deberta-v3-base-injection
This model is a fine-tuned version of microsoft/deberta-v3-base on the prompt-injection dataset. It achieves the following results on the evaluation set:
- Loss: 0.0673
- Accuracy: 0.9914
Model description
This model detects prompt injection attempts and classifies them as "INJECTION". Legitimate requests are classified as "LEGIT". The dataset assumes that legitimate requests are either all sorts of questions of key word searches.
Intended uses & limitations
If you are using this model to secure your system and it is overly "trigger-happy" to classify requests as injections, consider collecting legitimate examples and retraining the model with the promp-injection dataset.
Training and evaluation data
Based in the promp-injection dataset.
Training procedure
Training hyperparameters
The following hyperparameters were used during training:
- learning_rate: 2e-05
- train_batch_size: 8
- eval_batch_size: 8
- seed: 42
- optimizer: Adam with betas=(0.9,0.999) and epsilon=1e-08
- lr_scheduler_type: linear
- num_epochs: 3
Training results
Training Loss | Epoch | Step | Validation Loss | Accuracy |
---|---|---|---|---|
No log | 1.0 | 69 | 0.2353 | 0.9741 |
No log | 2.0 | 138 | 0.0894 | 0.9741 |
No log | 3.0 | 207 | 0.0673 | 0.9914 |
Framework versions
- Transformers 4.29.1
- Pytorch 2.0.0+cu118
- Datasets 2.12.0
- Tokenizers 0.13.3
About us
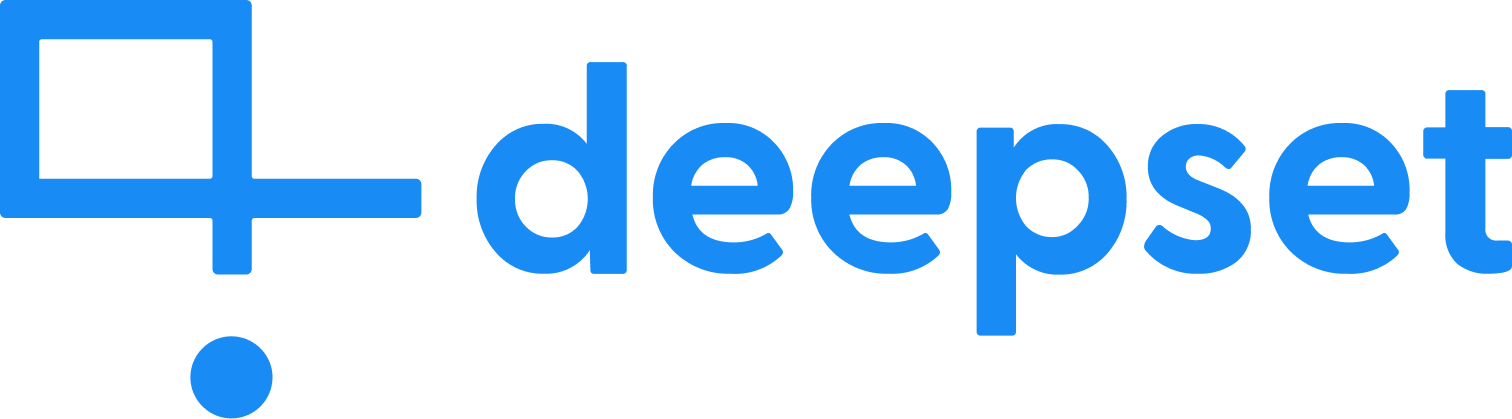
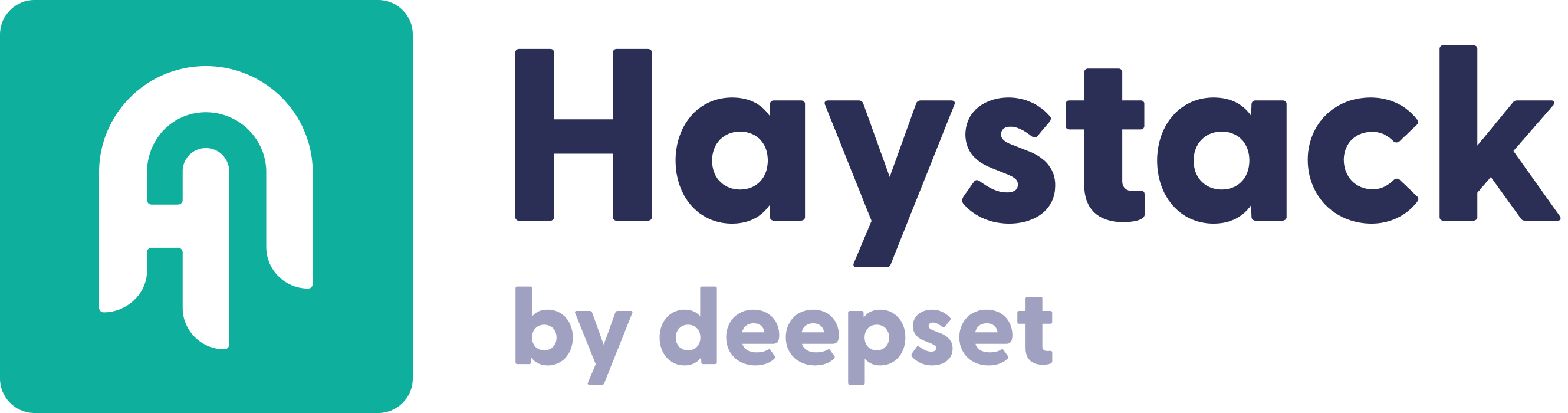
deepset is the company behind the production-ready open-source AI framework Haystack.
Some of our other work:
- Distilled roberta-base-squad2 (aka "tinyroberta-squad2")
- German BERT, GermanQuAD and GermanDPR, German embedding model
- deepset Cloud, deepset Studio
Get in touch and join the Haystack community
For more info on Haystack, visit our GitHub repo and Documentation.
We also have a Discord community open to everyone!
Twitter | LinkedIn | Discord | GitHub Discussions | Website | YouTube
By the way: we're hiring!
- Downloads last month
- 97,953