SwissBERT is a masked language model for processing Switzerland-related text. It has been trained on more than 21 million Swiss news articles retrieved from Swissdox@LiRI.
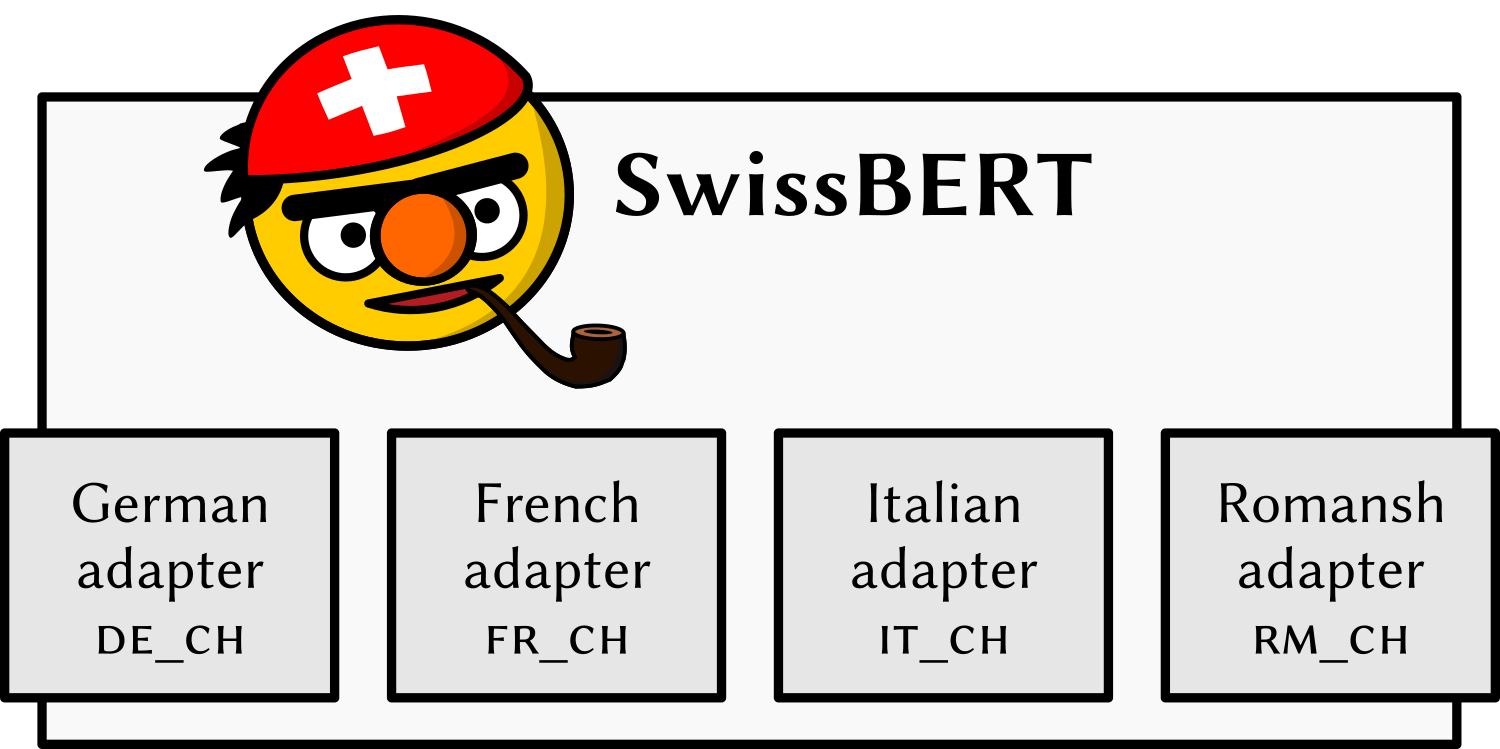
SwissBERT is based on X-MOD, which has been pre-trained with language adapters in 81 languages. For SwissBERT we trained adapters for the national languages of Switzerland – German, French, Italian, and Romansh Grischun. In addition, we used a Switzerland-specific subword vocabulary.
The pre-training code and usage examples are available here. We also release a version that was fine-tuned on named entity recognition (NER): https://huggingface.co/ZurichNLP/swissbert-ner
The SwissBERT model is described in the following paper: https://aclanthology.org/2023.swisstext-1.6/ (Vamvas et al., SwissText 2023).
Update 2024-01: Support for Swiss German
We added a Swiss German adapter to the model. More information in our post or in the paper (Vamvas et al., 2024).
Languages
SwissBERT contains the following language adapters:
lang_id (Adapter index) | Language code | Language |
---|---|---|
0 | de_CH |
Swiss Standard German |
1 | fr_CH |
French |
2 | it_CH |
Italian |
3 | rm_CH |
Romansh Grischun |
4 | gsw |
Swiss German |
License
Attribution-NonCommercial 4.0 International (CC BY-NC 4.0).
Usage (masked language modeling)
from transformers import pipeline
fill_mask = pipeline(model="ZurichNLP/swissbert")
German example
fill_mask.model.set_default_language("de_CH")
fill_mask("Der schönste Kanton der Schweiz ist <mask>.")
Output:
[{'score': 0.1373230218887329,
'token': 331,
'token_str': 'Zürich',
'sequence': 'Der schönste Kanton der Schweiz ist Zürich.'},
{'score': 0.08464793860912323,
'token': 5903,
'token_str': 'Appenzell',
'sequence': 'Der schönste Kanton der Schweiz ist Appenzell.'},
{'score': 0.08250337839126587,
'token': 10800,
'token_str': 'Graubünden',
'sequence': 'Der schönste Kanton der Schweiz ist Graubünden.'},
...]
French example
fill_mask.model.set_default_language("fr_CH")
fill_mask("Je m'appelle <mask> Federer.")
Output:
[{'score': 0.9943694472312927,
'token': 1371,
'token_str': 'Roger',
'sequence': "Je m'appelle Roger Federer."},
...]
Bias, Risks, and Limitations
- SwissBERT is mainly intended for tagging tokens in written text (e.g., named entity recognition, part-of-speech tagging), text classification, and the encoding of words, sentences or documents into fixed-size embeddings. SwissBERT is not designed for generating text.
- The model was adapted on written news articles and might perform worse on other domains or language varieties.
- While we have removed many author bylines, we did not anonymize the pre-training corpus. The model might have memorized information that has been described in the news but is no longer in the public interest.
Training Details
- Training data: German, French, Italian and Romansh documents in the Swissdox@LiRI database, until 2022.
- Training procedure: Masked language modeling
The Swiss German adapter was trained on the following two datasets of written Swiss German:
- SwissCrawl (Linder et al., LREC 2020), a collection of Swiss German web text (forum discussions, social media).
- A custom dataset of Swiss German tweets
Environmental Impact
- Hardware type: RTX 2080 Ti.
- Hours used: 10 epochs × 18 hours × 8 devices = 1440 hours
- Site: Zurich, Switzerland.
- Energy source: 100% hydropower (source)
- Carbon efficiency: 0.0016 kg CO2e/kWh (source)
- Carbon emitted: 0.6 kg CO2e (source)
Citations
@inproceedings{vamvas-etal-2023-swissbert,
title = "{S}wiss{BERT}: The Multilingual Language Model for {S}witzerland",
author = {Vamvas, Jannis and
Gra{\"e}n, Johannes and
Sennrich, Rico},
editor = {Ghorbel, Hatem and
Sokhn, Maria and
Cieliebak, Mark and
H{\"u}rlimann, Manuela and
de Salis, Emmanuel and
Guerne, Jonathan},
booktitle = "Proceedings of the 8th edition of the Swiss Text Analytics Conference",
month = jun,
year = "2023",
address = "Neuchatel, Switzerland",
publisher = "Association for Computational Linguistics",
url = "https://aclanthology.org/2023.swisstext-1.6",
pages = "54--69",
}
Swiss German adapter:
@inproceedings{vamvas-etal-2024-modular,
title={Modular Adaptation of Multilingual Encoders to Written Swiss German Dialect},
author={Jannis Vamvas and No{\"e}mi Aepli and Rico Sennrich},
booktitle={First Workshop on Modular and Open Multilingual NLP},
year={2024},
}
- Downloads last month
- 254