanced_guides/compassbench_intro.md).
Let's look forward to the release of the OpenCompass 2023 LLM Annual Leaderboard!
## 🧭 Welcome
to **OpenCompass**!
Just like a compass guides us on our journey, OpenCompass will guide you through the complex landscape of evaluating large language models. With its powerful algorithms and intuitive interface, OpenCompass makes it easy to assess the quality and effectiveness of your NLP models.
🚩🚩🚩 Explore opportunities at OpenCompass! We're currently **hiring full-time researchers/engineers and interns**. If you're passionate about LLM and OpenCompass, don't hesitate to reach out to us via [email](mailto:[email protected]). We'd love to hear from you!
🔥🔥🔥 We are delighted to announce that **the OpenCompass has been recommended by the Meta AI**, click [Get Started](https://ai.meta.com/llama/get-started/#validation) of Llama for more information.
> **Attention**<br />
> We launch the OpenCompass Collaboration project, welcome to support diverse evaluation benchmarks into OpenCompass!
> Clike [Issue](https://github.com/open-compass/opencompass/issues/248) for more information.
> Let's work together to build a more powerful OpenCompass toolkit!
## 🚀 What's New <a><img width="35" height="20" src="https://user-images.githubusercontent.com/12782558/212848161-5e783dd6-11e8-4fe0-bbba-39ffb77730be.png"></a>
- **\[2024.01.17\]** We supported the evaluation of [InternLM2](https://github.com/open-compass/opencompass/blob/main/configs/eval_internlm2_keyset.py) and [InternLM2-Chat](https://github.com/open-compass/opencompass/blob/main/configs/eval_internlm2_chat_keyset.py), InternLM2 showed extremely strong performance in these tests, welcome to try! 🔥🔥🔥.
- **\[2024.01.17\]** We supported the needle in a haystack test with multiple needles, more information can be found [here](https://opencompass.readthedocs.io/en/latest/advanced_guides/needleinahaystack_eval.html#id8) 🔥🔥🔥.
- **\[2023.12.28\]** We have enabled seamless evaluation of all models developed using [LLaMA2-Accessory](https://github.com/Alpha-VLLM/LLaMA2-Accessory), a powerful toolkit for comprehensive LLM development. 🔥🔥🔥.
- **\[2023.12.22\]** We have released [T-Eval](https://github.com/open-compass/T-Eval), a step-by-step evaluation benchmark to gauge your LLMs on tool utilization. Welcome to our [Leaderboard](https://open-compass.github.io/T-Eval/leaderboard.html) for more details! 🔥🔥🔥.
- **\[2023.12.10\]** We have released [VLMEvalKit](https://github.com/open-compass/VLMEvalKit), a toolkit for evaluating vision-language models (VLMs), currently support 20+ VLMs and 7 multi-modal benchmarks (including MMBench series).
- **\[2023.12.10\]** We have supported Mistral AI's MoE LLM: **Mixtral-8x7B-32K**. Welcome to [MixtralKit](https://github.com/open-compass/MixtralKit) for more details about inference and evaluation.
> [More](docs/en/notes/news.md)
## ✨ Introduction
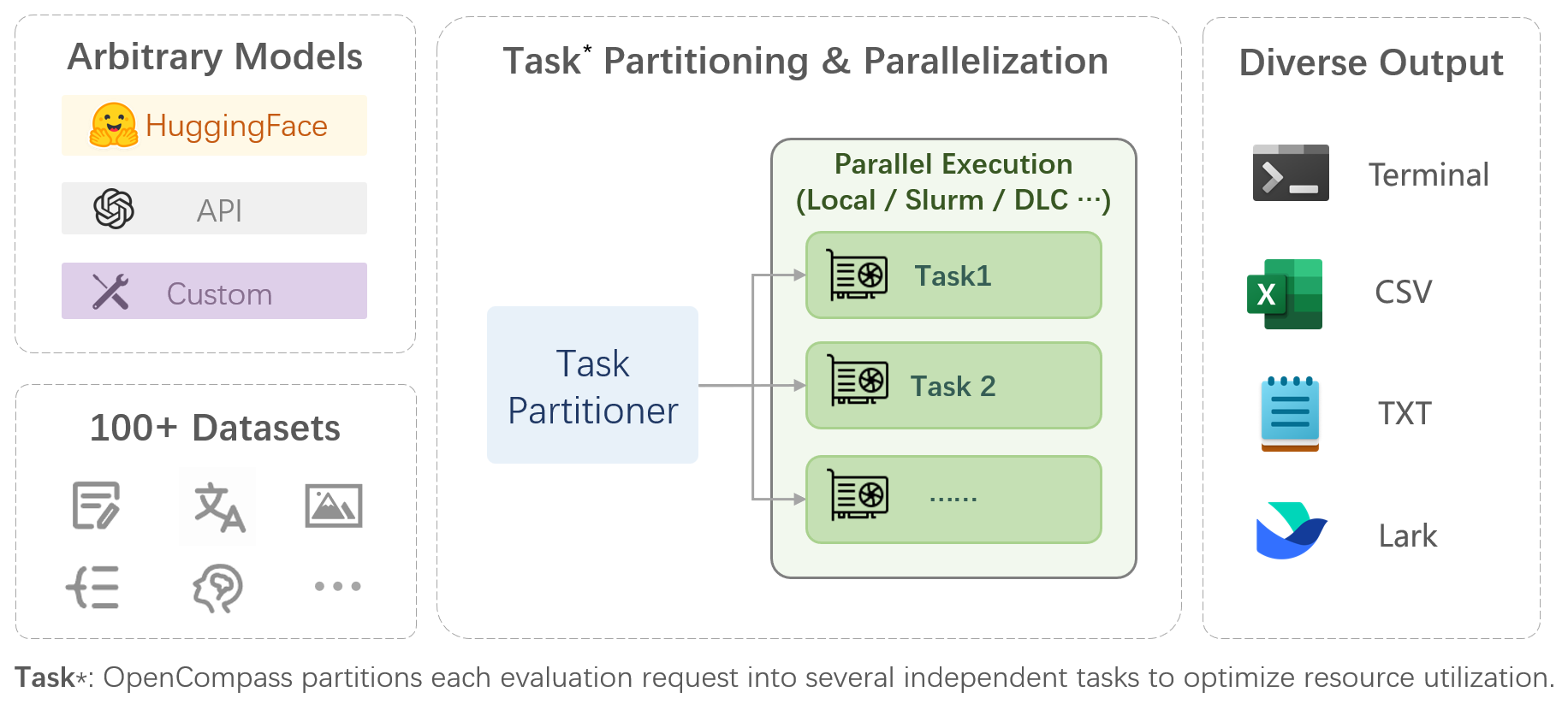
OpenCompass is a one-stop platform for large model evaluation, aiming to provide a fair, open, and reproducible benchmark for large model evaluation. Its main features include:
- **Comprehensive support for models and datasets**: Pre-support for 20+ HuggingFace and API models, a model evaluation scheme of 70+ datasets with about 400,000 questions, comprehensively evaluating the capabilities of the models in five dimensions.
- **Efficient distributed evaluation**: One line command to implement task division and distributed evaluation, completing the full evaluation of billion-scale models in just a few hours.
- **Diversified evaluation paradigms**: Support for zero-shot, few-shot, and chain-of-thought evaluations, combined with standard or dialogue-type prompt templates, to easily stimulate the maximum performance of various models.
- **Modular design with high extensibility**: Want to add new models or datasets, customize an advanced task division strategy, or even support a new cluster management system? Everything about OpenCompass can be easily expanded!
- **Experiment management and reporting mechanism**: Use config files to fully record each experiment, and support real-time reporting of results.
## 📊 Leaderboard
We provide [OpenCompass Leaderboard](https://opencompass.org.cn/rank) for the community to rank all public models and API models. If you would like to join the evaluation, please provide the model repository URL or a standard API interface to the email address `[email protected]`.
<p align="right"><a href="#top">🔝Back to top</a></p>
## 🛠️ Installation
Below are the steps for quick installation and datasets preparation.
### 💻 Environment Setup
#### Open-source Models with GPU
```bash
conda create --name opencompass python=3.10 pytorch torchvision pytorch-cuda -c nvidia -c pytorch -y
conda activate opencompass
git clone https://github.com/open-compass/opencompass opencompass
cd opencompass
pip install -e .
```
#### API Models with CPU-only
```bash
conda create -n opencompass python=3.10 pytorch torchvision torchaudio cpuonly -c pytorch -y
conda activate opencompass
git clone https://github.com/open-compass/opencompass opencompass
cd opencompass
pip install -e .
# also please install requiresments packages via `pip install -r requirements/api.txt` for API models if needed.
```
### 📂 Data Preparation
```bash
# Download dataset to data/ folder
wget https://github.com/open-compass/opencompass/releases/download/0.2.2.rc1/OpenCompassData-core-20240207.zip
unzip OpenCompassData-core-20240207.zip
```
Some third-party features, like Humaneval and Llama, may require additional steps to work properly, for detailed steps please refer to the [Installation Guide](https://opencompass.readthedocs.io/en/latest/get_started/installation.html).
<p align="right"><a href="#top">🔝Back to top</a></p>
## 🏗️ ️Evaluation
After ensuring that OpenCompass is installed correctly according to the above steps and the datasets are prepared, you can evaluate the performance of the LLaMA-7b model on the MMLU and C-Eval datasets using the following command:
```bash
python run.py --models hf_llama_7b --datasets mmlu_ppl ceval_ppl
```
OpenCompass has predefined configurations for many models and datasets. You can list all available model and dataset configurations using the [tools](./docs/en/tools.md#list-configs).
```bash
# List all configurations
python tools/list_configs.py
# List all configurations related to llama and mmlu
python tools/list_configs.py llama mmlu
```
You can also evaluate other HuggingFace models via command line. Taking LLaMA-7b as an example:
```bash
python run.py --datasets ceval_ppl mmlu_ppl \
--hf-path huggyllama/llama-7b \ # HuggingFace model path
--model-kwargs device_map='auto' \ # Arguments for model construction
--tokenizer-kwargs padding_side='left' truncation='left' use_fast=False \ # Arguments for tokenizer construction
--max-out-len 100 \ # Maximum number of tokens generated
--max-seq-len 2048 \ # Maximum sequence length the model can accept
--batch-size 8 \ # Batch size
--no-batch-padding \ # Don't enable batch padding, infer through for loop to avoid performance loss
--num-gpus 1 # Number of minimum required GPUs
```
> **Note**<br />
> To run the command above, you will need to remove the comments starting from `# ` first.
Through the command line or configuration files, OpenCompass also supports evaluating APIs or custom models, as well as more diversified evaluation strategies. Please read the [Quick Start](https://opencompass.readthedocs.io/en/latest/get_started/quick_start.html) to learn how to run an evaluation task.
<p align="right"><a href="#top">🔝Back to top</a></p>
## 📖 Dataset Support
<table align="center">
<tbody>
<tr align="center" valign="bottom">
<td>
<b>Language</b>
</td>
<td>
<b>Knowledge</b>
</td>
<td>
<b>Reasoning</b>
</td>
<td>
<b>Examination</b>
</td>
</tr>
<tr valign="top">
<td>
<details open>
<summary><b>Word Definition</b></summary>
- WiC
- SummEdits
</details>
<details open>
<summary><b>Idiom Learning</b></summary>
- CHID
</details>
<details open>
<summary><b>Semantic Similarity</b></summary>
- AFQMC
- BUSTM
</details>
<details open>
<summary><b>Coreference Resolution</b></summary>
- CLUEWSC
- WSC
- WinoGrande
</details>
<details open>
<summary><b>Translation</b></summary>
- Flores
- IWSLT2017
</details>
<details open>
<summary><b>Multi-language Question Answering</b></summary>
- TyDi-QA
- XCOPA
</details>
<details open>
<summary><b>Multi-language Summary</b></summary>
- XLSum
</details>
</td>
<td>
<details open>
<summary><b>Knowledge Question Answering</b></summary>
- BoolQ
- CommonSenseQA
- NaturalQuestions
- TriviaQA
</details>
</td>
<td>
<details open>
<summary><b>Textual Entailment</b></summary>
- CMNLI
- OCNLI
- OCNLI_FC
- AX-b
- AX-g
- CB
- RTE
- ANLI
</details>
<details open>
<summary><b>Commonsense Reasoning</b></summary>
- StoryCloze
- COPA
- ReCoRD
- HellaSwag
- PIQA
- SIQA
</details>
<details open>
<summary><b>Mathematical Reasoning</b></summary>
- MATH
- GSM8K
</details>
<details open>
<summary><b>Theorem Application</b></summary>
- TheoremQA
- StrategyQA
- SciBench
</details>
<details open>
<summary><b>Comprehensive Reasoning</b></summary>
- BBH
</details>
</td>
<td>
<details open>
<summary><b>Junior High, High School, University, Professional Examinations</b></summary>
- C-Eval
- AGIEval
- MMLU
- GAOKAO-Bench
- CMMLU
- ARC
- Xiezhi
</details>
<details open>
<summary><b>Medical Examinations</b></summary>
- CMB
</details>
</td>
</tr>
</td>
</tr>
</tbody>
<tbody>
<tr align="center" valign="bottom">
<td>
<b>Understanding</b>
</td>
<td>
<b>Long Context</b>
</td>
<td>
<b>Safety</b>
</td>
<td>
<b>Code</b>
</td>
</tr>
<tr valign="top">
<td>
<details open>
<summary><b>Reading Comprehension</b></summary>
- C3
- CMRC
- DRCD
- MultiRC
- RACE
- DROP
- OpenBookQA
- SQuAD2.0
</details>
<details open>
<summary><b>Content Summary</b></summary>
- CSL
- LCSTS
- XSum
- SummScreen
</details>
<details open>
<summary><b>Content Analysis</b></summary>
- EPRSTMT
- LAMBADA
- TNEWS
</details>
</td>
<td>
<details open>
<summary><b>Long Context Understanding</b></summary>
- LEval
- LongBench
- GovReports
- NarrativeQA
- Qasper
</details>
</td>
<td>
<details open>
<summary><b>Safety</b></summary>
- CivilComments
- CrowsPairs
- CValues
- JigsawMultilingual
- TruthfulQA
</details>
<details open>
<summary><b>Robustness</b></summary>
- AdvGLUE
</details>
</td>
<td>
<details open>
<summary><b>Code</b></summary>
- HumanEval
- HumanEvalX
- MBPP
- APPs
- DS1000
</details>
</td>
</tr>
</td>
</tr>
</tbody>
</table>
## OpenCompass Ecosystem
<p align="right"><a href="#top">🔝Back to top</a></p>
## 📖 Model Support
<table align="center">
<tbody>
<tr align="center" valign="bottom">
<td>
<b>Open-source Models</b>
</td>
<td>
<b>API Models</b>
</td>
<!-- <td>
<b>Custom Models</b>
</td> -->
</tr>
<tr valign="top">
<td>
- [InternLM](https://github.com/InternLM/InternLM)
- [LLaMA](https://github.com/facebookresearch/llama)
- [Vicuna](https://github.com/lm-sys/FastChat)
- [Alpaca](https://github.com/tatsu-lab/stanford_alpaca)
- [Baichuan](https://github.com/baichuan-inc)
- [WizardLM](https://github.com/nlpxucan/WizardLM)
- [ChatGLM2](https://github.com/THUDM/ChatGLM2-6B)
- [ChatGLM3](https://github.com/THUDM/ChatGLM3-6B)
- [TigerBot](https://github.com/TigerResearch/TigerBot)
- [Qwen](https://github.com/QwenLM/Qwen)
- [BlueLM](https://github.com/vivo-ai-lab/BlueLM)
- ...
</td>
<td>
- OpenAI
- Claude
- ZhipuAI(ChatGLM)
- Baichuan
- ByteDance(YunQue)
- Huawei(PanGu)
- 360
- Baidu(ERNIEBot)
- MiniMax(ABAB-Chat)
- SenseTime(nova)
- Xunfei(Spark)
- ……
</td>
</tr>
</tbody>
</table>
<p align="right"><a href="#top">🔝Back to top</a></p>
## 🔜 Roadmap
- [ ] Subjective Evaluation
- [ ] Release CompassAreana
- [ ] Subjective evaluation dataset.
- [x] Long-context
- [ ] Long-context evaluation with extensive datasets.
- [ ] Long-context leaderboard.
- [ ] Coding
- [ ] Coding evaluation leaderboard.
- [x] Non-python language evaluation service.
- [ ] Agent
- [ ] Support various agenet framework.
- [ ] Evaluation of tool use of the LLMs.
- [x] Robustness
- [x] Support various attack method
## 👷♂️ Contributing
We appreciate all contributions to improving OpenCompass. Please refer to the [contributing guideline](https://opencompass.readthedocs.io/en/latest/notes/contribution_guide.html) for the best practice.
## 🤝 Acknowledgements
Some code in this project is cited and modified from [OpenICL](https://github.com/Shark-NLP/OpenICL).
Some datasets and prompt implementations are modified from [chain-of-thought-hub](https://github.com/FranxYao/chain-of-thought-hub) and [instruct-eval](https://github.com/declare-lab/instruct-eval).
## 🖊️ Citation
```bibtex
@misc{2023opencompass,
title={OpenCompass: A Universal Evaluation Platform for Foundation Models},
author={OpenCompass Contributors},
howpublished = {\url{https://github.com/open-compass/opencompass}},
year={2023}
}
```
<p align="right"><a href="#top">🔝Back to top</a></p>
|