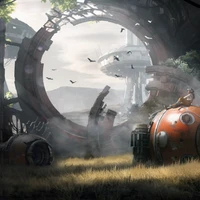
Acknowledge license to accept the repository
This repository is publicly accessible, but you have to accept the conditions to access its files and content.
北京智源人工智能研究院(以下简称“我们”或“研究院”)通过BAAI DataHub(data.baai.ac.cn)和COIG-PC HuggingFace仓库(https://huggingface.co/datasets/BAAI/COIG-PC)向您提供开源数据集(以下或称“数据集”),您可通过下载的方式获取您所需的开源数据集,并在遵守各原始数据集使用规则前提下,基于学习、研究、商业等目的使用相关数据集。
在您获取(包括但不限于访问、下载、复制、传播、使用等处理数据集的行为)开源数据集前,您应认真阅读并理解本《COIG-PC开源数据集使用须知与免责声明》(以下简称“本声明”)。一旦您获取开源数据集,无论您的获取方式为何,您的获取行为均将被视为对本声明全部内容的认可。
1. 平台的所有权与运营权
您应充分了解并知悉,BAAI DataHub和COIG-PC HuggingFace仓库(包括当前版本及全部历史版本)的所有权与运营权归智源人工智能研究院所有,智源人工智能研究院对本平台/本工具及开源数据集开放计划拥有最终解释权和决定权。
您知悉并理解,基于相关法律法规更新和完善以及我们需履行法律合规义务的客观变化,我们保留对本平台/本工具进行不定时更新、维护,或者中止乃至永久终止提供本平台/本工具服务的权利。我们将在合理时间内将可能发生前述情形通过公告或邮件等合理方式告知您,您应当及时做好相应的调整和安排,但我们不因发生前述任何情形对您造成的任何损失承担任何责任。
2. 开源数据集的权利主张
为了便于您基于学习、研究、商业的目的开展数据集获取、使用等活动,我们对第三方原始数据集进行了必要的格式整合、数据清洗、标注、分类、注释等相关处理环节,形成可供本平台/本工具用户使用的开源数据集。
您知悉并理解,我们不对开源数据集主张知识产权中的相关财产性权利,因此我们亦无相应义务对开源数据集可能存在的知识产权进行主动识别和保护,但这不意味着我们放弃开源数据集主张署名权、发表权、修改权和保护作品完整权(如有)等人身性权利。而原始数据集可能存在的知识产权及相应合法权益由原权利人享有。
此外,向您开放和使用经合理编排、加工和处理后的开源数据集,并不意味着我们对原始数据集知识产权、信息内容等真实、准确或无争议的认可,您应当自行筛选、仔细甄别,使用经您选择的开源数据集。您知悉并同意,研究院对您自行选择使用的原始数据集不负有任何无缺陷或无瑕疵的承诺义务或担保责任。
3. 开源数据集的使用限制
您使用数据集不得侵害我们或任何第三方的合法权益(包括但不限于著作权、专利权、商标权等知识产权与其他权益)。
获取开源数据集后,您应确保对开源数据集的使用不超过原始数据集的权利人以公示或协议等形式明确规定的使用规则,包括原始数据的使用范围、目的和合法用途等。我们在此善意地提请您留意,如您对开源数据集的使用超出原始数据集的原定使用范围及用途,您可能面临侵犯原始数据集权利人的合法权益例如知识产权的风险,并可能承担相应的法律责任。
4. 个人信息保护
基于技术限制及开源数据集的公益性质等客观原因,我们无法保证开源数据集中不包含任何个人信息,我们不对开源数据集中可能涉及的个人信息承担任何法律责任。
如开源数据集涉及个人信息,我们不对您使用开源数据集可能涉及的任何个人信息处理行为承担法律责任。我们在此善意地提请您留意,您应依据《个人信息保护法》等相关法律法规的规定处理个人信息。
为了维护信息主体的合法权益、履行可能适用的法律、行政法规的规定,如您在使用开源数据集的过程中发现涉及或者可能涉及个人信息的内容,应立即停止对数据集中涉及个人信息部分的使用,并及时通过“6. 投诉与通知”中载明的联系我们。
5. 信息内容管理
我们不对开源数据集可能涉及的违法与不良信息承担任何法律责任。
如您在使用开源数据集的过程中发现开源数据集涉及或者可能涉及任何违法与不良信息,您应立即停止对数据集中涉及违法与不良信息部分的使用,并及时通过“6. 投诉与通知”中载明的联系我们。
6. 投诉与通知
如您认为开源数据集侵犯了您的合法权益,您可通过010-50955974联系我们,我们会及时依法处理您的主张与投诉。
为了处理您的主张和投诉,我们可能需要您提供联系方式、侵权证明材料以及身份证明等材料。请注意,如果您恶意投诉或陈述失实,您将承担由此造成的全部法律责任(包括但不限于合理的费用赔偿等)。
7. 责任声明
您理解并同意,基于开源数据集的性质,数据集中可能包含来自不同来源和贡献者的数据,其真实性、准确性、客观性等可能会有所差异,我们无法对任何数据集的可用性、可靠性等做出任何承诺。
在任何情况下,我们不对开源数据集可能存在的个人信息侵权、违法与不良信息传播、知识产权侵权等任何风险承担任何法律责任。
在任何情况下,我们不对您因开源数据集遭受的或与之相关的任何损失(包括但不限于直接损失、间接损失以及可得利益损失等)承担任何法律责任。
8. 其他
开源数据集处于不断发展、变化的阶段,我们可能因业务发展、第三方合作、法律法规变动等原因更新、调整所提供的开源数据集范围,或中止、暂停、终止开源数据集提供业务。
Log in or Sign Up to review the conditions and access this dataset content.
COIG Prompt Collection
License
Default Licensing for Sub-Datasets Without Specific License Declaration: In instances where sub-datasets within the COIG-PC Dataset do not have a specific license declaration, the Apache License 2.0 (Apache-2.0) will be the applicable licensing terms by default.
Precedence of Declared Licensing for Sub-Datasets: For any sub-dataset within the COIG-PC Dataset that has an explicitly declared license, the terms and conditions of the declared license shall take precedence and govern the usage of that particular sub-dataset.
Users and developers utilizing the COIG-PC Dataset must ensure compliance with the licensing terms as outlined above. It is imperative to review and adhere to the specified licensing conditions of each sub-dataset, as they may vary.
What is COIG-PC?
The COIG-PC Dataset is a meticulously curated and comprehensive collection of Chinese tasks and data, designed to facilitate the fine-tuning and optimization of language models for Chinese natural language processing (NLP). The dataset aims to provide researchers and developers with a rich set of resources to improve the capabilities of language models in handling Chinese text, which can be utilized in various fields such as text generation, information extraction, sentiment analysis, machine translation, among others.
If you think COIG-PC is too huge, please refer to COIG-PC-Lite which is a subset of COIG-PC with only 200 samples from each task file.
Why COIG-PC?
The COIG-PC Dataset is an invaluable resource for the domain of natural language processing (NLP) for various compelling reasons:
Addressing Language Complexity: Chinese is known for its intricacy, with a vast array of characters and diverse grammatical structures. A specialized dataset like COIG-PC, which is tailored for the Chinese language, is essential to adequately address these complexities during model training.
Comprehensive Data Aggregation: The COIG-PC Dataset is a result of an extensive effort in integrating almost all available Chinese datasets in the market. This comprehensive aggregation makes it one of the most exhaustive collections for Chinese NLP.
Data Deduplication and Normalization: The COIG-PC Dataset underwent rigorous manual processing to eliminate duplicate data and perform normalization. This ensures that the dataset is free from redundancy, and the data is consistent and well-structured, making it more user-friendly and efficient for model training.
Fine-tuning and Optimization: The dataset’s instruction-based phrasing facilitates better fine-tuning and optimization of language models. This structure allows models to better understand and execute tasks, which is particularly beneficial in improving performance on unseen or novel tasks.
The COIG-PC Dataset, with its comprehensive aggregation, meticulous selection, deduplication, and normalization of data, stands as an unmatched resource for training and optimizing language models tailored for the Chinese language and culture. It addresses the unique challenges of Chinese language processing and serves as a catalyst for advancements in Chinese NLP.
Who builds COIG-PC?
The bedrock of COIG-PC is anchored in the dataset furnished by stardust.ai, which comprises an aggregation of data collected from the Internet.
And COIG-PC is the result of a collaborative effort involving engineers and experts from over twenty distinguished universities both domestically and internationally. Due to space constraints, it is not feasible to list all of them; however, the following are a few notable institutions among the collaborators:
- Beijing Academy of Artificial Intelligence, China
- Peking University, China
- The Hong Kong University of Science and Technology (HKUST), China
- The University of Waterloo, Canada
- The University of Sheffield, United Kingdom
- Beijing University of Posts and Telecommunications, China
- Multimodal Art Projection
- stardust.ai, China
- LinkSoul.AI, China
For the detailed list of engineers involved in the creation and refinement of COIG-PC, please refer to the paper that will be published subsequently. This paper will provide in-depth information regarding the contributions and the specifics of the dataset’s development process.
How to use COIG-PC?
COIG-PC is structured in a .jsonl file format. Each line in the file represents a single data record and is structured in JSON (JavaScript Object Notation) format. Below is a breakdown of the elements within each line:
instruction: This is a text string that provides the instruction for the task. For example, it might tell the model what to do with the input data.
input: This is the input data that the model needs to process. In the context of translation, it would be the text that needs to be translated.
output: This contains the expected output data after processing the input. In the context of translation, it would be the translated text.
split: Indicates the official split of the original dataset, which is used to categorize data for different phases of model training and evaluation. It can be 'train', 'test', 'valid', etc.
task_type: Contains major and minor categories for the dataset. Major categories are broader, while minor categories can be more specific subcategories.
domain: Indicates the domain or field to which the data belongs.
other: This field can contain additional information or metadata regarding the data record. If there is no additional information, it may be set to null.
Example
Here is an example of how a line in the COIG-PC dataset might be structured:
{
"instruction": "请把下面的中文句子翻译成英文",
"input": "我爱你。",
"output": "I love you.",
"split": "train",
"task_type": {
"major": ["翻译"],
"minor": ["翻译", "中译英"]
},
"domain": ["通用"],
"other": null
}
In this example: instruction tells the model to translate the following Chinese sentence into English. input contains the Chinese text "我爱你" which means "I love you". output contains the expected translation in English: "I love you". split indicates that this data record is part of the training set. task_type specifies that the major category is "Translation" and the minor categories are "Translation" and "Chinese to English". domain specifies that this data record belongs to the general domain. other is set to null as there is no additional information for this data record.
Update: Oct. 8, 2023
- v1.3: Upload all splits to the main branch as arrow datasets. All jsonl files are stored in the raw_json branch now. Remove 152 task files. Add 10 task files. In total, 275 task files updated.
- v1.2: Delete 31 bad task files. Update 99 task files. Rename 2 task files. Add 3 new task files. COIG-PC now has 3339 tasks in total.
- v1.1: Fix 00040-001-000 and 00050-003-000, ignore 00930 and 01373.
- v1.0: First version for arXiv paper.
- v0.6: Upload 28 new tasks. COIG-PC now has 3367 tasks in total.
- v0.5: Upload 202 new tasks. COIG-PC now has 3339 tasks in total.
- v0.4: Upload 1049 new tasks. COIG-PC now has 3137 tasks in total.
- v0.3: Upload 1139 new tasks. COIG-PC now has 2088 tasks in total.
- v0.2: Upload 422 new tasks. COIG-PC now has 949 tasks in total. Add "TopSamplenumPerTask" split where only "Samplenum" samples are used from each task.
- v0.1: Upload 527 tasks.
COIG-PC Citation
If you want to cite COIG-PC dataset, you could use this:
@misc{zhang2023chinese,
title={Chinese Open Instruction Generalist: A Preliminary Release},
author={Ge Zhang and Yemin Shi and Ruibo Liu and Ruibin Yuan and Yizhi Li and Siwei Dong and Yu Shu and Zhaoqun Li and Zekun Wang and Chenghua Lin and Wenhao Huang and Jie Fu},
year={2023},
eprint={2304.07987},
archivePrefix={arXiv},
primaryClass={cs.CL}
}
Contact Us
To contact us feel free to create an Issue in this repository.
- Downloads last month
- 10
Models trained or fine-tuned on BAAI/COIG-PC
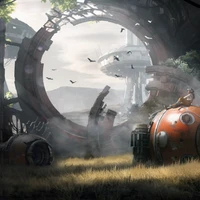