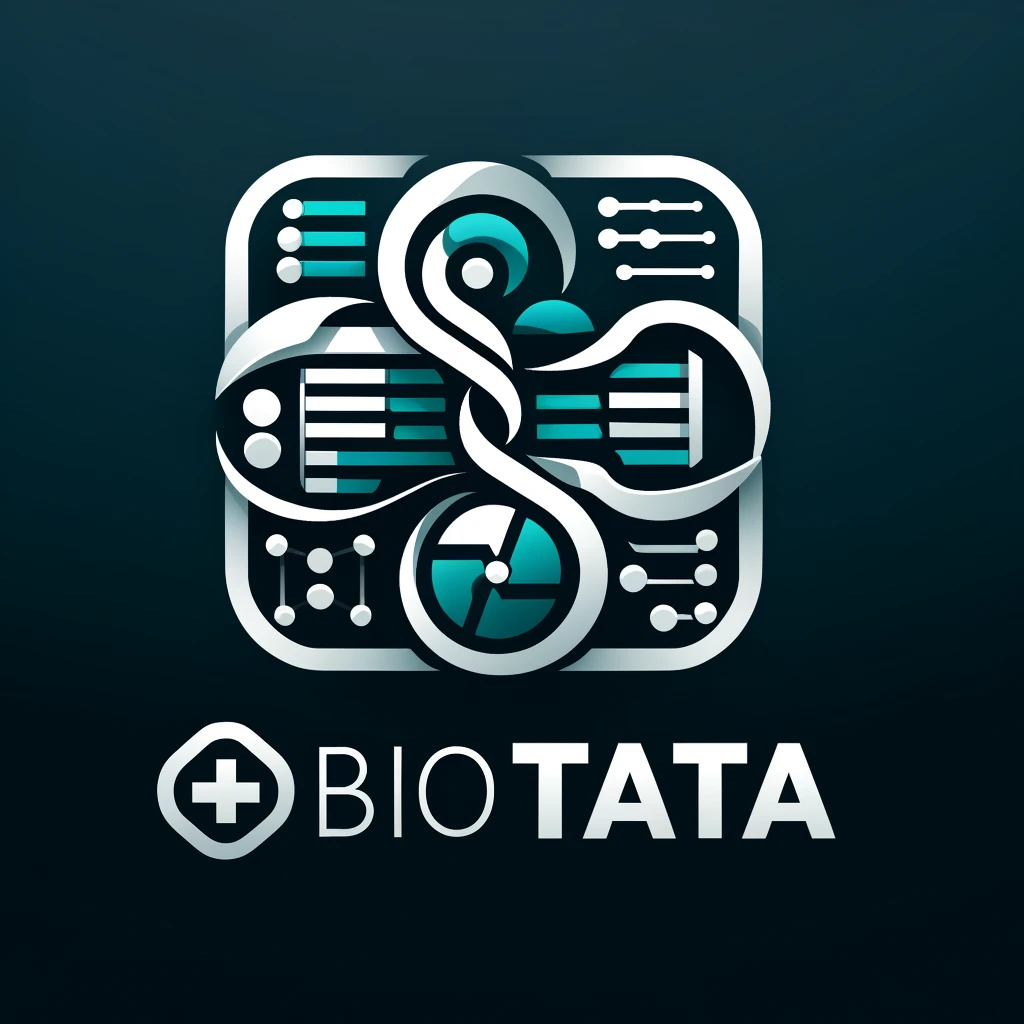
⚠️ STATE OF THE ART ⚠️
Model Card for Model ID
BioTATA 7B V1 is a hybrid model merged between BioMistral 7B Dare and a 4bit QLORA adapter trained on TATA/NO TATA sequences from InstaDeepAI nucleotide_transformer_downstream_tasks dataset (promoters_all subset)
Model Details
Model Description
- Developed by: Karim Akkari (kimou605)
- Model type: FP32
- Language(s) (NLP): English
- License: Apache 2.0
- Finetuned from model: BioMistral 7B Dare
Model Sources
- Repository: kimou605/BioTATA-7B
- Demo: BioTATA 7B Space
How to Get Started with the Model
!pip install transformers
!pip install accelerate
!pip install bitsandbytes
import os
import torch
import transformers
from transformers import (
AutoTokenizer,
AutoModelForCausalLM,
BitsAndBytesConfig,
pipeline
)
model_name='kimou605/BioTATA-7B'
model_config = transformers.AutoConfig.from_pretrained(
model_name,
)
tokenizer = AutoTokenizer.from_pretrained(model_name, trust_remote_code=True)
tokenizer.pad_token = tokenizer.eos_token
tokenizer.padding_side = "right"
# Activate 4-bit precision base model loading
use_4bit = True
# Compute dtype for 4-bit base models
bnb_4bit_compute_dtype = "float16"
# Quantization type (fp4 or nf4)
bnb_4bit_quant_type = "nf4"
# Activate nested quantization for 4-bit base models (double quantization)
use_nested_quant = True
compute_dtype = getattr(torch, bnb_4bit_compute_dtype)
bnb_config = BitsAndBytesConfig(
load_in_4bit=use_4bit,
bnb_4bit_quant_type=bnb_4bit_quant_type,
bnb_4bit_compute_dtype=compute_dtype,
bnb_4bit_use_double_quant=use_nested_quant,
)
model = AutoModelForCausalLM.from_pretrained(
model_name,
quantization_config=bnb_config,
)
pipeline = transformers.pipeline(
"text-generation",
model=model,
torch_dtype=torch.float16,
device_map="auto",
tokenizer=tokenizer,
)
messages = [{"role": "user", "content": "What is TATA"}]
prompt = tokenizer.apply_chat_template(messages, tokenize=False, add_generation_prompt=True)
outputs = pipeline(prompt, max_new_tokens=200, do_sample=True, temperature=0.01, top_k=50, top_p=0.95)
print(outputs[0]["generated_text"])
This will inference the model on 4.8GB Vram
Bias, Risks, and Limitations
This model has been developped to show how can a medical LLM adapt itself to identify sequences as TATA/NO TATA The adapter has been trained on a 53.3k rows for only 1 epoch (due to hardware limitations)
THIS MODEL IS FOR RESEARCH PURPOSES DO NOT USE IN PRODUCTION
Recommendations
Users (both direct and downstream) should be made aware of the risks, biases and limitations of the model.
Training Details
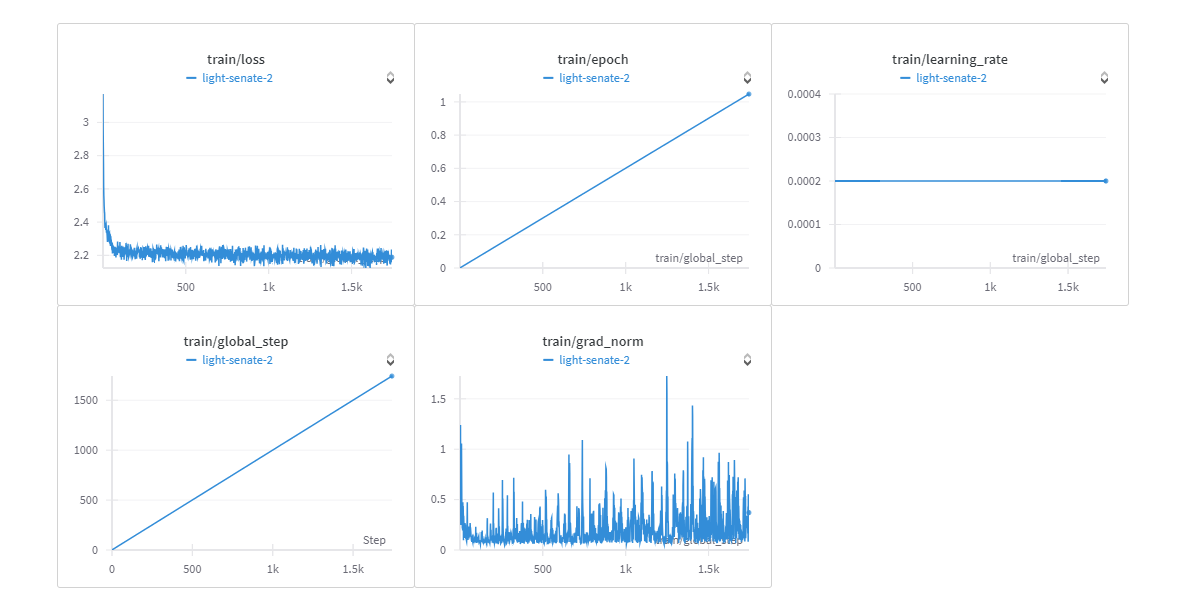
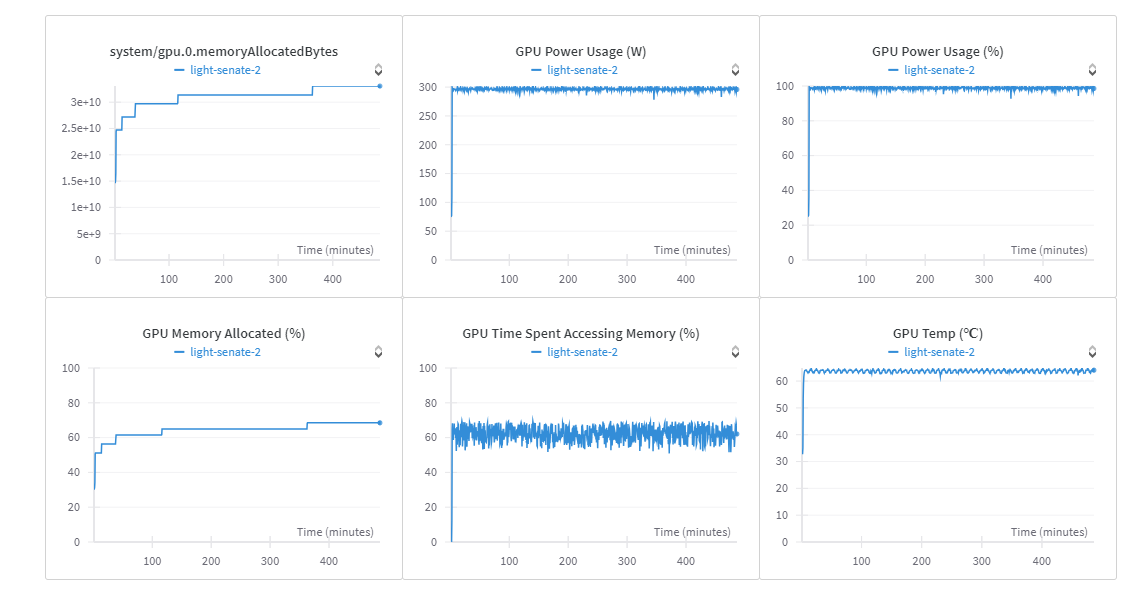
You can view training report here.
Training Data
kimou605/TATA-NOTATA-FineMistral-nucleotide_transformer_downstream_tasks
Training Procedure
Training Hyperparameters
- Training regime: BF16 4bits
Speeds, Sizes, Times
7h/ epoch batch_per_gpu 32 GPU: NVIDIA A40 45GB Vram
Environmental Impact
Carbon emissions can be estimated using the Machine Learning Impact calculator presented in Lacoste et al. (2019).
- Hardware Type: NVIDIA A40
- Hours used: 11H
- Cloud Provider: vast.ai
- Compute Region: Europe
Model Card Contact
Karim Akkari (kimou605)
- Downloads last month
- 13