MobileCLIP: Fast Image-Text Models through Multi-Modal Reinforced Training
MobileCLIP was introduced in MobileCLIP: Fast Image-Text Models through Multi-Modal Reinforced Training
(CVPR 2024), by Pavan Kumar Anasosalu Vasu, Hadi Pouransari, Fartash Faghri, Raviteja Vemulapalli, Oncel Tuzel.
This repository contains the MobileCLIP-S1 checkpoint.
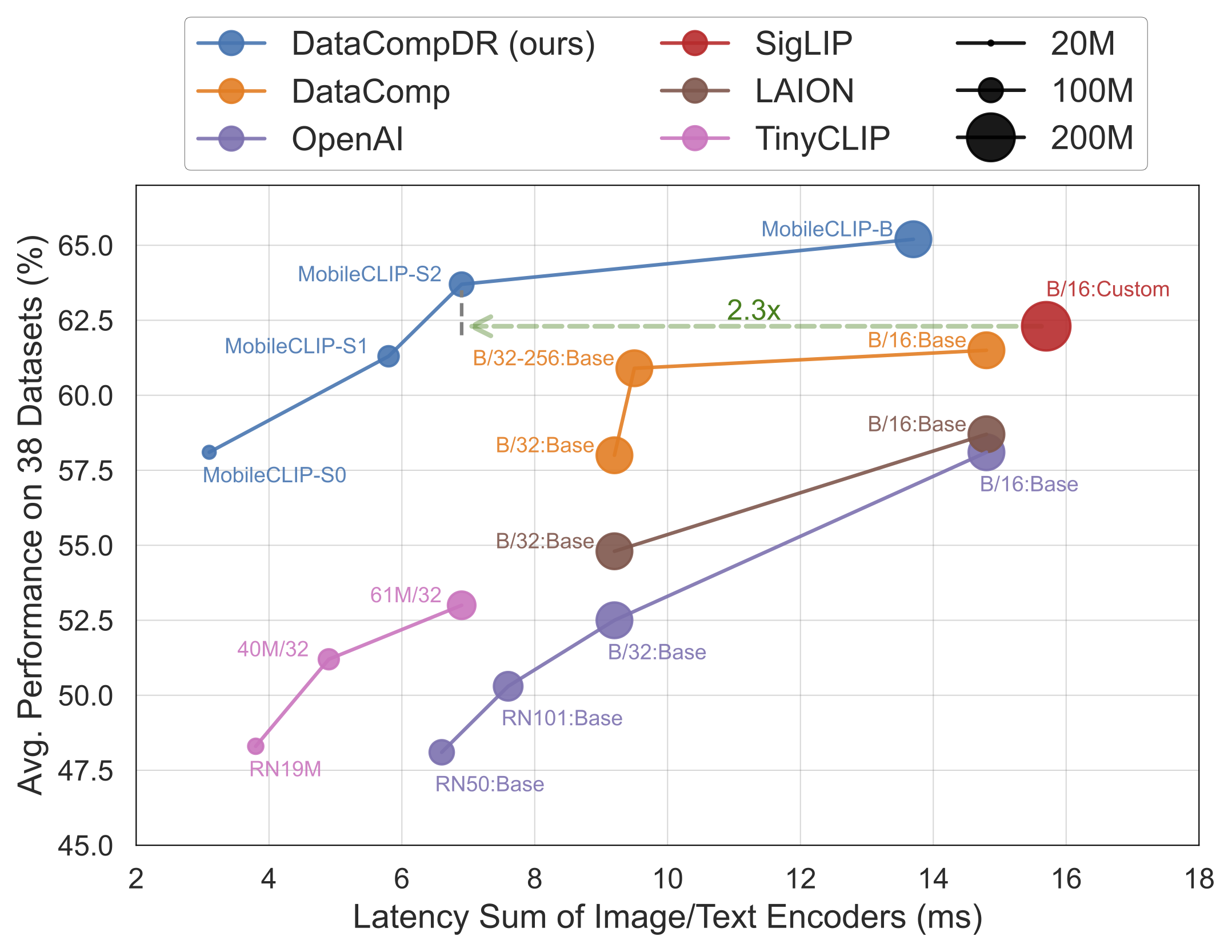
Highlights
- Our smallest variant
MobileCLIP-S0
obtains similar zero-shot performance as OpenAI's ViT-B/16 model while being 4.8x faster and 2.8x smaller.
MobileCLIP-S2
obtains better avg zero-shot performance than SigLIP's ViT-B/16 model while being 2.3x faster and 2.1x smaller, and trained with 3x less seen samples.
MobileCLIP-B
(LT) attains zero-shot ImageNet performance of 77.2% which is significantly better than recent works like DFN and SigLIP with similar architectures or even OpenAI's ViT-L/14@336.
Checkpoints
Model |
# Seen Samples (B) |
# Params (M) (img + txt) |
Latency (ms) (img + txt) |
IN-1k Zero-Shot Top-1 Acc. (%) |
Avg. Perf. (%) on 38 datasets |
MobileCLIP-S0 |
13 |
11.4 + 42.4 |
1.5 + 1.6 |
67.8 |
58.1 |
MobileCLIP-S1 |
13 |
21.5 + 63.4 |
2.5 + 3.3 |
72.6 |
61.3 |
MobileCLIP-S2 |
13 |
35.7 + 63.4 |
3.6 + 3.3 |
74.4 |
63.7 |
MobileCLIP-B |
13 |
86.3 + 63.4 |
10.4 + 3.3 |
76.8 |
65.2 |
MobileCLIP-B (LT) |
36 |
86.3 + 63.4 |
10.4 + 3.3 |
77.2 |
65.8 |
How to Use
First, download the desired checkpoint visiting one of the links in the table above, then click the Files and versions
tab, and download the PyTorch checkpoint.
For programmatic downloading, if you have huggingface_hub
installed, you can also run:
huggingface-cli download pcuenq/MobileCLIP-S1
Then, install ml-mobileclip
by following the instructions in the repo. It uses an API similar to open_clip
's.
You can run inference with a code snippet like the following:
import torch
from PIL import Image
import mobileclip
model, _, preprocess = mobileclip.create_model_and_transforms('mobileclip_s1', pretrained='/path/to/mobileclip_s1.pt')
tokenizer = mobileclip.get_tokenizer('mobileclip_s1')
image = preprocess(Image.open("docs/fig_accuracy_latency.png").convert('RGB')).unsqueeze(0)
text = tokenizer(["a diagram", "a dog", "a cat"])
with torch.no_grad(), torch.cuda.amp.autocast():
image_features = model.encode_image(image)
text_features = model.encode_text(text)
image_features /= image_features.norm(dim=-1, keepdim=True)
text_features /= text_features.norm(dim=-1, keepdim=True)
text_probs = (100.0 * image_features @ text_features.T).softmax(dim=-1)
print("Label probs:", text_probs)